Open Access
ARTICLE
A developed ant colony algorithm for cancer molecular subtype classification to reveal the predictive biomarker in the renal cell carcinoma
1 Department of Urology, Aerospace Center Hospital, Beijing, 100049, China
2 Beijing Institute of Technology, Beijing, 100081, China
3 Urology Surgery, Hebei Petro China Central Hospital, Langfang, 065000, China
* Corresponding Authors: BAO ZHANG. Email: ; ZHILONG REN. Email:
# These authors contributed to the work equally
(This article belongs to the Special Issue: Application of Deep Learning in Cancer)
BIOCELL 2023, 47(3), 555-567. https://doi.org/10.32604/biocell.2023.026254
Received 26 August 2022; Accepted 17 October 2022; Issue published 03 January 2023
Abstract
Background: Recently, researchers have been attracted in identifying the crucial genes related to cancer, which plays important role in cancer diagnosis and treatment. However, in performing the cancer molecular subtype classification task from cancer gene expression data, it is challenging to obtain those significant genes due to the high dimensionality and high noise of data. Moreover, the existing methods always suffer from some issues such as premature convergence. Methods: To address those problems, we propose a new ant colony optimization (ACO) algorithm called DACO to classify the cancer gene expression datasets, identifying the essential genes of different diseases. In DACO, first, we propose the initial pheromone concentration based on the weight ranking vector to accelerate the convergence speed; then, a dynamic pheromone volatility factor is designed to prevent the algorithm from getting stuck in the local optimal solution; finally, the pheromone update rule in the Ant Colony System is employed to update the pheromone globally and locally. To demonstrate the performance of the proposed algorithm in classification, different existing approaches are compared with the proposed algorithm on eight high-dimensional cancer gene expression datasets. Results: The experiment results show that the proposed algorithm performs better than other effective methods in terms of classification accuracy and the number of feature sets. It can be used to address the classification problem effectively. Moreover, a renal cell carcinoma dataset is employed to reveal the biological significance of the proposed algorithm from a number of biological analyses. Conclusion: The results demonstrate that CAPS may play a crucial role in the occurrence and development of renal clear cell carcinoma.Keywords
Cite This Article
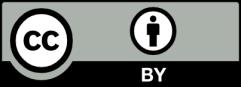
This work is licensed under a Creative Commons Attribution 4.0 International License , which permits unrestricted use, distribution, and reproduction in any medium, provided the original work is properly cited.