Open Access
ARTICLE
Exploring the mechanisms of magnolol in the treatment of periodontitis by integrating network pharmacology and molecular docking
Department of Veterinary Medicine, National Chung Hsing University, Taichung, Taiwan
* Corresponding Author: CHENG-HUNG LAI. Email:
(This article belongs to the Special Issue: Natural Products for Chronic Inflammatory Diseases: Pharmacology and Toxicology)
BIOCELL 2023, 47(6), 1317-1327. https://doi.org/10.32604/biocell.2023.028883
Received 13 January 2023; Accepted 10 March 2023; Issue published 19 May 2023
Abstract
Background: Magnolol, a bioactive extract of the Chinese herb Magnolia officinalis has a protective effect against periodontitis. This study is aimed to explore the mechanisms involved in the functioning of magnolol against periodontitis and provide a basis for further research. Methods: Network pharmacology analysis was performed based on the identification of related targets from public databases. The Protein-protein interaction (PPI) network was constructed to visualize the significance between the targets of magnolol and periodontitis. Subsequently, Gene ontology (GO) and Kyoto Encyclopedia of Genes and Genomes (KEGG) analysis were performed to predict the functions and the signal regulatory pathways involved in the action of magnolol against periodontitis. The “function-target-pathway” networks were constructed to analyze the core targets and pathways of magnolol against periodontitis. Molecular docking was used to verify the interaction of magnolol and core targets. Results: A total of 58 active targets of magnolol and 644 periodontitis-related targets were collected from public databases. A total of 25 targets of magnolol against periodontitis were identified based on the Venn diagram. GO analysis showed that magnolol has a role in the response to oxidative stress, nicotine, and lipopolysaccharide. KEGG enrichment analysis indicated that the mechanism of magnolol against periodontitis was mainly related to the tumor necrosis factor (TNF), phosphoinositide 3-kinase (PI3K/Akt), and mitogen-activated protein kinase (MAPK) signaling pathways. Combined with PPI network and molecular docking results, the core targets of magnolol against periodontitis included AKT1, MAPK8, MAPK14, TNF, and TP53. Conclusion: To summarize, the anti-periodontitis mechanisms of magnolol are potentially through regulating the TNF, PI3K/Akt, and MAPK signaling pathways.Keywords
Periodontitis is a chronic inflammatory disease (Sanz et al., 2020), causing chronic pain, gingival inflammation, and alveolar bone loss (Carvalho et al., 2021). Periodontitis is initiated by disturbances in the balance of the local microbial community (George, 2015). This affects oral health and may aggravate various systemic diseases like type 2 diabetes (Piero et al., 2019). Recent studies showed that periodontitis is the sixth most prevalent disease with a prevalence rate of approximately 50% (GBD 2017 Disease and Injury Incidence and Prevalence Collaborators, 2018). The periodontal treatment mainly focuses on eliminating dental plaque to reduce the number of bacteria, thereby stopping inflammation and its progression. Notably, the use of a local chemotherapeutic agent as an adjunctive to scaling and root planing (SRP) is regarded as the first treatment of option given its limited invasiveness (Taalab et al., 2021). The standard drugs employed for treating periodontitis are antibiotics and oral antiseptics including amoxicillin, metronidazole, and cefradine (Feres et al., 2021). However, the effects of these drugs are limited and also show inevitable drug toxicity. Traditional Chinese medicine (TCM) that emphasizes the holistic treatment has distinctive advantages such as less toxicity in the treatment of periodontitis. Nevertheless, there is lack of the research on the mechanisms of TCM use in treating periodontitis.
Magnolol, the major bioactive polyphenolic component of Magnolia officinalis, is known as a TCM in the treatment of gastrointestinal and kidney disorders (Lee et al., 2011). It possesses diverse biological functions, such as anti-cancer, anti-inflammation, and anti-oxidation (Chiu et al., 2020; Fukuyama et al., 2020). Multiple studies demonstrated that magnolol plays important roles against tumorigenesis by inhibiting proliferation, migration, and invasion of cancer cells (Shen et al., 2017; Zhu et al., 2019). Magnolol also can mitigate the inflammation and oxidative stress induced by periodontitis. For example, Lu et al. (2015) reported that magnolol inhibited P. gingivalis lipopolysaccharide-induced inflammation in macrophages (Lu et al., 2015). In another study, Liu et al. (2021) found that magnolol was beneficial to improve the impaired wound healing and inflammation caused by combined diabetes mellitus and periodontitis. While these findings suggest that magnolol could be a potential adjuvant for the treatment of periodontitis, the underlying therapeutic mechanisms remain unclear.
Network pharmacology is a powerful research tool based on the theory of pharmacology, biology, and bioinformatics to reveal the mechanisms of disease treatment (Xiong et al., 2020). In recent years, network pharmacology has emerged as a popular method to investigate the potential mechanism of TCM in disease treatments (Chen et al., 2018; Wu et al., 2020). Complex ingredients of TCM present great difficulty in the development of TCM research. Network pharmacology constructs and analyzes the complex “compound-target-disease” network from a systematic and holistic perspective. This approach is different from traditional pharmacology research methods (Shao and Zhang, 2013; Wang et al., 2021). Thus, we used the pharmacology network to investigate the potential mechanism of magnolol against periodontitis.
In this study, network pharmacology was used to screen targets of magnolol in treating periodontitis. Then, the anti-periodontitis mechanisms of magnolol were predicted by GO and KEGG enrichment analysis. Finally, molecular docking technology was used to verify the interaction of magnolol and anti-periodontitis targets. The research flow chart has been presented in Fig. 1.
Figure 1: Flow chart of the research in this study.
Identification of magnolol-related targets
Magnolol-related targets were obtained from Herb Ingredients’ Targets (HIT) (http://hit2.badd-cao.net/) (Yan et al., 2021) and GeneCards (https://www.genecards.org/) (Marilyn et al., 2010) databases. The keyword “magnolol” was applied as a search term, and duplicated targets or those without a Uniprot ID were removed.
Potential targets related to periodontitis
GeneCards, DisGeNET (https://www.disgenet.org/) (Piero et al., 2019), and Therapeutic Target Database (TTD) (http://db.idrblab.net/ttd/) (Chen et al., 2002) databases were used to screen the human periodontitis-related targets. The repeated targets and those with no Uniprot IDs were excluded. VennDiagram (version 1.7.3) (Chen and Boutros, 2011) was used to analyze the overlapping targets of magnolol against periodontitis.
Construction of the protein-protein interaction network
Based on the results of the Venn diagram, the overlapping targets of magnolol and periodontitis were imported into the STRING database to construct the PPI network (Damian et al., 2020). The standard of interaction degree value was set as >0.4. The complex relationships were visualized by ggraph (version 2.0.5) and igraph (version 1.3.1) of the R package, and then cytoHubba was used to select hub genes (Chin et al., 2014).
Functional enrichment analysis
Gene Ontology (GO) and Kyoto Encyclopedia of Genes and Genomes (KEGG) pathway enrichment were conducted using clusterProfiler (version 4.4.2) (Yu et al., 2012). The principle of KEGG analysis is based on the hypergeometric distribution model that evaluates the significance of the target gene set with the related to special gene and signaling pathway. In addition, a p-value < 0.05 was kept as significant in the GO and KEGG analysis in this study. The cytoHubba was applied to select the core targets based on GO and KEGG results, and the “function-target-pathway” network was conducted using the R language (circlize) (Gu et al., 2014).
Structures of the core target proteins were downloaded from the Research Collaboratory for Structural Bioinformatics Protein Data Bank (RCSB PDB, http://www.pdb.org/). Pymol and Autodock1.5.6 software were used to modify the protein structure by the removal of original ligands and water molecules, the addition of hydrogen, optimization of amino acids, and calculation of charges (Seeliger and Groot, 2013). The structure file of magnolol in mol2 format was downloaded from the ZINC (https://zinc.docking.org/) database. Open Babel GUI software was used to transform the downloaded protein and magnolol file into the PDBQT version. Finally, molecular docking was operated by Autodock1.5.6, and the results were visualized by Pymol.
Identification of targets of magnolol against periodontitis
According to the HIT and GeneCards databases, a total of 58 targets of magnolol were obtained after removing duplicates and targets without a UniProt ID. GeneCards, DisGeNET, and TTD databases were employed for searching periodontitis-related targets. A total of 664 targets was retrieved by removing duplicates. Then, using VennDiagram (Version 1.7.3), a total of 25 targets of magnolol in the treatment of periodontitis were selected (Table 1 and Fig. 2).
Figure 2: Venn diagram of the 25 intersecting targets related to magnolol and periodontitis.
PPI network of targets of magnolol against periodontitis
To investigate the interaction of 25 potential targets for magnolol against periodontitis, the PPI network of these targets was constructed, and a total of 207 interaction relationships were obtained. Then, we put the network into cytoHubaba and identified the top 10 hub targets with high connectivity which are presented as circle points in Fig. 3. These include tumor protein p53 (TP53), AKT serine/threonine kinase 1 (AKT1 1), tumor necrosis factor (TNF), mitogen-activated protein kinase 14 (MAPK14), caspase 3 (CASP3), matrix metallopeptidase 9 (MMP9), epidermal growth factor receptor (EGFR), catenin beta 1 (CTNNB1), epidermal growth factor (EGF), and mitogen-activated protein kinase 8 (MAPK8).
Figure 3: Protein-protein interaction (PPI) network of the 25 hub targets of magnolol against periodontitis.
Gene ontology and kyoto encyclopedia of genes and genomes enrichment results
A total of 25 hub genes of magnolol against periodontitis were analyzed by GO and KEGG enrichment. GO function enrichment showed that these 25 targets included 812 biological process (BP) terms, 11 cellular component (CC) terms, and 20 molecular function (MF) terms. The top 15 GO terms have been presented in Fig. 4A and Table 2. These include cellular response to chemical and oxidative stress (BP), vesicle lumen (CC), and phosphatase binding (MF). According to the KEGG pathway enrichment analysis, there were 128 related pathways enriched by targets, and the top 15 signaling pathways have been presented in Fig. 4B and Table 3. The results demonstrated that magnolol is mainly related to the PI3k/Akt, TNF, and MAPK signaling pathways (Fig. 4B).
Figure 4: Gene ontology (GO) and Kyoto Encyclopedia of Genes and Genomes (KEGG) enrichment analysis of 25 targets of magnolol against periodontitis. (A) The top 15 significantly enriched GO terms, including 5 biological progress (BP) terms, 5 cellular component (CC) terms, and 5 molecular function (MF) terms; (B) The top 15 KEGG pathways enrichment terms; (C) The “function-target-pathway” network based on the 25 core targets with top 15 GO terms and KEGG pathways; (D) The network of 10 key targets related to top 15 GO terms and KEGG enrichment pathways.
Combined with core targets, top 15 GO functions, and KEGG pathways of magnolol against periodontitis, the “function-target-pathway” network was constructed (Fig. 4C). Meanwhile, cytoHubba was used to select the top 10 core genes according to the maximal clique centrality (MCC) score. The top 10 core targets were MAPK1, TNF, AKT1, CASP3, MAPK8, NFKB1, BCL2, MAPK14, TP53, and EGFR. Results showed the top 15 GO functions and KEGG pathways were mainly associated with MAPK1 (25 enriched GO terms and pathways), TNF (21 enriched GO terms and pathways), and CASP3 (20 enriched GO terms and pathways). The top 10 core targets were largely enriched in the cellular response to chemical stress (7 targets), response to oxidative stress (7 targets), and response to lipopolysaccharide (6 targets). Additionally, the core targets were mainly related to the MAPK signaling pathway (9 enrichment targets), TNF signaling pathway (7 enrichment targets), as well as PI3K/Akt signaling pathway (6 enrichment targets) (Fig. 4D).
From the intersection of hub targets from the PPI and GO analysis, and KEGG networks, a total of 7 targets (TP53, AKT1, TNF, MAPK14, CASP3, EGFR, MAPK8) were selected and further used for molecular docking with magnolol (Fig. 5A). In order to verify the accuracy of magnolol and core target by molecular docking, the standard of binding affinity was set as <−5.0 kcal/mol, which suggested the ligand has a good binding activity to the receptor protein. As shown in Table 4 and Figs. 5B–5F, magnolol showed good binding with 5 core targets, namely MAPK14 (binding affinity = −6.54 kcal/mol, Fig. 5B), TP53 (binding affinity = −6.15 kcal/mol, Fig. 5C), TNF (binding affinity = −6 kcal/mol, Fig. 5D), MAPK8 (binding affinity = −5.7 kcal/mol, Fig. 5E), and AKT (binding affinity = −5.67 kcal/mol, Fig. 5F).
Figure 5: Molecular docking of core targets with magnolol. (A) Venn diagram of the hub-genes from Protein-Protein Interaction (PPI) and Gene ontology and Kyoto Encyclopedia of Genes and Genomes (GO + KEGG network); (B) Magnolol bound with mitogen-activated protein kinase 14 (MAPK14), binding affinity = −6.54 kcal/mol; (C) Magnolol bound with tumor protein p53 (TP53), binding affinity = −6.15 kcal/mol; (D) Magnolol bound with tumor necrosis factor (TNF), binding affinity = −6 kcal/mol; (E) Magnolol bound with mitogen-activated protein kinase 8 (MAPK8), binding affinity = −5.7 kcal/mol; (F) Magnolol bound with AKT1, binding affinity = −5.67 kcal/mol. Red boxes represent binding sites.
Periodontitis is a noninfectious chronic disease initiated by dysbiosis of the local microbial community (Lin et al., 2022). Periodontitis was shown to lead to the direct activation of osteoclast activity, and eventually cause the loss of alveolar bone due to the overactivation of the host immune response (Lamont et al., 2018). In short term, the traditional treatment methods including SRP (scaling and root planning) and oral antiseptics are effective in reducing the impact of periodontitis and improving the clinical symptoms (Elashiry et al., 2021). However, the effects of these therapies are limited and non-selective. The treatment of periodontitis is aimed to reduce the risk of infection and inflammation. In recent years, TCM the compounds used have been reported to have significant therapeutic effects on health disorders including periodontitis (Yuan et al., 2021; Scab et al., 2019). Magnolol, a binaphthalene polyphenolic compound derived from the stem bark and root of Magnolia officinalis is widely used in the treatment of inflammation, anxiety, etc. (Yang et al., 2008; Weeks, 2009; Cicalău et al., 2021). Previous studies showed that magnolol could alleviate the clinical symptoms associated with periodontitis (Ho et al., 2001; Lu et al., 2013). However, the mechanistic aspects of how magnolol functions in the treatment of periodontitis are still unclear. Through network pharmacology, we obtained 25 potential targets for magnolol against periodontitis. Network pharmacology, a new research tool, is largely used to reveal the complex mechanisms of TCM in treatment (Bi et al., 2021; Zhang et al., 2021). In the current study, we used network pharmacology to investigate the possible mechanisms of magnolol in the treatment of periodontitis.
Significantly, GO function analysis demonstrated that the 25 targets were mainly enriched in BPs, such as responses to oxidative stress, nicotine, and lipopolysaccharide. Oxidative stress has been demonstrated to be a crucial contributing factor in the development of periodontitis through the overaccumulation of reactive oxygen species (Sczepanik et al., 2020). For example, Ying et al. (2020) found that low-intensity pulsed ultrasound could eliminate oxidative stress through the PI3K-Akt/Nrf2 pathway to regulate alveolar bone homeostasis (Ying et al., 2020). Despite magnolol having shown anti-oxidative stress potential in several disorders such as acute alcoholic liver damage (Liu et al., 2019) and multiple sclerosis (Bibi et al., 2022), its effect on oxidative stress in periodontitis remains largely unclear. Interestingly, a previous investigation indicated that magnolol could inhibit oxidative stress to ameliorate the symptoms of diabetic periodontitis, which may account for the findings of the present study (Liu et al., 2021). Smoking is a risk factor for periodontitis as it disrupts the repair process during subsequent periodontal treatment (Garcia et al., 2018). A previous study indicated that nicotine consumption enhanced the inflammatory response and the development of periradicular lesions in rats (Pinto et al., 2020). Lipopolysaccharide, which is a bacterial virulence factor, is one of the main causes of the aggravation of edema and vasodilation in inflammatory periodontal tissues (Kim et al., 2021). A previous study reported that the activity of circulating lipopolysaccharide was increased in periodontitis patients, suggesting the essential role of targeting lipopolysaccharide in the treatment of periodontitis (Pussinen et al., 2022). Collectively, our findings demonstrated that the potential targets of magnolol against periodontitis were mainly implicated in the regulation of oxidative stress, nicotine, and lipopolysaccharide.
The KEGG pathway enrichment analysis showed that magnolol exerted its anti-periodontitis effect through multiple signaling pathways, including the TNF pathway, MAPK pathway, and the phosphatidylinositol-3-kinase (PI3K)-Akt signaling pathway. The TNF signaling pathway shows significant effects on the response to inflammation, regulation of the immune system, and induction of cell proliferation among many human pro-cancer processes (Lousa et al., 2022). The MAPK signaling pathway is involved in diverse inflammatory responses including regulating the expression levels of inflammatory factors, activating the immune system, and degrading matrix components (Kim et al., 2018). It was previously documented that the activation of the MAPK pathway in periodontitis aggravated inflammation and alveolar bone loss (Wang et al., 2020). Another study revealed that inhibition of the MAPK/c-fos/NFATC1 pathway could alleviate ligation-induced periodontitis (Xu et al., 2020). Notably, a previous study suggested that magnolol might suppress periodontitis by regulating the p38 MAPK signaling pathway (Lu et al., 2015). PI3K is a serine/threonine and lipid kinase, and Akt is the main downstream target of PI3K. PI3K/Akt signaling participates in the regulation of cell autophagy. Liu et al. (2018) reported that the lipopolysaccharide-induced inhibition of the PI3k/Akt/mTOR signaling pathway could promote the autophagy of human gingival fibroblasts (Liu et al., 2018). Taken together, KEGG enrichment analysis revealed that TNF, MAPK, and PI3K/Akt signaling pathways might be involved in the functional mechanisms of magnolol against periodontitis. In addition, we reported 10 hub targets from the PPI network, namely TP53, AKT1, TNF, MAPK14, CASP3, MMP9, EGFR, CTNNB1, EGF, and MAPK8. Further, 7 core targets (TP53, AKT1, TNF, MAPK14, CASP3, EGFR, and MAPK8) were obtained from the “function-target-pathway” network for molecular docking. The results showed that the binding energies of magnolol with AKT1, MAPK8, MAPK14, TNF, and TP53 were less than −5.0 kcal/mol. This suggests a good binding capability between these molecules. AKT1 is a serine/threonine kinase, which has critical roles in the regulation of osteoblast differentiation, suggesting the importance of AKT1 in the treatment of periodontitis (Eiichi et al., 2014). Several natural compounds, such as panduratin A, have been demonstrated to suppress osteoclastogenesis to prevent periodontitis progression by inhibiting MAPK signaling (Kim et al., 2018). A previous study found elevated MAPK8 in the human gingival fibroblasts under the pathological conditions of periodontitis (Herath et al., 2013). Moreover, another study indicated that suppression of the MAPK14 signaling could ameliorate the symptoms of periodontitis (Wang et al., 2021). Despite these findings, research into the role of MAPK8 and MAPK14 in the pathological process of periodontitis remains scarce. Unlike previous research, our study revealed the significance of both MAPK8 and MAPK14 in the anti-periodontitis mechanisms of magnolol functioning. TNF, an essential cytokine, which is related to the innate response to periodontal pathogens (Aleksandrowicz et al., 2021). Notably, a previous study revealed that the expression of TNF was remarkably increased in different inflammatory disorders including periodontitis (Li et al., 2020). TP53, a transcription factor, has direct effects on the processes of proliferation and differentiation in dental stem cells (Chen et al., 2021). The increased activity of TP53 could induce an immune and inflammatory response which promotes the occurrence of diseases including periodontitis (McCubrey et al., 2022). Collectively, magnolol could act on AKT1, MAPK8, MAPK14, TNF, and TP53 to exert the therapeutic effect on periodontitis.
Our study has some limitations. We only verified the interaction between the core targets and magnolol through molecular docking however, the effect of protein-molecule interactions counts on the binding site of the proteins. Besides, the crosstalk between the key targets and pathways revealed by network pharmacology analysis should be further elucidated. Additional in vitro and in vivo experiments are required to verify the pharmacological effect of magnolol against periodontitis and its mechanisms, which will be conducted in our subsequent investigations.
In conclusion, our findings demonstrated the multi-target and multi-pathway mechanisms of magnolol functions in the treatment of periodontitis. AKT1, MAPK8, MAPK14, TNF, and TP53 were revealed as core targets of magnolol against periodontitis. The underlying mechanisms of magnolol against periodontitis might be associated with the regulation of AKT, MAPK, and TNF signaling pathways. Our study provides the theoretical basis for the further investigations on the mechanisms of magnolol in treating periodontitis.
Funding Statement: The authors received no specific funding for this study.
Author Contributions: The authors confirm their contribution to the paper as follows: study conception and design: DC and CL; data collection: DC; analysis and interpretation of results: DC and CL; draft manuscript preparation: DC. All authors reviewed the results and approved the final version of the manuscript.
Availability of Data and Materials: The datasets used and/or analyzed during the current study are available from the corresponding author upon reasonable request.
Ethics Approval: Not applicable.
Conflicts of Interest: The authors declare that they have no conflicts of interest to report regarding the present study.
References
Aleksandrowicz P, Brzezińska-Błaszczyk E, Kozłowska E, Żelechowska P, Borgonovo A, Agier J (2021). Analysis of IL-1β, CXCL8, and TNF-α levels in the crevicular fluid of patients with periodontitis or healthy implants. BMC Oral Health 21: 120. https://doi.org/10.1186/s12903-021-01478-3 [Google Scholar] [PubMed] [CrossRef]
Bi J, Lin Y, Sun Y, Zhang M, Chen Q, Miu X, Tang L, Liu J, Zhu L, Ni Z (2021). Investigation of the active ingredients and mechanism of polygonum cuspidatum in asthma based on network pharmacology and experimental verification. Drug Design, Development Therapy 15: 1075–1089. https://doi.org/10.2147/DDDT.S275228 [Google Scholar] [PubMed] [CrossRef]
Bibi T, Khan A, Khan AU, Shal B, Ali H, Seo EK, Khan S (2022). Magnolol prevented brain injury through the modulation of Nrf2-dependent oxidative stress and apoptosis in PLP-induced mouse model of multiple sclerosis. Naunyn-Schmiedeberg’s Archives of Pharmacology 395: 717–733. https://doi.org/10.1007/s00210-022-02230-6 [Google Scholar] [PubMed] [CrossRef]
Carvalho R, Botelho J, Machado V, Mascarenhas P, Alcoforado G, Mendes J, Chambrone L (2021). Predictors of tooth loss during long-term periodontal maintenance: An updated systematic review. Journal of Clinical Periodontology 48: 1019–1036. https://doi.org/10.1111/jcpe.13488 [Google Scholar] [PubMed] [CrossRef]
Chen H, Boutros PC (2011). VennDiagram: A package for the generation of highly-customizable Venn and Euler diagrams in R. BMC Bioinformatics 12: 35. https://doi.org/10.1186/1471-2105-12-35 [Google Scholar] [PubMed] [CrossRef]
Chen L, Cao Y, Zhang H, Lv D, Zhao Y, Liu Y, Ye G, Chai Y (2018). Network pharmacology-based strategy for predicting active ingredients and potential targets of Yangxinshi tablet for treating heart failure. Journal of Ethnopharmacology 219: 359–368. https://doi.org/10.1016/j.jep.2017.12.011 [Google Scholar] [PubMed] [CrossRef]
Chen X, Ji ZL, Chen YZ (2002). TTD: Therapeutic target database. Nucleic Acids Research 30: 412–415. https://doi.org/10.1093/nar/30.1.412 [Google Scholar] [PubMed] [CrossRef]
Chen Y, Yu X, Kong J (2021). Identification of neuropeptides as potential crosstalks linking down syndrome and periodontitis revealed by transcriptomic analyses. Disease Markers 2021: 7331821. https://doi.org/10.1155/2021/7331821 [Google Scholar] [PubMed] [CrossRef]
Chin CH, Chen SH, Wu HH, Ho CW, Ko MT, Lin CY (2014). cytoHubba: Identifying hub objects and sub-networks from complex interactome. BMC Systems Biology 8: S11. https://doi.org/10.1186/1752-0509-8-S4-S11 [Google Scholar] [PubMed] [CrossRef]
Chiu KC, Shih YH, Wang TH, Lan WC, Li PJ, Juang HS, Hsia SM, Shen YW, Chen MYC, Shieh TM (2020). In vitro antimicrobial and antipro-inflammation potential of honokiol and magnolol against oral pathogens and macrophages. Journal of the Formosan Medical Association 120: 827–837. https://doi.org/10.1016/j.jfma.2020.09.002 [Google Scholar] [PubMed] [CrossRef]
Cicalău GIP, Babes PA, Calniceanu H, Popa A, Ciavoi G, Gilda ML, Ganea M, Scrobotă L (2021). Anti-inflammatory and antioxidant properties of carvacrol and magnolol, in periodontal disease and diabetes mellitus. Molecules 26: 6899. https://doi.org/10.3390/molecules26226899 [Google Scholar] [PubMed] [CrossRef]
Damian S, Gable AL, Nastou KC, David L, Rebecca K, Sampo P, Doncheva NT, Marc L, Fang T, Peer B (2020). The STRING database in 2021: Customizable protein-protein networks, and functional characterization of user-uploaded gene/measurement sets. Nucleic Acids Research 49: D605–D612. https://doi.org/10.1093/nar/gkaa1074 [Google Scholar] [PubMed] [CrossRef]
Eiichi S, Hiromi OS, Hideto A, Shoko O, Akiko S, Atsushi S, Toshifumi A, Chi ZJPO (2014). Akt activation is required for TGF-β1-induced osteoblast differentiation of MC3T3-E1 pre-osteoblasts. PLoS One 9: e112566. https://doi.org/10.1371/journal.pone.0112566 [Google Scholar] [PubMed] [CrossRef]
Elashiry M, Morandini AC, Timothius CJC, Ghaly M, Cutler CW (2021). Selective antimicrobial therapies for periodontitis: Win the “battle and the war”. International Journal of Molecular Sciences 22: 6459. https://doi.org/10.3390/ijms22126459 [Google Scholar] [PubMed] [CrossRef]
Feres M, Retamal-Valdes B, Fermiano D, Faveri M, Figueiredo L, Mayer M, Lee J, Bittinger K, Teles F (2021). Microbiome changes in young periodontitis patients treated with adjunctive metronidazole and amoxicillin. Journal of Periodontology 92: 467–478. https://doi.org/10.1002/jper.20-0128 [Google Scholar] [PubMed] [CrossRef]
Fukuyama Y, Kubo M, Harada K (2020). The search for, and chemistry and mechanism of, neurotrophic natural products. Journal of Natural Medicines 74: 648–671. https://doi.org/10.1007/s11418-020-01431-8 [Google Scholar] [PubMed] [CrossRef]
Garcia V, Gualberto E, Ervolino E, Nagata M, de Almeida J, Theodoro LJP, therapy p (2018). aPDT for periodontitis treatment in ovariectomized rats under systemic nicotine. Photodiagnosis and Photodynamic Therapy 22: 70–78. https://doi.org/10.1016/j.pdpdt.2018.02.017 [Google Scholar] [PubMed] [CrossRef]
GBD 2017 Disease and Injury Incidence and Prevalence Collaborators (2018). Global, regional, and national incidence, prevalence, and years lived with disability for 354 diseases and injuries for 195 countries and territories, 1990–2017: A systematic analysis for the Global Burden of Disease Study 2017. Lancet 392: 1789–1858. https://doi.org/10.1016/S0140-6736(18)32279-7 [Google Scholar] [PubMed] [CrossRef]
George H (2015). Periodontitis: From microbial immune subversion to systemic inflammation. Nature Reviews Immunology 15: 30–44. https://doi.org/10.1038/nri3785 [Google Scholar] [PubMed] [CrossRef]
Gu Z, Gu L, Eils R, Schlesner M, Brors B (2014). Circlize implements and enhances circular visualization in R. Bioinformatics 30: 2811–2812. https://doi.org/10.1093/bioinformatics/btu393 [Google Scholar] [PubMed] [CrossRef]
Herath TD, Darveau RP, Seneviratne CJ, Wang CY, Wang Y, Jin L (2013). Tetra- and penta-acylated lipid A structures of Porphyromonas gingivalis LPS differentially activate TLR4-mediated NF-κB signal transduction cascade and immuno-inflammatory response in human gingival fibroblasts. PLoS One 8: e58496. https://doi.org/10.1371/journal.pone.0058496 [Google Scholar] [PubMed] [CrossRef]
Ho KY, Tsai CC, Chen CP, Huang JS, Lin CC (2001). Antimicrobial activity of honokiol and magnolol isolated from Magnolia officinalis. Phytotherapy Research 15: 139–141. https://doi.org/10.1002/(ISSN)1099-1573 [Google Scholar] [CrossRef]
Kim H, Kim MB, Kim C, Hwang JK (2018). Inhibitory effects of panduratin a on periodontitis-induced inflammation and osteoclastogenesis through inhibition of MAPK pathways in vitro. Journal of Microbiology and Biotechnology 28: 190–198. https://doi.org/10.4014/jmb.1707.07042 [Google Scholar] [PubMed] [CrossRef]
Kim WJ, Park SY, Kim OS, Park HS, Jung JY (2021). Autophagy upregulates inflammatory cytokines in gingival tissue of patient with periodontitis and lipopolysaccharide-stimulated human gingival fibroblasts. Journal of Periodontology 93: 380–391. https://doi.org/10.1002/JPER.21-0178 [Google Scholar] [PubMed] [CrossRef]
Lamont RJ, Koo H, Hajishengallis G (2018). The oral microbiota: Dynamic communities and host interactions. Nature Reviews Microbiology 16: 745–759. https://doi.org/10.1038/s41579-018-0089-x [Google Scholar] [PubMed] [CrossRef]
Lee Y, Lee Y, Lee C, Jung J, Han S, Hong J (2011). Therapeutic applications of compounds in the Magnolia family. Pharmacology Therapeutics 130: 157–176. https://doi.org/10.1016/j.pharmthera.2011.01.010 [Google Scholar] [PubMed] [CrossRef]
Li Y, Yang J, Wu X, Sun W (2020). TNF-α polymorphisms might influence predisposition to periodontitis: A meta-analysis. Microbial Pathogenesis 143: 104113. https://doi.org/10.1016/j.micpath.2020.104113 [Google Scholar] [PubMed] [CrossRef]
Lin H, Chen H, Zhao X, Ding T, Wang Y, Chen Z, Tian Y, Zhang P, Shen Y (2022). Advances of exosomes in periodontitis treatment. Journal of Translational Medicine 20: 279. https://doi.org/10.1186/s12967-022-03487-4 [Google Scholar] [PubMed] [CrossRef]
Liu CM, Chen SH, Liao YW, Yu CH, Hsieh PL (2021). Magnolol ameliorates the accumulation of reactive oxidative stress and inflammation in diabetic periodontitis. Journal of the Formosan Medical Association 120: 1452–1458. https://doi.org/10.1016/j.jfma.2021.01.010 [Google Scholar] [PubMed] [CrossRef]
Liu X, Wang Y, Wu D, Li S, Wang C, Han Z, Wang J, Wang K, Yang Z, Wei Z (2019). Magnolol prevents acute alcoholic liver damage by activating PI3K/Nrf2/PPARγ and inhibiting NLRP3 signaling pathway. Frontiers in Pharmacology 10: 1459. https://doi.org/10.3389/fphar.2019.01459 [Google Scholar] [PubMed] [CrossRef]
Liu J, Wang X, Zheng M, Luan Q (2018). Lipopolysaccharide from Porphyromonas gingivalis promotes autophagy of human gingival fibroblasts through the PI3K/Akt/mTOR signaling pathway. Life Science 211: 133–139. https://doi.org/10.1016/j.lfs.2018.09.023 [Google Scholar] [PubMed] [CrossRef]
Lousa I, Reis F, Santos-Silva A, Belo L (2022). The signaling pathway of TNF receptors: Linking animal models of renal disease to human CKD. International Journal of Molecular Sciences 23: 3284. https://doi.org/10.3390/ijms23063284 [Google Scholar] [PubMed] [CrossRef]
Lu SH, Hsu WL, Chen TH, Chou TC (2015). Activation of Nrf2/HO-1signaling pathway involves the anti-inflammatory activity of magnolol in Porphyromonas gingivalis lipopolysaccharide-stimulated mouse RAW 264.7 macrophages. International Immunopharmacology 29: 770–778. https://doi.org/10.1016/j.intimp.2015.08.042 [Google Scholar] [PubMed] [CrossRef]
Lu SH, Huang RY, Chou TC (2013). Magnolol ameliorates ligature-induced periodontitis in rats and osteoclastogenesis: In vivo and in vitro study. Evidence-Based Complementary and Alternative Medicine 2013: 634095. https://doi.org/10.1155/2013/634095 [Google Scholar] [PubMed] [CrossRef]
Marilyn S, Irina D, Justin A, Naomi R, Tsippi IS, Michael S, Noam N, Iris B, Tirza D, Hagit K (2010). GeneCards version 3: The human gene integrator. Database 2010: baq020. https://doi.org/10.1093/database/baq020 [Google Scholar] [PubMed] [CrossRef]
McCubrey J, Yang L, Abrams S, Steelman L, Follo M, Cocco L, Ratti S, Martelli A, Augello G, Cervello MJC (2022). Effects of TP53 mutations and miRs on immune responses in the tumor microenvironment important in pancreatic cancer progression. Cells 11: 2155. https://doi.org/10.3390/cells11142155 [Google Scholar] [PubMed] [CrossRef]
Piero J, Ramírez-Anguita J, Saüch-Pitarch J, Ronzano F, Furlong LI (2019). The DisGeNET knowledge platform for disease genomics: 2019 update. Nucleic Acids Research 48: D845–D855. https://doi.org/10.1093/nar/gkz1021 [Google Scholar] [PubMed] [CrossRef]
Pinto K, Ferreira C, Guimarães A, Lima C, Pires F, Sassone L, Silva E (2020). Effects of alcohol and nicotine consumption on the development of apical periodontitis in rats: A correlative micro-computed tomographic, histological and immunohistochemical study. International Endodontic Journal 53: 1238–1252. https://doi.org/10.1111/iej.13344 [Google Scholar] [PubMed] [CrossRef]
Pussinen PJ, Kopra E, Pietiäinen M, Lehto M, Zaric S, Paju S, Salminen A (2022). Periodontitis and cardiometabolic disorders: The role of lipopolysaccharide and endotoxemia. Periodontol 89: 19–40. https://doi.org/10.1111/prd.12433 [Google Scholar] [PubMed] [CrossRef]
Sanz M, Marco Del Castillo A, Jepsen S, Gonzalez-Juanatey J, D’Aiuto F et al. (2020). Periodontitis and cardiovascular diseases: Consensus report. Journal of Clinical Periodontology 47: 268–288. https://doi.org/10.1111/jcpe.13189 [Google Scholar] [PubMed] [CrossRef]
Scab C, Sc A, Qw A, Tc A, Sl B (2019). A network pharmacology approach to investigate the anti-inflammatory mechanism of effective ingredients from Salvia miltiorrhiza. International Immunopharmacology 81: 106040. https://doi.org/10.1016/j.intimp.2019.106040 [Google Scholar] [PubMed] [CrossRef]
Sczepanik F, Grossi M, Casati M, Goldberg M, Glogauer M, Fine N, Tenenbaum HJP (2020). Periodontitis is an inflammatory disease of oxidative stress: We should treat it that way. Periodontol 84: 45–68. https://doi.org/10.1111/prd.12342 [Google Scholar] [PubMed] [CrossRef]
Seeliger D, Groot BL (2013). Ligand docking and binding site analysis with PyMOL and Autodock/Vina. Computer-Aided Molecular Design 24: 417–422. https://doi.org/10.1007/s10822-010-9352-6 [Google Scholar] [PubMed] [CrossRef]
Shao LI, Zhang B (2013). Traditional Chinese medicine network pharmacology: Theory, methodology and application. Chinese Journal of Natural Medicines 11: 110–120. https://doi.org/10.1016/S1875-5364(13)60037-0 [Google Scholar] [PubMed] [CrossRef]
Shen J, Ma H, Zhang T, Liu H, Yu L, Li G, Li H, Hu M (2017). Magnolol inhibits the growth of non-small cell lung cancer via inhibiting microtubule polymerization. Cellular Physiology Biochemistry 42: 1789–1801. https://doi.org/10.1159/000479458 [Google Scholar] [PubMed] [CrossRef]
Taalab MR, Mahmoud SA, Moslemany RME, Abdelaziz DM (2021). Intrapocket application of tea tree oil gel in the treatment of stage 2 periodontitis. BMC Oral Health 21: 239. https://doi.org/10.1186/s12903-021-01588-y [Google Scholar] [PubMed] [CrossRef]
Wang F, Long S, Zhang J (2021). Moringa oleifera Lam. leaf extract safely inhibits periodontitis by regulating the expression of p38α/MAPK14-OPG/RANKL. Archives of Oral Biology 132: 105280. https://doi.org/10.1016/j.archoralbio.2021.105280 [Google Scholar] [PubMed] [CrossRef]
Wang X, Wang ZY, Zheng JH, Shao LI (2021). TCM network pharmacology: A new trend towards combining computational, experimental and clinical approaches. Chinese Journal of Natural Medicines 19: 1–11. https://doi.org/10.1016/S1875-5364(21)60001-8 [Google Scholar] [PubMed] [CrossRef]
Wang L, Zheng J, Pathak JL, Chen Y, Liang D et al. (2020). SLIT2 overexpression in periodontitis intensifies inflammation and alveolar bone loss, possibly via the activation of MAPK pathway. Frontiers in Cell and Developmental Biology 8: 593. https://doi.org/10.3389/fcell.2020.00593 [Google Scholar] [PubMed] [CrossRef]
Weeks BS (2009). Formulations of dietary supplements and herbal extracts for relaxation and anxiolytic action: Relarian. Medical Science Monitor 15: Ra256–62. [Google Scholar] [PubMed]
Wu T, Yue R, He M (2020). A network pharmacology-based strategy for predicting active ingredients and potential targets of shuilu erxian dan in treating diabetic kidney disease. Journal of Ethnopharmacology 219: 359–368. https://doi.org/10.21203/rs.3.rs-54579/v1 [Google Scholar] [CrossRef]
Xiong WC, Wu HZ, Xiong YY, Liu B, Xie ZT, Wu ST, Yao YF, Yang YF (2020). Network pharmacology-based research of active components of albiziae flos and mechanisms of its antidepressant effect. Dangdai Yixue Kexue 40: 7. https://doi.org/10.1007/s11596-020-2155-7 [Google Scholar] [PubMed] [CrossRef]
Xu W, Chen X, Wang Y, Fan B, Guo K, Yang C, Yu S, Pang Y, Zhang S (2020). Chitooligosaccharide inhibits RANKL-induced osteoclastogenesis and ligation-induced periodontitis by suppressing MAPK/c-fos/NFATC1 signaling. Journal of Cellular Physiology 235: 3022–3032. https://doi.org/10.1002/jcp.29207 [Google Scholar] [PubMed] [CrossRef]
Yan D, Zheng G, Wang C, Chen Z, Mao T et al. (2021). HIT 2.0: An enhanced platform for herbal ingredients’ targets. Nucleic Acids Research 50: D1238–D1243. https://doi.org/10.1093/nar/gkab1011 [Google Scholar] [PubMed] [CrossRef]
Yang TC, Zhang SW, Sun LN, Wang H, Ren AM (2008). Magnolol attenuates sepsis-induced gastrointestinal dysmotility in rats by modulating inflammatory mediators. World Journal of Gastroenterology 14: 7353–7360. https://doi.org/10.3748/wjg.14.7353 [Google Scholar] [PubMed] [CrossRef]
Ying S, Tan M, Feng G, Kuang Y, Chen D, Li J, Song JJT (2020). Low-intensity Pulsed Ultrasound regulates alveolar bone homeostasis in experimental Periodontitis by diminishing Oxidative Stress. Theranostics 10: 9789–9807. https://doi.org/10.7150/thno.42508 [Google Scholar] [PubMed] [CrossRef]
Yu G, Wang LG, Han Y, He QY (2012). clusterProfiler: An R package for comparing biological themes among gene clusters. Omics: A Journal of Integrative Biology 16: 284–287. https://doi.org/10.1089/omi.2011.0118 [Google Scholar] [PubMed] [CrossRef]
Yuan C, Wang MH, Wang F, Chen PY, Wu HZ (2021). Network pharmacology and molecular docking reveal the mechanism of Scopoletin against non-small cell lung cancer. Life Sciences 270: 119105. https://doi.org/10.1016/j.lfs.2021.119105 [Google Scholar] [PubMed] [CrossRef]
Zhang Y, Yao Y, Fu Y, Yuan Z, Wu H (2021). Inhibition effect of oxyepiberberine isolated from coptis chinensis franch. On non-small cell lung cancer based on a network pharmacology approach and experimental validation. Journal of Ethnopharmacology 278: 114267. https://doi.org/10.1016/j.jep.2021.114267 [Google Scholar] [PubMed] [CrossRef]
Zhu M, Li B, Ma H, Huang X, Wu CZ (2019). Synthesis and in vitro antitumor evaluation of honokiol derivatives. Bioorganic Medicinal Chemistry Letters 30: 126849. https://doi.org/10.1016/j.bmcl.2019.126849 [Google Scholar] [PubMed] [CrossRef]
Cite This Article
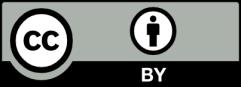