Open Access
REVIEW
Molecular dynamics-driven exploration of peptides targeting SARS-CoV-2, with special attention on ACE2, S protein, Mpro, and PLpro: A review
1 Green Chemistry and Sustainable Technology Cluster, Bioengineering Section, Universiti Kuala Lumpur, Malaysian Institute of Chemical and Bioengineering Technology (UniKL MICET), Alor Gajah, 78000, Malaysia
2 Institute of General and Ecological Chemistry, Faculty of Chemistry, Lodz University of Technology, Lodz, 90-924, Poland
3 Department of Chemical Science, Faculty of Science, Universiti Tunku Abdul Rahman, Kampar, 31900, Malaysia
4 Center for Agriculture and Food Research, Universiti Tunku Abdul Rahman, Kampar, 31900, Malaysia
* Corresponding Author: TSUN-THAI CHAI. Email:
(This article belongs to the Special Issue: Bioinformatics Study of Diseases)
BIOCELL 2023, 47(8), 1727-1742. https://doi.org/10.32604/biocell.2023.029272
Received 10 February 2023; Accepted 12 April 2023; Issue published 28 August 2023
Abstract
Molecular dynamics (MD) simulation is a computational technique that analyzes the movement of a system of particles over a given period. MD can provide detailed information about the fluctuations and conformational changes of biomolecules at the atomic level over time. In recent years, MD has been widely applied to the discovery of peptides and peptide-like molecules that may serve as severe acute respiratory syndrome coronavirus 2 (SARS-CoV-2) inhibitors. This review summarizes recent advances in such explorations, focusing on four protein targets: angiotensin-converting enzyme 2 (ACE2), spike protein (S protein), main protease (Mpro), and papain-like protease (PLpro). These four proteins are important druggable targets of SARS-CoV-2 because of their roles in viral entry, maturation, and infectivity of the virus. A review of the literature revealed that ACE2, S protein, and Mpro have received more attention in MD research than PLpro. Inhibitors of the four targets identified by MD simulations included peptides derived from food and other bioresources, peptides designed using the targets as templates, and peptide-like molecules retrieved from databases. Many of the inhibitors have yet to be validated in experimental assays for potency. Nevertheless, the role of MD simulation as an efficient tool in the early stages of anti-SARS-CoV-2 drug discovery agents has been demonstrated.Graphic Abstract
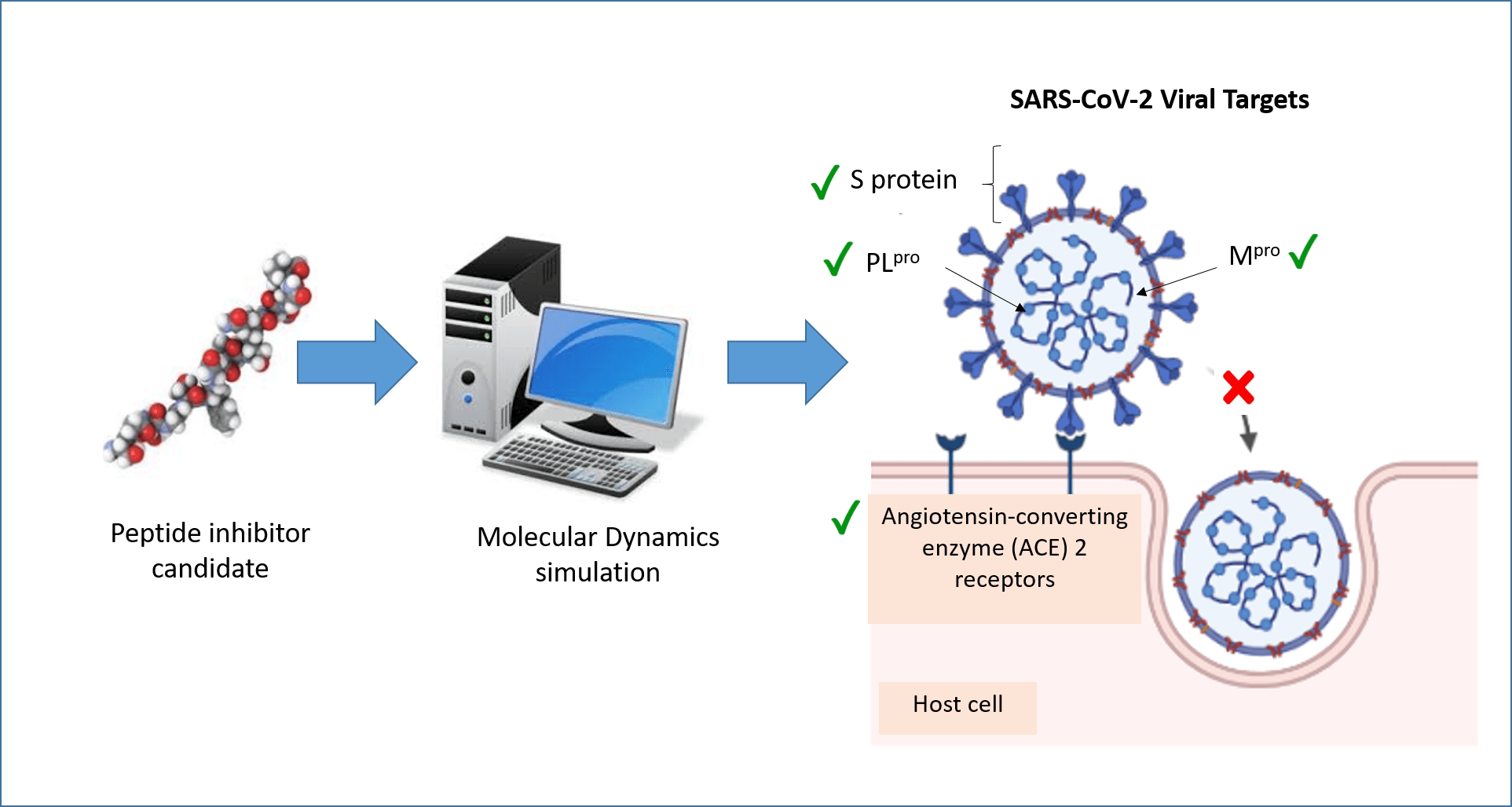
Keywords
Molecular dynamics (MD) simulation has had a dramatic impact on biopeptide research, especially in recent years. By definition, MD is a computer simulation method that calculates the motion of a system of particles, and their equilibrium are calculated in a predetermined period (Kondori et al., 2017). In the field of computational biology and chemistry, MD simulation focuses on the behavior of proteins and biomolecules at the atomic scale and very fine resolution (Hollingsworth and Dror, 2018). Here, MD simulation predicts the motion of atoms in proteins and biomolecules based on a general model of physics that governs interatomic interactions, which computational chemists refer to as a “force field” (Kleinschmidt et al., 2022).
The theory behind molecular mechanics (MM) MD is less complicated and slightly different from the quantum mechanics (QM) principles of MD. In a biomolecular system such as a protein molecule surrounded by water, if all the atomic positions in the system are known, the force exerted on each atom by all the other atoms can be calculated (Wang et al., 2006). This is done by treating each atom as a sphere with attached springs connecting neighboring atoms (Adcock and McCammon, 2006). Newton’s laws of motion, combined with the chemical potential of each atom, are used to predict the spatial position of each atom as a function of time (Tuckerman and Martyna, 2000). At each time step, the force that updates the position and velocity of each atom is calculated, and the process is repeated until the end of a fixed time frame, typically in the range of nanoseconds to milliseconds (Luehr et al., 2015). By combining MD with other experimental structural biology techniques (such as X-ray crystallography, nuclear magnetic resonance (NMR), and cryoelectron microscopy (cryo-EM)), activities of biomolecules, such as mutation, phosphorylation, protonation, and the addition or removal of a ligand have been discovered (Hollingsworth and Dror, 2018).
While many factors drive the advancement of MD in biopeptide research, one of them is higher accessibility and robustness of MD in recent years. Over the past 20 years, the advancement and impact of MD research have been driven by the use of highly sophisticated supercomputers. However, the introduction of powerful graphics processing units in personal computers for the convenience of researchers has allowed powerful MD to be run individually/locally at a relatively low cost (Friedrichs et al., 2009). For example, the minimum central processing unit set-up required for a hundred-nanoseconds MD simulation can be as simple as an Intel i5 processor with 16 GB of RAM and an RTX 2060 graphics card, depending on the total number of atoms in the system.
For MD studies, the common analyses performed include the bond distance, bond angle, dihedral angle, solvent-accessible surface area, and Coulombic and van der Waals interactions are among (Chowdhury et al., 2020). In addition, the root-mean-square-deviation (RMSD) and root-mean-square-fluctuation (RMSF) values for backbone, alpha carbon, and heavy atoms are also analyzed for either the protein target, ligand, and/or the protein-ligand complex (Tachoua et al., 2020). For the binding free energy, the calculations can be performed using MD simulation programs such as Groningen MAchine for Chemical Simulation (GROMACS) with external plugin g_mmpbsa (Kumari et al., 2014) or servers such as PRODIGY server, which measures the free energy based on intermolecular contacts and properties derived from the noninterface surface (Xue et al., 2016). The commonly used approaches for free energy calculation from MD results are the molecular mechanics/Poisson–Boltzmann surface area (MM/PBSA) and molecular mechanics/generalized Born surface area (MM/GBSA) methods (Hou et al., 2011).
In this review, we have focused on the application of MD to elucidate the interactions between peptides and selected host/viral protein targets that are key to host entry and viral proteolytic processing of severe acute respiratory syndrome coronavirus 2 (SARS-CoV-2), the causative agent of coronavirus disease of 2019 (COVID-19). These two phases are essential for viral virulence. The host target discussed here is the angiotensin-converting enzyme 2 (ACE2), whereas the viral targets are the receptor binding domain (RBD) on the spike protein (S protein), the main protease (Mpro), and the papain-like protease (PLpro). Specifically, we discuss here the technical aspects of force field, water model, box selection, and duration in recent studies to simulate the target protein-peptide-ligand systems. Examples of peptides discussed here were derived from food and other bioresources, as well as rationally designed peptides. This review aims to provide useful general and technical insights for researchers when using the MD technique to investigate biopeptide-target protein interactions in the future.
In chemistry, the force field can be described as the articulation or mathematical expression of the potential energy functions present in molecules or atoms with internal coordinates (Palmö et al., 1991; Monticelli and Tieleman, 2013; Dauber-Osguthorpe and Hagler, 2019). Each molecule has a three-dimensional structure consisting of chains of atoms that can be treated as a space-filling sphere inter-connected by strings or sticks, giving rise to the term “ball and stick” conformation. These atoms and chains can be brought together by using energy-based methods such as molecular mechanics and dynamics to assign potentials or forces to the space-filling atoms of the molecule. This is possible by adding the analytical expression of the energy surface as a function of the molecular coordinates, simply called the force field.
While the QM force field is believed to provide a high degree of accuracy in calculating the potential energy of a molecule (Söderhjelm and Ryde, 2009; Sami et al., 2021), the classical MM force field is commonly used for the analysis of biomolecular structures. The MM techniques define the potential energy functions of each atom with motion recorded in the Cartesian coordinates in much simpler terms compared to that in QM (Mackerell, 2004). In practice, there is a trade-off between lower computational requirements and longer simulation times. This consideration is particularly important for macromolecular structures such as proteins and nucleic acids, for which potential energy calculations can take a relatively long time. For the MD simulation of proteins and other biomolecules, Optimized Potentials for Liquid Simulations (OPLS), Chemistry at Harvard Macromolecular Mechanics (CHARMM), and Assisted Model Building with Energy Refinement (AMBER) force fields are commonly used for potential energy calculations (Wang and O’Mara, 2021).
The functional form of OPLS is shown in Eq. (1) below:
V(rN)=Ebonds+Eangles+Edihedrals+EnonbondedV(rN)=Ebonds+Eangles+Edihedrals+Enonbonded (1)
where,
Ebonds=∑bondsKr(r−r0)2Ebonds=∑bondsKr(r−r0)2
Eangles= ∑anglesKθ(θ− θ)2Eangles=∑anglesKθ(θ−θ)2
Edihedrals = ∑dihedrals(V12[1+cos(∅−∅1)] + V22 [1+cos(2∅−∅2)] + V32 [1+cos(3∅−∅3)] + V42 [1+cos(4∅−∅4)]
Enonbonded=∑i>jfij(Aijr12ij−Cijr6ij+qiqje24πε0rij)
The use of the OPLS3 force field has improved quantum chemical data for small molecules (Harder et al., 2016), producing a lower average root mean square (RMS) for X-ray and NMR structures when compared with the older OPLS versions of OPLS_2005 and OPLS2.1 (Mu et al., 2003; Kumar et al., 2016). Here, the dihedral term represents the angle between two planes passing through the same bond (Biswas and Mallik, 2020). In OPLS3, all four angles available in the planar are considered, compared to the simpler terms used in the CHARMM and AMBER force fields (Riniker, 2018; Best, 2019).
The CHARMM force field is another popular force field used for biomolecular simulations. The development series started with a united-atom force field CHARMM19, before progressing to the all-atom type force field CHARMM22, then to CHARMM27, and the latest CHARMM36 (Lemkul, 2020). The potential energy function for the all-atom CHARMM force field is shown below in Eq. (2):
V(rN)=Ebonds+Eangles+Edihedrals+ Eimpropers+EUrey−Bradley+Enonbonded (2)
where,
Ebonds=∑bondskb(b−b0)2Eangles=∑angleskθ(θ−θ0)2Edihedrals=∑dihedralsk∅[1+cos(n∅−δ)]Eimpropers=∑improperskω(ω−ω0)2EUrey−Bradley=∑Urey−Bradleyku(u−u0)2Enonbonded=∑nonbonded(εij[(Rminijrij)12−2(Rminijrij)6]+qiqjεr rij)
Generally, the calculation of the force field in atoms involves two main empirical functions, namely the bonded and non-bonded potentials. The dynamics of bond stretching and angle bending between atoms are described by the Ebonds and Eangles of the atoms. Simple harmonic motions are assumed, where the magnitude of a restoring force is proportional to the displacement of an atom from the equilibrium position (Campanella et al., 2021). While the bonded/non-bonded potentials between all force fields are fixed, the bonded potential energy terms for dihedral angles differ between OPLS and CHARMM force fields. In the case of amino acids/peptide bonds, where each peptide bond connects the planar amino acid residues, the psi and phi angles contribute significantly to the difference in degree angle. The OPLS uses a different equation to calculate the dihedral potential, while the AMBER and CHARMM force fields use the same dihedral potential equation. The dihedral angle describes the angular spring between the planes formed by the first three and last three atoms of consecutively bonded quadruple atoms, as shown in Fig. 1.
Figure 1: The dihedral angle is the angle (φ) between two adjacent bonding planes (1–4). The figure adopted from Herranz et al. (2022).
The functional equation for the AMBER force field is shown below in Eq. (3):
V(rN)=Ebonds+Eangles+Edihedrals+ Enonbonded+Enonbonded (3)
where,
Ebonds=∑bondsk2(b−b0)2Eangles=∑angleskθ(θ−θ0)2Edihedrals=∑dihedralsVn2[1+cos(n∅−γ)]Enonbonded=∑nonbondedAijRij12−BijRij6Enonbonded=∑nonbondedqiqjϵRij
Here, the differences between the numerical solution between atoms calculated using the OPLS, CHARMM, and AMBER equations are in the dihedral potential, the Urey-Bradley potential, and the non-bonded potential of the atoms (Sonibare et al., 2020).
Roles of Angiotensin-Converting Enzyme 2, S Protein, Mpro, and PLpro in the Life Cycle of SARS-CoV-2
As a detailed discussion of the life cycle of SARS-CoV-2 is beyond the scope of this review, we refer the reader to a recent, comprehensive review focusing on this topic by Brant et al. (2021). As shown in Fig. 2, ACE2 is the primary host receptor for SARS-CoV-2. Complexation of ACE2 with S protein can initiate viral entry into the human cell (Struck et al., 2012; Jia et al., 2021). The S protein contains S1 and S2 domains. The S1 domain contains the RBD domain that interacts with ACE2 (Guo et al., 2009; Koley et al., 2022). The S2 domain of the S protein is responsible for the fusion of the virus with the host cells (Song et al., 2018; Huang et al., 2020b). The ACE2-S protein interaction is considered a therapeutic target for COVID-19 (Cheng et al., 2020). Two S proteins can bind to an ACE2 dimer to accelerate viral penetration (Wu et al., 2021), as shown in Fig. 3 (Guo et al., 2021). Structural analyses using x-ray crystallography and biophysical attributes of protein-protein interactions reveal a huge interaction surface between SARS-CoV-2 and ACE2 (18 interactions) with high binding affinity (Corrêa Giron et al., 2020; Shirbhate et al., 2021). This can be seen in Fig. 4, where ACE2 amino acids in the domain S19-Q101 (blue domain) are shown to interact with the RBD of the S protein.
Figure 2: Schematic diagram of the life cycle of SARS-CoV-2, adapted from Wang et al. (2021).
Figure 3: S1 domains of two S proteins binding to the peptidase domains of an ACE2 dimer. Image was adapted from the RCSB PDB (rcsb.org) of PDB ID 7DWX (Yan et al., 2021) and recreated with the PyMOL software (Schrödinger and DeLano, 2020).
Figure 4: Cryo-EM structure (resolution 2.80 Å) of the RBD (red domain) of severe acute respiratory syndrome coronavirus 2 S protein binding to the ACE2 receptor protein (blue domain). Image was adapted from the RCSB PDB (rcsb.org) of PDB ID 7DQA (Liu et al., 2021) and recreated with the PyMOL software (Schrödinger and DeLano, 2020).
The pathology of the SARS-CoV-2 virus is similar to that of its ancestor, SARS-CoV (Hofmann and Pöhlmann, 2004). The SARS-CoV-2 genome shares up to 82% sequence identity with SARS-CoV and MERS-CoV and over 90% sequence identity for essential enzymes and structural proteins (Naqvi et al., 2020). Upon entry into the host cell, the SARS-CoV-2 RNA genome is translated into two large polyproteins, pp1a and pp1ab (Fig. 2). The two polyproteins are cleaved by two viral proteases into 16 mature non-structural proteins (nsp1-16) in preparation for subsequent viral genome replication and transcription. Two cysteine proteases, namely Mpro (also known as chymotrypsin-like protease, 3CLpro) and PLpro, are key to processing of two viral polyproteins. The proteolytic step is critical because inhibition of the viral proteases can interfere with the assembly of new viral particles (Mariano et al., 2020). It is, therefore, not surprising that the two proteases are attractive drug targets for SARS-CoV-2 (Brant et al., 2021; Citarella et al., 2021; Mengist et al., 2021).
Mpro is a homodimeric protease comprising two protomers (A and B) that, upon dimerization and activation, adopt the appropriate conformation to perform their catalytic function (Zhang et al., 2010; Citarella et al., 2021). The crystal structure (PDB ID: 6LU7) (Fig. 5) was determined at a high resolution of 2.1 Å, where each protomer consists of three domains. Domain I (residues 8–101) and domain II (residues 102–184) form an antiparallel β-barrel structure. Domain III (residues 201–303) contains five α-helices arranged in a largely antiparallel globular cluster. Domain III is connected to domain II by a long loop region (residues 185–200) (Jin et al., 2020). The inhibitor target site is located between domain I and domain II and consists of residues 164–168 and 89–191 (Jin et al., 2020). His41 and Cys145 form the catalytic dyad that is located at the cleft between domain I and domain II of Mpro (Tripathi et al., 2021).
Figure 5: Protomer A of the Mpro homodimer with its domains I, II, and III. The inhibitor target site is shown. Image was adapted from the RCSB PDB (rcsb.org) of PDB ID 6LU7 (Jin et al., 2020) and recreated with the PyMOL software (Schrödinger and DeLano, 2020).
PLpro cleaves the viral polyproteins, removes ubiquitin-like ISG15 protein modifications and, and with lower activity, the Lys48-linked polyubiquitin (Klemm et al., 2020). This was also observed in the earlier predecessors of the SARS coronavirus (Lindner et al., 2007; Bailey-Elkin et al., 2017). The castalytic triad in the active site of PLpro consists of Cys111, His272 and Asp286, (Fig. 6). Besides processing the viral polypeptide into functional proteins, PLpro can also dampen the host antiviral response by hijacking ubiquitin, a key enzyme in the host defense mechanism (Amin et al., 2021). PLpro also has a zinc-finger region that contributes to the activation of the protease by holding ubiquitin in place (Luo, 2016; Klemm et al., 2020).
Figure 6: PLpro apo-structure with its catalytic triad marked in magenta and the zinc-finger domain with Zn2+ ion (grey sphere). Image adapted from the RCSB PDB (rcsb.org) of PDB ID 6WUU (Rut et al., 2020) and recreated with the PyMOL software (Schrödinger and DeLano, 2020).
MD Simulation of Peptides Targeting Angiotensin-Converting Enzyme 2, S Protein, Mpro, and PLpro
In the quest to discover peptide candidates capable of antagonizing protein targets related to COVID-19, many computational studies have been reported in the literature. However, many of them stopped at the level of molecular docking; not all of them proceeded to analyze the candidate peptides by MD simulation. Compared with peptide-PLpro interaction, more MD studies were performed to analyze the interactions between peptides and three targets: ACE2, RBD/S protein, and Mpro. In Table 1, we have summarized crystal structures used in recent studies to dissect the interactions between peptides and the four protein targets of interest for this review.
Table 2 presents MD parameters employed in recent studies of peptides interacting with the four target proteins. GROMACS is the most commonly used free software, whereas OPLS is the most popular force field in the studies. OPLS was used because it is the default force field for protein/peptide simulations in some programs, such as Desmond (Maestro Schrödinger). For water models, transferable intermolecular potential with 3 points (TIP3P) was used in almost half of the studies. The majority of the studies performed MD simulations for only up to 100 ns, as a result of the practical trade-off between reasonable computational time and the current performance of available machines.
Peptide-angiotensin-converting enzyme 2 interactions
Among the studies presented in Table 2, an interesting biopeptide exploration is the screening of pea and amaranth peptide libraries for ACE2 inhibitors (Paredes-Ramos et al., 2022). The MD simulation was conducted using the Desmond package (https://www.schrodinger.com/), where OPLS3 was used as the force field. OPLS3 is the force field that is one version older than the current OPLS4. In the study (Paredes-Ramos et al., 2022), the blind docking approach was adopted; ligand docking was introduced to the entire protein surface without any prior knowledge of the target pocket (Hassan et al., 2017). Blind docking is employed when there are multiple possible binding sites and modes of peptide ligands, thus necessitating the scanning of the entire surface of a protein target (Hetényi and van der Spoel, 2006).
Paredes-Ramos et al. (2022) simulated the biopeptide-ACE2 complex in a cubic box, solvated with TIP3P-TIP4P models of water molecules. As mentioned above, almost half the number of the MD studies documented in this review employed the TIP3P water model. Both TIP3P and TIP4P water models used the rigid point water model, where the H–O–H geometry angle is fixed at 104.52° (Harrach and Drossel, 2014). The TIP3P water structure has three interaction points corresponding to the three atoms of the water molecule. TIP4P adds one dummy atom near the oxygen along the bisector of the H–O–H angle on the three-site model which has only the negative charge to improve the electrostatic distribution around the water molecule (Fig. 7) (Kiss and Baranyai, 2011). The computational cost of water simulation increases proportionally with the number of interaction sites in the water model (Shabane et al., 2019). Therefore, the use of TIP4P results in a longer simulation time when compared to TIP3P; this is because the central processing unit time is approximately proportional to the number of interatomic distances that need to be computed. In the study by Paredes-Ramos et al. (2022), a total simulation time of 20 ns was used.
Figure 7: H–O–H geometry of the TIP3P and TIP4P water models. Image was adapted from Kadaoluwa Pathirannahalage et al. (2021). The θHOH denotes the H–O–H angle (104.52° in the rigid points water model), whereas rOM denotes the distance of one dummy atom (M) to the oxygen atom in the 4-site water model.
The peptides LSDRFS, SDRFSY, and VIKP derived from the pea and amaranth were found to be potential inhibitors of the ACE2-S spike interaction (Paredes-Ramos et al., 2022). The entire structure of LSDRFS (head, center, and tail) was well attached to the ACE2 protein, creating a highly stable interaction, allowing the peptide to block a large region of ACE2 that is known to bind to the S protein (Vankadari, 2020). The peptide SDRFSY was attached to the protein in the center region of the ACE2 protein, with its head and tail amino acids free to rotate (Paredes-Ramos et al., 2022). Small motions are sufficient to stabilize a protein-ligand complex (Chen et al., 2020). The flexibility of docking can optimize the non-covalent interactions between a protein and a ligand, thus contributing to favorable changes in enthalpy. The flexibility can also increase the entropy or minimize the decrease in entropy of a protein-ligand complex; this is accomplished by releasing interfacial water, thus increasing the flexibility in part of the protein or ligand. The ligand/protein flexibility can improve the overall molecular flexibility and contribute to a favorable change in the Gibbs binding free energy (Zavodszky and Kuhn, 2005). The virtual screening analysis, supported by evidence from an in-vitro assay identified the smallest VIKP peptide as the weakest candidate for binding to ACE2 (Paredes-Ramos et al., 2022).
In another study, the potential of GWLEPLL, a peptide derived from milk and colostrum, as an ACE2 inhibitor was investigated using the OPLS force field (Pradeep et al., 2021). The GWLEPLL-ACE2 complex was solvated using a 3-site rigid water model of Simple-Point Charge (SPC) within a cube measuring 1 Å on each side. SPC is a rigid water model comparable to TIP3P and, therefore, requires less computational time/cost in calculations when compared to the TIP4P water model (Kadaoluwa Pathirannahalage et al., 2021). Being a smaller cubical model (1 Å from the protein-ligand complex to the simulation box-edge), fewer water molecules were simulated for the complex, and the simulation time could be reasonably extended to 50 ns (Pradeep et al., 2021). In the study, GWLEPLL interacted stably with the ACE2 S2 subsite, forming nine hydrogen bonds (H-bonds). The interactions stabilized the complex by reducing the root-mean-square (RMS) backbone of ACE2 and also its radius-of-gyration (Rg) (Pradeep et al., 2021).
The binding of the peptide EEAGGATAAQIEM to ACE2 was investigated (Yu et al., 2021). The peptide was derived from the tuna skeletal myosin heavy chain (NCBI accession BAA12730.1) after in silico gastrointestinal digestion. The in silico digestion simulation was accomplished by virtually proteolyzing the protein with pepsin, trypsin, and chymotrypsin using ExPASy Peptide Cutter (https://web.expasy.org/peptidecutter/) to predict the cleavage sites of the proteases. MD simulation analysis of the EEAGGATAAQIEM-ACE2 complex was performed in GROMACS 2018 with the CHARMM36 force field; the system was solvated in the TIP3P water model in a cubic box. The duration of the MD simulation was significantly longer (up to 100 ns) when compared with some studies in Table 2. The CHARMM36 force field, when applied in MD simulations, was able to reproduce various NMR observables and correlated well with experimental data; therefore, it is recommended for protein simulations (Huang and MacKerell, 2013). Compared to some of the biopeptides in the studies presented in Table 2, EEAGGATAAQIEM is a relatively long peptide of 14 residues. Thus, when compared with other peptides, more interaction points were found between EEAGGATAAQIEM and ACE2, including a higher number of intermolecular H-bonds. As long peptides can optimize points of interaction with target proteins, slightly longer peptides with limited folding are desirable for binding to protein targets, in this case against ACE2 (Araghi and Keating, 2016).
An integrated in vitro-in silico approach was used by Rangaswamy et al. (2021) to discover ACE2 inhibitory peptides from buckwheat and quinoa. The in silico analysis used Schrodinger’s SiteMap tool, which allowed the identification of five potential binding sites in ACE2. The authors subsequently docked 35 candidate peptides to ACE2 at the site with the highest Sitescore. The 35 peptides were generated by in vitro gastrointestinal digestion, followed by peptide identification using tandem mass spectrometric analysis. Docking analysis was performed and the NWRTVKYG-ACE2 complex was eventually chosen for 50 ns MD simulation. The OPLS force field and the SPC water model were selected in the MD simulation system. In the study, H-bonds and polar interactions observed in the docking analysis supported the selection of NWRTVKYG for MD simulation analysis. The ACE2 residues Gln102, Asp206, and Glu208, the key residues involved in H-bond interactions, and other amino acids forming polar interactions and responsible for peptide recognition Gln98, Asn194, Gly205 and Lys562, were shown to maintain their interactions with the peptide for the entire 50 ns duration (Rangaswamy et al., 2021).
Azurin is a 14 kDa protein secreted by the bacterium Pseudomonas aeruginosa. Peptides derived from azurin, such as p18 and p28, showed no signs of toxicity in the phase I clinical trials (Habault and Poyet, 2019), making them promising antagonists of ACE2. Sasidharan et al. (2021) docked azurin, p18, and p28 to ACE2, and subjected each complex to MD simulation for 60 ns using the OPLS force field and the TIP3P water model. Molecular docking revealed that azurin can bind to the collectrin domain of ACE2, whereas p18 and p28 can bind to the active peptidase domain of ACE2. However, MD simulation followed by MM/PBSA analysis showed that p28 had a higher affinity for S protein but bound only moderately to ACE2 (Sasidharan et al., 2021).
Peptide-S protein interactions
DYGAVNEVK is a peptide derived from fruit bromelain. In light of the high mutation rates of the RBD domain of SARS-CoV-2, the binding of DYGAVNEVK to wild-type and mutated RBD domains was compared (Tallei et al., 2022). Docking analysis showed that the peptide bound tightly to the wild-type RBD and the RBD of each mutant tested, especially when the mutation involved negatively charged, polar, and hydrophilic amino acids. In this case, the stability of the RBD was increased. Next, an all-atom MD simulation was performed using the AMBER99SB-ILDN force field (Tallei et al., 2022). This force field is known for its improved amino acid side-chain torsion potentials, making it more suitable for protein and peptide MD simulations than the previous versions of the AMBER force field (Lindorff-Larsen et al., 2010). The stability of each DYGAVNEVK-RBD complex was substantiated with RMSD and RMSF plots, coupled with MM/GBSA analysis (Tallei et al., 2022). The MM/GBSA analysis calculates the binding free energy of a protein-ligand complex and has been shown to effectively balance computational cost with accuracy (Huang et al., 2020a). To minimize computational cost, the study also used the triclinic cell and SPC water model during the MD simulation (Tallei et al., 2022). The use of the triclinic cell minimizes the use of water molecules as a triclinic cell has 29% less volume compared to a rectangular periodic box (Lindahl et al., 2001). Meanwhile, SPC is a simpler water model compared to the TIP3P/TIP4P water models (Paschek, 2004). The use of the latter two parameters can increase the speed of MD simulation calculations.
In another study, the RBD domains of the wild-type and beta variant (lineage B.1.351) were docked to bacteriocins derived from lactic acid bacteria, followed by MD simulation (Erol et al., 2021). Bacteriocins are antimicrobial peptides synthesized by ribosomes in bacteria. The peptides can kill or inhibit strains closely related or unrelated to the bacteriocin producers without harming these producers (Yang et al., 2014). Bacteriocins are widely used in the food industry as preservatives (Settanni and Corsetti, 2008; Johnson et al., 2018) and antimicrobial food packaging (Daba and Elkhateeb, 2020) as well as in the pharmaceutical industry as antibiotics (Balciunas et al., 2013; Chikindas et al., 2018). In the study by Erol et al. (2021), pediocin PA-1, salivaricin P, and salivaricin B were the most promising in inhibiting the entry of SARS-CoV-2 (including lineage B.1.351) into the human cells. The subsequent MD simulation analysis was conducted by using the Desmond package of Schrödinger’s Maestro program, using the OPLS3 force field with the TIP3P water model. The simulation was performed in the orthorhombic cell. MD simulation was conducted with a high computational cost totaling up to 6 µs for all simulation systems, with 500 ns for each bacteriocin-RBD complex; the analysis was also performed in triplicates (Erol et al., 2021). The authors also used a different isothermal-isobaric barostat system of the Martyna-Tuckerman-Tobias-Klein (MTTK) coupling method in the MD simulation (Erol et al., 2021) rather than the commonly used Berendsen and Langevin coupling method (Khalili et al., 2005). Although there is a lack of studies comparing the three isobaric coupling methods in protein and biomolecular MD simulations, the comparison in the metal-organic compound framework showed that the static properties are well reproduced with the three barostats (Rogge et al., 2015).
MD simulations were used to evaluate the potential inhibition of S protein by azurin and its peptides p18 and p28 (Sasidharan et al., 2021). Azurin, a molecule larger than p18 and p28, can bind to the S2 domain of the S protein; p18 and p28 could bind to the N- and C-terminals of the S1 domain, respectively. Stable binding between p28 and the S protein was evidenced by the RMSD plot. Within the S protein binding pocket, p28 adapted well, undergoing slight conformational changes before achieving conformational stability throughout the remainder of the MD simulation (Sasidharan et al., 2021).
Pradeep et al. (2021) investigated bovine milk-derived peptides as RBD binders. The most promising peptide, IQKVAGTW (−11.03 glide score), was predicted to be positioned in the central shallow pit of the RBD domain of the spike-ACE2 complex, stabilized by a high number of H-bonds. The number of H-bonds in the complex was also stable throughout the MD simulation (Pradeep et al., 2021).
An elegant peptide inhibitor study involved the design of RBD binders based on the peptide sequence of the ACE2 peptidase domain (Sitthiyotha and Chunsrivirot, 2020). Residues 21–45 of the peptidase domain, a coiled α1 helix of ACE2, were first truncated. The sequence was then optimized to one 25-mer non-designed peptide and 13 designed peptides. This was followed by MD simulation using AMBER FF14SB and the GLYCAM06j-1 force field. The authors used the GLYCAM06j-1 force field in their MD simulation due to glycoprotein structures in the RBD (Singh et al., 2016). Five designed peptides were proposed as promising SARS-CoV-2 inhibitors based on their RBD-binding affinities, which were superior to that of SBP1, an experimentally-validated RBD peptide binder (Sitthiyotha and Chunsrivirot, 2020).
Two antimicrobial peptide (AMP) databases, namely StraPep (http://isyslab.info/StraPep/) and PhytAMP (http://phytamp.hammamilab.org/main.php), were also tapped as sources of potential RBD inhibitors (Balmeh et al., 2021). Nearly 500 peptides were shortlisted from the two AMP databases based on criteria including allergenicity, toxicity, and hemolyticity before the peptides were docked to the S protein. The peptides with the best docking scores were subjected to a 45 ns MD simulation. Judging from the upwards trend of the RMSD plot obtained (Balmeh et al., 2021), the simulation duration should be increased. In addition, some of the peptides evaluated in the study, such as bacteriocin lactococcin-G (35-mer) and defensin Lc-def (47-mer), are longer (Balmeh et al., 2021) compared to peptides evaluated in other studies using MD durations of longer than 45 ns (Table 2).
To screen for RBD-binding peptides, Chowdhury et al. (2020) selected 51 peptides from the antiviral database AVPdb (http://crdd.osdd.net/servers/avpdb/index.php). Following molecular docking analysis, six RBD-peptide complexes were subjected to MD simulation. Residues Glu484, Tyr449, Gln493, Leu455, Tyr453, Tyr489, and Tyr505 of RBD were found to form non-covalent interactions with the antiviral peptides. H-bonds accounted for 53% of the total interactions. MD analysis also revealed reduced solvent-accessible surface area for all six complexes (Chowdhury et al., 2020).
Micasin, a peptide from the dermatophytic fungus Microsporum canis, has been reported as a promising RBD inhibitor, and the micasin-RBD complex was subjected to MD simulation (Gao and Zhu, 2021). Similar to the findings of Chowdhury et al. (2020), Gln493, Ser494, and Tyr453 of RBD were observed to form the largest number of H-bonds with micasin during the 40 ns duration. Notably, the mutation introduced into micasin expanded its interface region responsible for binding to RBD, resulting in a six-fold increase in binding affinity (Gao and Zhu, 2021).
In contrast to the relatively long peptides evaluated by Balmeh et al. (2021), much shorter S protein binding peptides (7 to 20 residues) were designed by Pei et al. (2021). The study focused on the design of synthetic ultrashort peptides based on the RBD residues known to interact with ACE2, namely K417, Y453, F486, Q498, and N501. The peptides were subjected to MD simulations. Binding energy analysis showed that the peptide SI5α-b had the strongest inhibitory activity, followed by the peptide SI5α; this is consistent with results of the in vitro antiviral assay and the peptide-RBD and ACE2 competitive ELISA assay (Balmeh et al., 2021).
Pant et al. (2020) reported a notable investigation to screen for Mpro-inhibitory peptides. They screened peptide-like inhibitors and other potential candidates in the CHEMBL and ZINC databases, drugs approved by the United States Food and Drug Administration, and molecules in clinical trials. Screening of 300 peptide-like structures led to the identification of four promising Mpro inhibitors (Fig. 8), which were subjected to MD simulation. While most of the MD studies cited in this review (Table 2) used the MM/PBSA method for binding energy calculations, Pant et al. (2020) performed binding energy calculations using the MM/GBSA method. Here, the peptide-like molecules provide a basic pharmacophore for the design of SARS-CoV-2 Mpro inhibitors. The amide linkage backbone gives them the flexibility to fit comfortably into the Mpro binding site. The four Mpro inhibitors highlighted by Pant et al. (2020) form H-bonds with residues Gln192, Glu166, His166, and His41, which comprise the ligand-binding site in Mpro. His41 is also part of the catalytic dyad of Mpro (Tripathi et al., 2021).
Figure 8: The chemical structures of the four best peptide-like Mpro inhibitors (C1–C4) reported by Pant et al. (2020). The two-dimensional structures of the inhibitors were drawn by using the freeware ACD/ChemSketch (version 2022.1.0, Advanced Chemistry Development, Inc. (ACD/Labs), Toronto, ON, Canada, www.acdlabs.com) based on SMILES strings retrieved from the CHEMBL database (Davies et al., 2015; Gaulton et al., 2016).
Milk-derived peptides have also been screened for Mpro inhibitors. Following the molecular docking-based screening of 326 di- and tripeptides generated by in silico digestion of bovine milk proteins, Behzadipour et al. (2021) identified QSW, a potential Mpro inhibitor. Similarly, Zhao et al. (2022) screened peptides generated from bovine lactoferrin by in silico digestion and identified a tetrapeptide GSRY as a potential Mpro inhibitor. Both QSW and GSRY interacted stably with residues Gly143, Glu166, and Gln189 of Mpro, which are known to interact with ligands of Mpro. The two peptides also formed an H-bond with a histidine residue (His41/His163) to inhibit the catalytic dyad of Mpro. While MD simulations were performed at 100 ns in both studies, the QSW-Mpro complex was simulated using the AMBER99SB force field (Behzadipour et al., 2021), whereas the GSRY-Mpro complex was simulated using the CHARMM36 force field (Zhao et al., 2022). The use of AMBER99SB was desirable, as it allows the pH of the QSW-Mpro complex to be set (Behzadipour et al., 2021). Owing to the protonation effect of certain amino acid residues at different pH values, the affinity of a peptide to a protein can also change (Keeble et al., 2019). In the MD study by Zhao et al. (2022), it was not possible to set the pH of the simulation using the CHARMM force field.
The search for Mpro-inhibitory peptides originating from fish proteins was the focus of two recent studies. The tuna-derived peptide EEAGGATAAQIEM was reported to form 11 H-bonds with the residues in domains I–III of Mpro (Yu et al., 2021). After 100 ns MD simulation, EEAGGATAAQIEM also bound tightly to Mpro residues known to interact stably with the N3 inhibitor (Yu et al., 2021), such as His164, His41, Gly143, and Gln189 (Jin et al., 2020). The study also showed a stable Rg value for the EEAGGATAAQIEM-Mpro complex; the value remained almost constant for 75% of the simulation time (Yu et al., 2021). The ribbonfish-derived Mpro-inhibitory peptide PTR was reported by Yathisha et al. (2022). The PTR-Mpro complex was subjected to a 20 ns MD simulation performed in GROMACS, using CHARMM27 with the TIP3P water model. Although the study suggested that PTR as the best Mpro inhibitor based on its interactions with the catalytic dyad of Mpro (Yathisha et al., 2022), the simulation time used in the study was insufficient. For better understanding of the complex, it would be desirable to increase the simulation time to at least 50 ns or even 100 ns.
A ricin-based peptide (LLMVNEATRFQTVSGFV, BRIP peptide) derived from barley was shown to be a promising Mpro inhibitor (Kashyap et al., 2022). The 17-residue peptide was docked and subjected to MD-simulation against five potential binding sites on Mpro. After 1000 ns of MD simulation, the peptide was observed to bind to the main inhibitor target of Mpro, namely the catalytic dyad His41 and Cys145. The peptide also formed more than a hundred non-bonded interactions, including H-bond, with Mpro. The peptide is also bound to the target region known as the S1 subsite (Asn142, Glu166, His164, and Met165) (Kashyap et al., 2022).
Sasidharan et al. (2021) investigated the potential of azurin and its peptides p18 and p28 as Mpro inhibitors. In the study, only p28 was observed to bind to domains II and III of Mpro with high affinity. The RMSD plot of the p28-Mpro complex showed higher deviations than that of the p28-S protein complex, especially after 50 ns of MD. This observation suggests the activity of p28 in conformational changes (Sasidharan et al., 2021).
Moradi and co-workers screened 11 plant-derived peptides from the literature that could potentially inhibit PLpro activity (Moradi et al., 2022). They found that the peptide VcTI from Veronica hederifolia provided effective molecular interactions at both the liable zinc site and the classic active site of PLpro. Eighteen residues of PLpro and 16 residues of VcTI were involved in the interactions, which were stable throughout the 20 ns of MD simulation (Moradi et al., 2022). The MD simulation of the VcTI-PLpro complex was performed using the OPLS force field with an SPC216 water model in the cubic box (Moradi et al., 2022), a relatively generic set-up for GROMACS system simulation (Lee et al., 2016). Using the same force field coupled to TIP3P water models in MD analysis, Sasidharan et al. (2021) demonstrated the affinity of p28, an azurin-derived peptide, to PLpro based on its binding to the finger domain of the protease that houses the catalytic zinc ion. The study revealed that p28 may play a multifunctional role as an inhibitor of protein-protein interaction, given its affinity to PLpro and also to S protein and Mpro (Sasidharan et al., 2021).
Similarities in molecular dynamics simulations of peptide-protein simulations
The MD simulations of peptides and target proteins in the peptide-ACE2, peptide-S protein, peptide-Mpro, and peptide-PLpro interactions discussed above share some similarities, which include:
i) The amino acid sequence of a peptide plays a crucial role in determining its interactions with proteins. Specifically, the unique arrangement of amino acids within the peptide is critical for establishing such interactions. MD simulations offer a valuable tool for investigating how the peptide’s sequence influences its binding affinity and the stability of the resulting peptide-protein complex.
ii) MD simulations have demonstrated that non-covalent interactions, such as hydrogen bonds, van der Waals interactions, and electrostatic interactions, are critical factors in the binding process between peptides and proteins. Understanding the characteristics of these interactions is vital for the advancement of peptide-based therapies.
iii) MD simulations can demonstrate how peptide binding induces conformational changes in proteins. Such changes may impact the function and stability of the protein; comprehending them is crucial for drug development.
iv) Water molecules play a critical role in mediating the interactions between the peptide and the protein. MD simulations can provide insight into the role of water molecules in stabilizing the peptide-protein complex and the formation of hydration shells.
v) The flexibility of the peptide and protein can impact the stability and binding affinity of the complex. MD simulations can provide information about the flexibility of both the peptide and the protein and how this affects the binding between them.
Future Perspectives and Conclusions
A number of potential candidate peptides may be the focus of more detailed investigations in the future. The potential candidates for peptide-ACE2 interactions range from synthetically designed to those derived from natural sources. Studies on peptide inhibition of ACE2 revealed that while the flexible ligands were able to release interfacial water and bound ACE2, the rigid ligand still effectively inhibited the S-binding region of ACE2. However, the length of the inhibitory peptides studied suggests that peptides with an optimal length between 8–14 amino acids bind tightly to ACE2. While the ACE2 inhibition study only targeted a specific S protein binding domain in ACE2, multiple target inhibition binding sites are available for S protein and RBD of the SARS-CoV2 virus. In addition, mutant variants of S protein and RBD were also a focus of the peptide inhibition study. Therefore, due to both factors, many peptide inhibitor candidates elucidated for binding to S protein, and RBD are much larger compared to ACE2 inhibitors. The size of these peptides can reach up to 47 mer, thus requiring long simulation durations (>50 ns) with independently repeated simulations to reach a conclusive result. Some potential synthetically modified peptide candidates for peptide-S protein interactions include those targeting the RBD of the S protein, such as the peptide EK1 and its derivatives. Peptides targeting other regions of the S protein, such as the heptad repeat regions, are also being investigated.
For peptide-Mpro and peptide-PLpro interactions, researchers are developing peptides that can target these proteases and potentially inhibit their activity. For peptide-Mpro interactions, researchers are investigating peptides that can target the active site of Mpro, such as the synthetic peptide GC376 (Fu et al., 2020; Ma et al., 2020). Potential candidates for peptide-PLpro interactions include those that target the catalytic site of PLpro, such as the synthetic naphthalene-based peptide GRL0617. Here, the ubiquitin-specific domain of PLpro shifts to form a π‐π interaction with the peptide, while simultaneously forming a deeper pocket to accommodate the ligand (Fu et al., 2021). Some interactions observed with these synthetic peptides share many similarities with the naturally derived peptides listed in Table 2.
Only a few studies discussed above tested the potency of the anti-SARS-CoV-2 peptides they reported using in vitro assays (e.g., Gao and Zhu, 2021; Pei et al., 2021; Sadremomtaz et al., 2022). In the remaining studies, the results of the MD simulation were considered to be the main evidence supporting the potential activity of the peptides. Despite its efficiency in elucidating the molecular details of peptide-target interactions, MD simulation remains a theoretical approach and has its limitations. Therefore, the important next step for most of the proposed candidates of ACE2-, S protein-, Mpro-, and PLpro-inhibitory peptides would be to enter the in vitro or experimental validation phase. This wet-lab testing step is particularly important for those peptides that have not been subjected to MD simulation for sufficient duration (Balmeh et al., 2021; Yathisha et al., 2022).
Between the two SARS-CoV-2 proteases involved in viral polyprotein processing, PLpro has received less attention in MD research aimed at discovering peptide-based PLpro inhibitors. Most MD studies on PLpro inhibitors in the literature have focused on small molecules, although peptides inhibitors are advantageous in terms of the tendency of macromolecules to occupy the active site of PLpro more extensively, in addition to having favorable potency and selectivity (Petushkova and Zamyatnin, 2020; Rut et al., 2020; Amin et al., 2021). To expedite the search for PLpro-inhibitory peptides, a potentially time-saving strategy would be to search the literature for PLpro-inhibitory peptides which were identified on the basis of molecular docking alone (Wong et al., 2021a, 2021b). Such peptides can then be evaluated by MD analysis and even coupled to in vitro PLpro-binding or -inhibition assays to discover peptide-based PLpro inhibitors.
Some of the studies discussed in this review may contribute to the repurposing of drugs or current antiviral compounds (Chowdhury et al., 2020; Pant et al., 2020). Other reports may facilitate the discovery of novel anti-SARS-CoV-2 agents from food and other bioresources (Yu et al., 2021; Yathisha et al., 2022; Moradi et al., 2022), thereby adding value to such biomaterials. Potential SARS-CoV-2 inhibitory peptides have also been identified from many other novel bioresources, such as edible insects (Wong et al., 2020; Ong et al., 2021). Unfortunately, such peptides have often not been validated by MD analysis. To expand the current pool of bioresources that can be tapped for candidates of anti-SARS-CoV-2 peptides, a follow-up evaluation of the aforementioned group of peptides by MD simulation is desirable.
In summary, the COVID-19 pandemic has led to a surge in research interest in the use of biomolecular simulation methodologies to accelerate the discovery of peptide-based SARS-CoV-2 inhibitors. MD simulation combined with molecular docking has been a key strategy in many of these investigations. Peptide and peptide-like candidates have been identified from various bioresources, bioactive peptide databases, druglike compound databases, and preclinical and approved drugs for their inhibition on ACE2, RBD/S protein, Mpro, and PLpro. Experimental validation is still required to confirm the potency of many of these peptides. Nonetheless, the role of MD simulation as a valuable and efficient tool in the early phases of the discovery of peptide-based anti-SARS-CoV-2 agents has been demonstrated. It is anticipated that, in the near future, MD simulation, coupled with other in silico and experimental methodologies, will continue to advance mechanistic understanding of the discovery, design, and biology of anti-SARS-CoV-2 peptides.
Funding Statement: The authors received no specific funding for this study.
Author Contributions: MZS and TTC wrote the manuscript. FCW and JB proofread and revised the content. All authors reviewed the content and approved the final version of the manuscript.
Ethics Approval: Not applicable.
Conflicts of Interest: The authors declare that they have no conflicts of interest to report regarding the present study.
References
Adcock SA, McCammon JA (2006). Molecular dynamics: Survey of methods for simulating the activity of proteins. Chemical Reviews 106: 1589–1615. https://doi.org/10.1021/cr040426m [Google Scholar] [PubMed] [CrossRef]
Amin SA, Banerjee S, Ghosh K, Gayen S, Jha T (2021). Protease targeted COVID-19 drug discovery and its challenges: Insight into viral main protease (Mpro) and papain-like protease (PLpro) inhibitors. Bioorganic & Medicinal Chemistry 29: 115860. https://doi.org/10.1016/j.bmc.2020.115860 [Google Scholar] [PubMed] [CrossRef]
Araghi RR, Keating AE (2016). Designing helical peptide inhibitors of protein-protein interactions. Current Opinion in Structural Biology 39: 27–38. https://doi.org/10.1016/j.sbi.2016.04.001 [Google Scholar] [PubMed] [CrossRef]
Bailey-Elkin BA, Knaap RCM, Kikkert M, Mark BL (2017). Structure and function of viral deubiquitinating enzymes. Journal of Molecular Biology 429: 3441–3470. https://doi.org/10.1016/j.jmb.2017.06.010 [Google Scholar] [PubMed] [CrossRef]
Balciunas EM, Castillo Martinez FA, Todorov SD, Franco BDGDM, Converti A, Oliveira RPDS (2013). Novel biotechnological applications of bacteriocins: A review. Food Control 32: 134–142. https://doi.org/10.1016/j.foodcont.2012.11.025 [Google Scholar] [CrossRef]
Balmeh N, Mahmoudi S, Fard NA (2021). Manipulated bio antimicrobial peptides from probiotic bacteria as proposed drugs for COVID-19 disease. Informatics in Medicine Unlocked 23. https://doi.org/10.1016/j.imu.2021.100515 [Google Scholar] [PubMed] [CrossRef]
Behzadipour Y, Gholampour M, Pirhadi S, Seradj H, Khoshneviszadeh M, Hemmati S (2021). Viral 3CLpro as a target for antiviral intervention using milk-derived bioactive peptides. International Journal of Peptide Research and Therapeutics 27: 2703–2716. https://doi.org/10.1007/s10989-021-10284-y [Google Scholar] [PubMed] [CrossRef]
Best RB (2019). Atomistic force fields for proteins. Methods in Molecular Biology 2022: 3–19. https://doi.org/10.1007/978-1-4939-9608-7 [Google Scholar] [CrossRef]
Biswas A, Mallik BS (2020). Conformational dynamics of aqueous hydrogen peroxide from first principles molecular dynamics simulations. Physical Chemistry Chemical Physics 22: 28286–28296. https://doi.org/10.1039/D0CP05451H [Google Scholar] [PubMed] [CrossRef]
Brant AC, Tian W, Majerciak V, Yang W, Zheng ZM (2021). SARS-CoV-2: From its discovery to genome structure, transcription, and replication. Cell & Bioscience 11: 136. https://doi.org/10.1186/s13578-021-00643-z [Google Scholar] [PubMed] [CrossRef]
Campanella B, Palleschi V, Legnaioli S (2021). Introduction to vibrational spectroscopies. ChemTexts 7: 5. https://doi.org/10.1007/s40828-020-00129-4 [Google Scholar] [CrossRef]
Chen M, Chen X, Schafer NP, Clementi C, Komives EA, Ferreiro DU, Wolynes PG (2020). Surveying biomolecular frustration at atomic resolution. Nature Communications 11: 5944. https://doi.org/10.1038/s41467-020-19560-9 [Google Scholar] [PubMed] [CrossRef]
Cheng H, Wang Y, Wang GQ (2020). Organ-protective effect of angiotensin-converting enzyme 2 and its effect on the prognosis of COVID-19. Journal of Medical Virology 92: 726–730. https://doi.org/10.1002/jmv.25785 [Google Scholar] [PubMed] [CrossRef]
Chikindas ML, Weeks R, Drider D, Chistyakov VA, Dicks LMT (2018). Functions and emerging applications of bacteriocins. Current Opinion in Biotechnology 49: 23–28. https://doi.org/10.1016/j.copbio.2017.07.011 [Google Scholar] [PubMed] [CrossRef]
Chowdhury SM, Talukder SA, Khan AM, Afrin N, Ali MA et al. (2020). Antiviral peptides as promising therapeutics against SARS-CoV-2. The Journal of Physical Chemistry B 124: 9785–9792. https://doi.org/10.1021/acs.jpcb.0c05621 [Google Scholar] [PubMed] [CrossRef]
Citarella A, Scala A, Piperno A, Micale N (2021). SARS-CoV-2 Mpro: A potential target for peptidomimetics and small-molecule inhibitors. Biomolecules 11: 607. https://doi.org/10.3390/biom11040607 [Google Scholar] [PubMed] [CrossRef]
Corrêa Giron C, Laaksonen A, Barroso da Silva FL (2020). On the interactions of the receptor-binding domain of SARS-CoV-1 and SARS-CoV-2 spike proteins with monoclonal antibodies and the receptor ACE2. Virus Research 285: 198021. https://doi.org/10.1016/j.virusres.2020.198021 [Google Scholar] [PubMed] [CrossRef]
Daba GM, Elkhateeb WA (2020). Bacteriocins of lactic acid bacteria as biotechnological tools in food and pharmaceuticals: Current applications and future prospects. Biocatalysis and Agricultural Biotechnology 28: 101750. https://doi.org/10.1016/j.bcab.2020.101750 [Google Scholar] [CrossRef]
Dauber-Osguthorpe P, Hagler AT (2019). Biomolecular force fields: Where have we been, where are we now, where do we need to go and how do we get there? The Journal of Computer-Aided Molecular Design 33: 133–203. https://doi.org/10.1007/s10822-018-0111-4 [Google Scholar] [PubMed] [CrossRef]
Davies M, Nowotka M, Papadatos G, Dedman N, Gaulton A, Atkinson F, Bellis L, Overington JP (2015). ChEMBL web services: Streamlining access to drug discovery data and utilities. Nucleic Acids Research 43: W612–W620. https://doi.org/10.1093/nar/gkv352 [Google Scholar] [PubMed] [CrossRef]
Erol I, Kotil SE, Fidan O, Yetiman AE, Durdagi S, Ortakci F (2021). In silico analysis of bacteriocins from lactic acid bacteria against SARS-CoV-2. Probiotics and Antimicrobial Proteins 15: 17–29 https://doi.org/10.1007/s12602-021-09879-0 [Google Scholar] [PubMed] [CrossRef]
Friedrichs MS, Eastman P, Vaidyanathan V, Houston M, Legrand S, Beberg AL, Ensign DL, Bruns CM, Pande VS (2009). Accelerating molecular dynamic simulation on graphics processing units. Journal of Computational Chemistry 30: 864–872. https://doi.org/10.1002/jcc.21209 [Google Scholar] [PubMed] [CrossRef]
Fu Z, Huang B, Tang J, Liu S, Liu M et al. (2021). The complex structure of GRL0617 and SARS-CoV-2 PLpro reveals a hot spot for antiviral drug discovery. Nature Communications 12: 488. https://doi.org/10.1038/s41467-020-20718-8 [Google Scholar] [PubMed] [CrossRef]
Fu L, Ye F, Feng Y, Yu F, Wang Q et al. (2020). Both boceprevir and GC376 efficaciously inhibit SARS-CoV-2 by targeting its main protease. Nature Communications 11: 4417. https://doi.org/10.1038/s41467-020-18233-x [Google Scholar] [PubMed] [CrossRef]
Gao B, Zhu S (2021). A fungal defensin targets the SARS−CoV−2 spike receptor−binding domain. Journal of Fungi 7: 553. https://doi.org/10.3390/jof7070553 [Google Scholar] [PubMed] [CrossRef]
Gaulton A, Hersey A, Nowotka M, Bento AP, Chambers J et al. (2016). The ChEMBL database in 2017. Nucleic Acids Research 45: D945–D954. https://doi.org/10.1093/nar/gkw1074 [Google Scholar] [PubMed] [CrossRef]
Guo L, Bi W, Wang X, Xu W, Yan R et al. (2021). Engineered trimeric ACE2 binds viral spike protein and locks it in “Three-up” conformation to potently inhibit SARS-CoV-2 infection. Cell Research 31: 98–100. https://doi.org/10.1038/s41422-020-00438-w [Google Scholar] [PubMed] [CrossRef]
Guo Y, Tisoncik J, McReynolds S, Farzan M, Prabhakar BS, Gallagher T, Rong L, Caffrey M (2009). Identification of a new region of SARS-CoV S protein critical for viral entry. Journal of Molecular Biology 394: 600–605. https://doi.org/10.1016/j.jmb.2009.10.032 [Google Scholar] [PubMed] [CrossRef]
Habault J, Poyet JL (2019). Recent advances in cell penetrating peptide-based anticancer therapies. Molecules 24: 927. https://doi.org/10.3390/molecules24050927 [Google Scholar] [PubMed] [CrossRef]
Han Y, Král P (2020). Computational design of ACE2-based peptide inhibitors of SARS-CoV-2. ACS Nano 14: 5143–5147. https://doi.org/10.1021/acsnano.0c02857 [Google Scholar] [PubMed] [CrossRef]
Harder E, Damm W, Maple J, Wu C, Reboul M et al. (2016). OPLS3: A force field providing broad coverage of drug-like small molecules and proteins. Journal of Chemical Theory and Computation 12: 281–296. https://doi.org/10.1021/acs.jctc.5b00864 [Google Scholar] [PubMed] [CrossRef]
Harrach MF, Drossel B (2014). Structure and dynamics of TIP3P, TIP4P, and TIP5P water near smooth and atomistic walls of different hydroaffinity. The Journal of Chemical Physics 140: 174501. https://doi.org/10.1063/1.4872239 [Google Scholar] [PubMed] [CrossRef]
Hassan NM, Alhossary AA, Mu Y, Kwoh C-K (2017). Protein-ligand blind docking using QuickVina-W with inter-process spatio-temporal integration. Scientific Reports 7: 15451. https://doi.org/10.1038/s41598-017-15571-7 [Google Scholar] [PubMed] [CrossRef]
Herranz M, Foteinopoulou K, Karayiannis NC, Laso M (2022). Polymorphism and perfection in crystallization of hard sphere polymers. Polymers 14: 4435. https://doi.org/10.3390/polym14204435 [Google Scholar] [PubMed] [CrossRef]
Hetényi C, van der Spoel D (2006). Blind docking of drug-sized compounds to proteins with up to a thousand residues. FEBS Letters 580: 1447–1450. https://doi.org/10.1016/j.febslet.2006.01.074 [Google Scholar] [PubMed] [CrossRef]
Hofmann H, Pöhlmann S (2004). Cellular entry of the SARS coronavirus. Trends in Microbiology 12: 466–472. https://doi.org/10.1016/j.tim.2004.08.008 [Google Scholar] [PubMed] [CrossRef]
Hollingsworth SA, Dror RO (2018). Molecular dynamics simulation for all. Neuron 99: 1129–1143. https://doi.org/10.1016/j.neuron.2018.08.011 [Google Scholar] [PubMed] [CrossRef]
Hou T, Wang J, Li Y, Wang W (2011). Assessing the performance of the MM/PBSA and MM/GBSA methods. 1. The accuracy of binding free energy calculations based on molecular dynamics simulations. Journal of Chemical Information and Modeling 51: 69–82. https://doi.org/10.1021/ci100275a [Google Scholar] [PubMed] [CrossRef]
Huang K, Luo S, Cong Y, Zhong S, Zhang JZH, Duan L (2020a). An accurate free energy estimator: Based on MM/PBSA combined with interaction entropy for protein-ligand binding affinity. Nanoscale 12: 10737–10750. https://doi.org/10.1039/C9NR10638C [Google Scholar] [PubMed] [CrossRef]
Huang J, MacKerellJr AD (2013). CHARMM36 all-atom additive protein force field: Validation based on comparison to NMR data. Journal of Computational Chemistry 34: 2135–2145. https://doi.org/10.1002/jcc.23354 [Google Scholar] [PubMed] [CrossRef]
Huang Y, Yang C, Xu XF, Xu W, Liu SW (2020b). Structural and functional properties of SARS-CoV-2 spike protein: Potential antivirus drug development for COVID-19. Acta Pharmacologica Sinica 41: 1141–1149. https://doi.org/10.1038/s41401-020-0485-4 [Google Scholar] [PubMed] [CrossRef]
Jia H, Neptune E, Cui H (2021). Targeting ACE2 for COVID-19 therapy: Opportunities and challenges. American Journal of Respiratory Cell and Molecular Biology 64: 416–425. https://doi.org/10.1165/rcmb.2020-0322PS [Google Scholar] [PubMed] [CrossRef]
Jin Z, Du X, Xu Y, Deng Y, Liu M et al. (2020). Structure of Mpro from SARS-CoV-2 and discovery of its inhibitors. Nature 582: 289–293. https://doi.org/10.1038/s41586-020-2223-y [Google Scholar] [PubMed] [CrossRef]
Johnson EM, Jung DY, Jin DY, Jayabalan DR, Yang DSH, Suh JW (2018). Bacteriocins as food preservatives: Challenges and emerging horizons. Critical Reviews in Food Science and Nutrition 58: 2743–2767. https://doi.org/10.1080/10408398.2017.1340870 [Google Scholar] [PubMed] [CrossRef]
Kadaoluwa Pathirannahalage SP, Meftahi N, Elbourne A, Weiss ACG, McConville CF et al. (2021). Systematic comparison of the structural and dynamic properties of commonly used water models for molecular dynamics simulations. Journal of Chemical Information and Modeling 61: 4521–4536. https://doi.org/10.1021/acs.jcim.1c00794 [Google Scholar] [PubMed] [CrossRef]
Kashyap P, Bhardwaj VK, Chauhan M, Chauhan V, Kumar A, Purohit R, Kumar A, Kumar S (2022). A ricin-based peptide BRIP from Hordeum vulgare inhibits Mpro of SARS-CoV-2. Scientific Reports 12: 12802. https://doi.org/10.1038/s41598-022-15977-y [Google Scholar] [PubMed] [CrossRef]
Keeble AH, Turkki P, Stokes S, Khairil Anuar INA, Rahikainen R, Hytönen VP, Howarth M (2019). Approaching infinite affinity through engineering of peptide-protein interaction. Proceedings of the National Academy of Sciences of the United States of America 116: 26523–26533. https://doi.org/10.1073/pnas.1909653116 [Google Scholar] [PubMed] [CrossRef]
Khalili M, Liwo A, Jagielska A, Scheraga HA (2005). Molecular dynamics with the united-residue model of polypeptide chains. II. Langevin and Berendsen-Bath dynamics and tests on model α-helical systems. The Journal of Physical Chemistry B 109: 13798–13810. https://doi.org/10.1021/jp058007w [Google Scholar] [PubMed] [CrossRef]
Kiss PT, Baranyai A (2011). Sources of the deficiencies in the popular SPC/E and TIP3P models of water. The Journal of Chemical Physics 134: 054106. https://doi.org/10.1063/1.3548869 [Google Scholar] [PubMed] [CrossRef]
Kleinschmidt AT, Chen AX, Pascal TA, Lipomi DJ (2022). Computational modeling of molecular mechanics for the experimentally inclined. Chemistry of Materials 34: 7620–7634. https://doi.org/10.1021/acs.chemmater.2c00292 [Google Scholar] [CrossRef]
Klemm T, Ebert G, Calleja DJ, Allison CC, Richardson LW et al. (2020). Mechanism and inhibition of the papain-like protease, PLpro, of SARS-CoV-2. The EMBO Journal 39: e106275. https://doi.org/10.15252/embj.2020106275 [Google Scholar] [PubMed] [CrossRef]
Koley T, Kumar M, Goswami A, Ethayathulla AS, Hariprasad G (2022). Structural modeling of Omicron spike protein and its complex with human ACE-2 receptor: Molecular basis for high transmissibility of the virus. Biochemical and Biophysical Research Communications 592: 51–53. https://doi.org/10.1016/j.bbrc.2021.12.082 [Google Scholar] [PubMed] [CrossRef]
Kondori J, Zendehboudi S, Hossain ME (2017). A review on simulation of methane production from gas hydrate reservoirs: Molecular dynamics prospective. Journal of Petroleum Science and Engineering 159: 754–772. https://doi.org/10.1016/j.petrol.2017.09.073 [Google Scholar] [CrossRef]
Kumar AD, Naveen S, Vivek HK, Prabhuswamy M, Lokanath NK, Kumar KA (2016). Synthesis, crystal and molecular structure of ethyl 2-(4-chlorobenzylidene)-3-oxobutanoate: Studies on antioxidant, antimicrobial activities and molecular docking. Chemical Data Collections 5: 36–45. [Google Scholar]
Kumari R, Kumar R, Lynn A (2014). g_mmpbsa—A GROMACS tool for high-throughput MM-PBSA calculations. Journal of Chemical Information and Modeling 54: 1951–1962. https://doi.org/10.1021/ci500020m [Google Scholar] [PubMed] [CrossRef]
Lee J, Cheng X, Swails JM, Yeom MS, Eastman PK et al. (2016). CHARMM-GUI input generator for NAMD, GROMACS, AMBER, OpenMM, and CHARMM/OpenMM simulations using the CHARMM36 additive force field. Journal of Chemical Theory and Computation 12: 405–413. https://doi.org/10.1021/acs.jctc.5b00935 [Google Scholar] [PubMed] [CrossRef]
Lemkul JA (2020). Chapter one—Pairwise-additive and polarizable atomistic force fields for molecular dynamics simulations of proteins. Progress in Molecular Biology and Translational Science 170: 1–71. [Google Scholar] [PubMed]
Linani A, Benarous K, Bou-Salah L, Yousfi M, Goumri-Said S (2022). Exploring structural mechanism of COVID-19 treatment with glutathione as a potential peptide inhibitor to the main protease: Molecular dynamics simulation and MM/PBSA free energy calculations study. International Journal of Peptide Research and Therapeutics 28: 559. https://doi.org/10.1007/s10989-022-10365-6 [Google Scholar] [PubMed] [CrossRef]
Lindahl E, Hess B, van der Spoel D (2001). GROMACS 3.0: A package for molecular simulation and trajectory analysis. Journal of Molecular Modeling 7: 306–317. https://doi.org/10.1007/s008940100045 [Google Scholar] [CrossRef]
Lindner HA, Lytvyn V, Qi H, Lachance P, Ziomek E, Ménard R (2007). Selectivity in ISG15 and ubiquitin recognition by the SARS coronavirus papain-like protease. Archives of Biochemistry and Biophysics 466: 8–14. https://doi.org/10.1016/j.abb.2007.07.006 [Google Scholar] [PubMed] [CrossRef]
Lindorff-Larsen K, Piana S, Palmo K, Maragakis P, Klepeis JL, Dror RO, Shaw DE (2010). Improved side-chain torsion potentials for the Amber ff99SB protein force field. Proteins: Structure, Function, and Bioinformatics 78: 1950–1958. https://doi.org/10.1002/prot.22711 [Google Scholar] [PubMed] [CrossRef]
Liu N, Zheng L, Xu J, Wang J, Hu C et al. (2021). Reduced graphene oxide membrane as supporting film for high-resolution cryo-EM. Biophysics Reports 7: 227–238. https://doi.org/10.52601/bpr.2021.210007 [Google Scholar] [PubMed] [CrossRef]
Luehr N, Jin AG, Martínez TJ (2015). Ab initio interactive molecular dynamics on graphical processing units (GPUs). Journal of Chemical Theory and Computation 11: 4536–4544. https://doi.org/10.1021/acs.jctc.5b00419 [Google Scholar] [PubMed] [CrossRef]
Luo H (2016). Interplay between the virus and the ubiquitin-proteasome system: Molecular mechanism of viral pathogenesis. Current Opinion in Virology 17: 1–10. https://doi.org/10.1016/j.coviro.2015.09.005 [Google Scholar] [PubMed] [CrossRef]
Ma C, Sacco MD, Hurst B, Townsend JA, Hu Y et al. (2020). Boceprevir, GC-376, and calpain inhibitors II, XII inhibit SARS-CoV-2 viral replication by targeting the viral main protease. Cell Research 30: 678–692. https://doi.org/10.1038/s41422-020-0356-z [Google Scholar] [PubMed] [CrossRef]
MackerellJr AD (2004). Empirical force fields for biological macromolecules: Overview and issues. Journal of Computational Chemistry 25: 1584–1604. https://doi.org/10.1002/(ISSN)1096-987X [Google Scholar] [CrossRef]
Mariano G, Farthing RJ, Lale-Farjat SLM, Bergeron JRC (2020). Structural characterization of SARS-CoV-2: Where we are, and where we need to be. Frontiers in Molecular Biosciences 7: 189. https://doi.org/10.3389/fmolb.2020.605236 [Google Scholar] [PubMed] [CrossRef]
Mengist HM, Dilnessa T, Jin T (2021). Structural basis of potential inhibitors targeting SARS-CoV-2 main protease. Frontiers in Chemistry 9: 590263. https://doi.org/10.3389/fchem.2021.622898 [Google Scholar] [PubMed] [CrossRef]
Monticelli L, Tieleman DP (2013). Force fields for classical molecular dynamics. In: Monticelli L, Salonen E (eds.Biomolecular Simulations: Methods and Protocols, pp. 197–213. Totowa, NJ: Humana Press. [Google Scholar]
Moradi M, Golmohammadi R, Najafi A, Moosazadeh Moghaddam M, Fasihi-Ramandi M, Mirnejad R (2022). In silico analysis of inhibiting papain-like protease from SARS-CoV-2 by using plant-derived peptides. International Journal of Peptide Research and Therapeutics 28: 24. https://doi.org/10.1007/s10989-021-10331-8 [Google Scholar] [PubMed] [CrossRef]
Mu, Kosov DS, Stock G (2003). Conformational dynamics of trialanine in water. 2. Comparison of AMBER, CHARMM, GROMOS, and OPLS force fields to NMR and infrared experiments. The Journal of Physical Chemistry B 107: 5064–5073. https://doi.org/10.1021/jp022445a [Google Scholar] [CrossRef]
Naqvi AAT, Fatima K, Mohammad T, Fatima U, Singh IK, Singh A, Atif SM, Hariprasad G, Hasan GM, Hassan MI (2020). Insights into SARS-CoV-2 genome, structure, evolution, pathogenesis and therapies: Structural genomics approach. Biochimica et Biophysica Acta—Molecular Basis of Disease 1866: 165878. https://doi.org/10.1016/j.bbadis.2020.165878 [Google Scholar] [PubMed] [CrossRef]
Ong JH, Liang CE, Wong WL, Wong FC, Chai TT (2021). Multi-target anti-SARS-CoV-2 peptides from mealworm proteins: An in silico study. Malaysian Journal of Biochemistry and Molecular Biology 24: 83–91. [Google Scholar]
Palmö K, Pietilä LO, Krimm S (1991). Construction of molecular mechanics energy functions by mathematical transformation of ab initio force fields and structures. Journal of Computational Chemistry 12: 385–390. https://doi.org/10.1002/jcc.540120312 [Google Scholar] [CrossRef]
Pant S, Singh M, Ravichandiran V, Murty USN, Srivastava HK (2020). Peptide-like and small-molecule inhibitors against COVID-19. Journal of Biomolecular Structure and Dynamics 39: 1–10. https://doi.org/10.1080/07391102.2020.1757510 [Google Scholar] [PubMed] [CrossRef]
Paredes-Ramos M, Conde Piñeiro E, Pérez-Sánchez H, López-Vilariño JM (2022). Evaluation of natural peptides to prevent and reduce the novel SARS-CoV-2 infection. Journal of Food Quality 2022. https://doi.org/10.1155/2022/2102937 [Google Scholar] [CrossRef]
Paschek D (2004). Temperature dependence of the hydrophobic hydration and interaction of simple solutes: An examination of five popular water models. The Journal of Chemical Physics 120: 6674–6690. https://doi.org/10.1063/1.1652015 [Google Scholar] [PubMed] [CrossRef]
Pei P, Qin H, Chen J, Wang F, He C et al. (2021). Computational design of ultrashort peptide inhibitors of the receptor-binding domain of the SARS-CoV-2 S protein. Briefings in Bioinformatics 22. https://doi.org/10.1093/bib/bbab243 [Google Scholar] [PubMed] [CrossRef]
Petushkova AI, ZamyatninJr AA (2020). Papain-like proteases as coronaviral drug targets: Current inhibitors, opportunities, and limitations. Pharmaceuticals 13: 277. https://doi.org/10.3390/ph13100277 [Google Scholar] [PubMed] [CrossRef]
Pradeep H, Najma U, Aparna HS (2021). Milk peptides as novel multi-targeted therapeutic candidates for SARS-CoV2. Protein Journal 40: 310–327. https://doi.org/10.1007/s10930-021-09983-8 [Google Scholar] [PubMed] [CrossRef]
Rangaswamy AN, Ashok A, Hanumanthappa P, Chandrashekaramurthy AS, Kumbaiah M, Hiregouda P, Sharma V, Sosalegowda AH (2021). Identification of potential peptide inhibitors of ACE-2 target of SARS-CoV-2 from buckwheat & quinoa. International Journal of Peptide Research and Therapeutics 27: 1799–1813. https://doi.org/10.1007/s10989-021-10211-1 [Google Scholar] [PubMed] [CrossRef]
Riniker S (2018). Fixed-charge atomistic force fields for molecular dynamics simulations in the condensed phase: An overview. Journal of Chemical Information and Modeling 58: 565–578. https://doi.org/10.1021/acs.jcim.8b00042 [Google Scholar] [PubMed] [CrossRef]
Rogge SMJ, Vanduyfhuys L, Ghysels A, Waroquier M, Verstraelen T, Maurin G, van Speybroeck V (2015). A comparison of barostats for the mechanical characterization of metal-organic frameworks. Journal of Chemical Theory and Computation 11: 5583–5597. https://doi.org/10.1021/acs.jctc.5b00748 [Google Scholar] [PubMed] [CrossRef]
Rut W, Lv Z, Zmudzinski M, Patchett S, Nayak D et al. (2020). Activity profiling and crystal structures of inhibitor-bound SARS-CoV-2 papain-like protease: A framework for anti–COVID-19 drug design. Science Advances 6: eabd4596. https://doi.org/10.1126/sciadv.abd4596 [Google Scholar] [PubMed] [CrossRef]
Sadremomtaz A, Al-Dahmani ZM, Ruiz-Moreno AJ, Monti A, Wang C et al. (2022). Synthetic peptides that antagonize the angiotensin-converting enzyme-2 (ACE-2) interaction with SARS-CoV-2 receptor binding spike protein. Journal of Medicinal Chemistry 65: 2836–2847. https://doi.org/10.1021/acs.jmedchem.1c00477 [Google Scholar] [PubMed] [CrossRef]
Sami S, Menger MFSJ, Faraji S, Broer R, Havenith RWA (2021). Q-force: Quantum mechanically augmented molecular force fields. Journal of Chemical Theory and Computation 17: 4946–4960. https://doi.org/10.1021/acs.jctc.1c00195 [Google Scholar] [PubMed] [CrossRef]
Sasidharan S, Selvaraj C, Singh SK, Dubey VK, Kumar S, Fialho AM, Saudagar P (2021). Bacterial protein azurin and derived peptides as potential anti-SARS-CoV-2 agents: Insights from molecular docking and molecular dynamics simulations. Journal of Biomolecular Structure and Dynamics 39: 5706–5721. https://doi.org/10.1080/07391102.2020.1787864 [Google Scholar] [PubMed] [CrossRef]
Schrödinger L, DeLano W (2020). PyMOL. http://www.pymol.org/pymol. [Google Scholar]
Settanni L, Corsetti A (2008). Application of bacteriocins in vegetable food biopreservation. International Journal of Food Microbiology 121: 123–138. https://doi.org/10.1016/j.ijfoodmicro.2007.09.001 [Google Scholar] [PubMed] [CrossRef]
Shabane PS, Izadi S, Onufriev AV (2019). General purpose water model can improve atomistic simulations of intrinsically disordered proteins. Journal of Chemical Theory and Computation 15: 2620–2634. https://doi.org/10.1021/acs.jctc.8b01123 [Google Scholar] [PubMed] [CrossRef]
Shirbhate E, Pandey J, Patel VK, Kamal M, Jawaid T, Gorain B, Kesharwani P, Rajak H (2021). Understanding the role of ACE-2 receptor in pathogenesis of COVID-19 disease: A potential approach for therapeutic intervention. Pharmacological Reports 73: 1539–1550. https://doi.org/10.1007/s43440-021-00303-6 [Google Scholar] [PubMed] [CrossRef]
Singh A, Tessier MB, Pederson K, Wang X, Venot AP, Boons GJ, Prestegard JH, Woods RJ (2016). Extension and validation of the GLYCAM force field parameters for modeling glycosaminoglycans. Canadian Journal of Chemistry 94: 927–935. https://doi.org/10.1139/cjc-2015-0606 [Google Scholar] [PubMed] [CrossRef]
Sitthiyotha T, Chunsrivirot S (2020). Computational design of 25-mer peptide binders of SARS-CoV-2. The Journal of Physical Chemistry B 124: 10930–10942. https://doi.org/10.1021/acs.jpcb.0c07890 [Google Scholar] [PubMed] [CrossRef]
Söderhjelm P, Ryde U (2009). How accurate can a force field become? A polarizable multipole model combined with fragment-wise quantum-mechanical calculations. The Journal of Physical Chemistry A 113: 617–627. https://doi.org/10.1021/jp8073514 [Google Scholar] [PubMed] [CrossRef]
Song W, Gui M, Wang X, Xiang Y (2018). Cryo-EM structure of the SARS coronavirus spike glycoprotein in complex with its host cell receptor ACE2. PLoS Pathogens 14: e1007236. https://doi.org/10.1371/journal.ppat.1007236 [Google Scholar] [PubMed] [CrossRef]
Sonibare K, Rathnayaka L, Zhang L (2020). Comparison of CHARMM and OPLS-aa forcefield predictions for components in one model asphalt mixture. Construction and Building Materials 236: 117577. https://doi.org/10.1016/j.conbuildmat.2019.117577 [Google Scholar] [CrossRef]
Struck A-W, Axmann M, Pfefferle S, Drosten C, Meyer B (2012). A hexapeptide of the receptor-binding domain of SARS corona virus spike protein blocks viral entry into host cells via the human receptor ACE2. Antiviral Research 94: 288–296. https://doi.org/10.1016/j.antiviral.2011.12.012 [Google Scholar] [PubMed] [CrossRef]
Tachoua W, Kabrine M, Mushtaq M, Ul-Haq Z (2020). An in-silico evaluation of COVID-19 main protease with clinically approved drugs. Journal of Molecular Graphics and Modelling 101: 107758. https://doi.org/10.1016/j.jmgm.2020.107758 [Google Scholar] [PubMed] [CrossRef]
Tallei TE, Fatimawali, Adam AA, Elseehy MM, El-Shehawi AM et al. (2022). Fruit bromelain-derived peptide potentially restrains the attachment of SARS-CoV-2 variants to hACE2: A pharmacoinformatics approach. Molecules 27: 260. https://doi.org/10.3390/molecules27010260 [Google Scholar] [PubMed] [CrossRef]
Tripathi MK, Singh P, Sharma S, Singh TP, Ethayathulla AS, Kaur P (2021). Identification of bioactive molecule from Withania somnifera (Ashwagandha) as SARS-CoV-2 main protease inhibitor. Journal of Biomolecular Structure and Dynamics 39: 5668–5681. https://doi.org/10.1080/07391102.2020.1790425 [Google Scholar] [PubMed] [CrossRef]
Tuckerman ME, Martyna GJ (2000). Understanding modern molecular dynamics: Techniques and applications. The Journal of Physical Chemistry B 104: 159–178. https://doi.org/10.1021/jp992433y [Google Scholar] [CrossRef]
Vankadari N (2020). Structure of furin protease binding to SARS-CoV-2 spike glycoprotein and implications for potential targets and virulence. The Journal of Physical Chemistry Letters 11: 6655–6663. https://doi.org/10.1021/acs.jpclett.0c01698 [Google Scholar] [PubMed] [CrossRef]
Wang YQ, Li QS, Zheng XQ, Lu JL, Liang YR (2021). Antiviral effects of green tea EGCG and its potential application against COVID-19. Molecules 26: 3962. https://doi.org/10.3390/molecules26133962 [Google Scholar] [PubMed] [CrossRef]
Wang L, O’Mara ML (2021). Effect of the force field on molecular dynamics simulations of the multidrug efflux protein p-glycoprotein. Journal of Chemical Theory and Computation 17: 6491–6508. https://doi.org/10.1021/acs.jctc.1c00414 [Google Scholar] [PubMed] [CrossRef]
Wang J, Wang W, Kollman PA, Case DA (2006). Automatic atom type and bond type perception in molecular mechanical calculations. Journal of Molecular Graphics and Modelling 25: 247–260. https://doi.org/10.1016/j.jmgm.2005.12.005 [Google Scholar] [PubMed] [CrossRef]
Wong FC, Ong JH, Chai TT (2020). Identification of putative cell-entry-inhibitory peptides against SARS-CoV-2 from edible insects: An in silico study. eFood 1: 357–368. https://doi.org/10.2991/efood.k.200918.002 [Google Scholar] [CrossRef]
Wong FC, Ong JH, Chai TT (2021a). SARS-CoV-2 spike protein-, main protease- and papain-like-protease-targeting peptides from seed proteins following gastrointestinal digestion: An in silico study. Phytomedicine Plus 1: 100016. https://doi.org/10.1016/j.phyplu.2020.100016 [Google Scholar] [PubMed] [CrossRef]
Wong FC, Ong JH, Kumar DT, Chai TT (2021b). In silico identification of multi-target anti-SARS-CoV-2 peptides from quinoa seed proteins. International Journal of Peptide Research and Therapeutics 27: 1837–1847. https://doi.org/10.1007/s10989-021-10214-y [Google Scholar] [PubMed] [CrossRef]
Wu J, Deng W, Li S, Yang X (2021). Advances in research on ACE2 as a receptor for 2019-nCoV. Cellular and Molecular Life Sciences 78: 531–544. https://doi.org/10.1007/s00018-020-03611-x [Google Scholar] [PubMed] [CrossRef]
Xue LC, Rodrigues JP, Kastritis PL, Bonvin AM, Vangone A (2016). PRODIGY: A web server for predicting the binding affinity of protein-protein complexes. Bioinformatics 32: 3676–3678. https://doi.org/10.1093/bioinformatics/btw514 [Google Scholar] [PubMed] [CrossRef]
Yan R, Zhang Y, Li Y, Ye F, Guo Y, Xia L, Zhong X, Chi X, Zhou Q (2021). Structural basis for the different states of the spike protein of SARS-CoV-2 in complex with ACE2. Cell Research 31: 717–719. https://doi.org/10.1038/s41422-021-00490-0 [Google Scholar] [PubMed] [CrossRef]
Yang SC, Lin CH, Sung CT, Fang JY (2014). Antibacterial activities of bacteriocins: Application in foods and pharmaceuticals. Frontiers in Microbiology 5: 241. https://doi.org/10.3389/fmicb.2014.00241 [Google Scholar] [PubMed] [CrossRef]
Yathisha UG, Srinivasa MG, Siddappa BCR, Mandal S, Dixit SR, Pujar GV, Bangera Sheshappa M (2022). Isolation and characterization of ACE-I inhibitory peptides from ribbonfish for a potential inhibitor of the main protease of SARS-CoV-2: An in silico analysis. Proteins: Structure, Function and Bioinformatics 90: 982–992. https://doi.org/10.1002/prot.26291 [Google Scholar] [PubMed] [CrossRef]
Yu Z, Kan R, Ji H, Wu S, Zhao W, Shuian D, Liu J, Li J (2021). Identification of tuna protein-derived peptides as potent SARS-CoV-2 inhibitors via molecular docking and molecular dynamic simulation. Food Chemistry 342: 128366. https://doi.org/10.1016/j.foodchem.2020.128366 [Google Scholar] [PubMed] [CrossRef]
Zavodszky MI, Kuhn LA (2005). Side-chain flexibility in protein-ligand binding: the minimal rotation hypothesis. Protein Science 14: 1104–1114. https://doi.org/10.1110/ps.041153605 [Google Scholar] [PubMed] [CrossRef]
Zhang S, Zhong N, Xue F, Kang X, Ren X, Chen J, Jin C, Lou Z, Xia B (2010). Three-dimensional domain swapping as a mechanism to lock the active conformation in a super-active octamer of SARS-CoV main protease. Protein & Cell 1: 371–383. https://doi.org/10.1007/s13238-010-0044-8 [Google Scholar] [PubMed] [CrossRef]
Zhao W, Li X, Yu Z, Wu S, Ding L, Liu J (2022). Identification of lactoferrin-derived peptides as potential inhibitors against the main protease of SARS-CoV-2. LWT 154: 112684. https://doi.org/10.1016/j.lwt.2021.112684 [Google Scholar] [PubMed] [CrossRef]
Cite This Article
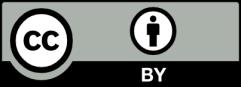
This work is licensed under a Creative Commons Attribution 4.0 International License , which permits unrestricted use, distribution, and reproduction in any medium, provided the original work is properly cited.