Open Access
ARTICLE
Prognosis and immunological role of HLA-DMA in lung adenocarcinoma
1 Department of Imaging, The Second Affiliated Hospital of Soochow University, Suzhou, 215000, China
2 Department of Imaging, Dongtai People’s Hospital, Dongtai, 224000, China
3 Institute of Imaging Medicine, Soochow University, Suzhou, 215000, China
4 Department of Orthopedics, Dongtai People’s Hospital, Dongtai, 224000, China
5 Department of Orthopedics, The Second Affiliated Hospital of Soochow University, Suzhou, 215000, China
6 Department of Pathology, The Third People’s Hospital of Nantong, Nantong, 226001, China
* Corresponding Authors: JUNKANG SHEN. Email: ; JUN YAN. Email:
# These authors contributed equally to this work
(This article belongs to the Special Issue: Bioinformatics Study of Diseases)
BIOCELL 2023, 47(6), 1279-1292. https://doi.org/10.32604/biocell.2023.027553
Received 03 November 2022; Accepted 16 February 2023; Issue published 19 May 2023
Abstract
Background: HLA-DMA presents pathogen-derived antigens to CD4+ and CD8+ T cells, respectively, and plays a significant part in initiating the immune response. So far, the impact of HLA expression on the prognosis of BC cells is controversial, because few studies have shown that the expressions of some HLA genes are related to the improvement of the survival rate. Up till now, however, the relationship between HLA-DMA and LUAD has not yet been assessed. Methods: We analyzed the TCGA database and assessed the prognostic value of HLA-DMA in LUAD. We conducted the Kruskal–Wallis and Wilcoxon signed-rank test and utilized logistic regression to assess the role of clinical-pathologic characteristics and HLA-DMA expression. Kaplan–Meier method and the multivariate and univariate Cox regression were also used for evaluating the prognosis-related factors of LUAD. GSEA was used to identify HLA-DMA-related key pathways. The ssGSEA of the TCGA data was used to investigate the correlations between HLA-DMA and cancer immune cell infiltration. Results: Low HLA-DMA expression was related to poorer disease-specific survival (DSS) and overall survival (OS) of LUAD patients. GSEA revealed that HLA-DMA was tightly interrelated with an immune response by the reactome activation of anterior hox genes in hindbrain development during the early embryogenesis signaling pathway. The expression of HLA-DMA was positively associated with cytotoxic cell infiltration and negatively related to the Th2 cell infiltration according to the ssGSEA. Western blotting and the CCK-8 assay showed that KD-HLA-DMA could significantly increase the proliferation of A549 cells and significantly reduce cell pyroptosis. Conclusion: All the observations implied that HLA-DMA was associated with patient prognosis and immune infiltration in LUAD.Keywords
Abbreviations
HLA-DMA | Major Histocompatibility Complex, Class II, DM α |
LUAD | Lung adenocarcinoma |
TCGA | The Cancer Genome Atlas |
ssGSEA | Single-sample gene set enrichment analysis |
GSEA | Gene set enrichment analysis |
SCLC | Small cell lung cancer |
NSCLC | Non-small cell lung cancer |
LCC | Large cell carcinoma |
LUSC | Squamous cell carcinoma |
CLIPs | Class II-related invariant chain peptides |
BP | Biological process |
MF | Molecular function |
CC | Cellular component |
PPI | Protein-protein interaction |
OS | Overall survival |
DSS | Disease-specific survival |
NES | Normalized enrichment scores |
CI | Confidence interval |
HR | Hazard risk |
ROC | Receiver operating characteristic |
AUC | Area under the curve |
KO | Knocking down |
KI | Knocking in |
Lung cancer has the largest figure in terms of incidence rate and mortality across the world. It is divided in light of tumor histology into two major categories: small-cell lung cancer (SCLC) and non-small cell lung cancer (NSCLC). NSCLC is further subdivided into several histological subtypes including lung adenocarcinoma (LUAD), large cell carcinoma (LCC), lung squamous cell carcinoma (LUSC), and lung adenosquamous carcinoma (de Bruin et al., 2014; Gillette et al., 2020; Zhu et al., 2020). LUAD is the most aggressive lung cancer with complex mechanisms, strong invasiveness, and poor prognosis (Shih et al., 2020; Zhang et al., 2020; Seguin et al., 2022). LUAD is the most common subtype of NSCLC (about 50%) and occurs in distant airways (Xie et al., 2020). Despite promising new therapies such as targeted therapies and immunotherapy, LUAD remains a major problem due to late diagnosis and the acquired or innate resistance of these tumors to anticancer treatment with a 5-year rate of survival of 15% (Zito Marino et al., 2019; Xu et al., 2020). Therefore, the study of effective prognostic biomarkers is a key to numerous considerations in the research on LUAD.
HLA-DMA belongs to the HLA class II α parachain homolog. This class II molecule is composed of an α (DMA) chain and a β chain (DMB), both anchored in the membrane. Class II molecules are present on antigen-presenting cells (APCs) (macrophages, dendritic cells [DCs], and B lymphocytes). The DMA gene contains five exons. Exon 1 encodes a precursor peptide, exon 2 and exon 3 encode two extracellular domains, and exon 4 encodes a transmembrane domain and a cytoplasmic tail (Baleeiro et al., 2022; He et al., 2022). DM plays a key role in catalyzing the release of class II-related invariant chain peptides (CLIPs) from newly synthesized MHC class II molecules and releasing peptide-binding sites to obtain antigenic peptides. In B cells, HLA-DO regulates the correlation between HLA-DM and MHC class II molecules. HLA genes have been shown to present pathogen-derived antigens to CD8+ and CD4+ T cells to act as a key role in the initiation of the immune response (Guce et al., 2013; Antohe et al., 2021; Kawazu et al., 2022). So far, the influence of HLA expression on the prognosis of BC cells is still controversial, Some researchers have suggested that HLA genes are related to the improvement of survival rate (Pos et al., 2012; McCaw et al., 2019), while others have not found such a relation (Shiina et al., 2009; Roudier et al., 2022). Few studies have found that HLA-DRA and HLA-DMA are significantly related to the improvement of Estrogen receptor (ER)-negative BC relapse-free survival (Liu and Hofman, 2022). Nevertheless, the role of HLA-DMA in LUAD has not been reported yet.
Processing and collecting data
TCGA database was our data source for obtaining RNA-Seq data, including immune system cell infiltration, gene expression, and comparative patient clinical information of 535 LUAD patients and 59 normal tissues in LUAD (https://portal.gdc.cancer.gov/). RNAseq data were first converted to the TPM format, all the data were retained and further analyzed according to the method provided by TCGA.
Both GSEA and GO functional enrichment analysis were expressed in R (3.6.3) by the Cluster Profifiler package (Zhu et al., 2021). The low and high expression levels of HLA-DMA differentially expressed genes (DEGs) were chosen to be researched. Biological process (BP), molecular function (MF), and cellular component (CC) were parts of the Gene Ontology (GO) analysis. GSEA was used to determine whether a set of genes defined by a priori is statistically significant and whether there is a consistent difference between two biological states. In addition, the enriched pathways within each phenotype were ranked using the normalized enrichment score (NES) and adjusted p-values (Subramanian et al., 2005).
Differentially expressed gene analysis
According to the median HLA-DMA expression level, the data were classified into low-expression groups and high-expression groups. Further analysis was then carried out through the t-test of unpaired students in the DESeq2 R package (3.6.3) (Love et al., 2014). The adjusted p < 0.05 and |log2-fold change (FC)| >1.5 were taken for new DEG thresholds.
Protein-protein interaction network analysis
The search tool for searching interacting gene database was (http://string-db.org) (Szklarczyk et al., 2019) protein-protein interactions (PPI) network for exploring co-regulated DEG and visualizing the role interactions of proteins with Cytoscape software (version 3.7.2). A comprehensive point of each pair of protein relationships in the database is dispersed in 0 and 1 and a PPI relationship with a higher score is more reliable.
The GSVA package (Hänzelmann et al., 2013) recognizes ssGSEA in R to value the relationship between HLA-DMA and 24 characteristic genes of immune cells and followed by a systematic analysis of immune filtrates of HLA-DMA in the published literature. Wilcoxon rank sum and Spearman correlation test was used to detect the immune cell infiltration between the HLA-DMA low-expression group and the high-expression group.
The Kaplan Meier (K-M) plotter could evaluate the value of 54 K Gene on the survival rate of 21 tumor types (http://kmplot.com/analysis/index.php?p=service&cancer=liver_rnaseq). Resource databases included TCGA, European Genome-phenome Archive (EGA), and Gene Expression Omnibus (GEO). The fundamental aim of the tool is to discover and verify the survival biomarkers based on a meta-analysis. The HLA-DMA was plotted in a K-M plotter to test its role on the survival (in days) of LUAD patients expressed by a K-M survival map and HLA-DMA expression A p-value < 0.05 was considered statistically significant.
BEAS-2B, 16HBE (human bronchial epithelial cell lines), A549, H1299, H292, and H358 cells (lung adenocarcinoma cell lines) (provided by the Cell Bank of the Chinese Academy of Sciences, Shanghai, China). Cells were seeded on 6-well plates, dishes, or flasks in Dulbecco’s minimal essential medium (DMEM) containing 10% FBS (at 37°C and 5% CO2) (provided by Gibco, USA).
A549 cells were transfected with HLA-DMA overexpression plasmid (provided by Brain Case, Shenzheng, China) and HLA-DMA small interfering RNA (siRNA) plasmid (provided by Brain Case, Shenzheng, China). The expression of HLA-DMA was then checked by western blot analysis. According to the manufacturer’s recommendations, cells were transiently transfected with plasmid DNA using Lipofectamine 8000 (provided by Beyotime, Shanghai, China). After 48 h of transfection, the cells were used for subsequent experiments.
The samples were homogenized in lysis buffer (50 mmol/L Tris,1% sodium deoxycholate, 1% NP-40, 5 mmol/L EDTA, 1% Triton X-100, pH 7.5, 1 mg/ml leupeptin, 1% sodium dodecyl sulfate [SDS], 10 mg/ml aprotinin, and 1 mmol/L phenylmethylsulfonyl fluoride [PMSF]) and centrifuged at 4°C for 20 min for separation. After the protein concentration was determined by Bradford assay (Bio-Rad), the supernatant (50 mg protein) was subjected to SDS polyacrylamide gel electrophoresis (PAGE). Proteins were transferred to a polyvinylidene fluoride (PVDF) membrane (Millipore) for 2 h at 300 mA through the transfer device. The membrane was blocked with 5% bovine serum albumin (BSA) and incubated subsequently with primary antibodies against HLA-DMA (mouse, 1:500; Santa Cruz), P-ERK (mouse, 1:500; Santa Cruz), CyclinD1 (mouse, 1:500; Santa Cruz), and GAPDH (rabbit, 1:1000; Abcam). After incubation with a secondary antibody conjugated with antimouse or antirabbit horseradish peroxidase, the proteins were assessed with an enhanced chemiluminescence system (ECL, Pierce Company, USA).
All sections were sealed with 0.3% Triton X-100, 10% donkey serum, and 1% (w/v) bovine serum albumin (BSA) at room temperature (RT) for 2 h, incubated with anti-HLA-DMA antibody (anti-mouse, 1:100 Santa Cruz) at 4°C overnight, and then incubated in biotinylated secondary antibodies (Vector Laboratories, Burlingame, CA, USA). The staining was observed with 3, 3′-diaminobenzidine (DAB) (Vector Laboratories). Cells with strong or moderate brown staining were counted as positive, cells without staining were counted as negative, and cells with weak staining were scored respectively.
Cell migration ability was checked after transfection. The transfected cells (2 × 105 cells/well cell density) were inoculated into each well of the 6-well plate and incubated till 100% confluence. Cells were scratched with the tip of the pipette and fresh-serum-free medium was added. After 12 h, the scratched cells were observed and imaged.
According to the manufacturer’s instructions, the commercial cell counting kit-8 (CCK)-8 (Dojindo, Kumamoto, Japan) was used to measure cell proliferation. In short, the cells (2 × 104 cells/well cell density) were seeded into 96 well plates and cultured overnight. The CCK-8 reagent was added to the well, incubated at 37°C for 2 h and the absorbance at 490 nm was read in the automatic plate reader. The experiment was repeated more than three times.
The statistical data obtained from TCGA was analyzed with R 3.6.3. Wilcoxon signed rank sum test and Wilcoxon rank sum test were used to compare the differences in HLA-DMA expression levels. Welch one-way Analysis of variance (ANOVA) was used, and then Bonferroni correction or t-test was used to examine the relationship between the expression of HLA-DMA and the grade of clinical-pathological factors. Normal and corrected Pearson κ, Fisher exact test, and univariate logistic regression were used to check the role of clinical pathological factors on HLA-DMA expression. In addition, multivariate and univariate Cox regression analyses were utilized to detect the impact of HLA-DMA on overall survival (OS) and clinical prognosis. All kinds of univariate analysis were included in multivariate analysis. The prognostic value of HLA-DMA was evaluated by plotting the K-M curve. Univariate Cox proportions were used to estimate the hazard ratio (HR) of individual disease-specific survival (DSS) and OS. The HR of individual elements was measured in a 95% confidence interval (CI). PROC package was used to analyze the ROC of HLA-DMA. The calculated value of the AUC ranged from 0.5 to 1.0, indicating that the recognition ability was 50%–100%. To investigate the role of HLA-DMA in the prognosis of LUAD for the 1, 3, and 5 year-OS, a time-dependent analysis of ROC curves was carried out. When the p-value was a two-tailed p ≤ 0.05, all statistical tests above were regarded as significant.
Differences in HLA-DMA expression in different groups
This study involved 535 LUAD patients, including 249 males and 286 females, the clinical data of whom consisted of N (spread to lymph nodes) stage, M (metastasis) stage, T (size of the tumor) stage, pathological stage, OS event, age, and gender (Table 1). The chi-square test showed that HLA-DMA was closely related to the T stage (p = 0.015) and the Fisher’s exact test revealed that HLA-DMA was closely related to gender (p = 0.003). A trend related to OS events was also discerned (p = 0.166). HLA-DMA was closely related to age (p < 0.001) in the Wilcoxon rank-sum test. The HLA-DMA expression was not so closely related to other clinicopathologic features.
Differential expression analysis of HLA-DMA in lung adenocarcinoma
We analyzed the HLA-DMA-related genes in the TCGA dataset and found that 4760 DEGs (86 were upregulated and 4674 were downregulated) were differentially expressed genes (DEGs) between cancer and normal patients, taking adjusted p < 0.05 and |logFC| > 1.5 as the cut-off standard. The DEG expression is shown in a volcano plot (Fig. 1A). The analysis of paired and unpaired differential expression between LUAD and normal groups revealed that the expression of HLA-DMA in normal tissues was much higher than that in the tumor group (Figs. 1B and 1C). A heat map was used to demonstrate the correlation between LUAD and 25 genes (Fig. 1D).
Figure 1: Differences in HLA-DMA expression and differentially expressed genes (DEGs). (A) The differentially expressed RNAs have been shown by volcanic plot (B, C) of HLA-DMA expression between the normal and lung adenocarcinoma (LUAD) groups. (D) 25 genes related to HLA-DMA were displayed by a heat map. “***” indicates p < 0.001.
Functional enrichment analysis of differentially expressed genes
Through GO analysis, we found that DEG-related HLA-DMA tightly regulated Systemic lupus erythematosus, alcoholism, DNA packaging complex, nucleosome, pre-mRNA 5′-splice site binding, protein-DNA complex, SL addition, via spliceosome, mRNA trans-splicing, formation of quadruple SL/U4/U5/U6 snRNP, and pre-mRNA 5′-splice site binding (Fig. 2A). The HLA-DMA network and its underlying LUAD co-expressed genes-related DEGs are also shown (Fig. 2B). The GSEA analysis indicated that the reactome activation of anterior hox genes in hindbrain development during early embryogenesis was negatively related to the high HLA-DMA expression (Fig. 2C). Further, the reactome amyloid fiber formation was also negatively related to high HLA-DMA expression (Fig. 2D). Reactome amyloid fiber formation and Reactome activation of anterior hox genes in hindbrain development during early embryogenesis were negatively related with high HLA-DMA expression (Fig. 2E).
Figure 2: HLA-DMA in lung adenocarcinoma (LUADC) was shown by Enrichment analysis. (A) Enrichment of biological processes correlated to HLA-DMA-related genes. (B) HLA-DMA and its network of 20 potential synergistic proteins. (C–E) Enrichment analysis results from the gene set enrichment analysis (GSEA).
Correlation of HLA-DMA with infiltration of different immune cells
To probe the relationship between the level of immune cell infiltration and the expression level of HLA-DMA in TPM format (quantized by the ssGSEA score). HLA-DMA expression was evidently negatively associated with the level of Th2 cell infiltration (Spearman R = −0.331, p < 0.001) (Fig. 3A) and was much lower in the group with high HLA-DMA expression (p < 0.001) (Fig. 3B). Meanwhile, cytotoxic cell infiltration was positively related to the expression of HLA-DMA (Spearman R = 0.442, p < 0.001) (Fig. 3C). This infiltration was higher in the group with high expression of HLA-DMA (p < 0.001) (Fig. 3D). Cytotoxic cells, aDC, DC, Eosinophils, B cells, Macrophages, CD8 T cells, iDC, Neutrophils, Mast cells, NK CD56dim cells, NK CD56bright cells, T cells, pDC, NK cells, T helper (Th) cells, Treg, Th1 cells, Th17 cells, and TFH also documented a positive relationship with HLA-DMA. However, Th2 cells and Tgd exhibited a poor association with HLA-DMA (Fig. 3E). This analysis revealed an important usefulness of HLA-DMA in LUAD immune infiltration. A heat map (Fig. 3F) displaying varying degrees of relationship of the 24 different tumor-infiltrating immune cell subsets has been presented.
Figure 3: Analysis results of HLA-DMA expression and immune infiltration. (A) HLA-DMA expression was negatively correlated with T helper (Th)2 cells. (B) Low and high HLA-DMA expression groups have different levels of Th2 cell infiltration. (C) The expression of HLADMA was obviously related to cytotoxic cell infiltration. (D) The level of cytotoxic cell infiltration in HLA-DMA low and high expression groups. (E) Relation between the comparatively enriched 24 immune cell types and the expression level of HLA-DMA. (F) Infiltration of immune cells (24 types) in lung adenocarcinoma (LUAD) was displayed by the heat map. “*” indicates p < 0.05, “***” indicates p < 0.001.
Associations between the expression of HLA-DMA and clinicopathologic variables
Bonferroni correction and Welch one-way ANOVA revealed that the expression of HLA-DMA was closely related to the OS event, pathologic stage, T stage, and smoker status (Figs. 4A–4D). Logistic regression analysis revealed that HLA-DMA was closely related to the histologic grade (p < 0.013), smoker status (p < 0.001), T stage (p = 0.002), and gender (p = 0.002). Further, HLA-DMA approached a correlating value with the histologic grade (p = 0.083) (Table 2).
Figure 4: Relations among different clinicopathological features and HLADMA expression. (A) The relation between the expression of HLA-DMA and the overall survival (OS) (B), the pathological stage, (C) smoker status, and (D) the T stage of lung adenocarcinoma (LUAD). “ns” indicates p ≥ 0.05; “*” indicates p < 0.05; “**” indicates p < 0.01; “***” indicates p < 0.001.
By univariate Cox regression analysis, we detected that the N stage (p < 0.001), T stage (p < 0.001), M stage (p = 0.006), and the pathologic stage (p < 0.001) were related to the poor prognosis of LUAD in the Cox regression model (Table 3). The N stage (p < 0.001) and T stage (p = 0.004) were important prognostic elements for OS as analyzed by multivariate Cox regression (Fig. 5A).
Figure 5: Prognostic value of HLA-DMA in lung adenocarcinoma (LUAD). (A) The forest plot for visualizing the multivariate Cox regression results. (B) Diagnostic receiver operating characteristic curve (ROC) curve of HLA-DMA. (C) Time-dependent receiver operating characteristic curve (ROC) curve of HLA-DMA.
The ROC analysis score of HLA-DMA supports the accuracy of diagnosis (95% CI: 0.717–0.789, AUC = 0.757) (Fig. 5B). A time-dependent ROC analysis was conducted to assess the time-dependent correctness of HLA-DMA to assess the 1, 3, and 5-year---OS values (Fig. 5C).
HLA-DMA is a good prognostic factor for lung adenocarcinoma
The prognostic value of HLA-DMA in the OS of LUAD was evaluated by the K-M survival curve. According to the HLA-DMA expression median value, we divided LUAD patients into two different expression groups. The high expression groups had a close association with better OS (HR = 0.67 (0.50–0.90), p = 0.008), T1, T2 subgroups of the T stage (HR = 0.59 (0.42–0.81), p = 0.001), the N0 subgroup of the N stage (HR = 0.49 (0.32–0.75), p = 0.001) and the “No” subgroup of “Smoker” (HR = 0.29 (0.12–0.71), p = 0.007) (Figs. 6A, 6C, 6D, 6F). The high expression of LUAD was related to a better DSS (HR = 0.63 (0.43–0.90), p = 0.013) and OS in the stage I subgroup of the pathologic stage (HR = 0.56 (0.35–0.92), p = 0.021) (Figs. 6B and 6E).
Figure 6: HLA-DMA expression in different subgroups has different prognostic values. (A, B) Effect of HLA-DMA on disease-specific survival (DSS) and overall survival (OS) of lung adenocarcinoma (LUAD). (C–G) Low HLA-DMA expression was related to a poor OS in these groups.
Experimental validation in cell lines
To investigate the role of HLA-DMA in LUAD, we used BEAS-2B, 16HBE (human bronchial epithelial cell lines), A549, H1299, H292, and H358 cells (lung adenocarcinoma cell lines). We used western blotting to check the expression of HLA-DMA in the cell lines. The results showed that the expression of HLA-DMA in lung adenocarcinoma cell lines was less than in human bronchial epithelial cell lines (Fig. 7A). We then did in vitro transfection of an HLA-DMA overexpression plasmid or HLA-DMA small interfering RNA (siRNA) plasmid into A549 cells. Western blotting was used to check the expression of HLA-DMA, cyclin D1, and P-ERK. The results showed that knocking down HLA-DMA markedly reduced the level of HLA-DMA expression compared with the control, and knocking in HLA-DMA obviously increased the level of HLA-DMA expression compared with the control. To further confirm the relationship between HLA-DMA and cell proliferation, we detected the protein expression of P-ERK, and cyclin D1 by Western blotting. The result showed that knockdown or KD-HLA-DMA markedly increased the expression of P-ERK, and cyclin D1 expression compared with control. Further, overexpression of HLA-DMA decreased the level of P-ERK and cyclin D1 expression compared with the control (Fig. 7B). We also used the CCK-8 assay to check the cell viability of A549 cells transfected with the HLA-DMA overexpression plasmid or HLA-DMA small interfering RNA (siRNA) plasmids. Cells transfected with the HLA-DMA overexpression plasmid revealed significantly diminished proliferation compared with the control and HLA-DMA small interfering RNA (siRNA) plasmids (Fig. 7C). To further scrutinize the effect of HLA-DMA in the migration process of LUAD, a wound healing assay was performed. It showed that overexpression of HLA-DMA could effectively inhibit the migration of LUAD (Fig. 7D). To identify the expression and distribution changes of HLA-DMA in normal and LUAD cells, we used an anti-HLA-DMA mouse monoclonal antibody to perform immunohistochemistry. The results showed that the expression of HLA-DMA was obviously higher in normal tissues than in LUAD (Fig. 7E).
Figure 7: The role of HLA-DMA in lung adenocarcinoma (LUAD). (A) The expression of HLA-DMA in human bronchial epithelial cell lines and lung adenocarcinoma cell lines. (B) The expression of HLA-DMA, P-ERK, and cyclin D1 in the control, KD-HLA-DMA, and OverHLADMA groups. (C) The Cell Counting Kit (CCK)-8 assay detected the cell viability of A549 cells transfected with HLA-DMA overexpression plasmid or HLADMA small interfering RNA (siRNA) plasmids. (D) The wound healing assay tested the cell migration capacity of A549 cells treated with HLA-DMA overexpression plasmid or HLADMA small interfering RNA (siRNA) plasmids. (E) Immunohistochemistry detected the distribution and expression changes of HLA-DMA in normal and LUAD tissues. “*” indicates p < 0.05; “**” indicates p < 0.01; “***” indicates p < 0.001.
To determine the molecular mechanisms of the three functional properties of HLA-DM, a lot of efforts have been made. These include the instability of all MHCII peptide complexes, including the stability of “empty” MHCII grooves, those formed with CLIP, and the editing of peptide libraries (Pos et al., 2012). DM has a domain organization similar to MHCII protein, which can only temporarily bind to the substrate before inducing transformation and releasing products. Therefore, DM cannot bind stably to MHC-peptide complexes (Gillette et al., 2020). DM did not seem to bind to the recombinant peptide-free empty MHC molecules (Santambrogio, 2022), although it is reported that DM binds to the empty MHC molecule produced in the normal cell environment (Guce et al., 2013). DM could induce any peptide to dissociate from the MHCII protein and had a profound impact on the peptide library presented by the MHCII protein to CD4 T cells (Shiina et al., 2009; Roudier et al., 2022). The low pH environment of the peptide loading chamber also promoted peptide exchange, while the neutral pH on the cell surface significantly slowed down peptide exchange. This editing mechanism is the key factor of advantage to the immune cells (Pos et al., 2012). Based on previous research, we speculate that lowering the expression of HLA-DM would increase immune infiltration in cancer progression and occurrence.
There is a positive relation between DC, NK CD56dim cells, Cytotoxic cells, aDC, Eosinophils, pDC, B cells, Macrophages, CD8 T cells, iDC, Mast cells, Neutrophils, NK CD56bright cells, T cells, NK cells, Th17 cells, Th1 cells, T helper cells, TFH, and Treg cells with HLA-DMA. Cytotoxic cells play a vital part in antitumor immunity as in the innate immune surveillance of tumors, NK cells play a prominent role (Linette and Carreno, 2022). NK cells are effectors of the innate immune system and have a significant impact on the prognosis and immunotherapy of cancer. The extent of NK cell infiltration was shown to be closely related to the prognosis of patients with various solid tumors (Villegas et al., 2002). Some studies have explored the role of NK cell marker genes in LUAD through scRNA-seq analysis. For example, a research team successfully developed a new prognostic prediction feature for LUAD patients based on the NK cell marker genes in the TCGA database, which was also verified (Song et al., 2022). DCs are considered professional antigen-presenting cells, capable of carrying out strong immune responses to tumor cells (Constantino et al., 2017; An et al., 2022) constructed a DC-related risk model by studying the DC-related gene spectrum, prognosis, and gene mutation of patients with bladder urothelial carcinoma (BLCA), and documented its effective performance in predicting the prognosis of immunotherapy. The extent of NK cell infiltration is also closely related to the prognosis of patients with various solid tumors. DCs and plasmacytoid dendritic cells (pDCs) play vital roles in cancer immune defense, and the relationship between Th17 cells and neutrophils is also very close (Amicarella et al., 2017). Interferon (IFN)-I has a good antitumor activity and was shown to be produced by pDCs (Saulep-Easton et al., 2014). pDCs play an important role in predicting the poor prognosis of LUAD and tumor immunity. If these types of immune cells are downregulated, it may promote the progress of LUAD. According to the net results of the ssGSEA, HLA-DMA plays an important role in regulating LUAD immune infiltration. The tumor markers of lung adenocarcinoma mainly include CEA, CA125, CA153, and CA724, etc. (Nakamura and Nishimura, 2017; Li et al., 2022). However, CEA was not only documented in LUAD but also in other cancers. At present, there is no reliable serum tumor marker with high specificity for the diagnosis of LUAD. This study shows that HLA-DMA has a low expression in LUAD. Moreover, compared with the higher-level HLA-DMA expression group, LUAD patients with low HLA-DMA resulted in poor DSS and OS. HLA-DMA was also closely related to the OS in LUAD as checked by the Cox HR model. The relationship between these prognostic indicators and HLA-DMA levels suggested that HLA-DMA may be a potential prognostic biomarker in LUAD. To further investigate the significance of HLA-DMA in lung adenocarcinoma, we did a series of in vitro experiments with A549 cells. The results showed that overexpressing HLA-DMA could significantly decrease the proliferation of A549cells and evidently reduce cell migration. Meanwhile, immunohistochemistry demonstrated that the expression of HLA-DMA was significantly higher in normal tissues than in LUAD tissues.
To summarize, the study proved the significance of HLA-DMA in LUAD. It was indicated that the lowered expression of HLA-DMA was related to poor OS in LUAD patients in our dataset. GSEA also revealed that mechanisms like reactome amyloid fiber formation and reactome activation of anterior hox genes in hindbrain development during early embryogenesis were correlated with the HLA-DMA expression. Additionally, we also identified the relationship between HLA-DMA and tumor-infiltrating immune cells. Further studies on the role of HLA-DMA in the development of LUAD will help to discover the potential mechanism of LUAD. On similar lines to previous research, our study shows that HLA-DMA plays a critical part in immune infiltration in LUAD. Although the role of HLA-DMA in LUAD tumorigenesis and development had been revealed, more experiments are necessary to test the relationship between HLA-DMA expression and LUAD development. In order to better study the role of HLA-DMA in LUAD, we need more clinical studies, which might also help us to propel drug development, evaluate tumor progress, and improve treatment strategies.
Funding Statement: This study was supported by grants from the National Natural Science Foundation of China (Grant No. 81971036). This study was also supported by China Medical and Health Development Foundation (Grant Nos. C202212-006; C202212-0014).
Author Contributions: The authors confirm contribution to the paper as follows: study conception and design: CHEN CHEN, QIN YU. Data collection: HAI-YAN ZHANG. Analysis and interpretation of results: JUN-KANG SHEN. Draft manuscript preparation: JIN CHEN, JUN YAN. All authors reviewed the results and approved the final version of the manuscript.
Availability of Data and Materials: The datasets generated during and/or analysed during the current study are available from the corresponding author on reasonable request.
Ethics Approval: This research project has been approved by the Ethics Committee of the Second Affiliated Hospital of Soochow University (approval no. JD-HG-2022-69). All participants have signed the informed consent form and are informed of relevant precautions. In addition, they have the right to withdraw and request the destruction of their data.
Conflicts of Interest: All authors declare that there is no conflict of interest in this study.
References
Amicarella F, Muraro M, Hirt C, Cremonesi E, Padovan E et al. (2017). Dual role of tumour-infiltrating T helper 17 cells in human colorectal cancer. Gut 66: 692–704. https://doi.org/10.1136/gutjnl-2015-310016 [Google Scholar] [PubMed] [CrossRef]
An B, Guo Z, Wang J, Zhang C, Zhang G, Yan L (2022). Derivation and external validation of dendritic cell-related gene signatures for predicting prognosis and immunotherapy efficacy in bladder urothelial carcinoma. Frontiers in Immunology 13: 1080947. https://doi.org/10.3389/fimmu.2022.1080947 [Google Scholar] [PubMed] [CrossRef]
Antohe I, Tanasa M, Dăscălescu A, Dănăilă C, Titieanu A, Zlei M, Ivanov I, Sireteanu A, Cianga P (2021). The MHC-II antigen presentation machinery and B7 checkpoint ligands display distinctive patterns correlated with acute myeloid leukaemias blast cells HLA-DR expression. Immunobiology 226: 152049. https://doi.org/10.1016/j.imbio.2020.152049 [Google Scholar] [PubMed] [CrossRef]
Baleeiro R, Bouwens C, Liu P, di Gioia C, Dunmall L et al. (2022). MHC class II molecules on pancreatic cancer cells indicate a potential for neo-antigen-based immunotherapy. Oncoimmunology 11: 2080329. [Google Scholar] [PubMed]
Constantino J, Gomes C, Falcao A, Neves BM, Cruz MT (2017). Dendritic cell-based immunotherapy: A basic review and recent advances. Immunologic Research 65: 798–810. https://doi.org/10.1007/s12026-017-8931-1 [Google Scholar] [PubMed] [CrossRef]
de Bruin E, McGranahan N, Mitter R, Salm M, Wedge D et al. (2014). Spatial and temporal diversity in genomic instability processes defines lung cancer evolution. Science 346: 251–256. https://doi.org/10.1126/science.1253462 [Google Scholar] [PubMed] [CrossRef]
Gillette M, Satpathy S, Cao S, Dhanasekaran S, Vasaikar S et al. (2020). Proteogenomic characterization reveals therapeutic vulnerabilities in lung adenocarcinoma. Cell 182: 200–225.e235. https://doi.org/10.1016/j.cell.2020.06.013 [Google Scholar] [PubMed] [CrossRef]
Guce A, Mortimer S, Yoon T, Painter C, Jiang W, Mellins ED, Stern LJ (2013). HLA-DO acts as a substrate mimic to inhibit HLA-DM by a competitive mechanism. Nature Structural & Molecular Biology 20: 90–98. https://doi.org/10.1038/nsmb.2460 [Google Scholar] [PubMed] [CrossRef]
He J, Chen J, Han X, Gu Q, Liang J, Sun M, Liu S, Yao Y, Shi L (2022). Association of HLA-DM and HLA class II genes with antibody response induced by inactivated Japanese encephalitis vaccine. HLA 99: 357–367. https://doi.org/10.1111/tan.14575 [Google Scholar] [PubMed] [CrossRef]
Hänzelmann S, Castelo R, Guinney J (2013). GSVA: Gene set variation analysis for microarray and RNA-seq data. BMC Bioinformatics 14: 7. https://doi.org/10.1186/1471-2105-14-7 [Google Scholar] [PubMed] [CrossRef]
Kawazu M, Ueno T, Saeki K, Sax N, Togashi Y et al. (2022). HLA class I analysis provides insight into the genetic and epigenetic background of immune evasion in colorectal cancer with high microsatellite instability. Gastroenterology 162: 799–812. https://doi.org/10.1053/j.gastro.2021.10.010 [Google Scholar] [PubMed] [CrossRef]
Li G, Zhang H, Zhang L, Liu H, Li S, Wang Y, Deng X, Khan NR (2022). Serum markers CA125, CA153, and CEA along with inflammatory cytokines in the early detection of lung cancer in high-risk populations. BioMed Research International 2022: 1394042–1394044. https://doi.org/10.1155/2022/1394042 [Google Scholar] [PubMed] [CrossRef]
Linette G, Carreno B (2022). On the twentieth anniversary of dendritic cell vaccines-riding the next wave. Cancer Research 82: 966–968. https://doi.org/10.1158/0008-5472.CAN-21-4440 [Google Scholar] [PubMed] [CrossRef]
Liu D, Hofman P (2022). Expression of NOTCH1, NOTCH4, HLA-DMA and HLA-DRA is synergistically associated with T cell exclusion, immune checkpoint blockade efficacy and recurrence risk in ER-negative breast cancer. Cellular Oncology 45: 463–477. https://doi.org/10.1007/s13402-022-00677-6 [Google Scholar] [PubMed] [CrossRef]
Love M, Huber W, Anders S (2014). Moderated estimation of fold change and dispersion for RNA-seq data with DESeq2. Genome Biology 15: 550. [Google Scholar] [PubMed]
McCaw T, Li M, Starenki D, Cooper S, Liu M, Meza-Perez S, Arend RC, Buchsbaum DJ, Forero A, Randall TD (2019). The expression of MHC class II molecules on murine breast tumors delays T-cell exhaustion, expands the T-cell repertoire, and slows tumor growth. Cancer Immunology, Immunotherapy 68: 175–188. https://doi.org/10.1007/s00262-018-2262-5 [Google Scholar] [PubMed] [CrossRef]
Nakamura H, Nishimura T (2017). History, molecular features, and clinical importance of conventional serum biomarkers in lung cancer. Surgery Today 47: 1037–1059. https://doi.org/10.1007/s00595-017-1477-y [Google Scholar] [PubMed] [CrossRef]
Pos W, Sethi D, Call M, Schulze M, Anders A, Pyrdol J, Wucherpfennig KW (2012). Crystal structure of the HLA-DM-HLA-DR1 complex defines mechanisms for rapid peptide selection. Cell 151: 1557–1568. https://doi.org/10.1016/j.cell.2012.11.025 [Google Scholar] [PubMed] [CrossRef]
Roudier J, Balandraud N, Auger I (2022). How RA associated HLA-DR molecules contribute to the development of antibodies to citrullinated proteins: The hapten carrier model. Frontiers in Immunology 13: 930112. https://doi.org/10.3389/fimmu.2022.930112 [Google Scholar] [PubMed] [CrossRef]
Santambrogio L (2022). Molecular determinants regulating the plasticity of the MHC class II immunopeptidome. Frontiers in Immunology 13: 878271. https://doi.org/10.3389/fimmu.2022.878271 [Google Scholar] [PubMed] [CrossRef]
Saulep-Easton D, Vincent F, Le Page M, Wei A, Ting S, Croce CM, Tam C, Mackay F (2014). Cytokine-driven loss of plasmacytoid dendritic cell function in chronic lymphocytic leukemia. Leukemia 28: 2005–2015. https://doi.org/10.1038/leu.2014.105 [Google Scholar] [PubMed] [CrossRef]
Seguin L, Durandy M, Feral C (2022). Lung adenocarcinoma tumor origin: A guide for personalized medicine. Cancers 14: 1759. https://doi.org/10.3390/cancers14071759 [Google Scholar] [PubMed] [CrossRef]
Shih D, Nayyar N, Bihun I, Dagogo-Jack I, Gill C et al. (2020). Genomic characterization of human brain metastases identifies drivers of metastatic lung adenocarcinoma. Nature Genetics 52: 371–377. https://doi.org/10.1038/s41588-020-0592-7 [Google Scholar] [PubMed] [CrossRef]
Shiina T, Hosomichi K, Inoko H, Kulski J (2009). The HLA genomic loci map: Expression, interaction, diversity and disease. Journal of Human Genetics 54: 15–39. https://doi.org/10.1038/jhg.2008.5 [Google Scholar] [PubMed] [CrossRef]
Song P, Li W, Guo L, Ying J, Gao S, He J (2022). Identification and validation of a novel signature based on NK cell marker genes to predict prognosis and immunotherapy response in lung adenocarcinoma by integrated analysis of single-cell and bulk RNA-sequencing. Frontiers in Immunology 13: 850745. https://doi.org/10.3389/fimmu.2022.850745 [Google Scholar] [PubMed] [CrossRef]
Subramanian A, Tamayo P, Mootha V, Mukherjee S, Ebert B et al. (2005). Gene set enrichment analysis: A knowledge-based approach for interpreting genome-wide expression profiles. Proceedings of the National Academy of Sciences of the United States of America 102: 15545–15550. https://doi.org/10.1073/pnas.0506580102 [Google Scholar] [PubMed] [CrossRef]
Szklarczyk D, Gable A, Lyon D, Junge A, Wyder S et al. (2019). STRING v11: Protein-protein association networks with increased coverage, supporting functional discovery in genome-wide experimental datasets. Nucleic Acids Research 47: D607–D613. https://doi.org/10.1093/nar/gky1131 [Google Scholar] [PubMed] [CrossRef]
Villegas FR, Coca S, Villarrubia VG, Jimenez R, Chillon MJ, Jareño J, Zuil M, Callol L (2002). Prognostic significance of tumor infiltrating natural killer cells subset CD57 in patients with squamous cell lung cancer. Lung Cancer 35: 23–28. https://doi.org/10.1016/S0169-5002(01)00292-6 [Google Scholar] [PubMed] [CrossRef]
Xie T, Li Y, Ying J, Cai W, Li J, Lee KY, Ricciuti B, Pacheco J, Xing P (2020). Whole exome sequencing (WES) analysis of transformed small cell lung cancer (SCLC) from lung adenocarcinoma (LUAD). Translational Lung Cancer Research 9: 2428–2439. https://doi.org/10.21037/tlcr-20-1278 [Google Scholar] [PubMed] [CrossRef]
Xu J, Zhang C, Wang X, Zhai L, Ma Y et al. (2020). Integrative proteomic characterization of human lung adenocarcinoma. Cell 182: 245–261.e217. https://doi.org/10.1016/j.cell.2020.05.043 [Google Scholar] [PubMed] [CrossRef]
Zhang C, Zhang Z, Sun N, Zhang Z, Zhang G, Wang F, Luo Y, Che Y, He J (2020). Identification of a costimulatory molecule-based signature for predicting prognosis risk and immunotherapy response in patients with lung adenocarcinoma. Oncoimmunology 9: 1824641. https://doi.org/10.1080/2162402X.2020.1824641 [Google Scholar] [PubMed] [CrossRef]
Zhu E, Dai C, Xie H, Su H, Hu X et al. (2020). Lepidic component identifies a subgroup of lung adenocarcinoma with a distinctive prognosis: A multicenter propensity-matched analysis. Therapeutic Advances in Medical Oncology 12: 1758835920982845. https://doi.org/10.1177/1758835920982845 [Google Scholar] [PubMed] [CrossRef]
Zhu C, Xiao H, Jiang X, Tong R, Guan J (2021). Prognostic biomarker DDOST and its correlation with immune infiltrates in hepatocellular carcinoma. Frontiers in Genetics 12: 819520. https://doi.org/10.3389/fgene.2021.819520 [Google Scholar] [PubMed] [CrossRef]
Zito Marino F, Bianco R, Accardo M, Ronchi A, Cozzolino I, Morgillo F, Rossi G, Franco R (2019). Molecular heterogeneity in lung cancer: From mechanisms of origin to clinical implications. International Journal of Medical Sciences 16: 981–989. https://doi.org/10.7150/ijms.34739 [Google Scholar] [PubMed] [CrossRef]
Cite This Article
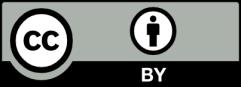
This work is licensed under a Creative Commons Attribution 4.0 International License , which permits unrestricted use, distribution, and reproduction in any medium, provided the original work is properly cited.