Open Access
REVIEW
Network biology: A promising approach for drug target identification against neurodevelopmental disorders
Amity Institute of Biotechnology, Amity University, Lucknow, 226028, India
* Corresponding Author: PRACHI SRIVASTAVA. Email:
(This article belongs to the Special Issue: Bioinformatics Study of Diseases)
BIOCELL 2023, 47(8), 1675-1687. https://doi.org/10.32604/biocell.2023.029624
Received 28 February 2023; Accepted 25 April 2023; Issue published 28 August 2023
Abstract
Biological entities are involved in complicated and complex connections; hence, discovering biological information using network biology ideas is critical. In the past few years, network biology has emerged as an integrative and systems-level approach for understanding and interpreting these complex interactions. Biological network analysis is one method for reducing enormous data sets to clinically useful knowledge for disease diagnosis, prognosis, and treatment. The network of biological entities can help us predict drug targets for several diseases. The drug targets identified through the systems biology approach help in targeting the essential biological pathways that contribute to the progression and development of the disease. The novel strategical approach of system biology-assisted pharmacology coupled with computer-aided drug discovery (CADD) can help drugs fight multifactorial diseases efficiently. In the present review, we have summarized the role and application of network biology for not only unfolding the mechanism of complex neurodevelopmental disorders but also identifying important drug targets for diseases like ADHD, Autism, Epilepsy, and Intellectual Disability. Systems biology has emerged as a promising approach to identifying drug targets and aiming for targeted drug discovery for the precise treatment of neurodevelopmental disorders.Keywords
The study of the molecular and genetic basis of diseases has been revolutionized in the past decade by the application of next-generation sequencing technologies and computational approaches. One of the biggest challenges these days is the identification of clinically relevant data from the plethora of data available. The functionality of biomolecules is widely known to be interdependent, forming a complex biomolecular network including protein-protein interaction (PPI), metabolic, signaling and transcription-regulatory networks. For instance, a disease is rarely an outcome of the dysfunction of a single gene; rather, it is a consequence of the malfunction of complex networks that regulate genes, tissues and organ systems (Liu et al., 2020). Network biology has emerged as an important approach to understand and integrate these complex networks and gain knowledge of clinically actionable data for understanding the molecular mechanism of diseases, which can provide biological insights for the diagnosis and treatment of these diseases. PPI network has turned out to be an asset in this context (Furlong, 2013). Based on the results of experimental methods, the human interactome was estimated to contain ~65000 protein interactions (Stumpf et al., 2008). Biological networks have been used to interpret disease mechanisms to study comorbidities, drug-target interactions and discover network-based biomarkers. Thus, network biology can be used to understand complex genotype-phenotype relations of human diseases (Furlong, 2013). In the present paper, we review the literature on network analysis related to neurodevelopmental disorders (NDDs).
NDDs are a class of disorders that affect brain development and functions characterized by the inability to reach cognitive, emotional, and motor developmental milestones; they pose a serious health problem affecting >3% of children worldwide (Parenti et al., 2020). NDDs include attention-deficit/hyperactivity disorder (ADHD), intellectual disability (ID), autism spectrum disorder (ASD) and epilepsy. Studies provide compelling evidence that many disease genes map to a much smaller number of biological subnetworks (Hormozdiari et al., 2015), suggesting the involved genes share a common molecular pathway, resulting in comorbidity of two or more of these disorders. For instance, a combination of ID, ASD and epilepsy is frequently reported in individual patients (Parenti et al., 2020). Network modelling approaches have been applied to explain the above-stated comorbidity and identify candidate genes leading to advancements in the effective treatment of NDDs.
By combining information from genomic data of the disease and the PPI network, network-based techniques may also be utilized to comprehensively evaluate and prioritize disease indications for therapeutic targets (Han et al., 2021). Drug target prioritization and identification are being aided by novel network propagation methods that incorporate heterogeneous biological networks (Ji et al., 2019). As one of the key objectives in drug discovery, the network biology technique for drug-target identification provides a quick and systematic evaluation of both individual and combination drug targets (Han et al., 2021). In the present review, we also discuss the application of network biology for the identification of drug-targets against neuro-developmental diseases.
Several types of biological networks include protein interaction networks, isoform-isoform networks, genetic interaction networks, metabolic networks, brain networks and drug-target networks. Fig. 1 depicts the various types of biological networks.
Figure 1: Different types of biological networks that can be studied through network biology.
Protein-protein interaction network
PPI depicts the relationships between two or more proteins characterized by biochemical interactions in cells. The nodes represent the proteins, and the edges explain the interaction between them. The protein interaction network (PIN) are a valuable tool for the study of large-scale interactomes and functional annotations of proteins and serve as an evolutionary analytical tool based on specific subnetworks and the identification of hub proteins (Hao et al., 2016). Table 1 enlists various bioinformatics tools for curating human protein network.
A protein isoform, also known as a protein variant, is a member of a set of highly similar proteins encoded by a single gene or gene family but differ genetically. While many isoforms serve the same or comparable biological tasks, some have distinct functions. Isoforms are formed via alternative transcription, splicing, 3′-end formation, translation and post-translational modification. A protein, which is a single node in the PPI network, transforms into a sub-network of interaction between the several isoforms encoded by the underlying gene; thus isoform-isoform network can be understood as a network and may offer potential application to identify an isoform-specific mutation that could help in the treatment of diseases (Liu et al., 2020). The IIIDB database can be freely accessed and allows searching for isoform-isoform interaction network modules (Tseng et al., 2015).
The genetic interaction network is the network demonstrating gene interrelations. These networks can forecast more known and unknown disease-gene connections for a certain disease. The genetic interaction network is able to predict disease-gene relationships without using generic protein-protein networks. More research is being done to tune the effectiveness of genetic interaction networks in identifying potential candidate genes. Genetic interaction networks can be analysed through STRING (Szklarczyk et al., 2021) and GeneMANIA (Warde-Farley et al., 2010).
The drug-target network is a bipartite network where some nodes represent the drug sets, and others represent the target (gene). Drug targets are nodes in the human interactome inextricably linked to treatment indication and side effects. The network-based location of each drug module also defines its pharmacological and biological relationships with other drugs (Liu et al., 2020). Table 2 enlists various bioinformatics tool for construction the drug-target network.
Brief Guide to Network Analysis
Many fundamental and translational neuroscience investigations continue to focus on the principal attributes of a node in a network as mentioned in Fig. 2 and described below:
Figure 2: Principal attributes for network analysis.
Degree: The number of connections (edges) between nodes determines the degree of a node in a network. The degree distribution is contrasting in nature, with nodes either having few or many connections.
Shortest path: The shortest path between two nodes is the one that requires the least number of nodes to connect them (Furlong, 2013). The use of shortest pathways to connect node pairs in biological networks is strongly motivated. The shortest route approach is frequently employed to assign orientations to edges in PPI networks to infer regulatory pathways from genes that match and identify genes or essential elements associated with cancer and other diseases (Liu et al., 2020).
Clustering coefficient: The clustering coefficient of a node is a measure of the degree of interconnectivity of its neighbors. It is calculated as the number of edges between neighbors of a node divided by the number of all possible edges between them. The value ranges from 0–1 (Furlong, 2013).
Betweenness centrality: Betweenness measures the global importance of nodes in communicating based on the number of shortest paths that pass through the node (Furlong, 2013). Studies have shown that proteins with a high degree of betweenness are more likely to be pleiotropic and disease-related genes. The betweenness of the brain functional networks has also been a topic of interest in testing brain activity (Liu et al., 2020).
Density: The percentage of actual connections among a network’s possible connections is known as “network density.” A connection between two “nodes” is referred to as a “possible connection” whether or not it actually exists. A network with higher associations will have higher density and lesser resilience to changes.
Degree distribution: The degree distribution indicates the probability that a network node chosen at random has a degree of k.
It is represented as pk,
pk=
where Nk is the number of nodes that have degree k and N is the total number of nodes.
A list of tools that can be used for network analysis is described in Table 3.
Role of Network Biology in Neurodevelopmental Disorders
Network biology approaches can be utilized in the study of various neurodevelopmental disorders as depicted in Fig. 3.
Figure 3: Important neurodevelopmental disorders included in the present study.
Attention-deficit hyperactivity disorder
ADHD is a neurodevelopmental condition that first appears in children and is characterized by persistent, inappropriate impulsivity, hyperactivity and inattention (Hayman and Fernandez, 2018). The worldwide prevalence of ADHD ranges from 1.4%–5% (Nickel et al., 2014). In childhood, ADHD is more common in men than women, with a 3:1 ratio between the sexes. There are three sub-categories of ADHD, pertaining to the type of behavioural symptoms: predominantly hyperactive/impulsive, predominantly inattentive, and combined type (Hayman and Fernandez, 2018). ADHD is often associated with other psychiatric and behavioural disorders, developmental disorders (e.g., dyslexia, autism), depression, and anxiety (Nickel et al., 2014). From the literature review, 105 genes associated with ADHD have been identified and genetics play an important role in this disorder. From twin studies, the heritability value of ADHD is found to be 0.76. In addition to biological variables like extremely low birth weight, prenatal nicotine exposure, stress, and alcohol intake, environmental pollutants such as lead and polychloride biphenyls, increased the probability of ADHD in children (Hayman and Fernandez, 2018). The current treatment of ADHD is age specific (Lola et al., 2019). Medications include methylphenidates (e.g., Ritalin, Concerta) and amphetamines (e.g., Adderall, Vyvanse) and behavioural therapy (Hayman and Fernandez, 2018).
Network biology for attention-deficit hyperactivity disorder symptom analysis
Using the network modelling approaches symptoms can be viewed as networks that reveal the complex relationship between the symptoms. The relationship between 18 symptoms in the Diagnostic Statistical Manual (DSM-5) criteria was explored through network biology. The outcomes of the ADHD symptom network were predicted using centrality, node strength and clustering coefficient.
Symptom networks indicated that symptoms are clearly split into two domains: inattentive and hyperactive/impulsive. Visual examination revealed that hyperactive/impulsive symptoms are tightly grouped, whereas inattentive symptoms are scattered. This trend was more distinct at the level of p 0.05.
Centrality was used to calculate the relatedness of individual symptoms, with higher values suggesting greater relative relevance of symptoms in the network. The symptom ‘loses things’ from the inattentive domain had the highest betweenness centrality, followed by symptoms ‘interrupts’, ‘motor driven’ and ‘leaves seats’ from the hyperactive domain. The study highlighted the importance of hyperactive symptoms in ADHD patients. Further analysis of the ADHD symptom network could reveal symptoms of clinical importance (Silk et al., 2019).
Identification of brain subnetworks related to attention-deficit hyperactivity disorder
The identification of subnetworks related to ADHD was based on the clustering of regions of interest (ROIs) of the brain. The clustering was done using a spectral clustering algorithm. Four clusters were identified, showing that the average functional brain network comprises four sub-networks. The sub-network related to ADHD was identified by calculating betweenness centrality, shortest path, clustering coefficient and the proposed graph entropy for each cluster of the control group and ADHD group. The only statistically significant correlation between children with ADHD and controls was the entropy in cluster 2.
The brain regions included in cluster 2 were composed of the bilateral pre- and post-central cortex, superior temporal gyrus, and inferior frontal gyri. This cluster presents statistically higher entropy for children with ADHD than for control (p-value = 0.002). The data showed significantly higher uncertainty or randomness in the sub-network structure of cluster 2 in children with ADHD than in the control (Lola et al., 2019).
Identification of drug-targets for attention-deficit hyperactivity disorder
Network-based pharmacology (Li, 2021) approaches were used for the identification of novel drug-targets for the prophylactic and therapeutic treatment of ADHD. Table 4 lists some drug-targets that can be targeted for effective prophylactic and therapeutic treatment of ADHD.
ASD is a heterogeneous group of disorders caused by abnormal brain development and neural reorganisation at a young age and is characterised by core abnormalities in two areas—social communication and restricted, repetitive sensory–motor behaviours (Lord et al., 2018). One in 100 children across the world is diagnosed with ASD, with the average affected male-to-female ratio being 4.2 (Zeidan et al., 2022). The heritability index of ASD was calculated to be 0.50 and the shared familial environmental influence was 0.04 (Sandin et al., 2017). Linkage and association studies were used to identify various susceptible loci (2q24–2q31, 7q, 17q11–17q21) and candidate genes (NRXN1, NLGN3, NLGN4, SHANK3, and CNTNAP2) that support the heterogeneity of ASD (Kumar and Christian, 2009). The only two FDA-approved drugs for use in children and adolescents with ASD include risperidone and aripiprazole (Goel et al., 2018).
Identification of novel genes related to autism spectrum disorder
Autistic-related neurological illnesses were identified, and a gene network for each member of the autism sibling group was created to detect genetic overlap between them. According to the findings, more than half of the known autism genes have also been related to other neurological illnesses. These results indicate the existence of molecular overlap and suggest that these conditions may share molecular mechanisms with autism, which may provide insight into understanding the hereditary basis of this complicated disorder.
A network-based approach was applied for the identification of disease-causing genes. A PPI network was generated that included proteins in the list of sibling autism disorders but not in the autism candidate list. A total of 334 novel genes that interact with autism genes were discovered. Using the same microarray data as the healthy controls, 87% of them were discovered to be significantly differentially expressed in autistic people (Parikshak et al., 2015).
Identification of drug-target for autism spectrum disorder
Network-based pharmacology (Li, 2021) approaches were used for the identification of novel drug-targets for the treatment of ASD. Table 5 below enlists some drugs that can be targeted for effective prophylactic and therapeutic treatment of ASD.
Epilepsy is a brain illness characterised by an enduring (i.e., persistent) proclivity to have seizures that are not triggered by any immediate central nervous system damage, as well as the neurobiological, cognitive, psychosocial, and social repercussions of seizure recurrences (Beghi, 2020).
Epilepsy is one of the most common neurological illnesses. It affects 50 million individuals globally at any given moment, according to the World Health Organisation, and 2% of the current world population has had epilepsy, has epilepsy currently, or will have epilepsy at some point in the future (Prinz, 2008).
Even though epilepsy refers to a group of disorders with a variety of causes and symptoms, all forms of epilepsy are characterised by recurrent seizures caused by abnormal electrical activity in the brain, most commonly in the form of synchronised discharges of nerve cells (neurons) that are not synchronously active during normal brain activity (Prinz, 2008).
Computer model for the understanding of epilepsy
In an ingenious sequence of experiments, Soltesz and colleagues merged the results of decades of study into a physiologically realistic, cellularly exact computer model of the dentate gyrus, a brain region implicated in temporal lobe epilepsy, the most common kind of epilepsy in adults. They demonstrated that the creation of a few numbers of highly linked “hub” neurons following brain injury is sufficient for the development of hyperexcitability and epilepsy using this computer model, which would be impossible to replicate in the brain (Morgan and Soltesz, 2008).
The neural network computer model developed by Soltesz (2008) is part of a new generation of large-scale, extremely detailed, and complex network models that attempt to rebuild entire brains or portions of brains in the computer. Models like this are starting to reveal new information regarding the significance of cellular and synaptic characteristics, as well as network topology, in influencing network activity in both healthy and diseased or disordered brains. Morgan and Soltesz’s network model (2008) is made up of 50,000 individual model neurons from a number of morphological and electrically diverse categories discovered in the dentate gyrus, and it preserves the empirically documented relative abundance ratios of those cell types.
Each model neuron is a complex, geographically extended model with anatomical, cellular, and electrical qualities tuned to reflect all available information from decades of research on the dentate gyrus and adjacent brain areas (Morgan and Soltesz, 2008).
Dentate gyrus simulations BY Morgan and Soltesz’s (2008) stand out as an example of well-executed modelling work. First, they made a clear observation: head injury causes two distinct alterations in the dentate gyrus, which are known to raise the risk of temporal lobe epilepsy. Two examples include the loss of mossy cells and the formation of new excitatory connections (mossy fibres) between granule cells. The healthy dentate gyrus does not have such excitatory recurrent connections between granule cells. Both of these effects of head trauma have been seen in the brains of epilepsy patients, making them potential candidates for a relationship between brain injury and epilepsy development. Second, the authors used their dentate gyrus model to test the effects of mossy cell loss and other topologically distinct types of mossy fibre sprouting on network excitability. Third, they arrived at the realistic and physiologically relevant conclusion that the formation of a few highly connected granule cell hubs is necessary and sufficient for the seizure-causing network hyperexcitability found in epileptic brains.
Identification of drug-targets for epilepsy
Network-based pharmacology (Li, 2021) approaches were used for the identification of novel drug-targets for treatment of epilepsy. Table 6 enlists some drug-targets that can be targeted for effective prophylactic and therapeutic treatment of epilepsy.
Neurodevelopmental abnormalities in individuals with intellectual disabilities are characterized by limitations in intellectual functioning and adaptive behaviour. These difficulties are frequently connected to mental health concerns (such as depression and anxiety), as well as neurodevelopmental diseases (such as autism spectrum disorders and attention deficit hyperactivity disorders).
Relationship between intellectual disability and autism spectrum disorder
Munson (Munson et al., 2008) found that there were various IQ-based subgroups of children with autism, each with different cognitive strengths and weaknesses, as well as the severity of autistic symptoms, after studying 456 children with autism. Finally, Deb and Prasad (1994) discovered that in people with autism and ID, impaired verbal and nonverbal communication, as well as the incidence of repetitive and restricted activities, were more common than people with only autism. When people with autism, whether they have ID or not, are compared to people without ID, the disparities are considerably more obvious.
Jackson et al. (2003) discovered that children with autism responded to verbal solicitations with less positive responses and more no responses in social interactions. Mandelbaum (2006) compared 242 children aged 7–9 years who were classified into one of four groups, including high IQ and autism; developmental language disorder produced similar patterns of performance, while low IQ and autism or low IQ produced similar patterns of performance but differed from the two previous groups. Sensory motor skills, oromotor skills, praxis, and ability/willingness to do activities were all improved in the two previous groups.
Genes linked to intellectual disability and autism spectrum disorder
Understanding the cellular and molecular mechanisms underlying the pathophysiology of ID and ASD-associated genes, as well as assessing the potential pathogenicity of new candidate genes for ID and ASD, functional categorization of proteins encoded by a majority of these genes and elucidation of common pathways, has become critical for understanding the cellular and molecular mechanisms underlying pathophysiology of these genes, and also for assessing the potential pathogenicity of new candidate genes for ID and ASD. As a result of this functional categorization, a variety of cellular functions regulating neuronal structure and function have emerged, which are influenced by abnormalities in the ID and ASD genes as described in Table 7 (Anand and Srivastava, 2014).
Identification of drug-targets for intellectual disability
Network-based pharmacology approaches (Li, 2021) were used for the identification of novel drug-targets for the treatment of ID. Table 8 below enlists some drug-targets that can be targeted for the effective prophylactic and therapeutic treatment of ID.
Use of Deep Learning in Network Biology and Target Identification
As all biological networks can efficiently maintain and quantify the interaction between components of cell systems underlying human diseases, artificial intelligence is a cutting-edge tool to identify novel disease targets and discover novel therapeutics. A number of alternative network techniques are offered to find disease targets, by network-based deep learning network biology analysis algorithms. More crucially, a variety of network-based biology analysis algorithms may investigate the network data from diverse perspectives and, as a result, can offer precise biological explanations (You et al., 2022). Biological networks may encapsulate the characteristics of biological entities and their relationships, making them effective at integrating complex biological data. A network can be conceptualised mathematically as a graph using the formula G = (V, E), where V and E are sets of nodes (vertices) and edges, respectively. Proteins, genes, illnesses, and medications are instances of nodes in biological networks. The edges of the network indicate various biochemical, physical or functional relationships between nodes. To identify therapeutic targets and find new medications, network-based biology analytic algorithms focus on studying molecular networks such as PPI networks, gene regulatory networks, metabolic networks, and drug-drug interaction networks (You et al., 2022).
The goal of graph embedding techniques is to develop a low-dimensional vector representation of a graph or of its constituent parts, such as its nodes. Often, this embedding is subsequently repurposed for tasks like link prediction or node classification. A graph domain equivalent to the image convolution in a convolutional neural network is provided by GCN approaches, which specify and employ a spectral- or spatial-based convolution over the graph.
It is crucial to correctly identify interactions of a target with different compounds to describe their binding affinity, or drug-target interactions (DTIs), after the discovery of a therapeutically useful target, such as a protein. This testing procedure produces a list of prospective drug candidates with strong binding affinities to the target, which is commonly known as a screening. Conducting manual screening on thousands of compounds in order to locate a single medicine is costly and time-consuming, as was already explained. Using DDI networks frequently, deep learning techniques attempt to get over this limitation. As a result, the graph deep learning framework often summarises the prediction of drug-target interactions as a link prediction problem. The drug-target prediction problem has been successfully handled by graph-based deep learning methods.
The molecular structure of drugs and targets is considered and examined in a different group of methodologies that define the DTI. In the GCN approach to the DTI prediction problem, the input consists of two graphs: a protein pocket graph and a 2-dimensional (2D) drug molecular graph.
This approach consists of two steps: (i) an initial unsupervised phase that includes an auto-encoder for learning common pocket features and (ii) a supervised graph convolutional binding classifier. The latter is made up of two GCN models that operate simultaneously in a drug GCN and a pocket GCN, which each extract characteristics from a protein pocket graph and a 2D molecule graph. A layer is in charge of combining protein interactions to create a joint drug-target imprint, which is subsequently divided into “binding” and “non-binding” groups (Muzio et al., 2021).
Target-based drug discovery has proven to be extremely effective. The method is not restricted to small-molecule drug discovery. Target-based approaches are commonly used to discover antibody drugs and other protein biologics. Target-based approaches have the advantage of being easier to execute because they are utilized based on the molecular mechanisms of a drug from an earlier stage, and they are generally faster, easier, and less expensive to develop and run (Croston, 2017).
Optimizing compounds in the absence of a known molecular target, as with a phenotypic strategy, can be difficult. Human genetics is one of the most powerful tools for identifying drug targets. When a human gene is linked to a disease, it is clear that a drug targeting that gene or gene product has a good chance of success.
Although network biology has advanced to new heights, a few limitations still remain. Real-world biological systems are far more complex, with interactions occurring at multiple scales, including gene, RNA, protein, and metabolic levels. The majority of studies tend to focus only on specific levels of interactions among the same type of biomolecules. With the advancement of high-throughput technologies and the accumulation of various omics data, performing network biology analysis by integrating multi-omics data would be intriguing. Most biological networks are static, whereas Real-world biological networks change their structures dynamically over time. Furthermore, some genes may only be expressed in certain tissues and at certain times; biological networks have temporal, dynamic and modular properties. In fact, multilayer biological networks have received a lot of attention in recent years. Multilayer biological network datasets, on the other hand, are not made public. It is possible to develop efficient data-driven frameworks to construct multilayer biological networks with the accumulation of various omics data and the development of network inference techniques Network biology applications require the collaboration of scientists from different fields. Network biology provides effective tools for investigating bioinformatics, and both biologists and medical workers are required to apply the theoretical results (Wang, 2022).
Many fundamental and translational neuroscience investigations continue to focus on candidate genes and candidate theories; thus, skeptics may question the relevance of monitoring whole systems. Biological complexity, on the other hand, must not be overlooked; genome-wide measures, in conjunction with specific gene and pathway research, are essential to uncover the underlying causes of neurodevelopmental and neurodegenerative diseases. Biologists can use well-designed, repeatable molecular profiling studies to test theories and concurrently generate new ones. Gene networks provide an organising structure that facilitates the process of hypothesis creation and testing, despite their vastness and seeming complexity. The overarching concept of using correlational and physical interaction molecular networks in neurobiology to explain molecular system changes may be expanded to a number of methodologies, allowing for examining connections at different levels of inquiry.
The findings of high-quality genome-wide studies will be critical in developing and testing theories that go beyond our current understanding to generate a more comprehensive picture of the issues faced by neurodevelopmental and neurodegenerative illnesses, and potential solutions (Neelroop and Parikshak, 2015).
At this point, network neuroscience has the potential to provide inspiration and resources to the area of child and adolescent psychology, allowing it to make significant progress in understanding the neurobiology of mental disorders that impact children and adolescents. Collaborations among cognitive neuroscientists, clinical psychologists, engineers, and computer scientists will provide the skills needed to harness the power of big data sets to advance our understanding of typical and atypical brain network development (Uddin and Karlsgodt, 2018).
Acknowledgement: We acknowledge the support of all the bioinformatics tools, software, and databases used in the present research. We also acknowledge the guidance of Amity Institute of Biotechnology, Amity University Uttar Pradesh (Lucknow campus) where the benchwork of the present study was carried out.
Funding Statement: The authors received no specific funding for this study.
Author Contributions: The authors confirm contribution to the paper as follows: study conception and design: Prekshi Garg, Prachi Srivastava; data collection: Wayez Naqvi, Ananya Singh; analysis and interpretation of results: Prekshi Garg, Wayez Naqvi, Ananya Singh; draft manuscript preparation: Wayez Naqvi, Ananya Singh; final approval of the manuscript: Prachi Srivastava. All authors reviewed the results and approved the final version of the manuscript.
Availability of Data and Materials: The datasets generated during and/or analysed during the current study are available in the PubMed and PubMed Central repository, https://pubmed.ncbi.nlm.nih.gov/, https://www.ncbi.nlm.nih.gov/pmc/.
Ethics Approval: Not applicable.
Conflicts of Interest: The authors declare that they have no conflicts of interest to report regarding the present study.
References
Anand K, Srivastava CE (2014). Intellectual disability and autism spectrum disorders: Causal genes and molecular mechanisms. Neuroscience & Biobehavioral Reviews 46: 161–174. https://doi.org/10.1016/j.neubiorev.2014.02.015 [Google Scholar] [PubMed] [CrossRef]
Armstrong D, Strange PG (2001). Dopamine D2 receptor dimer formation: Evidence from ligand binding. The Journal of Biological Chemistry 276: 22621–22629. https://doi.org/10.1074/jbc.M006936200 [Google Scholar] [PubMed] [CrossRef]
Beghi E (2020). The epidemiology of epilepsy. Neuroepidemiology 54: 185–191. https://doi.org/10.1159/000503831 [Google Scholar] [PubMed] [CrossRef]
Boison D (2008). The adenosine kinase hypothesis of epileptogenesis. Progress in Neurobiology 84: 249–262. https://doi.org/10.1016/j.pneurobio.2007.12.002 [Google Scholar] [PubMed] [CrossRef]
Butler-Ryan R, Wood IC (2021). The functions of repressor element 1-silencing transcription factor in models of epileptogenesis and post-ischemia. Metabolic Brain Disease 36: 1135–1150. https://doi.org/10.1007/s11011-021-00719-2 [Google Scholar] [PubMed] [CrossRef]
Chatr-Aryamontri A, Breitkreutz BJ, Oughtred R, Boucher L, Heinicke S et al. (2015). The BioGRID interaction database: 2015 update. Nucleic Acids Research 43: D470–D478. https://doi.org/10.1093/nar/gku1204 [Google Scholar] [PubMed] [CrossRef]
Chen Y, Ma K, Si H, Duan Y, Zhai H (2022). Network pharmacology integrated molecular docking to reveal the autism and mechanism of Baohewan Heshiwei Wen Dan Tang. Current Pharmaceutical Design 28: 3231–3241. https://doi.org/10.2174/1381612828666220926095922 [Google Scholar] [PubMed] [CrossRef]
Chen R, Xue G, Hölscher C (2021). The role of the TNFα-mediated astrocyte signaling pathway in epilepsy. Acta Epileptologica 3: 57. https://doi.org/10.1186/s42494-021-00059-9 [Google Scholar] [CrossRef]
Croston GE (2017). The utility of target-based discovery. Expert Opinion on Drug Discovery 12: 427–429. https://doi.org/10.1080/17460441.2017.1308351 [Google Scholar] [PubMed] [CrossRef]
Deb S, Prasad KBG (1994). The prevalence of autistic disorder among children with a learning disability. The British Journal of Psychiatry 165: 395–399. https://doi.org/10.1192/bjp.165.3.395 [Google Scholar] [PubMed] [CrossRef]
di Marco B, Bonaccorso CM, Aloisi E, D’Antoni S, Catania MV (2016). Neuro-inflammatory mechanisms in developmental disorders associated with intellectual disability and autism spectrum disorder: A neuro-immune perspective. CNS and Neurological Disorders Drug Targets 15: 448–463. https://doi.org/10.2174/1871527315666160321105039 [Google Scholar] [PubMed] [CrossRef]
Elsadek AE, Al-Shokary AH, Abdelghani WE, Kamal NM, Ibrahim AO, El-Shorbagy HH, Nour El Din DM (2020). Serum levels of interleukin-6 and tumor necrosis factor alpha in children with attention-deficit hyperactivity disorder. Journal of Pediatric Neurosciences 15: 402–408. https://doi.org/10.4103/JPN.JPN_1_20 [Google Scholar] [PubMed] [CrossRef]
Emma LB, Soldner AM (2019). Inhibition of human microsomal PGE2 synthase-1 reduces seizure-induced increases of P-glycoprotein expression and activity at the blood-brain barrier. FASEB Journal 33: 13966–13981. https://doi.org/10.1096/fj.201901460RR [Google Scholar] [PubMed] [CrossRef]
Freshour SL, Kiwala S, Cotto KC, Coffman AC, McMichael JF, Song JJ, Griffith M, Griffith OL, Wagner AH (2021). Integration of the drug-gene interaction database (DGIdb 4.0) with open crowdsource efforts. Nucleic Acids Research 49: 1144–1151. https://doi.org/10.1093/nar/gkaa1084 [Google Scholar] [PubMed] [CrossRef]
Furlong LI (2013). Human diseases through the lens of network biology. Trends in Genetics 29: 150–159. https://doi.org/10.1016/j.tig.2012.11.004 [Google Scholar] [PubMed] [CrossRef]
Gai J, Xing J, Wang Y, Lei J, Zhang C, Zhang J, Tang J (2022). Exploration of potential targets and mechanisms of Naringenin in treating autism spectrum disorder via network pharmacology and molecular docking. Medicine 101: e31787. https://doi.org/10.1097/MD.0000000000031787 [Google Scholar] [PubMed] [CrossRef]
Goel R, Hong JS, Findling RL, Ji NY (2018). An update on pharmacotherapy of autism spectrum disorder in children and adolescents. International Review of Psychiatry 30: 78–95. https://doi.org/10.1080/09540261.2018.1458706 [Google Scholar] [PubMed] [CrossRef]
Han Y, Wang C, Klinger K, Rajpal DK, Zhu C (2021). An integrative network-based approach for drug target indication expansion. PLoS One 16: e0253614. https://doi.org/10.1371/journal.pone.0253614 [Google Scholar] [PubMed] [CrossRef]
Hao T, Peng W, Wang Q, Wang B, Sun J (2016). Reconstruction and application of protein-protein interaction network. International Journal of Molecular Sciences 17: 907. https://doi.org/10.3390/ijms17060907 [Google Scholar] [PubMed] [CrossRef]
Hayman V, Fernandez TV (2018). Genetic insights into ADHD biology. Frontiers in Psychiatry 9: 2510. https://doi.org/10.3389/fpsyt.2018.00251 [Google Scholar] [PubMed] [CrossRef]
Hlushchenko I, Khanal P, Abouelezz A, Paavilainen VO, Hotulainen P (2018). ASD-associated de novo mutations in five actin regulators show both shared and distinct defects in dendritic spines and inhibitory synapses in cultured hippocampal neurons. Frontiers in Cellular Neuroscience 12: 217. https://doi.org/10.3389/fncel.2018.00217 [Google Scholar] [PubMed] [CrossRef]
Hormozdiari F, Penn O, Borenstein E, Eichler EE (2015). The discovery of integrated gene networks for autism and related disorders. Genome Research 25: 142–154. https://doi.org/10.1101/gr.178855.114 [Google Scholar] [PubMed] [CrossRef]
Iwase S, Bérubé NG, Zhou Z, Kasri NN, Battaglioli E, Scandaglia M, Barco A (2017). Epigenetic etiology of intellectual disability. The Journal of Neuroscience 37: 10773–10782. https://doi.org/10.1523/JNEUROSCI.1840-17.2017 [Google Scholar] [PubMed] [CrossRef]
Jackson CT, Fein D, Wolf J, Jones G, Hauck M, Waterhouse L, Feinstein C (2003). Responses and sustained interactions in children with mental retardation and autism. Journal of Autism and Developmental Disorders 33: 115–121. https://doi.org/10.1023/a:1022927124025 [Google Scholar] [PubMed] [CrossRef]
Ji X, Freudenberg JM, Agarwal P (2019). Integrating biological networks for drug target prediction and prioritization. Methods in Molecular Biology 1903: 203–218. https://doi.org/10.1007/978-1-4939-8955-3 [Google Scholar] [CrossRef]
Kalkan Z, Durasi İ.M, Sezerman U, Atasever-Arslan B (2016). Potential of GRID2 receptor gene for preventing TNF-induced neurodegeneration in autism. Neuroscience Letters 620: 62–69. https://doi.org/10.1016/j.neulet.2016.03.043 [Google Scholar] [PubMed] [CrossRef]
Kobayashi T, Washiyama K, Ikeda K (2010). Inhibition of G-protein-activated inwardly rectifying K+ channels by the selective norepinephrine reuptake inhibitors atomoxetine and reboxetine. Neuropsychopharmacology 35: 1560–1569. https://doi.org/10.1038/npp.2010.27 [Google Scholar] [PubMed] [CrossRef]
Koch E, Demontis D (2022). Drug repurposing candidates to treat core symptoms in autism spectrum disorder. Frontiers in Pharmacology 13: 995439. https://doi.org/10.3389/fphar.2022.995439 [Google Scholar] [PubMed] [CrossRef]
Kruth KA, Grisolano TM, Ahern CA, Williams AJ (2020). SCN2A channelopathies in the autism spectrum of neuropsychiatric disorders: A role for pluripotent stem cells? Molecular Autism 11: 1–11. https://doi.org/10.1186/s13229-020-00330-9 [Google Scholar] [PubMed] [CrossRef]
Kuhn M, Szklarczyk D, Franceschini A, Campillos M, von Mering C, Jensen LJ, Beyer A, Bork P (2010). STITCH 2: An interaction network database for small molecules and proteins. Nucleic Acids Research 38: 552–556. https://doi.org/10.1093/nar/gkp937 [Google Scholar] [PubMed] [CrossRef]
Kumar RA, Christian SL (2009). Genetics of autism spectrum disorders. Current Neurology and Neuroscience Reports 9: 188–197. https://doi.org/10.1007/s11910-009-0029-2 [Google Scholar] [PubMed] [CrossRef]
Law V, Knox C, Djoumbou Y, Jewison T, Guo AC et al. (2014). DrugBank 4.0: Shedding new light on drug metabolism. Nucleic Acids Research 42: 1091–1097. https://doi.org/10.1093/nar/gkt1068 [Google Scholar] [PubMed] [CrossRef]
Li S (2021). Network pharmacology evaluation method guidance—draft. World Journal of Traditional Chinese Medicine 7: 146–154. https://doi.org/10.4103/wjtcm.wjtcm_11_21 [Google Scholar] [CrossRef]
Licata L, Briganti L, Peluso D, Perfetto L, Iannuccelli M et al. (2012). MINT, the molecular interaction database: 2012 update. Nucleic Acids Research 40: 857–861. https://doi.org/10.1093/nar/gkr930 [Google Scholar] [PubMed] [CrossRef]
Liu C, Ma Y, Zhao J, Nussinov R, Zhang YC, Cheng F, Zhang ZK (2020). Computational network biology: Data, models, and applications. Physics Reports 846: 1–66. https://doi.org/10.1016/j.physrep.2019.12.004 [Google Scholar] [CrossRef]
Lola HM, Belete H, Gebeyehu A, Zerihun A, Yimer S, Leta K (2019). Attention deficit hyperactivity disorder (ADHD) among children aged 6 to 17 years old living in Girja district, rural Ethiopia. Behavioural Neurology 2019: 1–8. https://doi.org/10.1155/2019/1753580 [Google Scholar] [PubMed] [CrossRef]
Lord C, Elsabbagh M, Baird G, Veenstra-Vanderweele J (2018). Autism spectrum disorder. The Lancet 392: 508–520. https://doi.org/10.1016/S0140-6736(18)31129-2 [Google Scholar] [PubMed] [CrossRef]
Mandelbaum DE (2006). Sensorimotor performance in school-age children with autism, developmental language disorder, or low IQ. Developmental Medicine and Child Neurology 48: 33–39. https://doi.org/10.1017/S0012162206000089 [Google Scholar] [PubMed] [CrossRef]
McNamara JO, Scharfman HE (2012). Temporal lobe epilepsy and the BDNF receptor, TrkB. Jasper’s Basic Mechanisms of the Epilepsies 51: 514–531. https://doi.org/10.1111/j.1528-1167.2010.02832.x [Google Scholar] [CrossRef]
Misiak B, Wójta-Kempa M, Samochowiec J, Schiweck C, Aichholzer M, Reif A, Stańczykiewicz B (2022). Peripheral blood inflammatory markers in patients with attention deficit/hyperactivity disorder (ADHDA systematic review and meta-analysis. Progress in Neuro-Psychopharmacology and Biological Psychiatry 118: 110581. https://doi.org/10.1016/j.pnpbp.2022.110581 [Google Scholar] [PubMed] [CrossRef]
Montani C, Ramos-Brossier M, Ponzoni L, Gritti L, Cwetsch AW, Braida D, Sala C (2017). The X-linked intellectual disability protein IL1RAPL1 regulates dendrite complexity. The Journal of Neuroscience 37: 6606–6627. https://doi.org/10.1523/JNEUROSCI.3775-16.2017 [Google Scholar] [PubMed] [CrossRef]
Morgan RJ, Soltesz I (2008). Nonrandom connectivity of the epileptic dentate gyrus predicts a major role for neuronal hubs in seizures. Proceedings of the National Academy of Sciences of the United States of America 105: 6179–6184. https://doi.org/10.1073/pnas.0801372105 [Google Scholar] [PubMed] [CrossRef]
Mosca R, Céol A, Aloy P (2013). Interactome3D: Adding structural details to protein networks. Nature Methods 10: 47–53. https://doi.org/10.1038/nmeth.2289 [Google Scholar] [PubMed] [CrossRef]
Munson J, Dawson G, Sterling L, Beauchaine T, Zhou A et al. (2008). Evidence for latent classes of IQ in young children with autism spectrum disorder. American Journal of Mental Retardation 113: 439–452. https://doi.org/10.1352/2008.113:439-452 [Google Scholar] [PubMed] [CrossRef]
Muzio G, O’Bray L, Borgwardt K (2021). Biological network analysis with deep learning. Briefings in Bioinformatics 22: 1515–1530. https://doi.org/10.1093/bib/bbaa257 [Google Scholar] [PubMed] [CrossRef]
Neelroop N, Parikshak MJ (2015). Systems biology and gene networks in neurodevelopmental and neurodegenerative disorders. Nature Reviews Genetics 16: 441–458. https://doi.org/10.1038/nrg3934 [Google Scholar] [PubMed] [CrossRef]
Nickel J, Gohlke B-O, Erehman J, Banerjee P, Rong WW, Goede A, Dunkel M, Preissner R (2014). SuperPred: Update on drug classification and target prediction. Nucleic Acids Research 42: 26–31. https://doi.org/10.1093/nar/gku477 [Google Scholar] [PubMed] [CrossRef]
Owens AP (2021). Autonomic dysfunction in autism spectrum disorder. Frontiers in Integrative Neuroscience 15: 7870375. https://doi.org/10.3389/fnint.2021.787037 [Google Scholar] [PubMed] [CrossRef]
Parenti I, Rabaneda LG, Schoen H, Novarino G (2020). Neurodevelopmental disorders: From genetics to functional pathways. Trends in Neurosciences 43: 608–621. https://doi.org/10.1016/j.tins.2020.05.004 [Google Scholar] [PubMed] [CrossRef]
Parikshak NN, Gandal MJ, Geschwind DH (2015). Systems biology and gene networks in neurodevelopmental and neurodegenerative disorders. Nature Reviews Genetics 16: 441–458. https://doi.org/10.1038/nrg3934 [Google Scholar] [PubMed] [CrossRef]
Prasad S (2009). Human protein reference database-2009 update. Nucleic Acids Research 37: 767–772. https://doi.org/10.1093/nar/gkn892 [Google Scholar] [PubMed] [CrossRef]
Prinz AA (2008). Understanding epilepsy through network modeling. Proceedings of the National Academy of Sciences of the United States of America 105: 5953–5954. https://doi.org/10.1073/pnas.0802299105 [Google Scholar] [PubMed] [CrossRef]
Rosina E, Battan B, Siracusano M, di Criscio L, Hollis F, Pacini L, Bagni C (2019). Disruption of mTOR and MAPK pathways correlates with severity in idiopathic autism. Translational Psychiatry 9: 50. https://doi.org/10.1038/s41398-018-0335-z [Google Scholar] [PubMed] [CrossRef]
Sandin S, Lichtenstein P, Kuja-Halkola R, Hultman C, Larsson H, Reichenberg A (2017). The heritability of autism spectrum disorder. JAMA 318: 1182–1184. https://doi.org/10.1001/jama.2017.12141 [Google Scholar] [PubMed] [CrossRef]
Silk TJ, Malpas CB, Beare R, Efron D, Anderson V, Hazell P, Jongeling B, Nicholson JM, Sciberras E (2019). A network analysis approach to ADHD symptoms: More than the sum of its parts. PLoS One 14: e0211053. https://doi.org/10.1371/journal.pone.0211053 [Google Scholar] [PubMed] [CrossRef]
Song Z, Luo G, Han C, Jia G, Zhang B (2022). Potential targets and action mechanism of gastrodin in the treatment of attention-deficit/hyperactivity disorder: Bioinformatics and network pharmacology analysis. Evidence-Based Complementary and Alternative Medicine 2022: 1–12. https://doi.org/10.1155/2022/3607053 [Google Scholar] [PubMed] [CrossRef]
Sonuga-Barke EJS, Kumsta R, Schlotz W, Lasky-Su J, Marco R, Miranda A, Faraone SV (2011). A functional variant of the serotonin transporter gene (SLC6A4) moderates impulsive choice in attention-deficit/hyperactivity disorder boys and siblings. Biological Psychiatry 70: 230–236. https://doi.org/10.1016/j.biopsych.2011.01.040 [Google Scholar] [PubMed] [CrossRef]
Soltesz RJ (2008). Nonrandom connectivity of the epileptic dentate gyrus predicts a major role for neuronal hubs in seizures. Proceedings of the National Academy of Sciences of the United States of America 105: 6179–6184. [Google Scholar]
Srivastava N, Mishra BN, Srivastava P (2019). In-silico identification of drug lead molecule against pesticide exposed-neurodevelopmental disorders through network-based computational model approach. Current Bioinformatics 14: 460–467. https://doi.org/10.2174/1574893613666181112130346 [Google Scholar] [CrossRef]
Stumpf MPH, Thorne T, de Silva E, Stewart R, An HJ, Lappe M, Wiuf C (2008). Estimating the size of the human interactome. Proceedings of the National Academy of Sciences of the United States of America 105: 6959–6964. https://doi.org/10.1073/pnas.0708078105 [Google Scholar] [PubMed] [CrossRef]
Sun H, Ma D, Cheng Y, Li J, Zhang W, Jiang T, Meng H (2022). The JAK-STAT signaling pathway in epilepsy. Current Neuropharmacology 21. https://doi.org/10.2174/1570159X21666221214170234 [Google Scholar] [PubMed] [CrossRef]
Szklarczyk D, Franceschini A, Kuhn M, Simonovic M, Roth A et al. (2011). The STRING database in 2011: Functional interaction networks of proteins, globally integrated and scored. Nucleic Acids Research 39: 561–568. https://doi.org/10.1093/nar/gkq973 [Google Scholar] [PubMed] [CrossRef]
Szklarczyk D, Gable AL, Nastou KC, Lyon D, Kirsch R et al. (2021). The STRING database in 2021: Customizable protein-protein networks, and functional characterization of user-uploaded gene/measurement sets. Nucleic Acids Research 49: 605–612. https://doi.org/10.1093/nar/gkaa1074 [Google Scholar] [PubMed] [CrossRef]
Tovo-Rodrigues L, Rohde LA, Menezes AMB, Polanczyk GV, Kieling C, Genro JP, Hutz MH (2013). DRD4 rare variants in attention-deficit/hyperactivity disorder (ADHDFurther evidence from a birth cohort study. PLoS One 8: e85164. https://doi.org/10.1371/journal.pone.0085164 [Google Scholar] [PubMed] [CrossRef]
Tseng YT, Li W, Chen CH, Zhang S, Chen JJW, Zhou X, Liu CC (2015). IIIDB: A database for isoform-isoform interactions and isoform network modules. BMC Genomics 16: S10. https://doi.org/10.1186/1471-2164-16-S2-S10 [Google Scholar] [PubMed] [CrossRef]
Uddin LQ, Karlsgodt KH (2018). Future directions for examination of brain networks in neurodevelopmental disorders. Journal of Clinical Child and Adolescent Psychology 47: 483–497. https://doi.org/10.1080/15374416.2018.1443461 [Google Scholar] [PubMed] [CrossRef]
Verma A, Chauhan SS, Pankaj V, Srivastva N, Srivastava P (2020). Network biology approaches to identify the drug lead molecule for neurodevelopmental disorders in human. The Open Bioinformatics Journal 13: 15–24. https://doi.org/10.2174/1875036202013010015 [Google Scholar] [CrossRef]
Wang P (2022). Network biology: Recent advances and challenges. Gene and Protein in Disease 1: 101. https://doi.org/10.36922/gpd.v1i2.101 [Google Scholar] [CrossRef]
Warde-Farley D, Donaldson SL, Comes O, Zuberi K, Badrawi R et al. (2010). The GeneMANIA prediction server: Biological network integration for gene prioritization and predicting gene function. Nucleic Acids Research 38: 214–220. https://doi.org/10.1093/nar/gkq537 [Google Scholar] [PubMed] [CrossRef]
Wilens TE, Decker MW (2007). Neuronal nicotinic receptor agonists for the treatment of attention-deficit/hyperactivity disorder: Focus on cognition. Biochemical Pharmacology 74: 1212–1223. https://doi.org/10.1016/j.bcp.2007.07.002 [Google Scholar] [PubMed] [CrossRef]
Wongpaiboonwattana W, Plong-On O, Hnoonual A, Limprasert P (2020). Significant associations between 5-hydroxytryptaminetransporter-linked promoter region polymorphisms of the serotonin transporter (solute carrier family 6 member 4) gene and Thai patients with autism spectrum disorder. Medicine 99: e21946. https://doi.org/10.1097/MD.0000000000021946 [Google Scholar] [PubMed] [CrossRef]
Xing X, Zhang J, Wu K, Cao B, Li X, Jiang F, Li JD (2019). Suppression of Akt-mTOR pathway rescued the social behavior in Cntnap2-deficient mice. Scientific Reports 9: 3041. https://doi.org/10.1038/s41598-019-39434-5 [Google Scholar] [PubMed] [CrossRef]
You Y, Lai X, Pan Y, Zheng H, Vera J, Liu S, Deng S, Zhang L (2022). Artificial intelligence in cancer target identification and drug discovery. Signal Transduction and Targeted Therapy 7: 24. https://doi.org/10.1038/s41392-022-00994-0 [Google Scholar] [PubMed] [CrossRef]
Zeidan J, Fombonne E, Scorah J, Ibrahim A, Durkin MS, Saxena S, Yusuf A, Shih A, Elsabbagh M (2022). Global prevalence of autism: A systematic review update. Autism Research 15: 778–790. https://doi.org/10.1002/aur.2696 [Google Scholar] [PubMed] [CrossRef]
Zeng LH, Rensing NR, Wong M (2009). The mammalian target of rapamycin signaling pathway mediates epileptogenesis in a model of temporal lobe epilepsy. The Journal of Neuroscience 29: 6964–6972. https://doi.org/10.1523/JNEUROSCI.0066-09.2009 [Google Scholar] [PubMed] [CrossRef]
Zhang LL, Lai C, Su YH, Gan HZ, Li YC et al. (2022). A multi-target and multi-channel mechanism of action for Jiawei Yinhuo Tang in the treatment of social communication disorders in autism: Network pharmacology and molecular docking studies. Evidence-Based Complementary and Alternative Medicine 2022: 1–17. https://doi.org/10.1155/2022/4093138 [Google Scholar] [PubMed] [CrossRef]
Zhao H, Zhang H, Liu S, Luo W, Jiang Y, Gao J (2021). Association of peripheral blood levels of cytokines with autism spectrum disorder: A meta-analysis. Frontiers in Psychiatry 12: 670200. https://doi.org/10.3389/fpsyt.2021.670200 [Google Scholar] [PubMed] [CrossRef]
Cite This Article
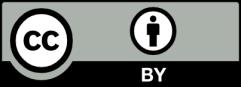