Open Access
ARTICLE
Prognostic prediction and expression validation of NSD3 in pan-cancer analyses
1 Translational Medicine Centre, Hunan Cancer Hospital, The Affiliated Cancer Hospital of Xiangya, School of Medicine, Central South University, Changsha, China
2 Hunan Provincial Clinical Research Centre for Oncoplastic Surgery, Hunan Cancer Hospital, The Affiliated Cancer Hospital of Xiangya, School of Medicine, Central South University, Changsha, China
3 School of Medicine, College of Medicine, Nursing and Health Science, National University of Ireland, Galway, Ireland
4 Central Laboratory, Hunan Cancer Hospital, The Affiliated Cancer Hospital of Xiangya, School of Medicine, Changsha, China
* Corresponding Authors: XIAO ZHOU. Email: ; YING LONG. Email:
# Sha Li and Yaqiong Liu contributed equally to this paper. They are co-first authors
BIOCELL 2023, 47(5), 1003-1019. https://doi.org/10.32604/biocell.2023.027209
Received 19 October 2022; Accepted 26 December 2022; Issue published 10 April 2023
Abstract
Background: Nuclear receptor binding SET domain protein-3 (NSD3) is a histone lysine methyltransferase and a crucial regulator of carcinogenesis in several cancers. We aimed to investigate the prognostic value and potential function of NSD3 in 33 types of human cancer. Methods: The data were obtained from The Cancer Genome Atlas. Kaplan-Meier analysis, CIBERSORT, gene set enrichment analysis, and gene set variation analysis were performed. The expression of NSD3 was measured using quantitative real-time polymerase chain reaction and western blot. Results: The expression of NSD3 was altered in pan-cancer samples. Patients with higher levels of NDS3 generally had shorter overall survival and disease-specific survival. Levels of NSD3 were positively correlated with DNA copy number variation (CNV) in pan-cancer. NSD3 expression was also associated with tumor mutation burden and microsatellite instability. The levels of immune-cell infiltration differed significantly between high and low NSD3 expression. NSD3 negatively correlated with levels of CD8+ T cells. Functional enrichment analysis showed that while NSD3 expression was positively associated with several immune cell-related and histone methylation-related pathways, it was negatively correlated with cell metabolism-related, drug transport-related, and drug metabolism-related pathways. NSD3 levels in the cell lines tested were significantly different. In U251 and NCI-H23 cells, silencing NSD3 inhibited cell proliferation and promoted apoptosis. Conclusions: NSD3 expression was changed in pan-cancer samples that was also verified in cell lines. NSD3 was associated with CNV and immune-cell infiltration. A poor prognosis was predicted in patients with high expression of NSD3. NSD3 might hence be a potential marker for predicting tumor prognosis.Keywords
Cancer is the leading cause of death worldwide, with its incidence on the rise (Sung et al., 2021). In recent years, despite advances that have been made in the diagnosis and treatment of cancer, there are still a large number of cancer patients who face difficulties in clinical treatment. Tumor heterogeneity is a difficult challenge to be solved in cancer treatment (McGranahan and Swanton, 2015; Dagogo-Jack and Shaw, 2018). Pan-cancer analysis studies could distinguish some mutual features or heterogeneity in key biological processes by exploring similarities and differences between genomic and cellular changes in many cancer types (Weinstein et al., 2013; Kinker et al., 2020; Zuo et al., 2020). Thus, pan-cancer analysis can help analyze and identify diagnostic and prognostic markers of cancer and provide some scientific clues for the individualized treatment of patients (Krishnamurthy et al., 2021; Yang et al., 2021).
Nuclear receptor binding SET domain protein-3 (NSD3), also known as WHSC1L1, is a member of the histone lysine methyltransferase family (Han et al., 2018). Studies have shown that NSD3 is involved in and regulates gene expression, cycle progression, and chromatin remodeling (Vougiouklakis et al., 2015; Shen et al., 2020). NSD3 has domains like the conserved sequence motif of Pro-Trp-Trp-Pro domains and plant homeodomain fingers. It plays an important role in chromatin-related biological processes by interacting with histones, DNA methylation readers, or modification proteins (Li et al., 2009; Husmann and Gozani, 2019; Davidovich and Zhang, 2021; Li et al., 2021). NSD3 recognizes and binds N-terminal peptides of histone H3 and can dimethylate or trimethylate histone H3 at lysine 36 (H3K36) (Li et al., 2009; Husmann and Gozani, 2019; Davidovich and Zhang, 2021; Li et al., 2021). Jeong et al. (2021) have shown that NSD3 could drive breast tumor initiation and metastatic progression by increasing H3K36 methylation by activating the notch signaling pathway. Aberrant NSD3 expression has been shown to be associated with many pathophysiological processes, including cardiac hypertrophy (Chen et al., 2019), epithelial-mesenchymal transition (EMT) (Jeong et al., 2021), and antiviral immune responses (Mino and Takeuchi, 2017). NSD3 has been characterized in recent years as a tumorigenesis-related gene (Voutsadakis, 2020; Li et al., 2021; Sun et al., 2021). Downregulation of NSD3 could inhibit the proliferation and migration of colorectal cancer cells (Yi et al., 2019). Mahmood et al. (2013) indicated that NSD3 is a driver of several cancers, including breast cancer, pancreatic adenocarcinoma, and lung cancer. However, previous studies have been limited to only specific cancer types.
This led us to hence obtain relevant data from The Cancer Genome Atlas (TCGA) database. NSD3 expression and its relationship with prognosis were evaluated in different types of cancers. The correlation was analyzed between NSD3 expression and microsatellite instability (MSI), tumor mutation burden (TMB), immune cell infiltration, and immune checkpoint molecules. This was aimed at further clarifying the role of NSD3 in tumor development and prognosis and providing a certain research basis for the individualized treatment of tumors.
Data downloading and processing
The batch effects normalized gene expression pan-cancer data, copy number variation (CNV), and clinical data of the patients were downloaded from the UCSC Xenadatabase (http://xena.ucsc.edu/). Strawberry Perl, version 5.32.1 (http://strawberryperl.com/), was used to extract the NSD3 expression data to generate a data matrix. Somatic mutations (VarScan2) of the pan-cancer set were collected from UCSC Xena.
Analysis of the expression pattern of nuclear receptor binding SET domain protein-3
The NSD3 expression was analyzed in 33 cancer types. Subsequently, patients with each cancer type were categorized into three or four groups according to the pathological stage, and the NSD3 expression pattern was then determined. To further investigate the cause of aberrant NSD3 in the different cancer types, correlations between expression levels and CNVs were evaluated. The R package “ggpubr” version 0.4.0 (https://www.rdocumentation.org/packages/ggpubr) was used to visualize the results.
Patient survival and pathological stage information were selected for prognosis analyses. NSD3 data was subjected to overall survival (OS) and disease-specific survival (DSS) analyses using the univariate Cox regression model. The OS and DSS analyses were performed using R packages “survival” version 2.43-3 (CRAN.R-project.org/package=survival) and “forestplot” version 1.10.1 (https://www.rdocumentation.org/packages/forestplot) (Durisová and Dedík, 1993). Patient survival was visualized using the “survminer” package, version 0.4.9 (https://www.rdocumentation.org/packages/survminer), and Kaplan-Meier analysis.
Correlation of nuclear receptor binding SET domain protein-3 expression with tumor mutation burden, tumor microsatellite instability, and immune checkpoint genes
TMB, MSI, and the expression of immune checkpoint genes are commonly used biomarkers that correlate with responses to immune checkpoint blockade (ICB) treatment (Cohen et al., 2019; Yamashita et al., 2019). In the current study, TMB scores were calculated using Perl script and normalized to the total length of the exons. MSI scores were calculated for each sample based on somatic mutation data downloaded from the TCGA (https://tcga.xenahubs.net). For all cases, MSI analysis with MANTIS (version 1.0.3) was performed as previously described (Bonneville et al., 2017). MANTIS was run with the whole-exome data. The microsatellite locus performance was assessed, and R (version 3.3.2) was used to compute the kernel density estimation functions. Correlations of NSD3 expression with TMB and MSI were subsequently analyzed by calculating Spearman’s rank correlation coefficient. The results are presented as radar charts prepared using the “fmsb” package, version 0.7.0 (https://www.rdocumentation.org/packages/fmsb). Co-expression of NSD3 with immune checkpoint genes was analyzed and the results were generated using the “pheatmap” package, version 1.0.12 (https://www.rdocumentation.org/packages/pheatmap).
Analysis of the relationship between nuclear receptor binding SET domain protein-3 and tumor microenvironment (TME)
ESTIMATE is an important tool for calculating immune and stromal scores using transcriptomic data. Here, we evaluated the immune and stromal scores for each sample using the “estimate” package and assessed the correlation between NSD3 expression and the scores for each cancer type. The online analytic tool CIBERSORT (https://cibersort.stanford.edu/) was used to evaluate the levels of infiltrating immunocytes in each tumor sample (Chen et al., 2018). The samples were stratified into two groups, high NSD3 expression and low, based on the median. Comparison of infiltrating immune cells between the two groups was investigated and visualized using “ggpubr”.
Functional enrichment analysis
Gene set enrichment analysis (GSEA) and gene set variation analysis (GSVA) are two different approaches that have been used to explore the biological functions of NSD3 in cancers (Damian and Gorfine, 2004; Hänzelmann et al., 2013). Data regarding the genes co-expressed with NSD3 were collected for each cancer type. Gene Ontology (GO) and Kyoto Expression of Genes and Genomes (KEGG) gene sets were downloaded from the GSEA website (http://www.gsea-msigdb.org/gsea/index.jsp). Functional enrichment analyses were conducted using the R packages “org.Hs.eg.db,” “clusterProfiler,” (Yu et al., 2012), and “enrichplot.” The gene sets for GSVA analysis were obtained from the Molecular Signatures Database (MSigDB) v7.2 (http://www.gsea-msigdb.org/gsea/msigdb/index.jsp), which was updated in September 2020. GSVA scores were calculated using the “GSVA” package. The correlation of NSD3 expression with signaling pathways was investigated. The top 15 most significant pathways with either positive or negative correlations have been presented.
Cell culture and siRNA experiments
The human microglia HMC3, normal lung epithelial cells BEAS-2B, and lung cancer cell lines (A549, NCI-H1650, and NCI-H23) were purchased from iCell Bioscience Inc. (Shanghai, China). The glioma cell line U251 was obtained from Procell Life Science & Technology Co., Ltd. (Wuhan, China) HMC3 cells were grown in Minimum Essential Medium. U251 and BEAS-2B were cultured in Dulbecco’s modified Eagle’s medium. A549 was grown in Ham’s F-12 medium. NCI-H1650 and NCI-H23 cells were cultured in RPMI-1640 medium. Cells were cultured in corresponding medium supplemented with 10% fetal bovine serum and 1% penicillin/streptomycin. All the cells were maintained in a humidified atmosphere of 5% CO2 at 37°C.
The si-NSD3 and negative control si-NC (HonorGene, Changsha, China) were transfected into the cells using Lipofectamine™3000 (Invitrogen, CA, USA). After 48 h, cells were collected and a follow-up experiment was performed.
Quantitative real-time polymerase chain reaction (qRT-PCR) analysis
Total RNA was extracted from cultured cells. cDNA was synthesized with the HiFiScript cDNA Synthesis Kit (CWBIO, Beijing, China), and PCR amplification of cDNA was carried out using UltraSYBR Mixture (Low ROX) (CWBIO, Beijing, China). The 2−ΔΔct method was performed to calculate the relative quantification. GAPDH was used as the internal control. The primer sequences were as follows: NSD3 F-GATTACAGAAAGGGTCGGCG, R-TCCACAGTTTCCTCAATCGCT, and GAPDH F-ACAGCCTCAAGATCATCAGC, R-GGTCATGAGTCCTTCCACGAT.
The total protein of cells was extracted with the Strong RIPA Lysis Buffer (Abiwell, Changsha, China). The BCA method was employed to analyze protein concentration. Proteins of different molecular masses were separated by 10% sodium dodecyl sulfate-polyacrylamide gel electrophoresis. Proteins were then transferred to a nitrocellulose membrane. The membranes were then blocked with 5% skim milk and incubated with primary antibodies and the secondary antibody. The primary antibodies were anti-NSD3 (11345-1-AP, Proteintech, Chicago, USA) and anti-β-actin (66009-1-Ig, Proteintech, Chicago, USA). The ECL Western Blotting Substrate (Abiwell, Changsha, China) was used to visualize the proteins. The chemiluminescence imaging system (ChemiScope 6000 Exp) was employed to capture images. β-actin was used as a control.
Cell counting kit-8 (CCK-8) assay
The cell proliferation rate was detected using the CCK-8 assay according to the manufacturer’s instructions. 5 × 103 cells were seeded into the wells and grown in a cell incubator at 37°C with 5% CO2. At 0, 24, and 48 h, 10 μL of CCK-8 solution (NU679, Dojindo, Kumamoto, Japan) was added and incubated with the cells. Proliferative activity was then detected.
Cell apoptosis was analyzed by the Annexin V-APC/ Propidium iodide (PI) apoptosis kit (KGA1030, KeyGENBioTECH, Nanjing, China) according to the manufacturer’s instructions. 5 μL of Annexin V-APC and 5 μL of PI were added and incubated with the cells for 10 min. The apoptosis rate was then measured using flow cytometry.
The Shapiro-Wilk normality test was used to check the normality of the variables. The unpaired Student’s t-test was performed to compare differences between the two groups with the normally distributed variables. The Wilcoxon test was conducted to compare the variables without normally distribution. The R package ggplot2 was adopted to visualize the data. A p-value < 0.05 was considered statistically significant.
Nuclear receptor binding SET domain protein-3 expression patterns across different cancer types
The expression pattern of NSD3 was investigated in different types of cancer using TCGA data. As shown in Fig. 1, the levels of NSD3 in various tumors were analyzed and ranked from high to low expression. NSD3 expression was the highest in acute myeloid leukemia (LAML) and the lowest in liver hepatocellular carcinoma (LIHC).
Figure 1: Nuclear receptor binding SET domain protein-3 (NSD3) expression levels across different cancer types.
Next, we assessed the 33 cancer types for differential expression of NSD3 based on the cancer stage. The following 10 cancers showed a correlation between NSD3 expression and the stage, including colon adenocarcinoma (COAD), head and neck squamous cell carcinoma (HNSC), kidney chromophobe (KICH), kidney renal clear cell carcinoma (KIRC), lung adenocarcinoma (LUAD), pancreatic adenocarcinoma (PAAD), skin cutaneous melanoma (SKCM), stomach adenocarcinoma (STAD), testicular germ cell tumors (TGCT), and thyroid carcinoma (THCA) (Figs. 2a–2j). Among them, the NSD3 expression was markedly different in stage I vs. stage II in COAD, HNSC, LUAD, PAAD, SKCM, STAD, and TGCT, vs. stage II of COAD, KICH, KIRC, LUAD and THCA, and that of stage III COAD and THCA. The expression differences among other stages were weak. While in KIRC and STAD, expression in stage I and stage II was different, in KICH, the expression was different in stage II and stage III. Thus, the expression of NSD3 was altered in some cancers with differences at different pathological stages. These observations suggested that differential expression of NSD3 might be weakly related to the pathological stage of cancer.
Figure 2: Relationship between nuclear receptor binding SET domain protein-3 (NSD3) expression and the pathological stage in different cancer types. (a) COAD, (b) HNSC, (c) KICH, (d) KIRC, (e) LUAD, (f) PAAD, (g) SKCM, (h) STAD, (i) TGCT, and (j) THCA.
Association of nuclear receptor binding SET domain protein-3 expression with cancer patient survival
We then performed OS and DSS analyses for each of the 33 cancer types. The NSD3 level was a high-risk factor in KICH, PAAD, or uveal melanoma (UVM) (Fig. 3a). Kaplan-Meier survival analysis demonstrated that high levels of NSD3 were associated with poor OS for KICH (Fig. 3b, p = 0.011), PAAD (Fig. 3d, p = 0.009), and UVM (Fig. 3e, p = 0.050) patients. Furthermore, our results indicated that NSD3 was a high-risk gene for DSS in patients with PAAD and UVM (Suppl. Fig. S1a). Kaplan-Meier curve analysis validated the predictive value of NSD3 for DSS in patient cohorts of PAAD (Suppl. Fig. S1b, p = 0.014) and UVM (Suppl. Fig. S1e, p = 0.044). These results indicated that high NDS3 expression might be associated with poor outcomes for patients with specific cancers.
Figure 3: Relationship between nuclear receptor binding SET domain protein-3 (NSD3) expression and overall survival (OS). (a) Forest plot of univariate Cox proportional hazard regression illustrating the impact of NSD3 expression on OS in 33 different cancer types. (b–e) Association between NSD3 mRNA levels and patient OS from the Kaplan-Meier plotter in KICH, LUSC, PAAD, and UVM.
Correlation of nuclear receptor binding SET domain protein-3 with copy number variations
Amplification of the chromosomal region 8p11–12, where NSD3 is located, is a common genetic alteration that has been implicated in the etiology of cancers (Turner-Ivey et al., 2017; Yuan et al., 2021). Accordingly, we explored the correlation between mRNA expression and CNVs of NSD3 across the 33 tumor types. Interestingly, a positive association was detected in breast invasive carcinoma (BRCA), cholangiocarcinoma (CHOL), COAD, esophageal carcinoma (ESCA), HNSC, LIHC, LUAD, lung squamous cell carcinoma (LUSC), rectum adenocarcinoma (READ), and STAD (Suppl. Fig. S2). This indicated that the high expression level of NSD3 in some cancers might be supported by DNA amplification.
Correlation of nuclear receptor binding SET domain protein-3 expression with tumor mutation burden, microsatellite instability, and immune checkpoint genes across cancer types
Next, we analyzed the correlations of NSD3 expression with TMB, MSI, and the expression of immune checkpoint genes. The results indicated that NSD3 expression was positively associated with TMB in adrenocortical carcinoma (ACC), uterine corpus endometrial carcinoma (UCEC), STAD, LUAD, LAML, and HNSC, and negatively associated with TMB in THCA, LIHC, kidney renal papillary cell carcinoma (KIRP), KIRC, and BRCA (Fig. 4a). NSD3 expression was related to MSI in the other types. Among them, NSD3 was positively associated with MSI in STAD, SARC, READ, LUSC, LUAD, and COAD, while the association was negative in SKCM and diffuse large B-cell lymphoma (DLBC) (Fig. 4b). These observations hinted that NSD3 might be related to TMB and MSI. The expression of immune checkpoint genes is one of the factors affecting ICB sensitivity. Pearson correlation coefficient analysis was performed between NSD3 expression and immune checkpoint genes across all 33 of the cancers (Fig. 4c). NSD3 showed an obvious association with most immune checkpoint genes in the majority cancers, suggesting that NSD3 might be involved in regulating the expression of the immune checkpoint genes.
Figure 4: Correlation of nuclear receptor binding SET domain protein-3 (NSD3) expression with tumor mutation burden (TMB), microsatellite instability (MSI), and immune-related genes. (a) The relationship between NSD3 expression and TMB in different cancer types. (b) The relationship between NSD3 expression and MSI in different cancer types. (c) The correlation between NSD3 expression and immune checkpoint genes (ICGs) in each cancer type. The upper left corner symbolizes the p-value and the lower right corner symbolizes the correlation coefficient. *p < 0.05, **p < 0.01, and ***p < 0.001.
Relationship between nuclear receptor binding SET domain protein-3expression and tumor microenvironment (TME)
TME is another essential factor that affects ICB sensitivity. Thus, the ESTIMATE algorithm was used to calculate immune and stromal scores for each tumor type and their relationships with NSD3 expression. Our results revealed that NSD3 expression negatively correlated with the immune scores of many cancer types, including urothelial bladder carcinoma (BLCA), BRCA, glioblastoma multiforme (GBM), KIRP, brain lower grade glioma (LGG), LUSC, ovarian serous cystadenocarcinoma (OV), pheochromocytoma and paraganglioma (PCPG), SARC, THCA, and UCEC (Fig. 5a). NSD3 expression also negatively correlated with stromal scores in pan-cancer analysis, except for positive correlations seen in prostate adenocarcinoma (PRAD) and KIRC (Fig. 5b). As infiltrating immune cells are an important component of the antitumor immune response, we consequently investigated the correlation between NSD3 expression and immune cells. As shown in Suppl. Fig. S3, NSD3 expression negatively correlated with the levels of CD8+ T cells, regulatory T cells (Tregs), and activated NK cells in BRCA and KIRC. NSD3 expression was positively correlated with the levels of M2 macrophages in GBM while it was and negative in BLCA, CESC, HNSC, and KIRP.
Figure 5: Association between nuclear receptor binding SET domain protein-3 (NSD3) expression and immune and stromal scores. (a) The correlation coefficients between NSD3 expression and immune scores in BLCA, BRCA, GBM, KIRP, LGG, LUSC, OV, PCPG, SARC, THCA, and UCEC. (b) Relationship between NSD3 expression and stromal scores in BLCA, GBM, KIRC, LGG, LUSC, PRAD, and SARC.
Functional annotation of nuclear receptor binding SET domain protein-3
GSEA and GSVA algorithms were performed for the functional enrichment analyses with NSD3-related genes. Enrichment analyses with KEGG pathways and GO terms for each cancer type have been shown in Fig. 6. NSD3 was associated with immune-related and glucose metabolism pathways, including antigen processing and presentation, RIG-I-like receptor signaling pathways, TOLL-like receptor signaling pathways, and pentose and glucuronate interconversions in many cancers (Fig. 6a). NSD3-related genes were mainly enriched in GO terms associated with artery morphogenesis, cell cycle, cell motility, immune response, and inflammatory response (Fig. 6b). GSVA analysis was adopted to determine the potential function of NSD3. As shown in Suppl. Fig. S4, NSD3 expression was positively associated with several immune cell-related and histone methylation-related pathways. In contrast, NSD3 expression was negatively correlated with cell metabolism-related, drug transport-related, and drug metabolism-related pathways. These results hinted at the possibility that NSD3 might influence cancer development by participating in or regulating specific signaling and metabolic pathways in cancer cells.
Figure 6: GSEA of nuclear receptor binding SET domain protein-3 (NSD3)-related genes. (a, b) Kyoto Encyclopedia of Genes and Genomes and Gene Ontology enrichment analysis of NSD3-related genes in CHOL, GBM, HNSC, KIRP, LGG, OV, PCPG, READ, and SARC.
Validation in nuclear receptor binding SET domain protein-3 expression in cell lines
Next, we examined NSD3 expression in the following cell lines: human microglia HMC3, normal lung epithelial cells BEAS-2B, and lung cancer cell lines (A549, NCI-H1650, and NCI-H23). The NSD3 levels were dysregulated at both the mRNA and protein levels (Fig. 7). As shown in Fig. 7a, NSD3 levels in the U251 cell line were higher than in the HMC3 lines. Compared with the BEAS-2B, NSD3 expression decreased in the A549 and NCI-H1650 lines, while it increased in NCI-H23 (Fig. 7b).
Figure 7: Validation of nuclear receptor binding SET domain protein-3 (NSD3) expression (n = 3). (a) The gene and protein levels of NSD3 in the human microglia cell line HMC3 and the glioma cell line U251. (b) The expression of NSD3 was detected in human normal lung epithelial cells (BEAS-2B) and lung cancer cell lines (A549, NCI-H1650, and NCI-H23). **p < 0.01, ***p < 0.001, and ****p < 0.0001.
Effects of nuclear receptor binding SET domain protein-3 on proliferation and apoptosis of U251 and NCI-H23 cells
Based on the above results (shown in Fig. 7), U251 and NCI-H23 cells were selected for further experimental analysis. The expression of NSD3 decreased in the si-NSD3 test samples compared with that in the si-NC (Fig. 8a). After the silencing of NSD3, the proliferative activity of U251 and NCI-H23 cells decreased (Fig. 8b). The apoptosis rate in the si-NSD3 group was higher than the si-NC group (Fig. 8c). This indicates that silencing NSD3 could inhibit the proliferation of U251 and NCI-H23 cells while promoting apoptosis.
Figure 8: Effects of nuclear receptor binding SET domain protein-3 (NSD3) on proliferation and apoptosis of U251 and NCI-H23 cells (n = 3). (a) The mRNA levels of NSD3 in the U251 and NCI-H23 cells. The proliferation (b) and apoptosis (c) profiles in U251 and NCI-H23 cells were detected after silencing NSD3. **p < 0.01, ***p < 0.001, and ****p < 0.0001.
NSD3, located on the 8p11-p12 locus has been considered a candidate oncogene as per reports (Yang et al., 2010; Turner-Ivey et al., 2017; Yuan et al., 2021). While accumulating evidence has indicated that NSD3 plays a vital role in tumorigenesis, metastasis, and treatment sensitivity in various cancer types (Yang et al., 2010; Jeong et al., 2021), a comprehensive pan-cancer analysis of NSD3 expression was absent. Therefore, we investigated the possible role of NSD3 in pan-cancer samples by evaluating the expression pattern, prognostic value, and potential function of NSD3. Moreover, the NSD3 expression was measured in multiple cell lines where the NSD3 level was significantly different in lung cancer cell lines: A549, NCI-H1650, and NCI-H23. We speculated that the reason for this result might be the complex internal mechanism of the cell type. Several studies have also found similar results, with significantly different gene expression in different cell lines, including non-small-cell lung cancer (Su et al., 2022), BLCA (Xu et al., 2021; Lu et al., 2022), and gastric cancer (Dai et al., 2021). Proliferation and apoptosis rates were also assessed and altered by the silencing of NSD3. The observations suggested that NSD3 could regulate cell proliferation and apoptosis in some cancers.
In the present study, we analyzed 33 different cancer types and found that NSD3 expression was markedly different across different cancers. In line with previous studies, NSD3 expression was upregulated in LUSC, ESCA, LIHC, and HNSC (Kang et al., 2013; Bourguignon et al., 2017; Voutsadakis, 2020). Further, the results of the Kaplan-Meier survival analysis showed that high levels of NSD3 indicated a poor prognosis for patients with PAAD and UVM. Previous studies also confirmed our results laterally (Mahmood et al., 2013; Katoh, 2016). All these observations suggested that NSD3 might be involved in the patient prognosis of our pan-cancer dataset.
CNVs exist widely in the human genome, leading to the altered expression of different genes (Trost et al., 2018). Multiple CNVs might lead to the heterogeneity of genome and molecular phenotype to affect the occurrence and development of complex diseases, including cancer (Qiu et al., 2018; Zheng et al., 2020). CNVs are a common source of genetic variation. Our correlation analysis showed that the expression of NSD3 was positively correlated with CNVs in LUSC, BRCA, and ESCA. These results suggest that the associated CNVs of NSD3 in these cancers might be one of the factors affecting the development of these malignancies. Growing evidence supports TMB and MSI as promising analytical markers for predicting ICB drug response in multiple solid tumors (Chan et al., 2019; Liu et al., 2019; Lin et al., 2020). Our current results also revealed the correlation of NSD3 with TMB and MSI levels. There was a significant correlation between NSD3 expression and immune checkpoint genes in different cancer types. Infiltrating immune cells also play vital roles in the immune response and escape during tumorigenesis and progression (Gajewski et al., 2013). Accordingly, NSD3 expression showed a negative correlation with the immune score and the level of infiltration of diverse immune cells, such as CD8+ T cells, which are important immune cells among tumor-infiltrating lymphocytes. Additionally, CD8+ T cells are the most powerful effectors in anti-cancer immune response and the pillars of cancer immunotherapy (Raskov et al., 2021). CD8+ T cells can infiltrate into the immunosuppressive tumor microenvironment and eliminate cancer cells (Hossain et al., 2021). A study found that the CD8+ T cell score could be used as a prognostic marker of triple-negative breast cancer, and a higher CD8+ T cell score associated with better survival (Oshi et al., 2020). Kim et al. (2021) have shown that NSD3 could affect cancer prognosis by reducing the number of CD8+ T cells. These results hinted at the probability that NSD3 might promote tumor development by suppressing the number of immune cells.
To further investigate the potential roles of NSD3 in several cancers, we performed functional enrichment analyses. We found that NSD3-related genes were mainly enriched in pathways that are dysregulated during the pathophysiological process of cancers, including pathways related to the immune cells, cell metabolism, cell cycle, cell motility, drug transport, and drug metabolism. A previous study indicated that NSD3 upregulation inhibits the Notch signaling pathway and represses breast cancer cell EMT by facilitating H3K36 methylation (Jeong et al., 2021). In addition, NSD3 knockdown in lung cancer and bladder cancer cells induced cell cycle arrest at the G(2)/M phase (Kang et al., 2013). Results from all these studies support the findings of our current study. Interestingly, while a study revealed that NSD3 promoted innate immunity during viral infection (Wang et al., 2017), no other study has reported the role of NSD3 in the regulation of cancer immunity yet. Our results strongly suggest that NSD3 exerted immune regulatory effects in cancers, but this indication needs to be verified with future experimental tests and evidence. Moreover, due to time and financial constraints, the expression of NSD3 in clinical samples would be identified in our future work. The effects of NSD3 on cell migration and invasion also need further analysis.
In conclusion, we evaluated the expression profile, prognostic value, and potential function of NSD3 in a pan-cancer analysis and verified the expression of NSD3 in cell lines. Silencing NSD3 could inhibit the proliferation of U251 and NCI-H23 cells while promoting apoptosis. Our results indicated that NSD3 might be a promising biomarker for predicting patient outcomes. These observations would further help to explore and confirm NSD3 functions in cancer development and progression to help identify new therapeutic approaches.
Acknowledgement: The authors are grateful for the support of TCGA. The authors also thank the preprint service provided by Research Square: https://assets.researchsquare.com/files/rs-591803/v1/e75e37e4-947d-48a4-9d41-ad75bb514c33.pdf?c=1631884716.
Funding Statement: The present study was supported in part by the National Natural Science Foundation of China (Grant No. 81703005), the Natural Science Foundation of Hunan Province (Grant Nos. 2017JJ3195 and 2018JJ3317), and the Key Research and Development Projects of Hunan Province (Grant No. 2018SK2120).
Author Contributions: The authors confirm contribution to the paper as follows: study conception and design: Y Long, SL, Y Liu, and XZ; data collection: SL, Y Liu, HLZ, CY, and XZ; analysis and interpretation of results: SL, Y Liu, YG, and XS; draft manuscript preparation: SL, Y Liu, and Y Long. All authors reviewed the results and approved the final version of the manuscript. SL and Y Liu contributed equally to this paper.
Availability of Data and Materials: The datasets generated during the current study are available from the corresponding author on reasonable request.
Ethics Approval: Not applicable.
Conflicts of Interest: The authors declare they have no conflicts of interest to report regarding the present study.
References
Bonneville R, Krook MA, Kautto EA, Miya J, Wing MR, Chen HZ, Reeser JW, Yu L, Roychowdhury S (2017). Landscape of microsatellite instability across 39 cancer types. JCO Precision Oncology 5: 1–15. https://doi.org/10.1200/PO.17.00073 [Google Scholar] [PubMed] [CrossRef]
Bourguignon LYW, Earle C, Shiina M (2017). Activation of matrix hyaluronan-mediated CD44 signaling, epigenetic regulation and chemoresistance in head and neck cancer stem cells. International Journal of Molecular Sciences 18: 1849. https://doi.org/10.3390/ijms18091849 [Google Scholar] [PubMed] [CrossRef]
Chan TA, Yarchoan M, Jaffee E, Swanton C, Quezada SA, Stenzinger A, Peters S (2019). Development of tumor mutation burden as an immunotherapy biomarker: Utility for the oncology clinic. Annals of Oncology 30: 44–56. https://doi.org/10.1093/annonc/mdy495 [Google Scholar] [PubMed] [CrossRef]
Chen K, Jian D, Zhao L, Zang X, Song W, Ma J, Jia Z, Wang X, Gao C (2019). Protective effect of histone methyltransferase NSD3 on ISO-induced cardiac hypertrophy. FEBS Letters 593: 2556–2565. https://doi.org/10.1002/1873-3468.13515 [Google Scholar] [PubMed] [CrossRef]
Chen B, Khodadoust MS, Liu CL, Newman AM, Alizadeh AA (2018). Profiling tumor infiltrating immune cells with CIBERSORT. Methods in Molecular Biology 1711: 243–259. https://doi.org/10.1007/978-1-4939-7493-1 [Google Scholar] [CrossRef]
Cohen R, Hain E, Buhard O, Guilloux A, Bardier A et al. (2019). Association of primary resistance to immune checkpoint inhibitors in metastatic colorectal cancer with misdiagnosis of microsatellite instability or mismatch repair deficiency status. JAMA Oncology 5: 551–555. https://doi.org/10.1001/jamaoncol.2018.4942 [Google Scholar] [PubMed] [CrossRef]
Dagogo-Jack I, Shaw AT (2018). Tumour heterogeneity and resistance to cancer therapies. Nature Reviews Clinical Oncology 15: 81–94. https://doi.org/10.1038/nrclinonc.2017.166 [Google Scholar] [PubMed] [CrossRef]
Dai W, Xiao Y, Tang W, Li J, Hong L et al. (2021). Identification of an EMT-related gene signature for predicting overall survival in gastric cancer. Frontiers in Genetics 12: 661306. https://doi.org/10.3389/fgene.2021.661306 [Google Scholar] [PubMed] [CrossRef]
Damian D, Gorfine M (2004). Statistical concerns about the GSEA procedure. Nature Genetics 36: 663. https://doi.org/10.1038/ng0704-663a [Google Scholar] [PubMed] [CrossRef]
Davidovich C, Zhang Q (2021). Allosteric regulation of histone lysine methyltransferases: From context-specific regulation to selective drugs. Biochemical Society Transactions 49: 591–607. https://doi.org/10.1042/BST20200238 [Google Scholar] [PubMed] [CrossRef]
Durisová M, Dedík L (1993). SURVIVAL—an integrated software package for survival curve estimation and statistical comparison of survival rates of two groups of patients or experimental animals. Methods and Findings in Experimental and Clinical Pharmacology 15: 535–540. [Google Scholar] [PubMed]
Gajewski TF, Schreiber H, Fu YX (2013). Innate and adaptive immune cells in the tumor microenvironment. Nature Immunology 14: 1014–1022. https://doi.org/10.1038/ni.2703 [Google Scholar] [PubMed] [CrossRef]
Han X, Piao L, Zhuang Q, Yuan X, Liu Z, He X (2018). The role of histone lysine methyltransferase NSD3 in cancer. OncoTargets and Therapy 11: 3847–3852. https://doi.org/10.2147/OTT [Google Scholar] [CrossRef]
Hossain MA, Liu G, Dai B, Si Y, Yang Q, Wazir J, Birnbaumer L, Yang Y (2021). Reinvigorating exhausted CD8(+) cytotoxic T lymphocytes in the tumor microenvironment and current strategies in cancer immunotherapy. Medicinal Research Reviews 41: 156–201. https://doi.org/10.1002/med.21727 [Google Scholar] [PubMed] [CrossRef]
Husmann D, Gozani O (2019). Histone lysine methyltransferases in biology and disease. Nature Structural & Molecular Biology 26: 880–889. https://doi.org/10.1038/s41594-019-0298-7 [Google Scholar] [PubMed] [CrossRef]
Hänzelmann S, Castelo R, Guinney J (2013). GSVA: Gene set variation analysis for microarray and RNA-seq data. BMC Bioinformatics 14: 7. https://doi.org/10.1186/1471-2105-14-7 [Google Scholar] [PubMed] [CrossRef]
Jeong GY, Park MK, Choi HJ, An HW, Park YU et al. (2021). NSD3-induced methylation of H3K36 activates NOTCH signaling to drive breast tumor initiation and metastatic progression. Cancer Research 81: 77–90. https://doi.org/10.1158/0008-5472.CAN-20-0360 [Google Scholar] [PubMed] [CrossRef]
Kang D, Cho HS, Toyokawa G, Kogure M, Yamane Y et al. (2013). The histone methyltransferase Wolf-Hirschhorn syndrome candidate 1-like 1 (WHSC1L1) is involved in human carcinogenesis. Genes, Chromosomes & Cancer 52: 126–139. https://doi.org/10.1002/gcc.22012 [Google Scholar] [PubMed] [CrossRef]
Katoh M (2016). Mutation spectra of histone methyltransferases with canonical SET domains and EZH2-targeted therapy. Epigenomics 8: 285–305. https://doi.org/10.2217/epi.15.89 [Google Scholar] [PubMed] [CrossRef]
Kim HS, Min KW, Kim DH, Son BK, Kwon MJ, Hong SM (2021). High WHSC1L1 expression reduces survival rates in operated breast cancer patients with decreased CD8+ T cells: Machine learning approach. Journal of Personalized Medicine 11: 636. https://doi.org/10.3390/jpm11070636 [Google Scholar] [PubMed] [CrossRef]
Kinker GS, Greenwald AC, Tal R, Orlova Z, Cuoco MS et al. (2020). Pan-cancer single-cell RNA-seq identifies recurring programs of cellular heterogeneity. Nature Genetics 52: 1208–1218. https://doi.org/10.1038/s41588-020-00726-6 [Google Scholar] [PubMed] [CrossRef]
Krishnamurthy N, Goodman AM, Barkauskas DA, Kurzrock R (2021). STK11 alterations in the pan-cancer setting: Prognostic and therapeutic implications. European Journal of Cancer 148: 215–229. https://doi.org/10.1016/j.ejca.2021.01.050 [Google Scholar] [PubMed] [CrossRef]
Li W, Tian W, Yuan G, Deng P, Sengupta D et al. (2021). Molecular basis of nucleosomal H3K36 methylation by NSD methyltransferases. Nature 590: 498–503. https://doi.org/10.1038/s41586-020-03069-8 [Google Scholar] [PubMed] [CrossRef]
Li Y, Trojer P, Xu CF, Cheung P, Kuo A et al. (2009). The target of the NSD family of histone lysine methyltransferases depends on the nature of the substrate. Journal of Biological Chemistry 284: 34283–34295. https://doi.org/10.1074/jbc.M109.034462 [Google Scholar] [PubMed] [CrossRef]
Lin A, Zhang J, Luo P (2020). Crosstalk between the MSI status and tumor microenvironment in colorectal cancer. Frontiers in Immunology 11: 2039. https://doi.org/10.3389/fimmu.2020.02039 [Google Scholar] [PubMed] [CrossRef]
Liu L, Bai X, Wang J, Tang XR, Wu DH et al. (2019). Combination of TMB and CNA stratifies prognostic and predictive responses to immunotherapy across metastatic cancer. Clinical Cancer Research 25: 7413–7423. https://doi.org/10.1158/1078-0432.CCR-19-0558 [Google Scholar] [PubMed] [CrossRef]
Lu H, Wu J, Liang L, Wang X, Cai H (2022). Identifying a novel defined pyroptosis-associated long noncoding RNA signature contributes to predicting prognosis and tumor microenvironment of bladder cancer. Frontiers in Immunology 13: 803355. https://doi.org/10.3389/fimmu.2022.803355 [Google Scholar] [PubMed] [CrossRef]
Mahmood SF, Gruel N, Nicolle R, Chapeaublanc E, Delattre O, Radvanyi F, Bernard-Pierrot I (2013). PPAPDC1B and WHSC1L1 are common drivers of the 8p11-12 amplicon, not only in breast tumors but also in pancreatic adenocarcinomas and lung tumors. American Journal of Pathology 183: 1634–1644. https://doi.org/10.1016/j.ajpath.2013.07.028 [Google Scholar] [PubMed] [CrossRef]
McGranahan N, Swanton C (2015). Biological and therapeutic impact of intratumor heterogeneity in cancer evolution. Cancer Cell 27: 15–26. https://doi.org/10.1016/j.ccell.2014.12.001 [Google Scholar] [PubMed] [CrossRef]
Mino T, Takeuchi O (2017). NSD3 keeps IRF3 active. Journal of Experimental Medicine 214: 3475–3476. https://doi.org/10.1084/jem.20171980 [Google Scholar] [PubMed] [CrossRef]
Oshi M, Asaoka M, Tokumaru Y, Yan L, Matsuyama R, Ishikawa T, Endo I, Takabe K (2020). CD8 T cell score as a prognostic biomarker for triple negative breast cancer. International Journal of Molecular Sciences 21: 6968. https://doi.org/10.3390/ijms21186968 [Google Scholar] [PubMed] [CrossRef]
Qiu M, Xia W, Chen R, Wang S, Xu Y et al. (2018). The circular RNA circPRKCI promotes tumor growth in lung adenocarcinoma. Cancer Research 78: 2839–2851. https://doi.org/10.1158/0008-5472.CAN-17-2808 [Google Scholar] [PubMed] [CrossRef]
Raskov H, Orhan A, Christensen JP, Gögenur I (2021). Cytotoxic CD8(+) T cells in cancer and cancer immunotherapy. British Journal of Cancer 124: 359–367. https://doi.org/10.1038/s41416-020-01048-4 [Google Scholar] [PubMed] [CrossRef]
Shen Y, Morishita M, di Luccio E (2020). High yield recombinant expression and purification of oncogenic NSD1, NSD2, and NSD3 with human influenza hemagglutinin tag. Protein Expression and Purification 166: 105506. https://doi.org/10.1016/j.pep.2019.105506 [Google Scholar] [PubMed] [CrossRef]
Su H, Fan G, Huang J, Qiu X (2022). LncRNA HOXC-AS3 promotes non-small-cell lung cancer growth and metastasis through upregulation of YBX1. Cell Death & Disease 13: 307. https://doi.org/10.1038/s41419-022-04723-x [Google Scholar] [PubMed] [CrossRef]
Sun Y, Xie J, Cai S, Wang Q, Feng Z, Li Y, Lu JJ, Chen W, Ye Z (2021). Elevated expression of nuclear receptor-binding SET domain 3 promotes pancreatic cancer cell growth. Cell Death & Disease 12: 913. https://doi.org/10.1038/s41419-021-04205-6 [Google Scholar] [PubMed] [CrossRef]
Sung H, Ferlay J, Siegel RL, Laversanne M, Soerjomataram I, Jemal A, Bray F (2021). Global cancer statistics 2020: GLOBOCAN estimates of incidence and mortality worldwide for 36 cancers in 185 countries. CA: A Cancer Journal for Clinicians 71: 209–249. https://doi.org/10.3322/caac.21660 [Google Scholar] [PubMed] [CrossRef]
Trost B, Walker S, Wang Z, Thiruvahindrapuram B, MacDonald JR et al. (2018). A comprehensive workflow for read depth-based identification of copy-number variation from whole-genome sequence data. American Journal of Human Genetics 102: 142–155. https://doi.org/10.1016/j.ajhg.2017.12.007 [Google Scholar] [PubMed] [CrossRef]
Turner-Ivey B, Smith EL, Rutkovsky AC, Spruill LS, Mills JN, Ethier SP (2017). Development of mammary hyperplasia, dysplasia, and invasive ductal carcinoma in transgenic mice expressing the 8p11 amplicon oncogene NSD3. Breast Cancer Research and Treatment 164: 349–358. https://doi.org/10.1007/s10549-017-4258-9 [Google Scholar] [PubMed] [CrossRef]
Vougiouklakis T, Hamamoto R, Nakamura Y, Saloura V (2015). The NSD family of protein methyltransferases in human cancer. Epigenomics 7: 863–874. https://doi.org/10.2217/epi.15.32 [Google Scholar] [PubMed] [CrossRef]
Voutsadakis IA (2020). 8p11.23 amplification in breast cancer: Molecular characteristics, prognosis and targeted therapy. Journal of Clinical Medicine 9: 3079. https://doi.org/10.3390/jcm9103079 [Google Scholar] [PubMed] [CrossRef]
Wang C, Wang Q, Xu X, Xie B, Zhao Y, Li N, Cao X (2017). The methyltransferase NSD3 promotes antiviral innate immunity via direct lysine methylation of IRF3. Journal of Experimental Medicine 214: 3597–3610. https://doi.org/10.1084/jem.20170856 [Google Scholar] [PubMed] [CrossRef]
Weinstein JN, Collisson EA, Mills GB, Shaw KR, Ozenberger BA, Ellrott K, Shmulevich I, Sander C, Stuart JM (2013). The cancer genome atlas pan-cancer analysis project. Nature Genetics 45: 1113–1120. https://doi.org/10.1038/ng.2764 [Google Scholar] [PubMed] [CrossRef]
Xu F, Tang Q, Wang Y, Wang G, Qian K, Ju L, Xiao Y (2021). Development and validation of a six-gene prognostic signature for bladder cancer. Frontiers in Genetics 12: 758612. https://doi.org/10.3389/fgene.2021.758612 [Google Scholar] [PubMed] [CrossRef]
Yamashita H, Nakayama K, Ishikawa M, Ishibashi T, Nakamura K et al. (2019). Relationship between microsatellite instability, immune cells infiltration, and expression of immune checkpoint molecules in Ovarian Carcinoma: Immunotherapeutic strategies for the future. International Journal of Molecular Sciences 20: 5129. https://doi.org/10.3390/ijms20205129 [Google Scholar] [PubMed] [CrossRef]
Yang ZQ, Liu G, Bollig-Fischer A, Giroux CN, Ethier SP (2010). Transforming properties of 8p11-12 amplified genes in human breast cancer. Cancer Research 70: 8487–8497. https://doi.org/10.1158/0008-5472.CAN-10-1013 [Google Scholar] [PubMed] [CrossRef]
Yang X, Miao Y, Wang J, Mi D (2021). A pan-cancer analysis of the HER family gene and their association with prognosis, tumor microenvironment, and therapeutic targets. Life Sciences 273: 119307. https://doi.org/10.1016/j.lfs.2021.119307 [Google Scholar] [PubMed] [CrossRef]
Yi L, Yi L, Liu Q, Li C (2019). Downregulation of NSD3 (WHSC1L1) inhibits cell proliferation and migration via ERK1/2 deactivation and decreasing CAPG expression in colorectal cancer cells. OncoTargets and Therapy 12: 3933–3943. https://doi.org/10.2147/OTT [Google Scholar] [CrossRef]
Yu G, Wang LG, Han Y, He QY (2012). ClusterProfiler: An R package for comparing biological themes among gene clusters. OMICS: A Journal of Integrative Biology 16: 284–287. https://doi.org/10.1089/omi.2011.0118 [Google Scholar] [PubMed] [CrossRef]
Yuan G, Flores NM, Hausmann S, Lofgren SM, Kharchenko V et al. (2021). Elevated NSD3 histone methylation activity drives squamous cell lung cancer. Nature 590: 504–508. https://doi.org/10.1038/s41586-020-03170-y [Google Scholar] [PubMed] [CrossRef]
Zheng M, Hu Y, Gou R, Nie X, Li X, Liu J, Lin B (2020). Identification three LncRNA prognostic signature of ovarian cancer based on genome-wide copy number variation. Biomedicine & Pharmacotherapy 124: 109810. https://doi.org/10.1016/j.biopha.2019.109810 [Google Scholar] [PubMed] [CrossRef]
Zuo S, Wei M, Wang S, Dong J, Wei J (2020). Pan-cancer analysis of immune cell infiltration identifies a prognostic immune-cell characteristic score (ICCS) in lung adenocarcinoma. Frontiers in Immunology 11: 1218. https://doi.org/10.3389/fimmu.2020.01218 [Google Scholar] [PubMed] [CrossRef]
Supplementary Materials
Figure S1: Relationship between nuclear receptor binding SET domain protein-3 (NSD3) expression and disease-specific survival (DSS). (a) Forest plot of univariate Cox proportional hazard regression illustrating the impact of NSD3 expression on DSS in 33 cancers. (b–e) Association of NSD3 mRNA levels with DSS from the Kaplan-Meier plotter in PAAD, PRAD, THYM, and UVM.
Figure S2: The correlation between nuclear receptor binding SET domain protein-3 (NSD3) expression and DNA amplification. The correlation coefficients between NSD3 expression and NSD3 copy number variation are shown in BRCA, CHOL, COAD, ESCA, HNSC, LIHC, LUAD, LUSC, READ, and STAD.
Figure S3: Correlation of nuclear receptor binding SET domain protein-3 (NSD3) expression with immune cell infiltration. (a) The numbers of CD8+ T cells were relative to NSD3 expression in BRCA, KIRC, KIRP, LGG, PRAD, THYM, and UCEC. (b) Numbers of Treg cells correlated with NSD3 in BRCA, KIRC, SKCM, and THCA. (c) M2 macrophages correlated with NSD3 in BLCA, CESC, GBM, HNSC, KIRP, and LAML. (d) Activated natural killer cells correlated with NSD3 in BRCA, HNSC, KIRP, and PRAD.
Figure S4: Gene set variation analysis (GSVA) of nuclear receptor binding SET domain protein-3 (NSD3)-related genes. The results are indicated as bar plots for the various cancer types: CHOL, GBM, HNSC, KIRP, LGG, OV, PCPG, READ, and SARC. The top 15 greatest enriched pathways of each cancer type are represented as bar graphs. Positive and negative correlations are illustrated using yellow and blue bars, respectively.
Cite This Article
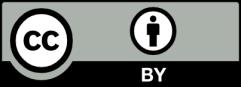
This work is licensed under a Creative Commons Attribution 4.0 International License , which permits unrestricted use, distribution, and reproduction in any medium, provided the original work is properly cited.