Open Access
ARTICLE
Study of molecular mechanisms underlying the medicinal plant Tripterygium wilfordii-derived compound celastrol in treating diabetic nephropathy based on network pharmacology and molecular docking
1 The Second School of Clinical Medicine, Zhejiang Chinese Medical University, Hangzhou, 310003, China
2 Urology & Nephrology Center, Department of Nephrology, Zhejiang Provincial People’s Hospital, Affiliated People’s Hospital, Hangzhou Medical College, Hangzhou, 310014, China
3 Department of Nephrology, The First Affiliated Hospital of Zhejiang Chinese Medical University (Zhejiang Provincial Hospital of Chinese Medicine), Hangzhou, 310000, China
* Corresponding Author: JUAN JIN. Email:
(This article belongs to the Special Issue: Natural Products for Chronic Inflammatory Diseases: Pharmacology and Toxicology)
BIOCELL 2023, 47(8), 1853-1867. https://doi.org/10.32604/biocell.2023.029353
Received 14 February 2023; Accepted 19 April 2023; Issue published 28 August 2023
Abstract
Background: Diabetic nephropathy (DN) is a serious complication of diabetes with rising prevalence worldwide. We aimed to explore the anti-DN mechanisms of the compound celastrol derived from the medicinal plant Tripterygium wilfordii. Methods: Celastrol-related targets were obtained from Herbal Ingredients’ Targets (HIT) and GeneCards databases. DN-related targets were retrieved from GeneCards, DisGeNET, and Therapeutic Targets Database (TTD). A Protein-protein interaction (PPI) network was established using the Search Tool for the Retrieval of Interacting Genes (STRING) database. Gene Ontology (GO) and Kyoto Encyclopedia of Genes and Genomes (KEGG) enrichment analyses were performed using ClusterProfiler. The cytoHubba plugin was used to select the top 10 hub targets. Molecular docking was performed employing PyMOL and AutoDock software. Cell counting kit-8 (CCK-8) and flow cytometry assays were used to detect the viability and apoptosis of NRK-52E cells, respectively. The mRNA expression levels of mitogen-activated protein kinase 3 (MAPK3), tumor necrosis factor (TNF), and AKT serine/threonine kinase 1 (AKT1) in NRK-52E cells were assessed using quantitative real-time polymerase chain reaction (qRT-PCR). Results: We obtained sixty-six overlapping targets of celastrol and DN. GO and KEGG analyses demonstrated that the core targets of celastrol and DN were mainly involved in the inflammatory and immune response, oxidative stress, advanced glycation end products (AGEs) and their receptors (RAGEs) (AGE-RAGE), TNF, interleukin 17 (IL-17), and MAPK signaling pathways. Finally, based on the good binding activity with celastrol, MAPK3, TNF, and AKT1 were identified as the foremost targets of celastrol. We observed that celastrol enhanced the viability of high glucose (HG)-treated NRK-52E cells and inhibited apoptosis in the in vitro assays. Moreover, celastrol decreased the mRNA expression levels of MAPK3, TNF, and AKT1 in high glucose (HG)-treated NRK-52E cells. Conclusion: Celastrol may treat DN by targeting APK3, TNF, and AKT1 and regulating inflammatory responses and oxidative stress through the AGE-RAGE, TNF, IL-17, and MAPK signaling pathways.Graphic Abstract
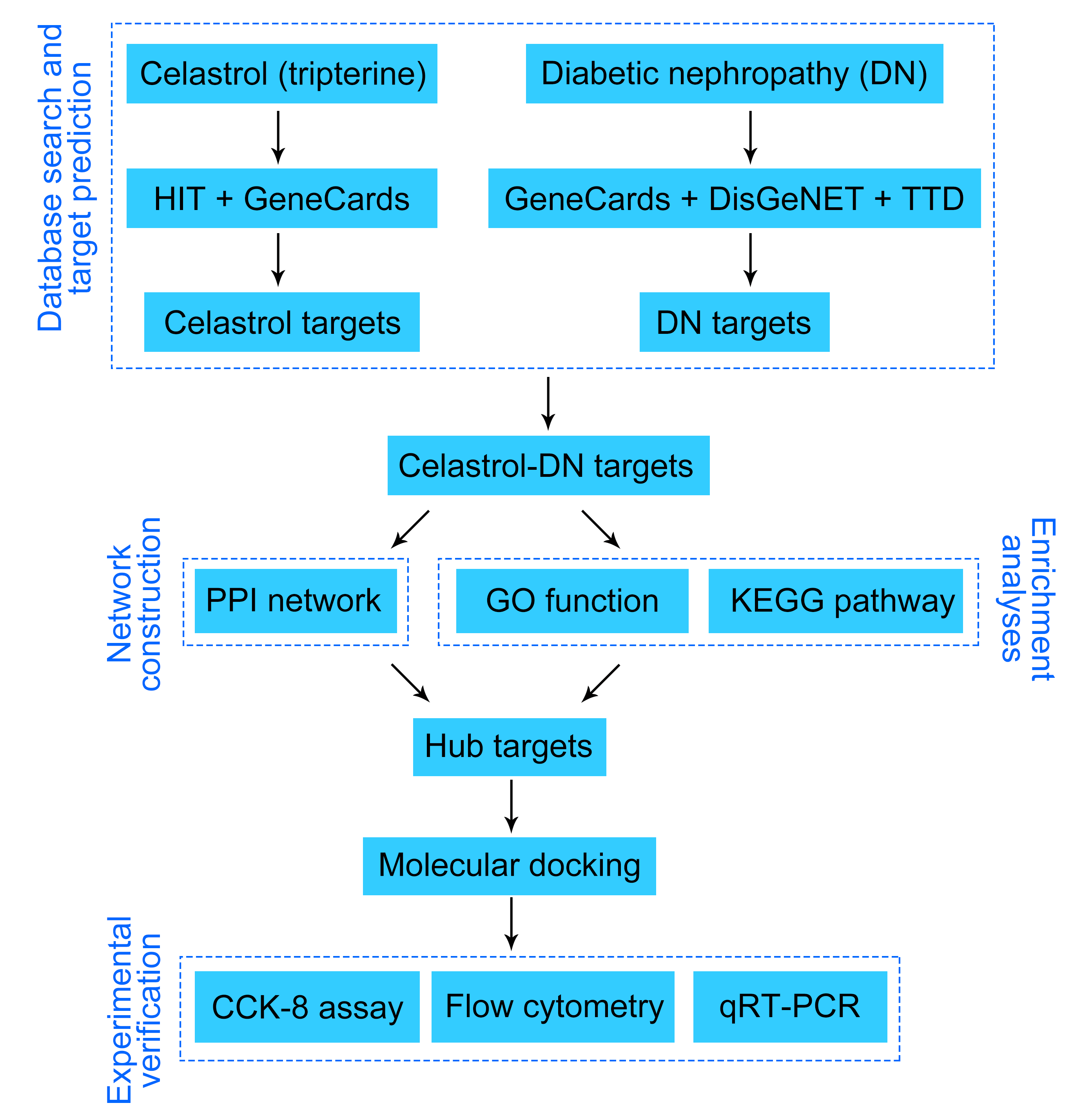
Keywords
Supplementary Material
Supplementary Material FileGraphical abstract
Diabetic nephropathy (DN), also termed diabetic kidney disease (DKD), is a severe complication of diabetes and the leading cause of end-stage renal disease (ESRD). Its incidence is on the rise worldwide (Zhang et al., 2018a; Khan et al., 2020; Tang et al., 2021). In 2019, approximately 463 million individuals were affected by DN worldwide, and this figure is predicted to rise to 700 million by 2045. DN is characterized by a gradual increase in urine albumin, elevated blood pressure, and decreased glomerular filtration rate (Lu et al., 2021b). At present, the primary therapeutic strategy for DN focuses on the control of glucose and blood pressure (Mohsen et al., 2021). Blocking the renin-angiotensin-aldosterone system (RAAS) remains the predominant approach of managing DN (Block et al., 2022). Furthermore, some agents, such as sodium-glucose cotransporter type 2 (SGLT2) inhibitors (Shaffner et al., 2021) and dipeptidyl peptidase-4 (DPP-4) inhibitors (Trakarnvanich et al., 2021), reportedly have favorable therapeutic potential for DN. Despite improvements in the treatment of DN, current therapeutic approaches are insufficient to completely delay DN progression and numerous DN patients eventually progress into ESRD (Johnson and Spurney, 2015). Therefore, there is an urgent need to further understand the pathological mechanisms of DN, thereby developing novel and effective therapies to prevent its progression and occurrence.
Traditional Chinese medicine has attracted a lot of attention worldwide due to its good efficacy and safety in the treatment of various disorders, such as malignant tumors and inflammatory diseases. Some active ingredients of Chinese herbs, such as geniposide (Li et al., 2020), catalpol (Chen et al., 2020), tripterygium glycosides (Guo et al., 2021a), and quercetin (Hu et al., 2022), have exhibited their beneficial effects in the treatment of DN. Celastrol, also named tripterine, is a natural bioactive ingredient isolated from the root extract of Tripterygium wilfordii (a traditional Chinese herb) (Wagh et al., 2021; Guo et al., 2021b; Zhang et al., 2022). Furthermore, it has been reported that celastrol can also be synthesized (Lu et al., 2021a). Celastrol is well-known for its multiple pharmacological effects, including anti-inflammatory, anti-autoimmune, anti-cancer, anti-oxidative, and neuroprotective functions (Wagh et al., 2021). This has made celastrol an appealing therapeutic drug for treating cancer, inflammatory conditions, and autoimmune disorders. For instance, celastrol has been indicated to inhibit inflammation by suppressing the reactive oxygen species (ROS)/nuclear factor kappa B subunit 1(NF-κB)/NLR family pyrin domain containing 3 (NLRP3) axis to mitigate rheumatoid arthritis (Jing et al., 2021). Notably, a previous study revealed that celastrol could suppress inflammation and alleviate renal injury to protect the kidney of diabetic rats by blocking the mitogen-activated protein kinase (MAPK)/NF-κB pathway (Zhang et al., 2019). Further, celastrol has been recently demonstrated to mitigate renal injury, suppress glomerular basement membrane thickening, and assist podocyte homeostasis to delay the progression of early DN in rats (Nie et al., 2020). Another study revealed the inhibition of proteinuria and the kidney-protective effects of celastrol in the treatment of DN (Liu et al., 2021). Despite these findings, the specific mechanisms underlying the anti-DN role of celastrol have not yet been fully illustrated. This prompted us to further investigate the efficacy of celastrol against DN and its associated mechanisms.
In recent years, network pharmacology has been widely employed in the investigation and development of traditional Chinese medicines. Network pharmacology aims to systematically exhibit the mechanism of drug intervention in disease networks by integrating biology, computer science, and bioinformatics, to name a few (Lin et al., 2021).
In this study, we investigated the therapeutic effects of celastrol on DN and related mechanisms. We also conducted in vitro experiments to verify the efficacy of celastrol and its mechanisms in the treatment of DN. We aimed to uncover the pharmacological mechanisms of the anti-DN role of celastrol through network pharmacology and molecular docking, thereby providing novel targets for treating DN.
Screening of celastrol-related targets
Herbal Ingredients’ Targets (HIT) database (version 2.0; http://hit2.badd-cao.net) is a comprehensive database to offer integrated information about medicinal herbs, herb-active compounds, and protein targets under different experimental conditions (Yan et al., 2022; Ye et al., 2011). GeneCards database (version 3.0; https://www.genecards.org/) is another integrative platform to provide annotated and predicted human gene information (Safran et al., 2010). The target genes of celastrol were acquired from HIT and GeneCards databases employing the keywords “Celastrol” and “Tripterine”, and only “Homo sapiens” target genes were selected. Celastrol-related targets were standardized in the UniProt database (https://www.uniprot.org/) and the duplications were removed.
Screening of diabetic nephropathy-related targets
DisGeNET (version 7.0; http://www.disgenet.org/) is a resource platform covering massive data about genes and variants related to human diseases. Therapeutic Targets Database (TTD; https://idrblab.org/ttd/) is focused on providing data about the known and investigated therapeutic protein targets, the targeted disease, the pathway, and the corresponding drugs/ligands directed at each of the targets (Chen et al., 2002; Zhou et al., 2022). Information about the DN-related target genes was collected using the term “Diabetic nephropathy” as queries from GeneCards, DisGeNET, and TTD platforms, with the species set to “Homo sapiens”. DN-related targets were standardized in the UniProt database and the duplications were removed.
Identification of potential targets of celastrol in the treatment of diabetic nephropathy
VennDiagram (version 1.7.3; http://bioinfogp.cnb.csic.es/tools/venny/index.html) is a widely applied tool in biological analysis that presents the differences between gene lists from distinct differential analyses (Wang et al., 2021). Screened celastrol-related and DN-related were imported into VennDiagram and intersection targets were obtained for further analysis. The network of intersection targets was visualized using Cytoscape software (version 3.8.2).
Construction of the protein-protein interaction network
PPI network was constructed by importing the celastrol-DN intersection genes into the STRING database (https://string-db.org/), which covers nearly all the interactions between proteins (Szklarczyk et al., 2021). Protein interactions were analyzed using ggraph (version 2.0.5) and igraph (version 1.3.1) R packages. The nodes of the PPI network denote proteins, and the edges denote the interactions between two proteins. The organism was limited to “Homo sapiens”, and the confidence score was set to “>0.4” (Ye et al., 2021; Khan et al., 2022). The other parameters were set to default (network type, full STRING network; size cutoff, no more than 10 interactors). Additionally, the cytoHubba (version 0.1) plugin was used to identify the hub nodes (top 10) in the PPI network. The following parameters of cytoHubba plugin were applied: ranking method, maximal clique centrality (MCC); Hubba nodes, and the top 10 nodes ranked by MCC (default).
Gene ontology functional and kyoto encyclopedia of genes and genomes pathway enrichment analyses
The GO database (http://geneontology.org) was used for gene function analysis including biological process (BP), cellular component (CC), and molecular function (MF) analyses. The KEGG database (https://www.kegg.jp) was utilized to determine biological functions and candidate targets. In this research study, GO functional annotation and KEGG pathway analyses were performed using ClusterProfiler (https://bioconductor.org/packages/release/clusterProfiler.html) in the R package. Furthermore, a hypergeometric test was used to evaluate the association of a specific gene ontology term or biological pathway with the query genes. The hypergeometric distribution formula applied is as follows:
where N is the total number of genes; M is the number of annotated genes in GO and KEGG pathways; n is the number of explored target genes of celastrol; k is the number of common genes of celastrol and annotated genes. A p value < 0.05 was considered to be significantly associated.
The cytoHubba plugin was used to screen out the top 10 hub genes obtained from the GO functional annotation and KEGG pathway analyses. The parameters of the cytoHubba plugin were set to “ranking method: MCC; Hubba nodes: top 10 nodes ranked by MCC (default)”. The circlize (version 0.4.15) R package (Gu et al., 2014) was utilized to visualize the network of the top 10 hub genes.
Molecular docking was conducted to find out the underlying interactions between celastrol and DN. Firstly, the crystal structures of the target proteins were downloaded from RCSB Protein Data Bank (PDB) (https://www.rcsb.org/), while the structure of celastrol in the mol2format was obtained from ZINC (https://zinc.docking.org/) (Ye et al., 2021). Subsequently, PyMOL (version 2.5) and AutoDockTools (version 1.5.6) software were used to remove water, eliminate the original ligands of the active center, hydrogenate, repair broken chains, optimize amino acids, and calculate the charges of the target proteins (Seeliger and de Groot, 2010). All the structures were saved in PDBQT format using Open Babel GUI software. All the flexible bonds of the small molecule ligands were set to be rotatable, whereas the receptors were set to be rigid. AutoDock Vina is a novel optimized molecular docking and virtual screening software, which uses a complicated gradient optimization approach to enhance the accuracy and speed of molecular docking in the course of the local optimization (Shang et al., 2023). We used AutoDock Vina for molecular docking and PyMOL for the visualization of docking results. The binding receptor-ligand activity was evaluated by the value of the binding energy. The lower the binding energy is, the more stable is the receptor-ligand binding as per reports (He et al., 2021). In this study, docking pairs that simultaneously met the criteria of binding energy <−5.0 kcal/mol and the formation of hydrogen bonds (Feng et al., 2021) were considered effective docking and reserved for further analysis.
Cell culture, diabetic nephropathy induction and celastrol treatment
The rat renal tubular epithelial cell line NRK-52E, was purchased from the American Type Culture Collection (Manassas, VA, USA). NRK-52E cells were cultured in Dulbecco’s Modified Eagle Medium (DMEM) supplemented with 10% fetal bovine serum (FBS) and 1% penicillin-streptomycin mixture at 37°C in 5% CO2. High glucose (HG) in the concentration of 30 mmol/L was used to induce a cell model of DN. Cells treated with 5.5 mmol/L glucose served as controls. Subsequently, the Celastrol (purity ≥ 98%; #IC0220) was purchased from Solarbio (Beijing, China). HG-induced cells were treated with different doses of celastrol (0.5, 1, and 2 μM). The doses of celastrol used were based on previous research (Fang and Chang, 2021).
A CCK-8 kit (#C0037, Beyotime, Shanghai, China) was used to probe the NRK-52E cell viability according to the manufacturer’s instructions. Briefly, 100 µL of NRK-52E cells were seeded in 96-well plates (2,000 cells/well), treated with various concentrations of celastrol, and cultured at 37°C in 5% CO2 for 24 h. The cells were then incubated with 10 µL of CCK-8 solution for another 2 h. Cell viability was evaluated using a microplate reader (DR-3518G, Hiwell Diatek, Wuxi, China).
Annexin V-fluorescein isothiocyanate (FITC) Cell Apoptosis Detection Kit (#C1062S, Beyotime) was used to assess NRK-52E cell apoptosis. After washing with phosphate-buffered saline (PBS) twice, cells were suspended in a binding buffer (300 µL). The cells were then stained with Annexin V-FITC (5 μL) for 15 min and propidium iodide (PI; 10 µL) for 10 min at 25°C in the dark. Cell apoptosis was assessed using a CytoFLEX S flow cytometer (Beckman, FL, USA) and then quantified using Cell Quest software (BD Biosciences, NJ, USA).
Quantitative real-time polymerase chain reaction
Total RNA was isolated from NRK-52E cells using the TRIzol reagent (#15596018, Invitrogen, CA, USA). Reverse transcription was performed for cDNA synthesis using the FastKing-RT SuperMix (#KR118-02, Tiangen, Beijing, China). qRT-PCR analysis was then carried out with the SYBR Green PCR Master Mix (#4364344; Thermo Fisher Scientific, MA, USA) on a CFX Connect Real-Time PCR Detection System (Bio-Rad, CA, USA). The following reaction program was applied: 95°C for 3 min, followed by 95°C for 12 s, and then 62°C for 40 s; 40 cycles. The gene expression was quantified using the 2−ΔΔCt method. Glyceraldehyde-3-phosphate dehydrogenase (GAPDH) was used as an internal reference. Primers used in this study are listed in Table 1.
Data were exhibited as mean ± standard deviation and analyzed using GraphPad 7.0 software (La Jolla, CA, USA). We applied one-way analysis of variance (ANOVA) followed by Tukey’s tests to compare differences between groups. p < 0.05 indicated statistical significance.
Selection of celastrol-diabetic nephropathy intersection targets
As outlined above, HIT and GeneCards databases were used for screening the potential celastrol-DN-related targets. A total of 144 target genes linked with celastrol were acquired after UniProt standardization and duplication deletion (Suppl. Table S1). Moreover, we obtained 939 DN-related target genes after eliminating nonstandard and duplicate targets (Suppl. Table S2). Finally, 66 intersection targets were screened out from the identified celastrol-related and DN-related targets (Fig. 1). The 66 candidate targets are listed in Suppl. Table S3.
Figure 1: Venn diagram (A) and network (B) of celastrol-DN intersection targets. Key: DN, diabetic nephropathy.
Protein-protein Interaction network analysis of celastrol-diabetic nephropathy intersection targets
To probe the interactions among the identified targets (n = 66), the PPI network was constructed using the STRING database. The obtained PPI network contained 66 nodes (proteins) and 1,168 edges (interaction pairs). The top 10 core network proteins (red nodes) were acquired using the cytoHubba plugin. These ten genes included interleukin 6 (IL6), Jun proto-oncogene (JUN), caspase 3 (CASP3), prostaglandin-endoperoxide synthase 2 (PTGS2), matrix metallopeptidase 9 (MMP9), signal transducer and activator of transcription 3 (STAT3), interleukin 1 beta (IL1B), mitogen-activated protein kinase 3 (MAPK3), tumor necrosis factor (TNF), and AKT serine/threonine kinase 1 (AKT1). Furthermore, some of these candidate genes such as JUN and STAT3 are transcriptional regulatory factors involved in cell growth (Fig. 2).
Figure 2: PPI network analysis of celastrol-DN intersection targets using STRING database. Red nodes represent the top 10 hub genes. Key: PPI, protein-protein interaction; DN, diabetic nephropathy.
Gene ontology functional and kyoto encyclopedia of genes and genomes pathway enrichment analyses
To investigate the potential functions and mechanisms of the 66 targets obtained in DN, the GO functional annotation and KEGG pathway analyses were performed. Based on GO function analysis, a total of 1860 BP, 95 MF, and 33 CC terms were obtained (p value < 0.05). As shown in Fig. 3A, the top 10 enriched GO functions were respectively selected from the BP, MF, and CC terms. Meanwhile, 164 enriched pathways were acquired through KEGG pathway analysis (c < 0.05). As displayed in Fig. 3B, the top 30 enriched pathways were then screened (p value < 0.05). KEGG results revealed that the 66 candidate targets were mainly correlated with AGE-RAGE, TNF, IL-17, and MAPK signaling pathways. Detailed information about the critical GO functions and KEGG pathways is provided in Tables 2 and 3, respectively.
Figure 3: GO functional and KEGG pathway enrichment analyses. (A) Top 10 enriched BP, CC, and MF terms from GO functional enrichment analysis. (B) Top 30 enriched pathways from KEGG pathway enrichment analysis. Key: GO, Gene Ontology; KEGG, Kyoto Encyclopedia of Genes and Genomes; BP, biological process; CC, cellular component; MF, molecular function.
Determination of hub genes based on Gene Ontology functional and kyoto encyclopedia of genes and genomes pathway enrichment analyses
To further ascertain which target plays a pivotal role in the 30 vitally enriched biological functions and pathways, the cytoHubbaplugin was used to screen the hub targets. The top 10 GO function-related targets were selected according to MCC scores. These were mitogen-activated protein kinase 1 (MAPK1), MAPK3, TNF, Janus kinase 2 (JAK2), Fas ligand (FASLG), mitogen-activated protein kinase 14 (MAPK14), transforming growth factor beta 1 (TGFB1), IL6, C-C motif chemokine ligand (CCL3), and AKT1 (MCC score ≥ 12) (Fig. 4A). Additionally, the top 10 KEGG pathway-related targets were also screened based on MCC scores, including nuclear factor kappa B subunit 1 (NFKB1), nuclear factor kappa B subunit p65 (RELA), AKT1, inhibitor of nuclear factor kappa B kinase subunit beta (IKBKB), mitogen-activated protein kinase 8 (MAPK8), MAPK3, MAPK1, TNF, MAPK14, and JUN (MCC score ≥ 12) (Fig. 4B).
Figure 4: Determination of hub targets originating from GO functional (A) and KEGG pathway (B) enrichment analyses. Key: GO, Gene Ontology; KEGG, Kyoto Encyclopedia of Genes and Genomes.
Determination of potential targets and molecular docking verification
To further assess the vital candidate targets, molecular docking was conducted to evaluate the reliability of the anti-DN targets of celastrol. Three intersection targets (MAPK3, TNF, and AKT1) were obtained from the hub target networks of PPI, GO, and KEGG (Fig. 5A). A binding energy value lower than −5 kcal/mol denoted good binding activity while a binding energy value lower than −7 kcal/mol denoted strong activity (Gai et al., 2022). The molecular docking results revealed that celastrol had a favorable binding ability with MAPK3 (binding energy = −7.03 kcal/mol), TNF (binding energy = −7.15 kcal/mol), and AKT1 (binding energy = −7.97 kcal/mol) (Table 4 and Fig. 5B).
Figure 5: Determination of hub targets and molecular docking assesment. (A) Acquisition of intersection targets from PPI, GO, and KEGG hub target networks. (B) Molecular models of the binding of celastrol with MAPK3, TNF, and AKT1. Key: PPI, protein-protein interaction; GO, Gene Ontology; KEGG, Kyoto Encyclopedia of Genes and Genomes; MAPK3, mitogen-activated protein kinase 3; TNF, tumor necrosis factor; AKT1, AKT serine/threonine kinase 1.
In vitro anti-DN effects of celastrol
To verify the therapeutic effects of celastrol on DN in vitro, 30 mmol/L HG was used to induce a cell model of DN, and cells were then treated with celastrol at different doses (0.5, 1, and 2 μM). Results of the CCK-8 assay showed that HG treatment significantly inhibited NRK-52E cell viability (p < 0.01), which was rescued by celastrol in a dose-dependent manner (p < 0.05; Fig. 6A). According to flow cytometry analysis, while HG markedly promoted the apoptosis of NRK-52E cells (p < 0.01), celastrol reversed this HG-induced cell apoptosis in a dose-dependent manner (p < 0.05; Fig. 6B). Further, qRT-PCR results showed that the mRNA expression levels of MAPK3, TNF, and AKT1 in NRK-52E cells were observably elevated by HG treatment (p < 0.01; Fig. 6C). Similarly, celastrol was found to decrease the mRNA expression levels of MAPK3, TNF, and AKT1 in HG-treated NRK-52E cells in a dose-dependent manner (p < 0.05; Fig. 6C).
Figure 6: In vitro therapeutic effects of celastrol on DN. (A) Detection of NRK-52E cell viability using CCK-8. (B) Detection of NRK-52E cell apoptosis using flow cytometry. (C) Detection of the mRNA expression levels of MAPK3, TNF, and AKT1 in NRK-52E cells using qRT-PCR. Data were exhibited as mean ± standard deviation. *p < 0.05 and **p < 0.01 vs. Control group; ##p < 0.01 vs. HG group; &p < 0.05 vs. HG + celastrol (1 μM) group. Key: DN, diabetic nephropathy; CCK-8, cell counting kit-8; MAPK3, mitogen-activated protein kinase 3; TNF, tumor necrosis factor; AKT1, AKT serine/threonine kinase 1; qRT-PCR, quantitative real-time polymerase chain reaction.
DN is one of the most common microvascular complications of diabetes mellitus, manifesting as thickened glomerular basement membrane, enhanced extracellular matrix formation, and podocyte loss (Han et al., 2017). DN tends to develop into ESRD which has high morbidity and mortality rates (Zhu et al., 2021; Noor et al., 2021). Natural bioactive compounds such as terpenoids have exhibited their potential to treat DN. For example, paeoniflorin could inhibit TLR4-mediated inflammation and ameliorate the clinical symptoms and the severity of DN (Shao et al., 2019). In another study, catalpol could mitigate endothelial dysfunction and inflammation to impede the progression of DN (Shu et al., 2021). Celastrol, a quinone methide triterpene, extracted from Tripterygium wilfordii has been shown to possess various pharmacological properties, including anti-inflammatory, anti-autoimmune, anti-cancer, and neuroprotective activities (Wagh et al., 2021; Lim et al., 2021; Gu et al., 2018). Further, celastrol has been extensively used in the treatment of autoimmune diseases, such as rheumatoid arthritis (Jing et al., 2021) and systemic lupus erythematosus (Song et al., 2020). An increasing number of studies indicated that celastrol could alleviate the pathological injury in DN (Nie et al., 2020; Zhan et al., 2018). Moreover, a recent study has elaborated that CN suppressed proteinuria, and showed anti-inflammatory and kidney-protective functions celastrol in DN (Liu et al., 2021). However, the mechanisms underlying the anti-DN effects of celastrol have not yet been fully elucidated. Therefore, it is essential to further explore the therapeutic effects of celastrol and its mechanisms in the treatment of DN.
In this study, sixty-six intersection targets of celastrol and DN were screened based on public databases. In the PPI network of 66 targets, IL6, JUN, CASP3, PTGS2, MMP9, STAT3, IL1B, MAPK3, TNF, and AKT1 were identified as the top 10 core targets for celastrol in the treatment of DN. The hub targets, such as IL6, IL1B, and TNF, were mainly associated with inflammatory and immune responses. Chronic low-grade inflammation has been a crucial characteristic of DN and a failure in addressing inflammation is a primary contributing factor in the constant development of DN (Wu et al., 2021). A previous study demonstrated that celastrol could reduce levels of IL6, IL1B, and TNF to attenuate oxidative damage of type 2 diabetes (Cascão et al., 2017). Further evidence indicated that down-regulation of IL6, IL1, and TNF can alleviate the pathological injury of DN (Wu et al., 2020; Sun et al., 2020). Another study showed that the inhibition of the STAT3 pathway-mediated inflammation can attenuate DN (Zhang et al., 2021). Further, celastrol was found to effectively inhibit the secretion of pro-inflammatory cytokines, including IL6, IL1B, and TNF-α, thus mitigating DN (Zhan et al., 2018). This accounts for the key role of IL6, IL1B, and TNF in treating DN as revealed in our study. Collectively, these results suggest that the anti-DN effects of celastrol can be partly attributed to its potent anti-inflammatory activity.
According to the GO functional enrichment analysis, the potential targets of celastrol against DN were mainly concentrated in BPs, such as response to lipopolysaccharide, response to molecules of bacterial origin, and oxidative stress. Relevant findings have been shown in other reports. For example the development of DN was associated with lipopolysaccharide-induced inflammation (Jiang et al., 2020), gut microbiota dysbiosis (Fernandes et al., 2019), and oxidative stress (Gerardo Yanowsky-Escatell et al., 2020), which were partially in line with our findings. Notably, a previous study indicated the crosstalk between inflammation and oxidative stress mechanisms in DN pathogenesis (Samsu, 2021). Another study found that celastrol could inhibit oxidative stress to ameliorate the pathological damage of the chronic complications of diabetes (Guan et al., 2016). The core targets related to GO functions included MAPK1, MAPK3, TNF, JAK2, FASLG, MAPK14, TGFB1, IL6, CCL3, and AKT1, which mainly participate in the regulation of inflammatory responses. For instance, MAPK signaling pathways are vital for the inflammatory process, including the secretion of pro-inflammatory cytokines induced by ROS (Galganska et al., 2021). CCL3 is a chemokine that triggers various pro-inflammatory reactions such as leukocyte chemotaxis and pushes T cells into the inflammatory tissue area from blood circulation (Zhang et al., 2018b). Consistent with our data, previous data has documented the involvement of CCL3 in DN development (Araújo et al., 2020). To summarize, our findings further indicate the complexity of the pathological mechanisms of DN, including inflammatory responses, response to lipopolysaccharide, response to molecules of bacterial origin, and oxidative stress.
KEGG pathway enrichment analysis showed that celastrol could address DN primarily through AGE-RAGE, TNF, IL-17, and MAPK signaling pathways. Evidence has indicated that the increase in end products of advanced glycosylation (AGEs) and its receptor (RAGE) can contribute to the onset and development of DN and nephron cellular injury (Barocio-Pantoja et al., 2021). Notably, a recent study has demonstrated that inhibiting AGE-RAGE-mediated inflammation signaling pathways could prevent the development and progression of DN (Chen et al., 2022). Further, accumulating evidence showed that the pro-inflammatory cytokines, TNF and IL-17, play a vital part in the development and progression of DN (Park et al., 2019; Kim et al., 2021). Additionally, blocking the TNF/IL-17 axis has been proven to alleviate pathological injury of DN (Ma et al., 2019). In terms of the MAPK signaling pathway, it was found to be associated with multiple pathological mechanisms of DN, including inflammation and oxidative stress (Song et al., 2018; Zhu et al., 2020; Ma et al., 2021). Interestingly, a previous study observed that celastrol-loaded nanomicelles could ameliorate inflammation, lipid accumulation, and insulin resistance in obese mice (Zhao et al., 2019). Thus, it is reasonable to hypothesize the positive effects of celastrol on inflammation, lipid homeostasis, and insulin resistance in preventing DN progression. Besides, our data showed that NFKB1, RELA, AKT1, IKBKB, MAPK8, MAPK3, MAPK1, TNF, MAPK14, and JUN were the key celastrol targets in the KEGG analysis. Similarly, these targets, such as MAPK8, MAPK3, MAPK1, TNF, and MAPK14, are mainly involved in inflammatory responses. Collectively, these data further demonstrate that celastrol inhibits DN progression mainly by regulating inflammatory responses through the AGE-RAGE, TNF, IL-17, and MAPK signaling pathways.
In the present study, we finally obtained three overlapping targets (MAPK3, TNF, and AKT1) from the PPI, GO, and KEGG networks of the hub targets. Molecular docking showed that celastrol exhibited good binding abilities with MAPK3, TNF, and AKT1.
An increasing number of studies demonstrates that cell apoptosis is one of the major mechanisms in the development and progression of DN. Elevated apoptosis has been found in the kidneys of DN patients and suppression of the cell apoptosis in DN mice could mitigate DN symptoms (Fan et al., 2022). It was previously revealed that celastrol pre-treatment reversed HG-induced cell apoptosis to ameliorate the pathological injury of DN (Zhan et al., 2018). Consistent with this report, our data also showed that celastrol significantly inhibited the apoptosis of HG-treated NRK-52E cells. Additionally, we found that the viability of HG-treated NRK-52E cells was suppressed compared with control cells, which was however reversed by celastrol treatment.
MAPK3, also known as ERK1, participates in a wide range of cellular processes including proliferation, inflammation, and cellular metabolism (Chen et al., 2019; Kassouf and Sumara, 2020). Further, MAPK3/MAPK1 signaling could enhance pancreatic β-cell mass and insulin production (Kassouf and Sumara, 2020). TNF, which is a common pro-inflammatory cytokine acts as a switch in immunity (Fischer et al., 2020). Therefore, TNF inhibitors have been developed and clinically applied to treat inflammatory and autoimmune disorders (Fischer et al., 2020). AKT1 is a key mediator of cellular survival and growth, which is involved in inflammatory responses (Zhang et al., 2021; Vergadi et al., 2017). An animal model-based investigation indicated that celastrol could suppress the expression of pro-inflammatory cytokines (e.g., TNF-α) to mitigate neuropathic pain in rats (Jin et al., 2022). Furthermore, a recent study has demonstrated that downregulation of the levels of MAPK3 and TNF by blocking AKT1 signaling could prevent the progression of DN (Li and Xu, 2022). We observed that celastrol effectively decreased the expression of MAPK3, TNF, and AKT1 in HG-treated NRK-52E cells. This was similar to that reported in research (Jin et al., 2022; Li and Xu, 2022). Collectively, these findings indicate that celastrol can be used to treat DN where MAPK3, TNF, and AKT1 are the pivotal therapeutic targets in its action.
Our study has some limitations. First, the data used in this study were from public databases that need to be updated in real-time. This makes it possible that some other vital targets or pathways associated with DN might have been overlooked. Second, we only verified the in vitro therapeutic effects of celastrol on DN without in vivo validation. Third, the crosstalk between the core mechanisms (e.g., inflammation and oxidative stress) revealed in this study remains to be further elucidated. These limitations will be perfected and addressed in our subsequent studies.
This study reveals that celastrol exerts therapeutic effects on DN through multiple pathways and mechanisms, including AGE-RAGE, TNF, IL-17, and MAPK signaling pathways, the inflammatory and immune response, and oxidative stress. MAPK3, TNF, and AKT1 are the foremost targets of celastrol against DN. Additionally, the anti-DN effects of celastrol and its therapeutic targets were confirmed in vitro. Our study provides a direction for follow-up animal and clinical studies, deepens our insights into the mechanisms related to DN, and furnishes a reference for developing celastrol as a novel drug for the treatment of DN.
Funding Statement: This work was supported by the Zhejiang Province Chinese Medicine Modernization Program Grant [Number 2020ZX001] and the “Pioneer” and “Leading Goose” R&D Program of Zhejiang Grant [Number 2023C03075].
Author Contributions: The authors confirm their contribution to the paper as follows: study conception and design: FQ, PR, and JJ; data collection: FQ, PR, LZ, DZ, and WH; analysis and interpretation of results: FQ and JJ; draft manuscript preparation: FQ. All authors reviewed the results and approved the final version of the manuscript.
Availability of Data and Materials: The datasets used and/or analyzed during the current study are available from the corresponding author upon reasonable request.
Ethics Approval: Not applicable.
Conflicts of Interest: The authors declare that they have no conflicts of interest to report regarding the present study.
Supplementary Materials: The supplementary materials are available online at DOI: 103.32604/biocell.2023.029353.
References
Araújo LS, Torquato BGS, da Silva CA, Dos Reis Monteiro MLG, Dos Santos Martins ALM, da Silva MV, Dos Reis MA, Machado JR (2020). Renal expression of cytokines and chemokines in diabetic nephropathy. BMC Nephrology 21: 308. https://doi.org/10.1186/s12882-020-01960-0 [Google Scholar] [PubMed] [CrossRef]
Barocio-Pantoja M, Quezada-Fernández P, Cardona-Müller D, Jiménez-Cázarez MB, Larios-Cárdenas M et al. (2021). Green tea extract increases soluble RAGE and improves renal function in patients with diabetic nephropathy. Journal of Medicinal Food 24: 1264–1270. [Google Scholar] [PubMed]
Block TJ, Batu D, Cooper ME (2022). Recent advances in the pharmacotherapeutic management of diabetic kidney disease. Expert Opinion on Pharmacotherapy 23: 791–803. https://doi.org/10.1080/14656566.2022.2054699 [Google Scholar] [PubMed] [CrossRef]
Cascão R, Fonseca JE, Moita LF (2017). Celastrol: A spectrum of treatment opportunities in chronic diseases. Frontiers in Medicine 4: 69. https://doi.org/10.3389/fmed.2017.00069 [Google Scholar] [PubMed] [CrossRef]
Chen Z, Gao YJ, Hou RZ, Ding DY, Song DF, Wang DY, Feng Y (2019). MicroRNA-206 facilitates gastric cancer cell apoptosis and suppresses cisplatin resistance by targeting MAPK2 signaling pathway. European Review for Medical and Pharmacological Sciences 23: 171–180. [Google Scholar]
Chen X, Ji ZL, Chen YZ (2002). TTD: Therapeutic target database. Nucleic Acids Research 30: 412–415. https://doi.org/10.1093/nar/30.1.412 [Google Scholar] [PubMed] [CrossRef]
Chen J, Peng H, Chen C, Wang Y, Sang T et al. (2022). NAG-1/GDF15 inhibits diabetic nephropathy via inhibiting AGE/RAGE-mediated inflammation signaling pathways in C57BL/6 mice and HK-2 cells. Life Sciences 311: 121142. https://doi.org/10.1016/j.lfs.2022.121142 [Google Scholar] [PubMed] [CrossRef]
Chen J, Yang Y, Lv Z, Shu A, Du Q, Wang W, Chen Y, Xu H (2020). Study on the inhibitive effect of Catalpol on diabetic nephropathy. Life Sciences 257: 118120. https://doi.org/10.1016/j.lfs.2020.118120 [Google Scholar] [PubMed] [CrossRef]
Fan Y, Fan H, Li P, Liu Q, Huang L, Zhou Y (2022). Mitogen-activating protein kinase kinase kinase kinase-3, inhibited by Astragaloside IV through H3 lysine 4 monomethylation, promotes the progression of diabetic nephropathy by inducing apoptosis. Bioengineered 13: 11517–11529. https://doi.org/10.1080/21655979.2022.2068822 [Google Scholar] [PubMed] [CrossRef]
Fang J, Chang X (2021). Celastrol inhibits the proliferation and angiogenesis of high glucose-induced human retinal endothelial cells. Biomedical Engineering Online 20: 65. https://doi.org/10.1186/s12938-021-00904-5 [Google Scholar] [PubMed] [CrossRef]
Feng C, Zhao M, Jiang L, Hu Z, Fan X (2021). Mechanism of modified Danggui Sini Decoction for knee osteoarthritis based on network pharmacology and molecular docking. Evidence-Based Complementary and Alternative Medicine 2021: 6680637. https://doi.org/10.1155/2021/6680637 [Google Scholar] [PubMed] [CrossRef]
Fernandes R, Viana SD, Nunes S, Reis F (2019). Diabetic gut microbiota dysbiosis as an inflammaging and immunosenescence condition that fosters progression of retinopathy and nephropathy. Biochimica et Biophysica Acta-Molecular Basis of Disease 1865: 1876–1897. [Google Scholar] [PubMed]
Fischer R, Kontermann RE, Pfizenmaier K (2020). Selective targeting of TNF receptors as a novel therapeutic approach. Frontiers in Cell and Developmental Biology 8: 401. https://doi.org/10.3389/fcell.2020.00401 [Google Scholar] [PubMed] [CrossRef]
Gai J, Xing J, Wang Y, Lei J, Zhang C, Zhang J, Tang J (2022). Exploration of potential targets and mechanisms of Naringenin in treating autism spectrum disorder via network pharmacology and molecular docking. Medicine 101: e31787. https://doi.org/10.1097/MD.0000000000031787 [Google Scholar] [PubMed] [CrossRef]
Galganska H, Jarmuszkiewicz W, Galganski L (2021). Carbon dioxide inhibits COVID-19-type proinflammatory responses through extracellular signal-regulated kinases 1 and 2, novel carbon dioxide sensors. Cellular and Molecular Life Sciences 78: 8229–8242. https://doi.org/10.1007/s00018-021-04005-3 [Google Scholar] [PubMed] [CrossRef]
Gerardo Yanowsky-Escatell F, Andrade-Sierra J, Pazarín-Villaseñor L, Santana-Arciniega C, de Jesús Torres-Vázquez E, Samuel Chávez-Iñiguez J, Ángel Zambrano-Velarde M, Martín Preciado-Figueroa F (2020). The role of dietary antioxidants on oxidative stress in diabetic nephropathy. Iranian Journal of Kidney Diseases 14: 81–94. [Google Scholar] [PubMed]
Gu Z, Gu L, Eils R, Schlesner M, Brors B (2014). circlize implements and enhances circular visualization in R. Bioinformatics 30: 2811–2812. https://doi.org/10.1093/bioinformatics/btu393 [Google Scholar] [PubMed] [CrossRef]
Gu L, Kwong JMK, Yadegari D, Yu F, Caprioli J, Piri N (2018). The effect of celastrol on the ocular hypertension-induced degeneration of retinal ganglion cells. Neuroscience Letters 670: 89–93. https://doi.org/10.1016/j.neulet.2018.01.043 [Google Scholar] [PubMed] [CrossRef]
Guan Y, Cui ZJ, Sun B, Han LP, Li CJ, Chen LM (2016). Celastrol attenuates oxidative stress in the skeletal muscle of diabetic rats by regulating the AMPK-PGC1α-SIRT3 signaling pathway. International Journal of Molecular Medicine 37: 1229–1238. https://doi.org/10.3892/ijmm.2016.2549 [Google Scholar] [PubMed] [CrossRef]
Guo HB, Peng JQ, Xuan W, Zhang KK, Zhong GZ, Chen WH, Shi GX (2021a). Efficacy of tripterygium glycosides for diabetic nephropathy: A meta-analysis of randomized controlled trials. BMC Nephrology 22: 304. https://doi.org/10.1186/s12882-021-02487-8 [Google Scholar] [PubMed] [CrossRef]
Guo L, Zhang Y, Al-Jamal KT (2021b). Recent progress in nanotechnology-based drug carriers for celastrol delivery. Biomaterials Science 9: 6355–6380. https://doi.org/10.1039/D1BM00639H [Google Scholar] [PubMed] [CrossRef]
Han Q, Zhu H, Chen X, Liu Z (2017). Non-genetic mechanisms of diabetic nephropathy. Frontiers in Medicine 11: 319–332. https://doi.org/10.1007/s11684-017-0569-9 [Google Scholar] [PubMed] [CrossRef]
He D, Li Q, Du G, Sun J, Meng G, Chen S (2021). Research on the mechanism of guizhi to treat nephrotic syndrome based on network pharmacology and molecular docking technology. Biomed Research International 2021: 8141075. https://doi.org/10.1155/2021/8141075 [Google Scholar] [PubMed] [CrossRef]
Hu T, Yue J, Tang Q, Cheng KW, Chen F, Peng M, Zhou Q, Wang M (2022). The effect of quercetin on diabetic nephropathy (DNA systematic review and meta-analysis of animal studies. Food & Function 13: 4789–4803. https://doi.org/10.1039/D1FO03958J [Google Scholar] [PubMed] [CrossRef]
Jiang Y, Yang L, Yang X, Yin S, Zhuang F, Liu Z, Wang Y, Liang G, Qian J (2020). The imidazopyridine derivative X22 prevents diabetic kidney dysfunction through inactivating NF-κB signaling. Biochemical and Biophysical Research Communications 525: 877–882. https://doi.org/10.1016/j.bbrc.2020.03.016 [Google Scholar] [PubMed] [CrossRef]
Jin GJ, Peng X, Chen ZG, Wang YL, Liao WJ (2022). Celastrol attenuates chronic constrictive injury-induced neuropathic pain and inhibits the TLR4/NF-κB signaling pathway in the spinal cord. Journal of Natural Medicines 76: 268–275. https://doi.org/10.1007/s11418-021-01564-4 [Google Scholar] [PubMed] [CrossRef]
Jing M, Yang J, Zhang L, Liu J, Xu S et al. (2021). Celastrol inhibits rheumatoid arthritis through the ROS-NF-κB-NLRP3 inflammasome axis. International Immunopharmacology 98: 107879. https://doi.org/10.1016/j.intimp.2021.107879 [Google Scholar] [PubMed] [CrossRef]
Johnson SA, Spurney RF (2015). Twenty years after ACEIs and ARBs: Emerging treatment strategies for diabetic nephropathy. American Journal of Physiology-Renal Physiology 309: F807–F820. https://doi.org/10.1152/ajprenal.00266.2015 [Google Scholar] [PubMed] [CrossRef]
Kassouf T, Sumara G (2020). Impact of conventional and atypical MAPKs on the development of metabolic diseases. Biomolecules 10: 1256. https://doi.org/10.3390/biom10091256 [Google Scholar] [PubMed] [CrossRef]
Khan NU, Lin J, Liu X, Li H, Lu W, Zhong Z, Zhang H, Waqas M, Shen L (2020). Insights into predicting diabetic nephropathy using urinary biomarkers. Biochimica et Biophysica Acta (BBA)—Proteins and Proteomics 1868: 140475. [Google Scholar] [PubMed]
Khan SA, Wu Y, Li AS, Fu XQ, Yu ZL (2022). Network pharmacology and molecular docking-based prediction of active compounds and mechanisms of action of Cnidii Fructus in treating atopic dermatitis. BMC Complementary Medicine and Therapies 22: 275. https://doi.org/10.1186/s12906-022-03734-7 [Google Scholar] [PubMed] [CrossRef]
Kim KH, Hong GL, Jung DY, Karunasagara S, Jeong WI, Jung JY (2021). IL-17 deficiency aggravates the streptozotocin-induced diabetic nephropathy through the reduction of autophagosome formation in mice. Molecular Medicine 27: 25. https://doi.org/10.1186/s10020-021-00285-4 [Google Scholar] [PubMed] [CrossRef]
Li F, Chen Y, Li Y, Huang M, Zhao W (2020). Geniposide alleviates diabetic nephropathy of mice through AMPK/SIRT1/NF-κB pathway. European Journal of Pharmacology 886: 173449. https://doi.org/10.1016/j.ejphar.2020.173449 [Google Scholar] [PubMed] [CrossRef]
Li S, Xu G (2022). Qishen Yiqi dripping pill protects diabetic nephropathy by inhibiting the PI3K-AKT signaling pathways in rats. Evidence-Based Complementary and Alternative Medicine 2022: 6239829. https://doi.org/10.1155/2022/6239829 [Google Scholar] [PubMed] [CrossRef]
Lim HY, Ong PS, Wang L, Goel A, Ding L, Li-Ann Wong A, Ho PC, Sethi G, Xiang X, Goh BC (2021). Celastrol in cancer therapy: Recent developments, challenges and prospects. Cancer Letters 521: 252–267. https://doi.org/10.1016/j.canlet.2021.08.030 [Google Scholar] [PubMed] [CrossRef]
Lin D, Zeng Y, Tang D, Cai Y (2021). Study on the mechanism of Liuwei Dihuang pills in treating Parkinson’s disease based on network pharmacology. BioMed Research International 2021: 4490081. [Google Scholar] [PubMed]
Liu P, Zhang J, Wang Y, Shen Z, Wang C, Chen DQ, Qiu X (2021). The active compounds and therapeutic target of Tripterygium wilfordii Hook. f. in attenuating proteinuria in diabetic nephropathy: A review. Frontiers in Medicine 8: 747922. https://doi.org/10.3389/fmed.2021.747922 [Google Scholar] [PubMed] [CrossRef]
Lu Y, Liu Y, Zhou J, Li D, Gao W (2021a). Biosynthesis, total synthesis, structural modifications, bioactivity, and mechanism of action of the quinone-methide triterpenoid celastrol. Medicinal Research Reviews 41: 1022–1060. https://doi.org/10.1002/med.21751 [Google Scholar] [PubMed] [CrossRef]
Lu L, Zhong Z, Gu J, Nan K, Zhu M, Miao C (2021b). ets1 associates with KMT5A to participate in high glucose-mediated EndMT via upregulation of PFN2 expression in diabetic nephropathy. Molecular Medicine 27: 74. https://doi.org/10.1186/s10020-021-00339-7 [Google Scholar] [PubMed] [CrossRef]
Ma J, Li YJ, Chen X, Kwan T, Chadban SJ, Wu H (2019). Interleukin 17A promotes diabetic kidney injury. Scientific Reports 9: 2264. https://doi.org/10.1038/s41598-019-38811-4 [Google Scholar] [PubMed] [CrossRef]
Ma L, Wu F, Shao Q, Chen G, Xu L, Lu F (2021). Baicalin alleviates oxidative stress and inflammation in diabetic nephropathy via Nrf2 and MAPK signaling pathway. Drug Design, Development and Therapy 15: 3207–3221. https://doi.org/10.2147/DDDT.S319260 [Google Scholar] [PubMed] [CrossRef]
Mohsen M, Elberry AA, Mohamed Rabea A, Abdelrahim MEA, Hussein RRS (2021). Recent therapeutic targets in diabetic nephropathy. International Journal of Clinical Practice 75: e14650. https://doi.org/10.1111/ijcp.14650 [Google Scholar] [PubMed] [CrossRef]
Nie Y, Fu C, Zhang H, Zhang M, Xie H, Tong X, Li Y, Hou Z, Fan X, Yan M (2020). Celastrol slows the progression of early diabetic nephropathy in rats via the PI3K/AKT pathway. BMC Complementary Medicine and Therapies 20: 321. https://doi.org/10.1186/s12906-020-03050-y [Google Scholar] [PubMed] [CrossRef]
Noor S, Mohammad T, Ashraf GM, Farhat J, Bilgrami AL, Eapen MS, Sohal SS, Yadav DK, Hassan MI (2021). Mechanistic insights into the role of serum-glucocorticoid kinase 1 in diabetic nephropathy: A systematic review. International Journal of Biological Macromolecules 193: 562–573. https://doi.org/10.1016/j.ijbiomac.2021.10.165 [Google Scholar] [PubMed] [CrossRef]
Park J, Guan Y, Sheng X, Gluck C, Seasock MJ et al. (2019). Functional methylome analysis of human diabetic kidney disease. JCI Insight 4: e128886. https://doi.org/10.1172/jci.insight.128886 [Google Scholar] [PubMed] [CrossRef]
Safran M, Dalah I, Alexander J, Rosen N, Iny Stein T et al. (2010). GeneCards version 3: The human gene integrator. Database 2010: baq020. https://doi.org/10.1093/database/baq020 [Google Scholar] [PubMed] [CrossRef]
Samsu N (2021). Diabetic nephropathy: Challenges in pathogenesis, diagnosis, and treatment. BioMed Research International 2021: 1497449. https://doi.org/10.1155/2021/1497449 [Google Scholar] [PubMed] [CrossRef]
Seeliger D, de Groot BL (2010). Ligand docking and binding site analysis with PyMOL and Autodock/Vina. Journal of Computer-Aided Molecular Design 24: 417–422. https://doi.org/10.1007/s10822-010-9352-6 [Google Scholar] [PubMed] [CrossRef]
Shaffner J, Chen B, Malhotra DK, Dworkin LD, Gong R (2021). Therapeutic targeting of SGLT2: A new era in the treatment of diabetes and diabetic kidney disease. Frontiers in Endocrinology 12: 749010. https://doi.org/10.3389/fendo.2021.749010 [Google Scholar] [PubMed] [CrossRef]
Shang L, Wang Y, Li J, Zhou F, Xiao K, Liu Y, Zhang M, Wang S, Yang S (2023). Mechanism of Sijunzi Decoction in the treatment of colorectal cancer based on network pharmacology and experimental validation. Journal of Ethnopharmacology 302: 115876. https://doi.org/10.1016/j.jep.2022.115876 [Google Scholar] [PubMed] [CrossRef]
Shao YX, Gong Q, Qi XM, Wang K, Wu YG (2019). Paeoniflorin ameliorates macrophage infiltration and activation by inhibiting the TLR4 signaling pathway in diabetic nephropathy. Frontiers in Pharmacology 10: 566. https://doi.org/10.3389/fphar.2019.00566 [Google Scholar] [PubMed] [CrossRef]
Shu A, Du Q, Chen J, Gao Y, Zhu Y, Lv G, Lu J, Chen Y, Xu H (2021). Catalpol ameliorates endothelial dysfunction and inflammation in diabetic nephropathy via suppression of RAGE/RhoA/ROCK signaling pathway. Chemico-Biological Interactions 348: 109625. https://doi.org/10.1016/j.cbi.2021.109625 [Google Scholar] [PubMed] [CrossRef]
Song Y, Wang X, Qin S, Zhou S, Li J, Gao Y (2018). Esculin ameliorates cognitive impairment in experimental diabetic nephropathy and induces anti-oxidative stress and anti-inflammatory effects via the MAPK pathway. Molecular Medicine Reports 17: 7395–7402. https://doi.org/10.3892/mmr.2018.8727 [Google Scholar] [PubMed] [CrossRef]
Song XQ, Zhang Y, Yang NN, Dai EQ, Wang L, Du HT (2020). Molecular mechanism of celastrol in the treatment of systemic lupus erythematosus based on network pharmacology and molecular docking technology. Life Sciences 240: 117063. https://doi.org/10.1016/j.lfs.2019.117063 [Google Scholar] [PubMed] [CrossRef]
Sun T, Liu Y, Liu L, Ma F (2020). MicroRNA-544 attenuates diabetic renal injury via suppressing glomerulosclerosis and inflammation by targeting FASN. Gene 723: 143986. https://doi.org/10.1016/j.gene.2019.143986 [Google Scholar] [PubMed] [CrossRef]
Szklarczyk D, Gable AL, Nastou KC, Lyon D, Kirsch R et al. (2021). The STRING database in 2021: Customizable protein-protein networks, and functional characterization of user-uploaded gene/measurement sets. Nucleic Acids Research 49: D605–D612. https://doi.org/10.1093/nar/gkaa1074 [Google Scholar] [PubMed] [CrossRef]
Tang G, Li S, Zhang C, Chen H, Wang N, Feng Y (2021). Clinical efficacies, underlying mechanisms and molecular targets of Chinese medicines for diabetic nephropathy treatment and management. Acta Pharmaceutica Sinica B 11: 2749–2767. https://doi.org/10.1016/j.apsb.2020.12.020 [Google Scholar] [PubMed] [CrossRef]
Trakarnvanich T, Satirapoj B, Suraamornkul S, Chirananthavat T, Sanpatchayapong A, Claimon T (2021). Effect of dipeptidyl peptidase-4 (DPP-4) inhibition on biomarkers of kidney injury and vascular calcification in diabetic kidney disease: A randomized controlled trial. Journal of Diabetes Research 2021: 7382620. https://doi.org/10.1155/2021/7382620 [Google Scholar] [PubMed] [CrossRef]
Vergadi E, Ieronymaki E, Lyroni K, Vaporidi K, Tsatsanis C (2017). Akt signaling pathway in macrophage activation and M1/M2 polarization. The Journal of Immunology 198: 1006–1014. https://doi.org/10.4049/jimmunol.1601515 [Google Scholar] [PubMed] [CrossRef]
Wagh PR, Desai P, Prabhu S, Wang J (2021). Nanotechnology-based celastrol formulations and their therapeutic applications. Frontiers in Pharmacology 12: 673209. https://doi.org/10.3389/fphar.2021.673209 [Google Scholar] [PubMed] [CrossRef]
Wang L, Wang Z, Yang Z, Yang K, Yang H (2021). Study of the active components and molecular mechanism of Tripterygium wilfordii in the treatment of diabetic nephropathy. Frontiers in Molecular Biosciences 8: 664416. https://doi.org/10.3389/fmolb.2021.664416 [Google Scholar] [PubMed] [CrossRef]
Wu L, Liu C, Chang DY, Zhan R, Sun J et al. (2021). Annexin A1 alleviates kidney injury by promoting the resolution of inflammation in diabetic nephropathy. Kidney International 100: 107–121. https://doi.org/10.1016/j.kint.2021.02.025 [Google Scholar] [PubMed] [CrossRef]
Wu X, Pan C, Chen R, Zhang S, Zhai Y, Guo H (2020). BML-111 attenuates high glucose-induced inflammation, oxidative stress and reduces extracellular matrix accumulation via targeting Nrf2 in rat glomerular mesangial cells. International Immunopharmacology 79: 106108. https://doi.org/10.1016/j.intimp.2019.106108 [Google Scholar] [PubMed] [CrossRef]
Yan D, Zheng G, Wang C, Chen Z, Mao T et al. (2022). HIT 2.0: An enhanced platform for herbal ingredients’ targets. Nucleic Acids Research 50: D1238–D1243. https://doi.org/10.1093/nar/gkab1011 [Google Scholar] [PubMed] [CrossRef]
Ye J, Li L, Hu Z (2021). Exploring the molecular mechanism of action of Yinchen Wuling powder for the treatment of hyperlipidemia, using network pharmacology, molecular docking, and molecular dynamics simulation. Biomed Research International 2021: 9965906. https://doi.org/10.1155/2021/9965906 [Google Scholar] [PubMed] [CrossRef]
Ye H, Ye L, Kang H, Zhang D, Tao L et al. (2011). HIT: Linking herbal active ingredients to targets. Nucleic Acids Research 39: D1055–D1059. https://doi.org/10.1093/nar/gkq1165 [Google Scholar] [PubMed] [CrossRef]
Zhan X, Yan C, Chen Y, Wei X, Xiao J, Deng L, Yang Y, Qiu P, Chen Q (2018). Celastrol antagonizes high glucose-evoked podocyte injury, inflammation and insulin resistance by restoring the HO-1-mediated autophagy pathway. Molecular Immunology 104: 61–68. https://doi.org/10.1016/j.molimm.2018.10.021 [Google Scholar] [PubMed] [CrossRef]
Zhang M, Chen Y, Yang MJ, Fan XR, Xie H, Zhang L, Nie YS, Yan M (2019). Celastrol attenuates renal injury in diabetic rats via MAPK/NF-κB pathway. Phytotherapy Research: PTR 33: 1191–1198. https://doi.org/10.1002/ptr.6314 [Google Scholar] [PubMed] [CrossRef]
Zhang M, He L, Liu J, Zhou L (2021). Luteolin attenuates diabetic nephropathy through suppressing inflammatory response and oxidative stress by inhibiting STAT3 pathway. Experimental and Clinical Endocrinology & Diabetes 129: 729–739. [Google Scholar]
Zhang J, Liu J, Qin X (2018a). Advances in early biomarkers of diabetic nephropathy. Revista da Associacao Medica Brasileira 64: 85–92. https://doi.org/10.1590/1806-9282.64.01.85 [Google Scholar] [PubMed] [CrossRef]
Zhang G, Liu HB, Zhou L, Cui XQ, Fan XH (2018b). CCL3 participates in the development of rheumatoid arthritis by activating AKT. European Review for Medical and Pharmacological Sciences 22: 6625–6632. [Google Scholar] [PubMed]
Zhang W, Wu Z, Qi H, Chen L, Wang T et al. (2022). Celastrol upregulated ATG7 triggers autophagy via targeting Nur77 in colorectal cancer. Phytomedicine 104: 154280. https://doi.org/10.1016/j.phymed.2022.154280 [Google Scholar] [PubMed] [CrossRef]
Zhang L, Yan T, Wang W, Wu Q, Li G, Li D, Stovall DB, Wang Y, Li Y, Sui G (2021). AKT1 is positively regulated by G-quadruplexes in its promoter and 3'-UTR. Biochemical and Biophysical Research Communications 561: 93–100. https://doi.org/10.1016/j.bbrc.2021.05.029 [Google Scholar] [PubMed] [CrossRef]
Zhao J, Luo D, Zhang Z, Fan N, Wang Y, Nie H, Rong J (2019). Celastrol-loaded PEG-PCL nanomicelles ameliorate inflammation, lipid accumulation, insulin resistance and gastrointestinal injury in diet-induced obese mice. Journal of Controlled Release 310: 188–197. https://doi.org/10.1016/j.jconrel.2019.08.026 [Google Scholar] [PubMed] [CrossRef]
Zhou Y, Zhang Y, Lian X, Li F, Wang C, Zhu F, Qiu Y, Chen Y (2022). Therapeutic target database update 2022: Facilitating drug discovery with enriched comparative data of targeted agents. Nucleic Acids Research 50: D1398–D1407. https://doi.org/10.1093/nar/gkab953 [Google Scholar] [PubMed] [CrossRef]
Zhu W, Li YY, Zeng HX, Liu XQ, Sun YT, Jiang L, Xia LL, Wu YG (2021). Carnosine alleviates podocyte injury in diabetic nephropathy by targeting caspase-1-mediated pyroptosis. International Immunopharmacology 101: 108236. https://doi.org/10.1016/j.intimp.2021.108236 [Google Scholar] [PubMed] [CrossRef]
Zhu Y, Zhu C, Yang H, Deng J, Fan D (2020). Protective effect of ginsenoside Rg5 against kidney injury via inhibition of NLRP3 inflammasome activation and the MAPK signaling pathway in high-fat diet/streptozotocin-induced diabetic mice. Pharmacological Research 155: 104746. https://doi.org/10.1016/j.phrs.2020.104746 [Google Scholar] [PubMed] [CrossRef]
Supplementary Materials
TABLE S1. Celastrol-related targets
TABLE S2. DN-related targets. DN, diabetic nephropathy
TABLE S3. Celastrol-DN intersection targets. DN, diabetic nephropathy
Cite This Article
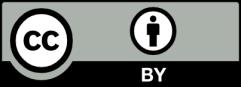
This work is licensed under a Creative Commons Attribution 4.0 International License , which permits unrestricted use, distribution, and reproduction in any medium, provided the original work is properly cited.