Open Access
ARTICLE
FGD5 as a novel prognostic biomarker and its association with immune infiltrates in lung adenocarcinoma
1 Department of Respiratory Medicine, Central South University, Xiangya Hospital, Changsha, 410078, China
2 Xiangya School of Medicine, Department of Medical Microbiology, Central South University, Changsha, 410078, China
* Corresponding Authors: LING QIN. Email: ; YURONG TAN. Email:
(This article belongs to the Special Issue: Regulation of Immune Cell Functions in Tumor Microenvironment)
BIOCELL 2023, 47(11), 2503-2516. https://doi.org/10.32604/biocell.2023.031565
Received 11 July 2023; Accepted 14 September 2023; Issue published 27 November 2023
Abstract
Background: Non-small cell lung cancer (NSCLC) has a poor prognosis with a low 5-year survival rate. Lung adenocarcinoma (LUAD) accounts for 50%. Facio-genital dysplasia-5 (FGD5), a member of a subfamily of Rho GTP-GDP exchange factors, may be a good molecular biomarker for diagnosis and prognosis. Objective: To explore the clinical application of FGD5, the study was designed to investigate the prognosis value of FGD5 expression and its correlation with immune infiltrates in LUAD patients. Methods: Through the Wilcoxon signed-rank test and logistic regression, the correlation between clinical characteristics and FGD5 expression was analyzed. Kaplan–Meier plotter analysis, Cox regression, and a receiver operating characteristic (ROC) curve were constructed to evaluate the influence of FGD5 on prognosis. The function of FGD5 in lung cancer was analyzed using Gene set enrichment analysis (GSEA) and Single Sample Gene Set Enrichment Analysis (ssGSEA). Results: Compared to normal tissues, FGD5 was downregulated in LUAD. FGD5 may be a potential diagnostic and prognostic biomarker of lung cancer for its association with improved overall survival (OS), progression-free interval (PFI), and disease-specific survival (DSS) in LUAD. Results of functional analysis suggested that FGD5 was involved in the immune response for its association with immune infiltration. Conclusion: FGD5 may be a significant molecular biomarker for diagnosis and prognosis, presenting as an independent prognostic risk factor for LUAD.Graphic Abstract
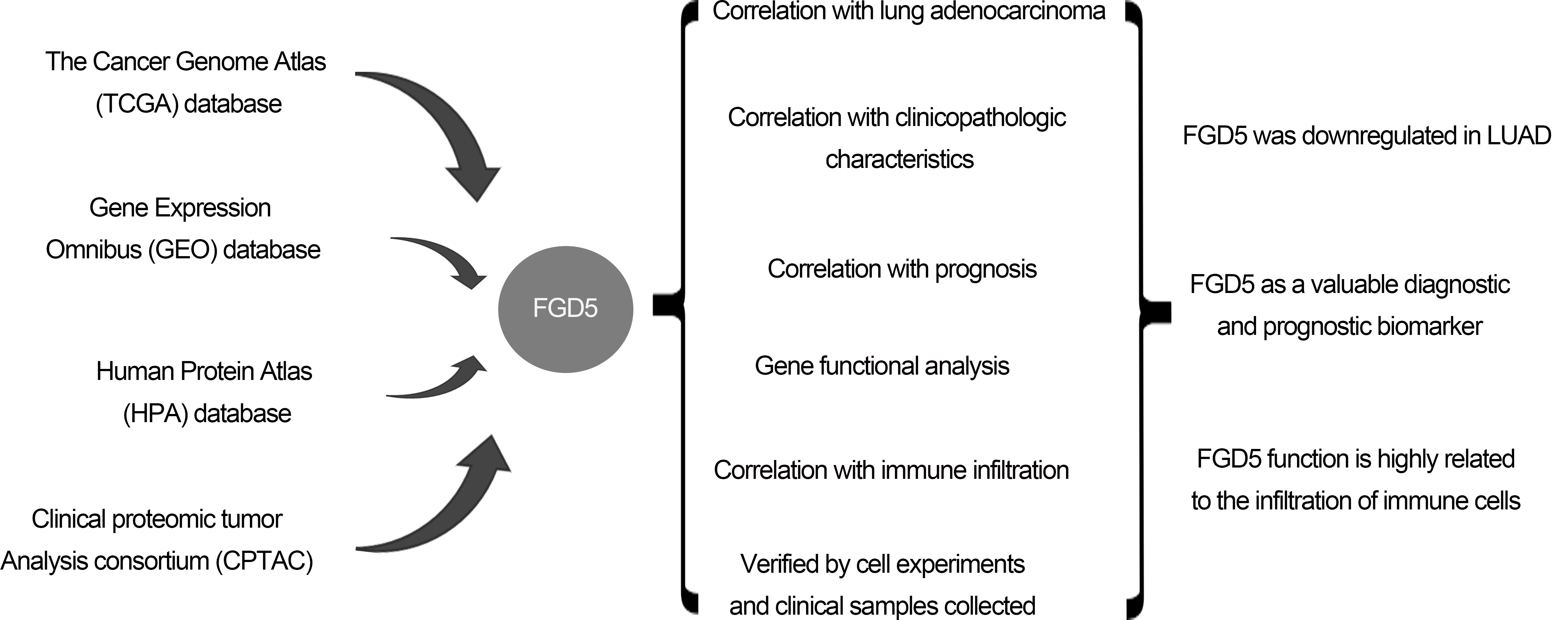
Keywords
Lung cancer may be classified by cell type into two categories: non-small cell lung cancer (NSCLC) and small cell cancer. Most lung cancers are of the NSCLC type, which includes three main subtypes. The most prevalent subtype of NSCLC is lung adenocarcinoma (LUAD), accounting for 50% of all lung cancer cases (Denisenko et al., 2018). Over the past few decades, researchers have developed multimodal treatment strategies, including radiotherapy, immunotherapy, and non-invasive surgical resection. However, doctors and patients still deal with the challenges of cancer metastasis and recurrence problems (Rizvi et al., 2018). Due to poor screening, about 70% of patients present local tumor progression or metastasis at diagnosis, leading to disappointing treatment outcomes for most cancers. This is especially true for lung cancer, which has a relative 5-year overall survival rate of only 18% (Babar et al., 2021; Siegel et al., 2014). Therefore, it is necessary and urgent to identify and apply new and more valuable prognostic biomarkers to improve current tumor diagnosis.
Faciogenital dysplasia-5 (FGD5) is a member of the FYVE, Rho GTP/GDP exchange factor, and pleckstrin homology domain-containing family (Farhan et al., 2017). FGD5 activates Rho GTPase playing a role in cell motility regulation and cytoskeleton remodeling (Nobes and Hall, 1995; Park et al., 2021). Lack of FGD5 in mice results in the failure of VEGF signaling, disordered angiogenesis, and is lethal to mouse embryos (Farhan et al., 2017). Multiple studies show that FGD5 has also been identified as a critical regulator of tumor cell proliferation and associated with poor prognosis in primary breast cancers (Gatza et al., 2014; Valla et al., 2018). Studies on gastric cancer have shown that FGD5 promotes tumor growth by regulating EGFR ubiquitination (Chen et al., 2021). Similarly, FGD5 can also interact with Sox2 protein to reduce ubiquitination, thus enhancing Sox2 protein stability and promoting gastric carcinogenesis (You et al., 2021). However, the potential mechanism of FGD5 in LUAD is unclear.
In this work, using bioinformatics tools and analyses, we investigated the expression of FGD5, its prognostic value, and the correlation between FGD5 and tumor-infiltrating immune cells (TIILs) in a tumor microenvironment (TME). Furthermore, we verified the correlation of FGD5 with immune cells in vitro.
We downloaded the RNA expression profiles (RNA-Seq2 level 3 data; platform: Illumina HiSeq 2000, through December 2019) and clinical data of LUAD patients from The Cancer Genome Atlas (TCGA) database (https://portal.gdc.cancer.gov/). We also downloaded RNA sequence data from Gene Expression Omnibus (GEO), including GSE116959, GSE32863, and GSE31210. Expression levels of FGD5 protein in lung tissue were investigated using the immunohistochemistry data from the Human Protein Atlas (HPA) database (https://www.proteinatlas.org/) (Uhlén et al., 2015) and the Clinical Proteomic Tumor Analysis Consortium (CPTAC) (Chen et al., 2019) with the UALCAN database (http://ualcan.path.uab.edu/analysis-prot.html) (Chandrashekar et al., 2017).
Transcriptome data from 33 cancer types in TCGA were used to analyze the expression difference of FGD5 in pan-cancer. The expression difference of FGD5 in TCGA_LUAD was analyzed using paired and unpaired methods. In addition, FGD5 expressions in GSE116959, GSE32863, and GSE31210 were obtained from the GEO database for verification.
Analysis of FGD5-interacting protein
The GeneMANIA database (http://www.genemania.org) (Warde-Farley et al., 2010) was applied to construct the FGD5 interaction network.
Analysis of differentially expressed genes (DEGs) between high and low FGD5 expression groups in LUAD patients
Taking the median expression value of FGD5 as the limit, the inclusion of FGD5 expression value above the median in LUAD samples was classified as the high expression group of FGD5, and the inclusion of expression value below the median was classified as the low expression group of FGD5. The two groups were compared, and the criteria of |log2FC| ≥ 1 and p-value < 0.05 were used to screen for significant differential genes.
Gene ontology (GO) analysis and kyoto encyclopedia of genes and genomes (KEGG) analyses
GO and KEGG pathway analyses were performed on significantly different genes screened after grouping according to FGD5 expression level to explore FGD5 function (Subramanian et al., 2005). GO and KEGG enrichment analyses were performed using the clusterProfiler package (Yu et al., 2012).
Gene set enrichment analysis (GSEA)
DEGs between high-and low-FGD5 expression groups in the training set were calculated using the R packages mentioned above. Then, GSEA (http://software.broadinstitute.org/gsea/index.jsp) (Subramanian et al., 2005; Yu et al., 2012) was performed to identify the hallmarks of the high-FGD5 expression group compared to the low-FGD5 expression group.
Analysis of immune infiltration and its correlation with FGD5 expression
TIMER (https://cistrome.shinyapps.io/timer/) (Li et al., 2016, 2017), an interactive web portal, is a good tool to analyze immune abnormalities and screen for immune-related molecular biomarkers. In addition, TIMER was also applied to investigate the relationship between FGD5 expression and different sets of gene markers in immune cells by using the “Correlation” module. The correlations of FGD5 expression with immune infiltration were evaluated by purity-correlated partial Spearman’s correlation and statistical significance.
Kaplan–Meier plotter database and prognoScan database analysis
We used KM Plotter (http://kmplot.com) (Lánczky and Győrffy, 2021) and PrognoScan database (http://dna00.bio.kyutech.ac.jp/PrognoScan/) (Mizuno et al., 2009) to analyze the prognostic value of FGD5 in LUAD.
Prognostic model generation and prediction
Kaplan–Meier plots were created, and the log-rank test was performed using a survival package. The diagnostic ROC curve, diagnostic time-dependent curve, and nomogram model analysis were created using R packages, including pROC, timeROC, and survival packages (Liu et al., 2018). Clinical data used in this section were retrieved from the TCGA database.
Lung cancer sample collection, cell culture and real-time RT-PCR
Postoperative cancer tissue and adjacent normal tissue samples from 6 lung cancer patients were collected from the Second Xiangya Hospital. This experiment strictly complied with the Helsinki Declaration of the World Medical Congress, and all samples were obtained with the informed consent of patients and approved by the ethical review committee of Xiangya Medical College. Human normal lung epithelial cell HBE, lung cancer cell PC9, H1299, and A549 cells were cultured in DMEM plus 10% fetal bovine serum (FBS) at 37°C and 5% CO2. Total RNA was extracted from tissue and cell samples using TRIzol reagent (Takara, Kyoto, Japan). Primers were synthesized by Sangon Biotech Co., Ltd. (Shanghai, China).
The primers sequences are listed below:
FGD5: 5′-CCTTGTCATCGCACAGGAACTG-3′ (forward), 5′-CTCTGCCTTCATGGTCCATGTC-3′ (reverse); PDL1: 5′-TGCCGACTACAAGCGAATTACTG-3′ (forward), 5′-CTGCTTGTCCAGATGACTTCGG-3′ (reverse); PDL2: 5′-CTCGTTCCACATACCTCAAGTCC-3′ (forward), 5′-CTGGAACCTTTAGGATGTGAGTG-3′ (reverse); CXCL12: 5′-CTCAACACTCCAAACTGTGCCC-3′ (forward), 5′-CTCCAGGTACTCCTGAATCCAC-3′ (reverse); CXCL10: 5′-GGTGAGAAGAGATGTCTGAATCC-3′ (forward), 5′-GTCCATCCTTGGAAGCACTGCA-3′ (reverse). Each sample was reverse transcribed into cDNA using TranScript Uni All-in-One First-Strand cDNA Synthesis SuperMix for qPCR (Transgen, Beijing, China). Then the cDNA was synthesized by reverse transcription and amplified using 2× SYBR Green qPCR Master Mix (Bimake, Houston, USA) according to the manufacturer’s instructions. qPCR was performed at 95°C for 3 min, and 40 cycles of 95°C for 15 s, 60°C for 30 s, and 72°C for 30 s. GAPDH and β-actin were the internal references for the other target genes. The relative expression levels of mRNA were calculated using the 2−ΔΔCT method.
Construction of FGD5 high-expressed vector
PCDNA3.1-FGD5/3 × Flag-EGFP was constructed and synthesized by GenScript Biology (Beijing, China). H1299 and PC9 cells were transfected with Lipofectamine 2000 (Invitrogen, NY, USA) when the densities of H1299 and PC9 cells were 70%. Then, 500 mg/ml of G418 was used for screening, and the cell culture medium was changed every 3–5 days. When a large number of cells died, the concentration of G418 was halved to maintain screening, and positive clones were selected. The culture was maintained with 200 mg/ml G418. The expression level of FGD5 was detected by fluorescence microscope and real-time qPCR.
Statistical analysis was performed using R (v.4.2.1). The differences between groups were compared using the Wilcoxon rank-sum test or Student’s t-test. Correlations were determined using Pearson or Spearman correlation tests. Kaplan-Meier plots were created, and log-rank tests were performed to identify the difference between survival curves. p < 0.05 were considered statistically significant.
FGD5 was downregulated in LUAD
We analyzed the expression of FGD5 mRNA across various cancer types. As shown in Fig. 1A, compared with normal tissues, FGD5 mRNA expression was significantly downregulated in BLCA, BRCA, CESC, COAD, KICH, KIRP, LUAD, LUSC, PRAD, READ, and UCEC, while CHOL, KIRC, LIHC, and PCPG were significantly upregulated. These results suggest that the expression of FGD5 differs significantly between different tumor types. Additionally, we used the GEO, TCGA, and HPA databases to verify that FGD5 mRNA is indeed significantly downregulated in LUAD (Fig. 1B). This result is consistent with the UALCAN database results, which indicate that FGD5 protein expression in LUAD is significantly lower than that in normal tissues (Fig. 1C). Similarly, immunohistochemical staining results for FGD5 from the HPA database also showed the downregulation of FGD5 protein in LUAD tissues (Fig. 1D), and (Courtesy of Human Protein Atlas). These results suggest that both mRNA and protein expression of FGD5 are downregulated in LUAD.
Figure 1: mmRNA and protein expression levels of FGD5 in LUAD. (A) Expression pattern of FGD5 in various cancer types. (B) FGD5 transcript expression level in GSECA116959, GSE31210, GSE32863, and TCGA_LUAD. (C) Expression of FGD5 protein in LUAD and normal tissues was compared using the UALCAN database. (D) Immunohistochemical evidence from the Human Protein Atlas for comparison of FGD5 protein in LUAD and normal tissue (Image credit: Human Protein Atlas, www.proteinatlas.org, (Uhlén et al., 2015. Image available at the following URL: v23.proteinatlas.org/humancell. Colwill and Gräslund, 2011)) (*p < 0.05, **p < 0.01, and ***p < 0.001). ns, nonsignificant.
Correlation between FGD5 expression and clinical characteristics in LUAD patients
At present, there is a lack of studies investigating the function of FGD5 in LUAD, which impedes a clear understanding of FGD5’s role in LUAD development. However, assessing the correlation between clinicopathological features and FGD5 expression levels can effectively help elucidate FGD5’s role in LUAD progression. Thus, we used the Fisher, Chi-squared, and Wilcoxon tests to analyze the correlation between FGD5 expression and clinical characteristics (Table 1). The analyses showed that a low FGD5 expression was significantly associated with smoking (p = 0.037), while no significant associations were observed with other features.
High FGD5 expression correlates with good prognosis in LUAD
An outstanding biomarker can often serve multiple clinical purposes, such as diagnosis and prognosis. ROC analysis results for TCGA_LUAD showed that FGD5’s AUC value was 0.982 (95% CI: 0.967–0.997), with a cut-off value was 2.888. The sensitivity and specificity were 96.6 and 95.7, respectively. Compared to the positive predictive value of 71.3%, the negative predictive value was as high as 99.6%. This result shows the potential of FGD5 as an excellent biomarker for distinguishing normal tissue from LUAD tissue.
We then assessed whether FGD5 has prognostic value for LUAD. The results of the Kaplan–Meier plot indicated that it was associated with good overall survival (OS) (HR = 0.53(0.41–0.68), p = 8.4e-07) (Figs. 2A and 2B), and the PrognoScan database demonstrated that high expression of FGD5 was significantly correlated with good OS in the GSE13213 (p = 0.006889) and GSE31210 (p = 0.0367) cohorts (Figs. 2C and 2D). Moreover, in TCGA_LUAD, higher expression of FGD5 exhibited good OS (p = 0.002), progress-free interval (PFI) (p = 0.042), and disease-specific survival (DSS) (p = 0.009) (Figs. 2E–2G).
Figure 2: Diagnostic and prognostic value analysis of FGD5. (A) ROC curve for FGD5 in LUAD samples (Cao and López-de-Ullibarri, 2019); (B) Kaplan-Meier survival analysis FGD5 (226985_at) overall survival (OS); (C and D) survival curves using PrognoScan database; (E) OS survival analysis in TCGA-LUAD database; (F) progression-free interval (PFI); (G) disease-specific survival (DSS).
Next, we performed Univariate Cox and Multivariate Cox regression analyses to further evaluate the predictive value of FGD5 on clinical outcomes. As shown in Table 2, Univariate Cox analysis showed that high FGD5 expression was significantly correlated with good OS (p = 0.002). Furthermore, multivariate regression analysis confirmed that FGD5 expression served as an independent prognostic factor for OS in LUAD patients (p = 0.015).
By constructing the protein interaction network using GeneMania, we found that FGD5 is closely related to 20 genes, including HSPA12B, MYCT1, and AGDRL4 (Fig. 3A). Functional enrichment of 20 closely related genes was significantly associated with guanyl-nucleotide exchange factor activity, Rho GTPase binding, and Ras guanyl-nucleotide exchange factor activity. By analyzing co-expressed genes, FGD5 was negatively correlated with HSPE1, DPY3, MTX2, SNRP, UQCC2, SF3B6, SNRPE, POLR2H, MRPS15, and NIF3L1, but positively correlated with ZNF366, ARHGEF15, RHOJ, SH2B3, FLI1, ACVRL1, GIMAP8, PECAM, STARD8, and CD93 in LUAD (Figs. 3A and 3B). In addition, a total of 1089 significantly different genes were identified in LUAD after comparing the FGD5 high expression group and low expression group including 380 significantly down-regulated genes and 709 significantly up-regulated genes. After KEGG analysis of these differential genes, the results showed that signaling pathways involved include Neuroactive ligand-receptor interaction, Protein digestion and absorption, Metabolism of xenobiotics by cytochrome P450, Drug metabolism-cytochrome P450, Steroid hormone biosynthesis, ECM-receptor interaction, Ascorbate and aldarate metabolism, Chemical carcinogenesis, Pentose and glucuronate interconversions, and Complement and coagulation cascades (Figs. 3C–3F).
Figure 3: Interacting proteins, KEGG, and GO enrichment analysis for FGD5. (A) Construction of FGD5 Gene Interaction Network using GeneMania Online Database. (B) Heat maps showing the top 10 genes positively and negatively correlated with FGD5 expression in LUAD; (C) KEGG analysis of significantly differentially expressed genes grouped according to FGD5 expression in LUAD; (D–F) GO analysis of significantly differentially expressed genes grouped according to FGD5 expression in LUAD (***p < 0.001).
FGD5-related signaling pathways based on GSEA
We analyzed the pathways involved in FGD5 by GSEA to better understand FGD5’s function in LUAD. As shown in Fig. 4, we found that olfactory transduction, pathways in cancer, neuroactive ligand–receptor interaction, cytokine–cytokine receptor interaction, chemokine signaling pathway, regulation of actin cytoskeleton, calcium signaling pathway, focal adhesion, toll-like receptor signaling pathway, JAK-STAT signaling pathway, T cell receptor signaling pathway, and natural killer cell-mediated cytotoxicity were enriched in FGD5 high expression phenotype.
Figure 4: FGD5-related signaling pathways based on GSEA.
Immune cell infiltration analysis of FGD5 in TCGA-LUAD
We analyzed the correlation between FGD5 expression and 24 types of infiltrating immune cells in LUAD patients using ssGSEA (Fig. 5A). The results indicate that FGD5 upregulation is associated with increased infiltration levels of macrophages, iDCs, pDCs, and mast cells (p < 0.001, Fig. 5B). Additionally, high FGD5 expression corresponded to high immune infiltration scores for macrophages, iDCs, pDCs, B cells, mast cells, neutrophils, DCs, Th1 cells, eosinophils, T cells, Tregs, cytotoxic cells, TFH, Tcm, Tem, aDC, CD8 T cells, NK cells, NK CD56dim cells, and T helper cells (Fig. 5C). However, Th2 cells showed the opposite trend.
Figure 5: Correlation of FGD5 expression with immune infiltration level. (A) FGD5 is positively correlated with macrophage cell, iDC, pDC, and mast cell infiltration. (B) Correlation of FGD5 expression with different types of immune cells. (C) Correlation scatter diagram of four important immune cells including macrophages, iDCs, pDCs, mast cells, and FGD5 expression in LUAD (*p < 0.05, **p < 0.01, and ***p < 0.001). ns, no significance.
Significant correlation between FGD5 expression and multiple immune markers
To further elucidate FGD5’s role in immune infiltration, we analyzed the association between FGD5 and various immunological features including CD8+ T cells, T cells (general), Monocytes, B cells, tumor-associating macrophages (TAMs), M1 macrophages, M2 macrophages, Natural killer cells, Neutrophils, Dendritic cells, Th1, Th2, Th17, Tfh, Treg, and T cell exhaustion using the TIMER database. In clinical biopsies of tumors, immune infiltration dissection may be affected by tumor purity. Expression of FGD5 correlated significantly with immune markers adjusted for tumor purity, such as PD-1 (p = 0.010), CTLA4 (p = 3.81E-05), and PDL1 (p = 1.63E-07) in LUAD (Table 3).
FGD5 in lung cancer cells promoted chemotaxis of immune cells, but enhanced T cell exhaustion
We evaluated the expression difference of FGD5 mRNA between lung cancer and normal cell lines using qRT-PCR technology. As shown in Fig. 6A, the results showed that compared with normal cells, FGD5 mRNA expression in lung cancer cells, including PC9, H1299, and A549, was lower. In addition, we also detected FGD5 mRNA expression in cancer tissues and adjacent normal tissues of 6 lung cancer patients collected by ourselves, and the results also showed that FGD5 mRNA was significantly down-regulated in lung cancer tissues (Fig. 6B). These results are consistent with those obtained by bioinformatics analysis. Then, we constructed FGD5 high-expressed cell lines and verified them by qRT-PCR (Fig. 6C). Further experiments showed that FGD5 promoted the expression of CXCL10, CCL12, PDL1 and PDL2 (Figs. 6D–6G).
Figure 6: The effects of FGD5 on immune cell chemotaxis and T cell exhaustion were assayed using real-time qPCR (n = 5). (A) Expression of FGD5 mRNA in lung cancer and normal cell lines. (B) Expression of FGD5 mRNA in lung cancer tissue and adjacent normal tissue. (C) Expression of FGD5 mRNA in lung cancer cell lines PC9 and H1299 after transfection with FGD5 high expression plasmid. (D) The effects of FGD5 on CXCL10 expression. (E) The effects of FGD5 on CCL12 expression. (F) The effects of FGD5 on PDL1 expression. (G) Effects of FGD5 on PDL2 expression (*p < 0.05, **p < 0.01, ***p < 0.001 and ****p < 0.0001).
LUAD is one of the most common causes of cancer-related deaths worldwide (Dai et al., 2021). Although scientists have developed several approaches to improve patients’ quality of life, most LUAD patients still face poor clinical outcomes. The mechanisms of LUAD pathogenesis and progression so far remain unclear (Tang et al., 2022). Therefore, elucidating the biological mechanism of LUAD occurrence and development is a prerequisite for clinical diagnosis and subsequent treatment (Duma et al., 2019). It is gratifying that some breakthroughs in omics methods, such as genomics, epigenomics, functional genomics, metabolomics, and proteomics, have efficiently enabled the identification of potentially novel potential biomarkers that help to develop accurate diagnosis and treatment strategies (Tang et al., 2022; Eckhardt et al., 2020). This study, based on bioinformatics analysis of public data, is the first to report FGD5 as a valuable marker for the diagnosis and prognosis of LUAD.
FGD5 is considered an important member of the Rho family of guanine nucleotide exchange factors, presumably playing a key role in endothelial cell apoptosis and angiogenesis (Chen et al., 2021). In this study, bioinformatic analysis showed that genes closely related to FGD5 are involved in guanyl-nucleotide exchange factor activity, Rho GTPase binding, and Ras guanyl-nucleotide exchange factor activity. Furthermore, these signaling pathways are associated with tumor formation, proliferation, migration, diffusion, and angiogenesis. All these findings suggest that FGD5 plays an important role in tumor occurrence and development. However, there is a lack of studies on the role of FGD5 in tumors.
Our results showed distinct FGD5 expression in different tumors. A few published studies show that upregulation of FGD5 in breast and gastric cancer promotes the growth of both cancers by enhancing the stability of the epidermal growth factor receptor (EGFR) (Chen et al., 2021; Li et al., 2021). EGFR transmembrane protein can signal important extracellular growth factors into the cell through its own cytoplasmic kinase activity (Da Cunha Santos et al., 2011). However, mutations in EGFR are frequently found in NSCLC, occurring in about 40% to 60% of never-smokers and approximately 17% of LUAD patients (Gadgeel and Wozniak, 2013). Mutant EGFR activates the PI3K/Akt/mTOR pathway, which plays a fundamental role in tumor oncogenesis, proliferation, and survival (Makinoshima et al., 2015). A recent study found that FGD5 can both remodel the cytoskeleton by coupling phosphoinositide 3-kinase (PI3)/mTORC2 and regulate the retention of VEGFR2 in recycling endosomes (Farhan et al., 2017). In this study, the results showed the downregulation of FGD5 at both mRNA and protein levels in LUAD patients. It has good diagnostic and prognostic value with high accuracy in predicting LUAD, indicating that FGD5 may have a complex function in cancers.
We further obtained FGD5 co-expressed genes, and found that FGD5 was co-expressed with ZNF366, ARHGEF15, RHOJ, SH2B3, FLI1, ACVRL1, GIMAP8, PECAM1, STARD8, and CD93 in LUAD. Pro-angiogenic factors derived from tumor cells lead to the formation of abnormal vascular networks that are characterized by immaturity, disorder, and permeability (Viallard and Larrivée, 2017). Blocking CD93 in mice can improve drug delivery efficiency, thereby improving the anti-tumor effects of gemifloric uracil and citabine (Sun et al., 2021). Studies have shown that a large increase in intratumoral effector T cells occurs after blocking the CD93 pathway, which in turn can increase the sensitivity of immune checkpoint therapy in mice (Sun et al., 2021). However, our analysis revealed a clear consistency in mRNA expression levels between FGD5 and CD93.
Furthermore, we implemented ssGSEA analysis in LUAD and found that high expression of FGD5 shoqed a significant positive correlation with the infiltration of various immune cells, including macrophages, iDCs, pDCs, and mast cells. CXCL10 expression induces the recruitment of immune cells, especially CD8+ T cells (Shang et al., 2022). CCL12 chemokines can enhance the recruitment of macrophages, cDCs, and pDCs at the cellular level and are associated with the augmentation of classical NF-κB subunit (Lopes et al., 2018). We validated the positive correlations between FGD5 expression and CXCL10 and CCL12. However, FGD5 was able to strongly induce the expression of PDL1 and PDL2, markers of T cell exhaustion, on tumor cells. We hypothesize that a certain level of FGD5 is important for maintaining pulmonary angiogenesis and regulating immune cell infiltration, but high levels of FGD5 expression still have adverse effects on lung cancer patients.
While our study provides new insights into the relationship between FGD5 expression and prognostic value in patients with LUAD, some limitations need to be considered. First, for most of the data in this study came from online databases, we were unable to obtain some important clinical information. Second, although we collected samples for verification, our self-owned sample size was limited. Third, the biological function and potential mechanism of FGD5 in LUAD require further studies and rigorous systematic experiments both in vivo and in vitro.
In this study, we confirmed that FGD5 was significantly downregulated in LUAD, and we identified FGD5 as a valuable diagnostic and prognostic biomarker. Additionally, we found that FGD5 function is highly related to the infiltration of immune cells.
Acknowledgement: None.
Funding Statement: The authors received no specific funding for this study.
Author Contributions: The authors confirm contribution to the paper as follows: study conception and design: YT, LQ; data collection: ZT; analysis and interpretation of results: ZT, LW, GW; draft manuscript preparation: YT, ZT. All authors reviewed the results and approved the final version of the manuscript.
Availability of Data and Materials: The data that support the findings of this study are available from the corresponding author.
Ethics Approval: The study was approved by the Institutional Ethic Committee of Xiangya School of Medicine, Central South University (No. 2017–S000) and written informed consent from all participants.
Conflicts of Interest: The authors declare that they have no conflicts of interest to report regarding the present study.
References
Babar L, Modi P, Anjum F (2021). Lung cancer screening. In: StatPearls. Treasure Island (FLStatPearls Publishing. [Google Scholar]
Cao R, López-de-Ullibarri I (2019). ROC curves for the statistical analysis of microarray data. Methods in Molecular Biology 1986: 245–253. https://doi.org/10.1007/978-1-4939-9442-7 [Google Scholar] [CrossRef]
Chandrashekar DS, Bashel B, Balasubramanya SAH, Creighton CJ, Ponce-Rodriguez I, Chakravarthi BVSK, Varambally S (2017). UALCAN: A portal for facilitating tumor subgroup gene expression and survival analyses. Neoplasia 19: 649–658. https://doi.org/10.1016/j.neo.2017.05.002 [Google Scholar] [PubMed] [CrossRef]
Chen F, Chandrashekar DS, Varambally S, Creighton CJ (2019). Pan-cancer molecular subtypes revealed by mass-spectrometry-based proteomic characterization of more than 500 human cancers. Nature Communications 10: 5679. https://doi.org/10.1038/s41467-019-13528-0 [Google Scholar] [PubMed] [CrossRef]
Chen N, Han X, Yin B, Bai X, Wang Y (2021). FGD5 facilitates tumor growth by regulating EGFR ubiquitination in gastric cancer. Biochemical and Biophysical Research Communications 562: 43–49. https://doi.org/10.1016/j.bbrc.2021.04.106 [Google Scholar] [PubMed] [CrossRef]
Colwill K, Gräslund S (2011). A roadmap to generate renewable protein binders to the human proteome. Nature Methods 8: 551–558. https://doi.org/10.1038/nmeth.1607 [Google Scholar] [PubMed] [CrossRef]
Da Cunha Santos G, Shepherd FA, Tsao MS (2011). EGFR mutations and lung cancer. Annual Review of Pathology 6: 49–69. https://doi.org/10.1146/annurev-pathol-011110-130206 [Google Scholar] [PubMed] [CrossRef]
Dai P, Tang Z, Ruan P, Bajinka O, Liu D, Tan Y (2021). Gimap5 inhibits lung cancer growth by interacting with M6PR. Frontiers in Oncology 11: 699847. https://doi.org/10.3389/fonc.2021.699847 [Google Scholar] [PubMed] [CrossRef]
Denisenko TV, Budkevich IN, Zhivotovsky B (2018). Cell death-based treatment of lung adenocarcinoma. Cell Death and Disease 9: 117. https://doi.org/10.1038/s41419-017-0063-y [Google Scholar] [PubMed] [CrossRef]
Duma N, Santana-Davila R, Molina JR (2019). Non-small cell lung cancer: Epidemiology, screening, diagnosis, and treatment. Mayo Clinic Proceedings 94: 1623–1640. https://doi.org/10.1016/j.mayocp.2019.01.013 [Google Scholar] [PubMed] [CrossRef]
Eckhardt M, Hultquist JF, Kaake RM, Huttenhain R, Krogan NJ (2020). A systems approach to infectious disease. Nature Reviews Genetics 21: 339–354. https://doi.org/10.1038/s41576-020-0212-5 [Google Scholar] [PubMed] [CrossRef]
Farhan MA, Azad AK, Touret N, Murray AG (2017). FGD5 regulates VEGF receptor-2 coupling to PI3 kinase and receptor recycling. Arteriosclerosis Thrombosis and Vascular Biology 37: 2301–2310. https://doi.org/10.1161/ATVBAHA.117.309978 [Google Scholar] [PubMed] [CrossRef]
Gadgeel SM, Wozniak A (2013). Preclinical rationale for PI3K/Akt/mTOR pathway inhibitors as therapy for epidermal growth factor receptor inhibitor-resistant non-small-cell lung cancer. Clinical Lung Cancer 14: 322–332. https://doi.org/10.1016/j.cllc.2012.12.001 [Google Scholar] [PubMed] [CrossRef]
Gatza ML, Silva GO, Parker JS, Fan C, Perou CM (2014). An integrated genomics approach identifies drivers of proliferation in luminal-subtype human breast cancer. Nature Genetics 46: 1051–1059. https://doi.org/10.1038/ng.3073 [Google Scholar] [PubMed] [CrossRef]
Lánczky A, Győrffy B (2021). Web-based survival analysis tool tailored for medical research (KMplotDevelopment and implementation. Journal of Medical Internet Research 23: e27633. https://doi.org/10.2196/27633 [Google Scholar] [PubMed] [CrossRef]
Li K, Zhang TT, Zhao CX, Wang F, Cui B et al. (2021). Faciogenital Dysplasia 5 supports cancer stem cell traits in basal-like breast cancer by enhancing EGFR stability. Science Translational Medicine 13: eabb2914. https://doi.org/10.1126/scitranslmed.abb2914 [Google Scholar] [PubMed] [CrossRef]
Li T, Fan J, Wang B, Traugh N, Chen Q, Liu JS, Li B, Liu XS (2017). TIMER: A web server for comprehensive analysis of tumor-infiltrating immune cells. Cancer Research 77: e108–e110. https://doi.org/10.1158/0008-5472.CAN-17-0307 [Google Scholar] [PubMed] [CrossRef]
Li B, Severson E, Pignon JC, Zhao H, Li T et al. (2016). Comprehensive analyses of tumor immunity: Implications for cancer immunotherapy. Genome Biology 17: 174. https://doi.org/10.1186/s13059-016-1028-7 [Google Scholar] [PubMed] [CrossRef]
Liu J, Lichtenberg T, Hoadley KA, Poisson LM, Lazar AJ et al. (2018). An integrated TCGA pan-cancer clinical data resource to drive high-quality survival outcome analytics. Cell 173: 400–416.e411. https://doi.org/10.1016/j.cell.2018.02.052 [Google Scholar] [PubMed] [CrossRef]
Lopes N, Charaix J, Cédile O, Sergé A, Irla M (2018). Lymphotoxin α fine-tunes T cell clonal deletion by regulating thymic entry of antigen-presenting cells. Nature Communications 9: 1262. https://doi.org/10.1038/s41467-018-03619-9 [Google Scholar] [PubMed] [CrossRef]
Makinoshima H, Takita M, Saruwatari K, Umemura S, Obata Y et al. (2015). Signaling through the phosphatidylinositol 3-kinase (PI3K)/mammalian target of rapamycin (mTOR) axis is responsible for aerobic glycolysis mediated by glucose transporter in epidermal growth factor receptor (EGFR)-mutated lung adenocarcinoma. Journal of Biological Chemistry 290: 17495–17504. https://doi.org/10.1074/jbc.M115.660498 [Google Scholar] [PubMed] [CrossRef]
Mizuno H, Kitada K, Nakai K, Sarai A (2009). PrognoScan: A new database for meta-analysis of the prognostic value of genes. BMC Medical Genomics 2: 18. https://doi.org/10.1186/1755-8794-2-18 [Google Scholar] [PubMed] [CrossRef]
Nobes CD, Hall A (1995). Rho, rac, and cdc42 GTPases regulate the assembly of multimolecular focal complexes associated with actin stress fibers, lamellipodia, and filopodia. Cell 81: 53–62. https://doi.org/10.1016/0092-8674(95)90370-4 [Google Scholar] [PubMed] [CrossRef]
Park S, Guo Y, Negre J, Preto J, Smithers CC, Azad AK, Overduin M, Murray AG, Eitzen G (2021). Fgd5 is a Rac1-specific Rho GEF that is selectively inhibited by aurintricarboxylic acid. Small GTPases 12: 147–160. https://doi.org/10.1080/21541248.2019.1674765 [Google Scholar] [PubMed] [CrossRef]
Rizvi S, Khan SA, Hallemeier CL, Kelley RK, Gores GJ (2018). Cholangiocarcinoma-evolving concepts and therapeutic strategies. Nature Reviews Clinical Oncology 15: 95–111. https://doi.org/10.1038/nrclinonc.2017.157 [Google Scholar] [PubMed] [CrossRef]
Shang S, Yang YW, Chen F, Yu L, Shen SH et al. (2022). TRIB3 reduces CD8+T cell infiltration and induces immune evasion by repressing the STAT1-CXCL10 axis in colorectal cancer. Science Translational Medicine 14: eabf0992. https://doi.org/10.1126/scitranslmed.abf0992 [Google Scholar] [PubMed] [CrossRef]
Siegel R, Ma J, Zou Z, Jemal A (2014). Cancer statistics, 2014. CA: A Cancer Journal for Clinicians 64: 9–29. https://doi.org/10.3322/caac.21208 [Google Scholar] [PubMed] [CrossRef]
Subramanian A, Tamayo P, Mootha VK, Mukherjee S, Ebert BL et al. (2005). Gene set enrichment analysis: A knowledge-based approach for interpreting genome-wide expression profiles. Proceedings of the National Academy of Sciences of the United States of America 102: 15545–15550. https://doi.org/10.1073/pnas.0506580102 [Google Scholar] [PubMed] [CrossRef]
Sun Y, Chen W, Torphy RJ, Yao S, Zhu G et al. (2021). Blockade of the CD93 pathway normalizes tumor vasculature to facilitate drug delivery and immunotherapy. Science Translational Medicine 13: eabc8922. https://doi.org/10.1126/scitranslmed.abc8922 [Google Scholar] [PubMed] [CrossRef]
Tang Z, Wang L, Bajinka O, Wu G, Tan Y (2022). Abnormal gene expression regulation mechanism of myeloid cell nuclear differentiation antigen in lung adenocarcinoma. Biology 11: 1047. https://doi.org/10.3390/biology11071047 [Google Scholar] [PubMed] [CrossRef]
Uhlén M, Fagerberg L, Hallström BM, Lindskog C, Oksvold P et al. (2015). Proteomics, tissue-based map of the human proteome. Science 347: 1260419. https://doi.org/10.1126/science.1260419 [Google Scholar] [PubMed] [CrossRef]
Valla M, Mjønes PG, Engstrøm MJ, Ytterhus B, Bordin DL, van Loon B, Akslen LA, Vatten LJ, Opdahl S, Bofin AM (2018). Characterization of FGD5 expression in primary breast cancers and lymph node metastases. Journal of Histochemistry and Cytochemistry 66: 787–799. https://doi.org/10.1369/0022155418792032 [Google Scholar] [PubMed] [CrossRef]
Viallard C, Larrivée B (2017). Tumor angiogenesis and vascular normalization: Alternative therapeutic targets. Angiogenesis 20: 409–426. https://doi.org/10.1007/s10456-017-9562-9 [Google Scholar] [PubMed] [CrossRef]
Warde-Farley D, Donaldson SL, Comes O, Zuberi K, Badrawi R et al. (2010). The GeneMANIA prediction server: Biological network integration for gene prioritization and predicting gene function. Nucleic Acids Research 38: W214–220. https://doi.org/10.1093/nar/gkq537 [Google Scholar] [PubMed] [CrossRef]
You H, Gao S, Xu X, Yuan H (2021). Faciogenital dysplasia 5 confers the cancer stem cell-like traits of gastric cancer cells through enhancing Sox2 protein stability. Environmental Toxicology 36: 2426–2435. https://doi.org/10.1002/tox.23355 [Google Scholar] [PubMed] [CrossRef]
Yu G, Wang LG, Han Y, He QY (2012). clusterProfiler: An R package for comparing biological themes among gene clusters. OMICS: A Journal of Integrative Biology 16: 284–287. https://doi.org/10.1089/omi.2011.0118 [Google Scholar] [PubMed] [CrossRef]
Cite This Article
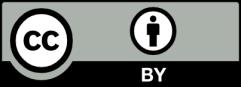
This work is licensed under a Creative Commons Attribution 4.0 International License , which permits unrestricted use, distribution, and reproduction in any medium, provided the original work is properly cited.