Open Access
ARTICLE
Analysis of functional hub genes indicates DLGAP5 is linked to lung adenocarcinoma prognosis
1 Department of Thoracic Surgery, Thoracic Cancer Center, The Sixth Affiliated Hospital, Sun Yat-sen University, Guangzhou, 510655, China
2 Department of Thoracic Surgery, The First Affiliated Hospital of Shantou University Medical College, Shantou, 515041, China
3 Department of Microbiology, Zhongshan School of Medicine, Key Laboratory for Tropical Diseases Control of the Ministry of Education, Sun Yat-sen University, Guangzhou, China
* Corresponding Authors: XIANYU QIN. Email: ; HONGYING LIAO. Email:
# These authors contributed equally to this work
BIOCELL 2023, 47(11), 2453-2469. https://doi.org/10.32604/biocell.2023.030032
Received 19 March 2023; Accepted 22 June 2023; Issue published 27 November 2023
Abstract
Introduction: The difficulty in treating lung adenocarcinoma (LUAD) is caused by a shortage of knowledge about the biological mechanisms and a lack of treatment choices. Objectives: The aim of this study was to identify a valuable molecular target for the treatment of LUAD. Methods: Using multiple databases, we screened for hub genes in LUAD using Cytoscape and explored the expression and prognosis of DLG associated protein 5 (DLGAP5) in LUAD. We investigated the genetic variation, functional enrichment, and epigenetic activity of DLGAP5. Furthermore, we evaluated the relationship between the tumor microenvironment (TME) and DLGAP5. Results: Our study identified 10 hub genes in LUAD: CDC45, KIAA0101, DLGAP5, CDT1, NCAPG, CCNB1, CDCA5, CDC20, KIF11, and AURKA. We discovered that DLGAP5 was overexpressed and associated with poor prognosis in LUAD. DLGAP5 exhibited an overall genetic variation frequency of 2%, and its DNA promoter was hypomethylated in LUAD (p < 0.05). The expression of DLGAP5 in LUAD showed a positive correlation with the majority of N6-methyladenosine (m6A)-methylation genes. Additionally, DLGAP5 was primarily associated with the cell cycle in LUAD. Notably, there was a significant favorable association between DLGAP5 and CD274, CTLA4, HAVCR2, and LAG3 in LUAD. Conclusion: DLGAP5 may be a therapeutic target for LUAD, as it affects cancer cells proliferation and development through the regulation of cell-cycle checkpoints and modulation of immune cell infiltration and immune checkpoints in the TME.Graphic Abstract
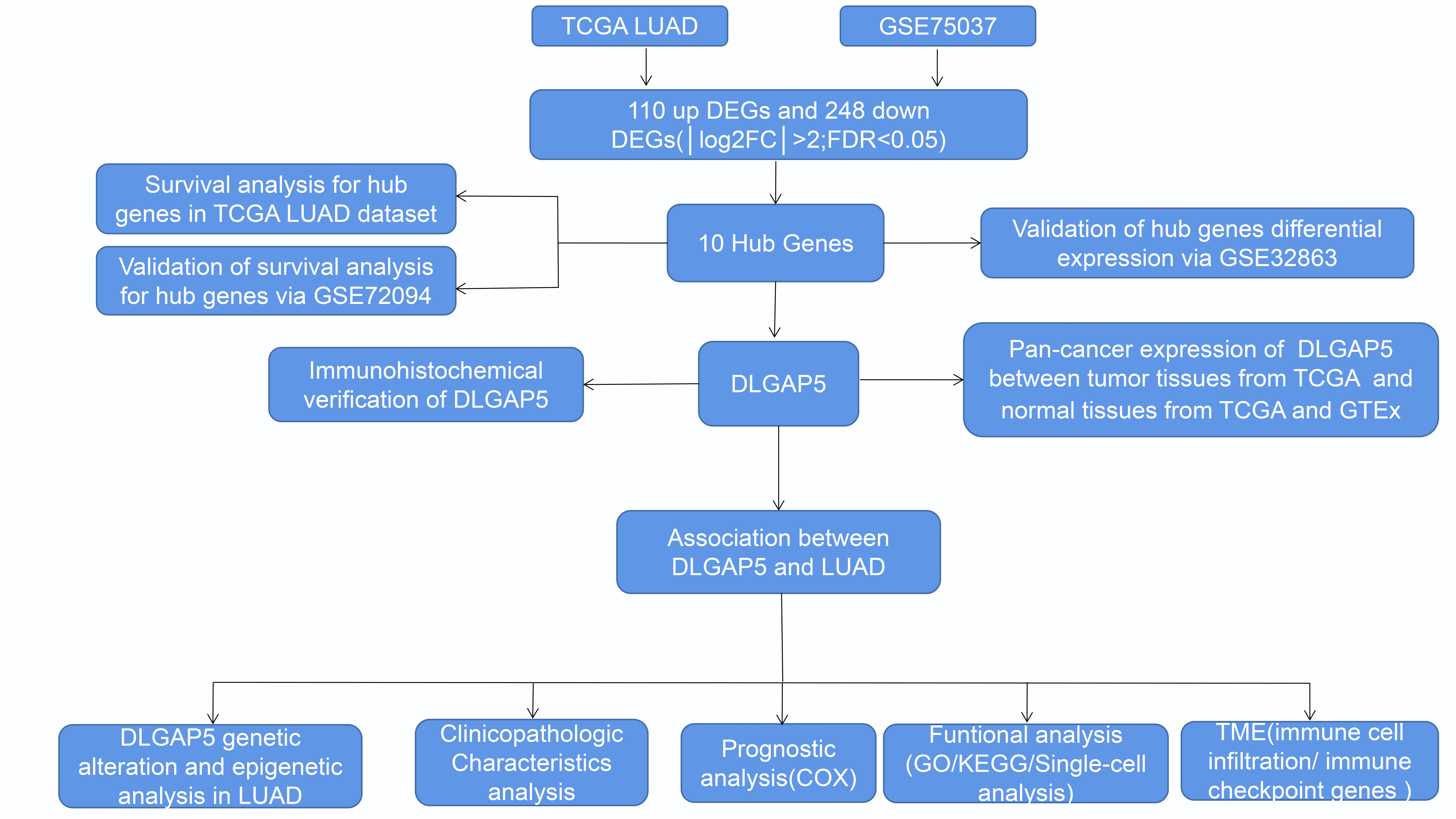
Keywords
Supplementary Material
Supplementary Material FileLung cancer, including small cell and non-small cell lung cancer, is one of the leading causes of death worldwide (Sung et al., 2021). Non-small cell lung cancer encompasses three pathological types: squamous cell carcinoma, adenocarcinoma, and large cell lung cancer. Among them, lung adenocarcinoma (LUAD) is the most prevalent type (Hirsch et al., 2017).
The prognosis of LUAD has significantly improved due to the development of various therapeutic approaches, including chemotherapy, surgery, targeted therapy, radiotherapy, and immunotherapy (Succony et al., 2021). Unfortunately, a poor 5-year overall survival rate is observed in LUAD patients, primarily because some are diagnosed at an advanced stage (Herbst et al., 2018). Hence, it is crucial to identify effective therapeutic targets and prognostic biomarkers.
DLGAP5, also known as DLG-associated protein 5, is a gene that codes for DLG7 protein. During mitosis, DLG7 plays an important role in spindle assembly, kinetochore fiber (K-fiber) stabilization, and chromosomal segregation (Hsu et al., 2004; Wong and Fang, 2006; Ye et al., 2011). The upregulation of DLGAP5 has been observed in colon cancer, breast cancer, prostate cancer, liver cancer, and endometrial cancer (Branchi et al., 2019; Chen et al., 2023; Horning et al., 2018; Tsou et al., 2003; Zhang et al., 2018). Further, high expression of DLGAP5 can confer resistance to G2-M arrest induced by a mitosis inhibitor and reduce the dependence of prostate cancer cell growth on the androgen receptor signaling pathway (Horning et al., 2018). Another study found that silencing DLGAP5 using siRNA significantly suppresses the proliferation and invasion of hepatocellular carcinoma cells (Liao et al., 2013). Similarly, Li et al. (2022) have demonstrated that DLGAP5 overexpression neutralizes the effects of miR-409-5p on SKOV-3 cell growth, G2/M arrest, and apoptosis. Given these studies, DLGAP5 overexpression may contribute to cancer development.
However, to date, there have been a limited number of systematic and comprehensive studies on the mechanism of DLGAP5 in LUAD. In our research, we utilized data from gene expression omnibus (GEO) and the cancer genome atlas (TCGA), in addition to our findings, to convincingly demonstrated that DLGAP5 expression is substantially upregulated in LUAD and is associated with poor prognosis. Our findings reveal the potential mechanism of DLGAP5 in LUAD and emphasize its impact on the tumor microenvironment (TME), providing valuable insights into a novel approach for antitumor interventions.
Data collection and processing
RNA sequencing data and clinical information were obtained from the GEO and TCGA databases. The inclusion criteria were as follows: (a) diagnosis of lung adenocarcinoma; (b) the dataset included more than 100 samples; (c) the cancer samples had clinical data including, at minimum, sample serial number, survival status, and survival time; (d) datasets containing paired samples (normal vs. tumor) could be used even if they lack clinical data. The exclusion criteria were as follows: (a) samples without essential clinical information; (b) samples with missing expression data for more than half of the genes. Suppl. Table S1 provides the essential details of the included datasets.
Screening of differentially expressed genes
The Limma package in R was used to identify differentially expressed genes (DEGs) between LUAD samples and normal samples in the GSE75037 and TCGA datasets. The threshold values of DEGs were set as |log2FC| ≥ 2 and false discovery rate (FDR) < 0.05. A Venn diagram was generated using Xiantao Academic Tools to visualize the common upregulated and downregulated DEGs.
Selection of hub genes and survival and expression analysis
The STRING database was utilized to examine functional protein association networks (Szklarczyk et al., 2021). The DEGs were first submitted to the STRING database. A combined score of higher than 0.4 was used to extract all protein–protein interactions (PPI) pairs. Nodes with the highest degree (0.900) were identified as essential for ensuring the overall stability of the network. The cytoHubba plugin for Cytoscape (v3.9.0) was used to determine the degrees of all nodes (Chin et al., 2014). The MCC algorithm of the Cytoscape cytoHubba application was employed to select hub genes. The Gene Expression Profiling Interactive Analysis (GEPIA) database was used for the overall survival analysis of the hub genes. At the same time, dataset GSE72094 was employed to validate the prognosis of the hub genes using the R software package ‘survival’. In addition, dataset GSE32863 was utilized to confirm the differential expression of the hub genes.
Clinicopathological characteristics and prognosis analysis of DLGAP5 in lung adenocarcinoma
The clinical data from the TCGA LUAD dataset was used to investigate the association between DLGAP5 expression and clinicopathological characteristics of LUAD patients. DLGAP5 expression levels were categorized into two groups using its median value in the TCGA LUAD dataset. The effects of DLGAP5 expression and related clinico-pathological factors on the survival of patients with LUAD were analyzed through univariate and multivariate Cox regression analysis.
Immunohistochemical validation and western blot analysis of DLGAP5 expression
To assess differences in DLGAP5 protein expression, immunohistochemical (IHC) images of DLGAP5 were obtained from the Human Protein Atlas (HPA). Furthermore, paraffin specimens of 30 LUAD samples were collected from the pathology department of the Sixth Affiliated Hospital, Sun Yat-sen University. Each sample included adjacent normal tissues and matched tumors. The IHC method was applied to evaluate DLGAP5 expression in LUAD and adjacent normal tissues. DLGAP5 antibody (Affinity Biosciences; DF6920) was utilized in the IHC procedure. Cell morphology and staining patterns were observed at low power (100×). Five random fields of view were selected and recorded. The results of the IHC were quantitatively analyzed and compared using Image J software and GraphPad Prism 8. In addition, we used western blotting to investigate the differences in DLGAP5 protein expression between A549 (lung adenocarcinoma cells) and BEAS-2B (lung epithelial cells) cells.
DLGAP5 expression and survival analysis in a pan-cancer dataset
The standardized pan-cancer dataset, which included expression profile data and clinical information, was downloaded from the University of California Santa Cruz (UCSC) database. The expression data of DLGAP5 in samples was extracted and transformed using the log2 (x + 0.001) function. Cancer types with fewer than 3 samples were excluded, and duplicate data was removed. Subsequently, the expression and clinical data of 33 tumor types were collected. R software was used to analyze the difference between normal and tumor tissues and generate box plots for each tumor. In addition, the relationship between DLGAP5 expression and prognosis in each tumor was analyzed using the log-rank method.
Genetic alteration and epigenetic analysis of DLGAP5 in lung adenocarcinoma
The open-access tool c-BioPortal provides access to 225 cancer studies, enabling users to analyze, visualize, and explore multidimensional cancer genome data. DLGAP5 gene mutations were investigated using c-BioPortal.UALCAN is an interactive online tool that offers detailed multi-omics information on several cancers. We utilized UALCAN to assess the promoter methylation ofDLGAP5. Moreover, we compiled a list of 21 genes associated with RNA m6A methylation from previous research (Jiang et al., 2021). From the TCGA pan-cancer dataset, we extracted the expression data for DLGAP5 and 21 RNA m6A-methylation-related genes. Thereafter, we investigated the relationship between DLGAP5 and the genes associated with RNA m6Amethylation. The findings were visualized and presented in the form of a heatmap.
Functional enrichment analysis of DLGAP5
The transcriptome data of the TCGA LUAD dataset were divided into two groups, high and low, based on the median value of DLGAP5 expression. Differential expression analysis was performed using the “Limma” package in R, selecting genes with |log2FC| ≥ 1 and FDR < 0.05. DEGs related to DLGAP5 were further screened using a threshold of qvalue < 0.05, p.adj < 0.05, and count ≥ 2. Gene Ontology (GO) and Kyoto Encyclopedia of Genes and Genomes (KEGG) analysis of these DLGAP5-related DEGs were conducted using the “ClusterProfiler” package in R. Finally, the outcomes of the enrichment analysis were visualized using a dot plot.
Single-cell analysis of DLGAP5 in lung adenocarcinoma
The CancerSEA is a specialized database, which aims to comprehensively elucidate various functional conditions of tumor cells at the single-cell level (Yuan et al., 2019). The database contains an atlas of single-cell functional states, encompassing data from 41,900 tumor single cells across 25 different tumor types and a total of 280 different cell types. The database provides information on 14 different functional statuses, including cell cycle, epithelial-mesenchymal transition (EMT), angiogenesis, inflammation, apoptosis, DNA damage, metastasis, stemness, DNA repair, hypoxia, invasion, differentiation, proliferation, and quiescence. In our study, we investigated the functional state of DLGAP5 in LUAD using the CancerSEA database.
Association between DLGAP5 expression and tumor cell infiltration and immunotherapy prediction in lung adenocarcinoma
The TME plays a crucial role in tumor formation and progression. In this study, we employed two methods, namely the Estimation of STromal and Immune cells in MAlignant Tumor tissues using Expression data (ESTIMATE) score and Microenvironment Cell Populations (MCP)-counter score, to evaluate the association between DLGAP5 and tumor immune cell infiltration in the TCGA LUAD cohort using the SangerBox online tool. The Wilcoxon signed-rank test was utilized to compare the immune infiltration levels between low-DLGAP5 and high-DLGAP5 groups. Additionally, to predict the potential response to immunotherapy in patients with various levels of DLGAP5, the Tumor Immune Dysfunction and Exclusion (TIDE) algorithm was applied to the TCGA LUAD cohort.
Correlation between DLGAP5 and immune check point genes in pan-cancer
Based on the previous research (Thorsson et al., 2018), we compiled 60 immune checkpoint (ICP) genes, consisting of 36 immune stimulators and 24 immune inhibitors. We investigated the relationship between ICP genes and DLGAP5 expression using the SangerBox tools. The results were visualized and presented as a heat map.
The statistical analysis was carried out using the R version 4.1.0. Survival analysis was conducted using the Log-rank test and the Kaplan-Meier method. Cox regression analysis was utilized for both univariate and multivariate analysis. The Student’s t-test or the Wilcoxon rank sum test was utilized to compare continuous variables, as appropriate. The Fisher’s exact test or Chi-square test was applied to compare categorical clinicopathological variables. Spearson correlation was applied for correlation analysis. Significance levels were indicated as follows: ns, not significant, p ≥ 0.05; *p < 0.05; **p < 0.01; ***p < 0.001; ****p < 0.0001.
Screening of differentially expressed genes
To identify key genes related to LUAD, DEGs between normal tissues and LUAD were analyzed using the GSE75037 dataset from the GEO database and TCGA LUAD-related datasets. In the GSE75037 dataset, 229 genes were upregulated and 458 genes were downregulated in LUAD compared to normal tissues. In the TCGA LUAD dataset, 226 genes were upregulated and 435 genes were downregulated (FDR < 0.05, |log2FC| > 2) (Figs. 1A and 1B). To identify commonly differentially expressed genes, a Venn diagram was used to visualize the overlap between the two datasets. Among these two datasets, 110 genes were upregulated and 248 genes were downregulated (Figs. 1C and 1D).
Figure 1: Identification of differentially expressed genes (DEGs) based on The Cancer Genome Atlas (TCGA) and GSE75037 datasets. The volcano plot depicts the 687 DEGs (|log2FC| > 2; false discovery rate (FDR) < 0.05) in GSE 75037 lung adenocarcinoma (LUAD) tissues vs. normal lung samples (A); The volcano plot depicts the 661 DEGs (|log2FC| > 2; FDR < 0.05) in TCGA LUAD tissues vs. normal lung samples (B); 110 upregulated DEGs between TCGA and GSE75037 datasets (C); 248 downregulated DEGs between TCGA and GSE75037 datasets (D).
Protein-protein interaction network construction and hub gene screening
To explore the relationship among the DEGs, the STRING database was used to construct a protein-protein interaction (PPI) network (Suppl. Fig. S1A). The MCC algorithm was used in cytoHubba to identify the most significant nodes in the PPI network, resulting in the extraction of the top 10 central nodes (Suppl. Fig. S1B). The hub genes identified were CDC45, KIAA0101, DLGAP5, CDT1, NCAPG, CCNB1, CDCA5, CDC20, KIF11, and AURKA.
Validation of prognostic values and the expression patterns of hub genes
The GEPIA database was employed to investigate the prognostic utility of identified hub genes. By setting the median expression of the hub genes as the cut-off point, it was observed that the low-expression group had better overall survival compared with the high-expression group (p < 0.05) (Figs. 2A–2J). The survival analysis was further validated using dataset GSE72094, which yielded consistent results (Suppl. Fig. S2). To further explore the differential expression of hub genes, another dataset, GSE32863, was analyzed, which also demonstrated significant upregulation of these hub genes in tumor tissues (p < 0.05) (Fig. 2K). Taken together, these results suggest that these hub genes may play a crucial role in the development of LUAD.
Figure 2: Validation of the expression comparison and prognostic values of hub genes. Survival analysis of hub genes using the Gene Expression Profiling Interactive Analysis (GEPIA) database (A–J); The expression comparison of hub genes in GSE32863 (K) (****p < 0.0001).
Analysis of clinicopathologic characteristics of DLGAP5 in lung adenocarcinoma
To further explore the potential clinical significance of DLGAP5 in LUAD, we conducteda differential analysis of DLGAP5 expression for various clinicopathologic characteristics using TCGA LUAD samples. The findings revealed that DLGAP5 expression was substantially upregulated in males. DLGAP5 expression was lower in the group aged over 65 compared with the group aged 65 or below. Futher, DLGAP5 expression was higher in the T2 group compared with the T1 group. Moreover, DLGAP5 expression was higher in the N1, N2, and N3 groups compared to the N0 group. Lastly, the expression levels of DLGAP5 in Stage III and Stage IV groups were higher than those in Stage I and Stage II groups (Suppl. Figs. S3A–S3F). These findings suggest that the expression levels of DLGAP5 in LUAD may increase as the disease progresses.
Furthermore, we conducted univariate logistic regression analysis to investigate the relationship between DLGAP5 and the clinicopathologic variables (Table 1).
High DLGAP5 expression was positively associated with the invasion depth (T2, T3, and T4 vs. T1; OR, 1.982; 95% CI, 1.374–2.874; p < 0.001), lymph node metastasis (N1, N2, and N3 vs. N0; OR, 2.164; 95% CI, 1.489–3.165; p < 0.001), pathologic stage (Stage III, Stage IV vs. Stage I and Stage II; OR, 2.093; 95% CI, 1.361–3.257; p < 0.001), gender (male vs. female; OR, 1.685; 95% CI, 1.198–2.378; p = 0.003), and age (more than 65 years vs. 65 years or lower; OR, 0.689; 95% CI, 0.486–0.974; p = 0.035). Collectively, these findings suggest that DLGAP5 may function as an oncogene in LUAD.
Prognostic analysis of DLGAP5 in lung adenocarcinoma
To investigate the independent prognostic role of DLGAP5 in LUAD, we conducted a multivariate Cox regression analysis. Table 2 presents the baseline characteristics of the patients. Compared with the low-expression DLGAP5 group, the high-expression DLGAP5 group had a higher proportion of younger patients, males, positive lymph node metastasis, and distant metastasis. The multivariate Cox regression analysis demonstrated that DLGAP5 expression, T stage, N stage, and M stage were independent prognostic factors of overall survival (OS) in LUAD (Table 3). These results indicate that DLGAP5 may serve as a prognostic biomarker for LUAD.
Differential expression and prognosis analysis of DLGAP5 in the pan-cancer dataset
To evaluate the significance of DLGAP5 in tumorigenesis, we performed a pan-cancer differential expression analysis comparing DLGAP5 expression between tumor tissues and normal tissues. DLGAP5 expression was higher in cancer tissues compared with normal tissues in 30 tumor types (p < 0.05) (Fig. 3A). In addition, we performed a pan-cancer prognosis analysis of DLGAP5. There were 12 tumor types, including Brain Lower Grade Glioma (LGG), Kidney renal papillary cell carcinoma (KIRP), Adrenocortical carcinoma (ACC), Liver hepatocellular carcinoma (LIHC), Kidney Chromophobe (KICH), LUAD, Mesothelioma (MESO), Pancreatic adenocarcinoma (PAAD), Kidney renal clear cell carcinoma (KIRC), Uveal Melanoma (UVM), Sarcoma (SARC), and Bladder Urothelial Carcinoma (BLCA), where high DLGAP5 expression was associated with poor prognosis (Fig. 3B). These results suggest that DLGAP5 may have a universal impact on the development and progression of cancers.
Figure 3: Differential expression (A) and prognosis analysis (B) of DLGAP5 in pan-cancer (*p < 0.05; ***p < 0.001).
Immunohistochemical validation and western blot analysis for the expression of DLGAP5 in lung adenocarcinoma
Previous studies have shown that DLGAP5 is upregulated at the mRNA level in LUAD. To determine whether DLGAP5 is also upregulated at the protein level, we performed further investigation. Analysis of the HPA database revealed that DLGAP5 was mainly expressed in the cytoplasm. DLGAP5 expression in LUAD tissues was predominantly strong or medium (Figs. 4A and 4B). In contrast, the expression of DLGAP5 in normal tissues was either weak or undetectable. To verify DLGAP5 protein expression, IHC staining was performed using 30 pairs of samples collected from the Sixth Affiliated Hospital, Sun Yat-sen University. Representative images from three patients are displayed in Figs. 4C–4E. DLGAP5 was found to be accumulated in LUAD tissues, whereas its expression in normal tissues was weak or absent. We found that DLGAP5 expression was substantially higher in LUAD tissues compared with normal tissues by randomly selecting five fields of view from each sample and using Image J to calculate the average optical density (AOD) (Fig. 4F). Furthermore, we analyzed the expression of DLGAP5 between A549 and BEAS-2B cells using western blotting. We found that DLGAP5 documented a significantly higher expression in A549 compared to BEAS-2B (Figs. 4G and 4H).
Figure 4: Immunohistochemical validation and western blot analysis for the expression of DLGAP5 in LUAD. Immunohistochemical staining analysis of DLGAP5 in LUAD from the Human Protein Atlas (HPA) dataset (A, B); Typical representatives of immunohistochemical data of DLGAP5 in paired LUAD samples (C, D, E); The statistical results of the differential expression of DLGAP5 in 30 paired LUAD samples (F) (***p < 0.001); DLGAP5 protein expression was explored by Western blot analysis and semiquantitative analysis of data for each group, n = 3/group (G, H) (***p < 0.001).
Genetic alteration and epigenetic analysis of DLGAP5 in lung adenocarcinoma
One of the crucial factors influencing gene expression is genetic alteration (Matharu and Ahituv, 2020). Hence, we explored the genetic alteration status of DLGAP5 by utilizing the cBioPortal database, which included a total of 11 datasets comprising 3745 patients with LUAD. The OncoPrintanalysis revealed that genomic alterations in DLGAP5 were present in 2% of patients (Fig. 5B). The mutation frequencies of DLGAP5 varied across different datasets, with 3.25% in the Broad dataset (Imielinski et al., 2012), 2.6% in the OncoSG dataset (Chen et al., 2020), and 2.5% in the TCGA dataset (Firehose Legacy) (Fig. 5A). Further examination demonstrated that the DLGAP5 gene exhibited eight mutation sites (Fig. 5C).
Figure 5: DLGAP5 genomic alterations in LUAD analyzed in the cBioPortal database and ULACAN database. The alteration types and frequency of DLGAP5 from different LUAD studies (A); OncoPrint of DLGAP5 gene alterations in the cancer cohort (different colors means different types of genetic alterations) (B); Mutation sites of the DLGAP5 gene in LUAD (C). The promoter methylation level of in LUAD (*p < 0.05) (D).
Gene expression is regulated by various epigenetic modifications, including DNA promoter methylation and RNA m6A methylation, which play a role in the development and progression of cancers (Chen et al., 2017). In this study, we utilized the UALCAN database to investigate the promoter methylation level of the DLGAP5 gene in LUAD tumor tissues compared with normal tissues. The result indicated that the methylation of the DLGAP5 promoter in LUAD tumor tissues was markedly lower than that in normal tissues (p = 0.031) (Fig. 5D). Furthermore, we investigated the relationship between DLGAP5 and m6A-methylation-related genes in LUAD. The analyses showed that the high-DLGAP5-expression group exhibited higher expression levels of the following genes: RBM15B, METTL14, WTAP, ZC3H13, VIRMA, YTHDF2, CBLL1, RBM15, YTHDC1, YTHDF3, IGF22BP1, YTHDF1, HNRNPA2B1, HNRNPC, FMR1, LRPPRC, and ELAVL1 (Fig. 6A). Then, we performed a correlation analysis and found significant positive correlations between DLGAP5 and several m6A-methylation-related genes, including YTHDF1 (r = 0.219, p < 0.001), YTHDF3 (r = 0.224, p < 0.001), HNRNPA2B1 (r = 0.396, p < 0.001), IGF2BP1 (r = 0.392, p < 0.001), ELAVL1 (r = 0.428, p < 0.001), LRPPRC (r = 0.490, p < 0.001), RBM15B (r = 0.148, p < 0.001), FMR1 (r = 0.197, p < 0.001), RBM15 (r = 0.325, p < 0.001), WTAP (r = 0.355, p < 0.001), CBLL1 (r = 0.383, p < 0.001), VIRMA (r = 0.424, p < 0.001), and HNRNPC (r = 0.519, p < 0.001) (Figs. 6B–6R). These findings suggest that RNA m6A methylation and DNA promoter methylation may play a crucial role in the epigenetic regulation of DLGAP5 in LUAD.
Figure 6: Relationship between DLGAP5 expression and RNA m6A-methylation-related genes in LUAD. High-DLGAP5 expression group with higher expression of METTL14, RBM15, RBM15B, WTAP, VIRMA, CBLL1, ZC3H13, YTHDC1, YTHDF1, YTHDF2, YTHDF3, IGF22BP1, HNRNPA2B1, HNRNPC, FMR1, LRPPRC, ELAVL1 (A); DLGAP5 expression has positive correlation with YTHDF1, YTHDF3, HNRNPA2B1, IGF2BP1, ELAVL1, LRPPRC, RBM15B, FMR1, RBM15, WTAP, CBLL1, VIRMA, and HNRNPC (B–R) (ns, p ≥ 0.05; *p < 0.05; **p < 0.01; ***p < 0.001).
Functional enrichment analysis of DLGAP5
To gain insights into the underlying mechanisms by which DLGAP5 contributes to the poor prognosis of LUAD, we conducted GO/KEGG analysis and identified a total of 442 DEGs, including 260 downregulated genes and 182 upregulated genes (Fig. 7A). The top 50 upregulated and downregulated DEGs were used to generate a heat map (Fig. 7B). Using the criteria p.adj < 0.05, qvalue < 0.05, and count ≥ 2, we analyzed DLGAP5-related DEGs and found that they were involved in 415 biological processes, 92 cell components, 51 molecular functions, and 11 KEGG pathways. The top 10 items for KEGG, molecular functions, cell components, and biological processes were displayed in a bubble graph (Figs. 7C–7F). GO analysis revealed that DLGAP5-related DEGs were primarily associated with cell cycle, chromosome, carbohydrate derivative binding, and other biological processes. KEGG pathway analysis revealed their significant connections to cellular senescence, oocyte meiosis, cell cycle, and other pathways. Moreover, utilizing the single-cell CancerSEA database, we investigated the association between DLGAP5 and 14 functional states in the pan-cancer. The analyses revealed significantly strong positive correlations between DLGAP5 and cell cycle (r = 0.71, p = 0.00), invasion (r = 0.67, p = 0.00), DNA repair (r = 0.56, p = 0.00), proliferation (r = 0.56, p = 0.00), DNA damage (r = 0.54, p = 0.00), EMT (r = 0.48, p = 0.00), and metastasis (r = 0.45, p = 0.00) (Figs. 8A–8G). Taken together, these findings provide further insight into the functional implications of DLGAP5 in LUAD and its association with critical processes involved in cancer progression.
Figure 7: Identification and functional analysis of DLGAP5-related differentially expressed genes (DEGs). The volcano plot depicts the 442 DEGs (|log2FC| > 1; false discovery rate (FDR) < 0.05) in the TCGA LUAD dataset of high vs. low expression of DLGAP5 (A); The heatmap shows the top 50 upregulated and downregulated DEGs (B); Gene Ontology (GO) functional enrichment analysis of DEGs (C–E); Kyoto Encyclopedia of Genes and Genomes (KEGG) functional enrichment analysis of DEGs in TCGA LUAD data (F).
Figure 8: Correlation analysis between DLGAP5 and functional status of cancer cells by using the CancerSEA database. The correlation between DLGAP5 and the functional status of cancer cells (cell cycle, invasion, DNA repair, proliferation, DNA damage, epithelial-mesenchymal transition (EMT), and Metastasis) (A–G) (***p < 0.001).
The correlation between DLGAP5 expression and immune cell infiltration, ICP genes, and immunotherapy response in lung adenocarcinoma
We employed two algorithms, the ESTIMATE score and MCP-counter score to evaluate the relationship between tumor immune infiltration and DLGAP5 expression in the TCGA LUAD dataset using Sangerbox tools. In the ESTIMATE score analysis, the results showed that the high-DLGAP5-expression group had a significantly lower Stromal Score (p < 0.05), Immune Score (p < 0.05), and Estimate Score (p < 0.05) compared with the low-DLGAP5-expression group (Figs. 9A–9C). The MCP-counter score analysis showed that the high-DLGAP5-expression group exhibited higher levels of CD8_T_cells (p < 0.001), higher levels of cytotoxic_lymphocytes (p < 0.001), lower levels of B_lineage (p < 0.001), higher levels of NK_cells (p < 0.0001), higher levels of monocytic_lineage (p < 0.01), lower levels of myeloid_dendritic_cells (p < 0.0001), lower levels of neutrophils (p < 0.0001), lower levels of endothelial_cells (p < 0.0001), and higher levels of fibroblasts (p < 0.001) compared with the low-DLGAP5-expression group (Fig. 9D).
Figure 9: The correlation between DLGAP5 and the immune cell infiltration of the tumor microenvironment (TME). Immune cell infiltration of TME was evaluated by the Estimation of STromal and Immune cells in MAlignant Tumor tissues using Expression data (ESTIMATE) algorithm (A–C) (*p < 0.05); Immune cell infiltration of TME was evaluated by the Microenvironment Cell Populations (MCP) Counter algorithm (D) (−p ≥ 0.05; **p < 0.01; ***p < 0.001; ****p < 0.0001).
ICP genes affect the proliferation and development of cancer cells by regulating immune surveillance (Muenst et al., 2016). To probe the potential role of DLGAP5 in immunotherapy, we examined the relationships between DLGAP5 expression and two main categories of immune modulators in human cancers, revealing a correlation between DLGAP5 and immune modulators across various cancer types. We discovered that DLGAP5 expression showed positive correlations with the majority of immune inhibitors and immune stimulators in Glioma (GBMLAA), LGG, Pan-kidney cohort (KIPAN), KIRC, Thyroid carcinoma (THCA), UVM, Lymphoid Neoplasm Diffuse Large B-cell Lymphoma (DLBC), LIHC, MESO, LUAD, BLCA, Breast invasive carcinoma (BRCA), Prostate adenocarcinoma (PRAD), Colon adenocarcinoma (COAD), and Colon adenocarcinoma/Rectum (COADREAD). Conversely, the DLGAP5 expression exhibited negative correlations with immune inhibitors and immune stimulators in Thymoma (THYM), Glioblastoma multiforme (GBM), Lung squamous cell carcinoma (LUSC), and Cervical squamous cell carcinoma (CESC). Notably, in LUAD, DLGAP5 showed a strikingly strong positive correlation with CD274 (PD-L1), LAG3, HAVCR2, and CTLA4 (Fig. 10A). Furthermore, we utilized the TIDE database to evaluate the effect of DLGAP5 on the immunotherapy response in LUAD. The results revealed that patients with higher DLGAP5 expression in the TCGA cohort exhibited poorer responses to immunotherapy (Figs. 10B and 10C).
Figure 10: Correlation between DLGAP5 and with immune checkpoint (ICP) genes (A) and immunotherapy response (B, C) (*p < 0.05; ****p < 0.0001).
A comprehensive understanding of the pathogenesis of LUAD and the discovery of promising valuable biomarkers are crucial for identifying potential therapeutic targets and improving patient outcomes. The findings of our research suggest that DLGAP5 may serve as a promising target for the treatment and prognosis of LUAD. A previous report has shown that DLGAP5 has a prognostic value in non‑small cell lung cancer (Wang et al., 2018), which is consistent with our findings, supporting the significance of DLGAP5 in lung cancer. However, our study goes beyond previous research by providing a comprehensive exploration of the role of DLGAP5 in LUAD, including the analysis of its association with genes involved in m6A modifications and ICP.
Based on a comprehensive analysis of expression and survival, we identified 10 hub genes (CDC45, KIAA0101, DLGAP5, CDT1, NCAPG, CCNB1, CDCA5, CDC20, KIF11, and AURKA) that might be associated with LUAD progression. Notably, most of these genes have been studied in the context of LUAD, further supporting their potential significance in LUAD. For example, Huang et al. (2019) identified CDC45 as an oncogene for lung cancer. Further, KIAA0101 has been characterized as a prognostic marker in LUAD, affecting the regulation of tumor immune infiltration (Hu et al., 2020). Cao et al. (2021) have found that lung cancer cell proliferation, viability, and cisplatin resistance are inhibited by CDT1. Sun et al. (2022) identified NCAPG as an important component in NSCLC oncogenesis and progression, providing a potential target for NSCLC treatment and prognosis prediction. Another study discovered that CCNB1 upregulation facilitates the progression of LUAD cells, which may be negatively regulated by miR-139-5p (Bao et al., 2022). Li et al. (2021) discovered that KIF11 is critical for the proliferation of LUAD cancer cells and has potential as a promising therapeutic target and independent prognostic factor for LUAD patients. Another team demonstrated that AURKA activity is necessary for epidermal growth factor receptor (EGFR)-mutant lung adenocarcinoma cells to develop resistance in response to EGFR inhibitors (Shah et al., 2019). Furthermore, Shen et al. (2022) found that silencing the oncogene CDCA5 induces apoptosis and G1 phase arrest in LUAD cells via the p53-p21 signaling pathway. Notwithstanding these findings, the underlying mechanism of DLGAP5 in LUAD has seldom been analyzed comprehensively.
In our study, we found that DLGAP5 expression was upregulated in LUAD, as verified by our own samples in the IHC and western blot experiments. Besides, we observed that DLGAP5 expression, along with T, N, and M stages had independent prognostic effects on the survival of LUAD patients. Furthermore, the results of pan-cancer analysis revealed differential expression of DLGAP5 in 30 types of common tumors, with 12 of them exhibiting high DLGAP5 expression and poor prognosis. Interestingly, DLGAP5 was shown to be associated with the prognosis of some kinds of patients, including breast cancer, colorectal cancer, endometrial cancer, liver cancer, and prostate cancer. These findings, combined with our data, indicate the possibility of a universal mechanism through which DLGAP5 influences tumor growth.
To study the reason behind the high expression of DLGAP5 in LUAD, we utilized the c-BioPortal database to explore the mutation profile of DLGAP5. Interestingly, we found that the mutation rate of in LUAD was only 2%, which does not fully account for its high expression in LUAD. In addition, we found hypomethylation in the promoter region of DLGAP5 in LUAD tumor tissues compared with normal tissues, which can partially explain the elevated mRNA levels of DLGAP5in LUAD tumors. RNA m6A methylation plays a crucial role in the control of RNA expression. Therefore, we conducted a correlation analysis between DLGAP5 and m6A methylation-related genes, discovering that they were strongly correlated in LUAD. This suggested that the mechanism of m6A methylation may be crucial in regulating DLGAP5 expression in LUAD. Another study reported that the m6A methylation regulator heterogeneous nuclear ribonucleoprotein C (HNRNPC) promotes the growth and metastasis of non-small cell lung cancer through its regulation of DLGAP5 (Shi et al., 2022), which supports our findings. There may be additional mechanisms, such as chromosomal histone modification or RNA m1A/m5C modification, that contribute to the upregulation of DLGAP5 in LUAD. However, these potential mechanisms were not investigated given the scope of this study.
To further study the molecular mechanisms of DLGAP5 in LUAD, we conducted GO and KEGG analyses. These analyses revealed several signaling pathways related to LUAD proliferation, invasion, and metastasis, including the cell cycle, cell senescence, the p53 signaling pathway, and DNA replication. Interestingly, these pathways have been studied in LUAD. For instance, Kim et al. (2021) have shown that PAF restructures the dimerization partner, RB-like, E2F and multi-vulval class B (DREAM) complex to promote lung tumorigenesis by circumventing cell quiescence. Another report found that inhibition of dyskerin pseudouridine synthase 1 (DKC1) induced telomere-related cell senescence and apoptosis in LUAD (Kan et al., 2021). Additionally, Wang et al. (2021) reported that the autophagy-p53-Zeb1 axis can be utilized by cancer stem cells for self-renewal, oncogenesis, and development. Furthermore, another study found that DDX59 promotes DNA replication in LUAD (You et al., 2017). These findings highlight the significance of the aforementioned signaling pathways in LUAD and provide new directions for further research. In our study, we employed the CancerSEA to investigate the function of DLGAP5 in LUAD at the single-cell analysis level. We discovered a significant association between DLGAP5 and the cell cycle (r = 0.71, p < 0.001), which corroborates the findings of the GO and KEGG bulk analyses. These results support the notion that DLGAP5 influences LUAD growth primarily by affecting cell cycle regulation.
The TME is essential for promoting tumor growth, invasiveness, and metastasis (Hernández-Camarero et al., 2021). We utilized the ESTIMATE score and MCP-counter score to evaluate the involvement of DLGAP5 in the TME of LUAD. Our results showed that the high-DLGAP5-expression group had a lower Stromal Score, lower Immune Score, and lower Estimate Score, indicating higher tumor purity. These findings indicate that DLGAP5 may affect immune cell infiltration in the TME. Feng et al. (2021) also reported a positive correlation between elevated DLGAP5 expression and immune infiltration level in clear cell renal cell carcinoma, which supports our findings. Another study found that DLGAP5 expression has a positive correlation with Th2 cells and a negative correlation with NK cells in bladder cancer (Rao et al., 2022). Moreover, we investigated the association between ICP genes and DLGAP5 expression and found a strikingly positive correlation between DLGAP5 and CD274 (PD-L1), LAG3, HAVCR2, and CTLA4. These genes are among the biomarkers of T-cell exhaustion (Wherry, 2011), which refers to the impairment or loss of T-cell functions in patients with chronic infections or cancer. Hence, the expression of DLGAP5 may contribute to T-cell exhaustion within the TME in LUAD. Lastly, we used the TIDE database to evaluate the impact of DLGAP5 on the efficacy of immunotherapy in LUAD. Our analysis revealed that patients with high DLGAP5 expression exhibited poorer responses to immunotherapy. Collectively, these findings suggest that DLGAP5 could be a valuable immunotherapeutic target for LUAD.
Our study has some limitations that should be noted before making broad conclusions. First, this work relied primarily on bioinformatics analysis. Therefore, further in vivo and in vitro studies are needed to validate the potential role of DLGAP5 in LUAD. Second, this research utilized retrospective data, which may introduce potential biases and confounding factors. Thus, future prospective studies are warranted to verify our findings. Third, the lack of clinical trials evaluating anti-DLGAP5 therapeutic monoclonal antibodies is a limitation. As a result, the absence of concrete examples and clinical data hinders our ability to demonstrate the efficacy of anti-DLGAP5 drugs in prolonging survival in LUAD patients.
In summary, our research findings indicate that DLGAP5 is upregulated in LUAD, and its expression level is associated with the prognosis of LUAD patients. DLGAP5 seems to exert significant effects on LUAD cell proliferation and invasion through various mechanisms, including regulation of cell-cycle checkpoints and modulation of immune cell infiltration and immune checkpoints in the TME. These findings suggest that DLGAP5 holds promise as a therapeutic target and potential prognostic biomarker for LUAD. Further in vitro and in vivo studies are necessary to confirm our observations and elucidate the precise role of DLGAP5 in LUAD.
Acknowledgement: We would like to thank Xiantao Academic and Sangerbox Tools for their technical assistance with our bioinformatics analysis.
Funding Statement: The study was funded by the supporting funds for scientific research of the Sixth Affiliated Hospital, Sun Yat-sen University (P20200217202404781).
Author Contributions: The authors confirm contribution to the paper as follows: Conceptualization, HZ, and RL; methodology, HZ, and RL; software, WC and YZ; validation, XY, HZ, and XQ; formal analysis, HZ, RL, and YC; investigation, ZL; resources, FQ; data curation, HL; writing—original draft preparation, HZ; writing—review and editing, HZ and HL; visualization, YC; supervision, XQ; funding acquisition, HL. All authors reviewed the results and approved the final version of the manuscript.
Availability of Data and Materials: We used publicly available datasets in this study. The TCGA LUAD cohort is available at https://portal.gdc.cancer.gov; GSE75037, GSE32863, and GSE72094 datasets from the GEO database are available at https://www.ncbi.nlm.nih.gov/geo. The original contributions of the study are represented in the article. The datasets generated during and/or analyzed during the current study are available from the corresponding author on reasonable request.
Ethics Approval: The clinical research ethics committee of the Sixth Affiliated Hospital of Sun Yat-sen University reviewed and approved the study involving human participants (2022ZSLYEC-514). The research was carried out in accordance with the principles of the Helsinki Declaration (1964).
Conflicts of Interest: The authors declare that they have no conflicts of interest to report regarding the present study.
Supplementary Materials: The supplementary materials are available online at https://doi.org/10.32604/biocell.2023.030032.
References
Bao B, Yu X, Zheng W (2022). MiR-139-5p targeting CCNB1 modulates proliferation, migration, invasion and cell cycle in lung adenocarcinoma. Molecular Biotechnology. https://doi.org/10.1007/s12033-022-00465-5 [Google Scholar] [PubMed] [CrossRef]
Branchi V, García SA, Radhakrishnan P, Győrffy B, Hissa B, Schneider M, Reißfelder C, Schölch S (2019). Prognostic value of DLGAP5 in colorectal cancer. International Journal of Colorectal Disease 34: 1455–1465. https://doi.org/10.1007/s00384-019-03339-6 [Google Scholar] [PubMed] [CrossRef]
Cao Y, Li P, Wang H, Li L, Li Q (2021). SIRT3 promotion reduces resistance to cisplatin in lung cancer by modulating the FOXO3/CDT1 axis. Cancer Medicine 10: 1394–1404. https://doi.org/10.1002/cam4.3728 [Google Scholar] [PubMed] [CrossRef]
Chen Y, Hong T, Wang S, Mo J, Tian T, Zhou X (2017). Epigenetic modification of nucleic acids: From basic studies to medical applications. Chemical Society Reviews 46: 2844–2872. https://doi.org/10.1039/C6CS00599C [Google Scholar] [PubMed] [CrossRef]
Chen R, Liu J, Hu J, Li C, Liu Y, Pan W (2023). DLGAP5 knockdown inactivates the Wnt/β-catenin signal to repress endometrial cancer cell malignant activities. Environmental Toxicology 38: 685–693. https://doi.org/10.1002/tox.23720 [Google Scholar] [PubMed] [CrossRef]
Chen J, Yang H, Teo ASM, Amer LB, Sherbaf FG et al. (2020). Genomic landscape of lung adenocarcinoma in East Asians. Nature Genetics 52: 177–186. https://doi.org/10.1038/s41588-019-0569-6 [Google Scholar] [PubMed] [CrossRef]
Chin CH, Chen SH, Wu HH, Ho CW, Ko MT, Lin CY (2014). cytoHubba: Identifying hub objects and sub-networks from complex interactome. BMC Systems Biology 8: S11. https://doi.org/10.1186/1752-0509-8-S4-S11 [Google Scholar] [PubMed] [CrossRef]
Feng Y, Li F, Yan J, Guo X, Wang F et al. (2021). Pan-cancer analysis and experiments with cell lines reveal that the slightly elevated expression of DLGAP5 is involved in clear cell renal cell carcinoma progression. Life Sciences 287: 120056. https://doi.org/10.1016/j.lfs.2021.120056 [Google Scholar] [PubMed] [CrossRef]
Herbst RS, Morgensztern D, Boshoff C (2018). The biology and management of non-small cell lung cancer. Nature 553: 446–454. https://doi.org/10.1038/nature25183 [Google Scholar] [PubMed] [CrossRef]
Hernández-Camarero P, López-Ruiz E, Marchal JA, Perán M (2021). Cancer: A mirrored room between tumor bulk and tumor microenvironment. Journal of Experimental & Clinical Cancer Research 40: 217. https://doi.org/10.1186/s13046-021-02022-5 [Google Scholar] [PubMed] [CrossRef]
Hirsch FR, Scagliotti GV, Mulshine JL, Kwon R, Curran WJ, Wu YL, Paz-Ares L (2017). Lung cancer: Current therapies and new targeted treatments. Lancet 389: 299–311. https://doi.org/10.1016/S0140-6736(16)30958-8 [Google Scholar] [PubMed] [CrossRef]
Horning AM, Wang Y, Lin CK, Louie AD, Jadhav RR et al. (2018). Single-cell RNA-seq reveals a subpopulation of prostate cancer cells with enhanced cell-cycle-related transcription and attenuated androgen response. Cancer Research 78: 853–864. https://doi.org/10.1158/0008-5472.CAN-17-1924 [Google Scholar] [PubMed] [CrossRef]
Hsu JM, Lee YCG, Yu CTR, Huang CYF (2004). Fbx7 functions in the SCF complex regulating Cdk1-cyclin B-phosphorylated hepatoma up-regulated protein (HURP) proteolysis by a proline-rich region. The Journal of Biological Chemistry 279: 32592–32602. https://doi.org/10.1074/jbc.M404950200 [Google Scholar] [PubMed] [CrossRef]
Hu S, Zeng W, Zhang W, Xu J, Yu D, Peng J, Wei Y (2020). KIAA0101 as a new diagnostic and prognostic marker, and its correlation with gene regulatory networks and immune infiltrates in lung adenocarcinoma. Aging 13: 301–339. https://doi.org/10.18632/aging.104144 [Google Scholar] [PubMed] [CrossRef]
Huang J, Li Y, Lu Z, Che Y, Sun S et al. (2019). Analysis of functional hub genes identifies CDC45 as an oncogene in non-small cell lung cancer—A short report. Cellular Oncology 42: 571–578. https://doi.org/10.1007/s13402-019-00438-y [Google Scholar] [PubMed] [CrossRef]
Imielinski M, Berger AH, Hammerman PS, Hernandez B, Pugh TJ et al. (2012). Mapping the hallmarks of lung adenocarcinoma with massively parallel sequencing. Cell 150: 1107–1120. https://doi.org/10.1016/j.cell.2012.08.029 [Google Scholar] [PubMed] [CrossRef]
Jiang X, Liu B, Nie Z, Duan L, Xiong Q, Jin Z, Yang C, Chen Y (2021). The role of m6A modification in the biological functions and diseases. Signal Transduction and Targeted Therapy 6: 74. https://doi.org/10.1038/s41392-020-00450-x [Google Scholar] [PubMed] [CrossRef]
Kan G, Wang Z, Sheng C, Yao C, Mao Y, Chen S (2021). Inhibition of DKC1 induces telomere-related senescence and apoptosis in lung adenocarcinoma. Journal of Translational Medicine 19: 161. https://doi.org/10.1186/s12967-021-02827-0 [Google Scholar] [PubMed] [CrossRef]
Kim MJ, Cervantes C, Jung YS, Zhang X, Zhang J et al. (2021). PAF remodels the DREAM complex to bypass cell quiescence and promote lung tumorigenesis. Molecular Cell 81: 1698–1714.e6. https://doi.org/10.1016/j.molcel.2021.02.001 [Google Scholar] [PubMed] [CrossRef]
Li W, Lin J, Huang J, Chen Z, Sheng Q, Yang F, Yang X, Cui X (2022). MicroRNA-409-5p inhibits cell proliferation, and induces G2/M phase arrest and apoptosis by targeting DLGAP5 in ovarian cancer cells. Oncology Letters 24: 261. https://doi.org/10.3892/ol.2022.13381 [Google Scholar] [PubMed] [CrossRef]
Li Z, Yu B, Qi F, Li F (2021). KIF11 serves as an independent prognostic factor and therapeutic target for patients with lung Adenocarcinoma. Frontiers in Oncology 11: 670218. https://doi.org/10.3389/fonc.2021.670218 [Google Scholar] [PubMed] [CrossRef]
Liao W, Liu W, Yuan Q, Liu X, Ou Y et al. (2013). Silencing of DLGAP5 by siRNA significantly inhibits the proliferation and invasion of hepatocellular carcinoma cells. PLoS One 8: e80789. https://doi.org/10.1371/journal.pone.0080789 [Google Scholar] [PubMed] [CrossRef]
Matharu N, Ahituv N (2020). Modulating gene regulation to treat genetic disorders. Nature Reviews Drug Discovery 19: 757–775. https://doi.org/10.1038/s41573-020-0083-7 [Google Scholar] [PubMed] [CrossRef]
Muenst S, Läubli H, Soysal SD, Zippelius A, Tzankov A, Hoeller S (2016). The immune system and cancer evasion strategies: Therapeutic concepts. Journal of Internal Medicine 279: 541–562. https://doi.org/10.1111/joim.12470 [Google Scholar] [PubMed] [CrossRef]
Rao X, Cao H, Yu Q, Ou X, Deng R, Huang J (2022). NEAT1/MALAT1/XIST/PKD—Hsa-Mir-101-3p—DLGAP5 axis as a novel diagnostic and prognostic biomarker associated with immune cell infiltration in bladder cancer. Frontiers in Genetics 13: 892535. https://doi.org/10.3389/fgene.2022.892535 [Google Scholar] [PubMed] [CrossRef]
Shah KN, Bhatt R, Rotow J, Rohrberg J, Olivas V et al. (2019). Aurora kinase A drives the evolution of resistance to third-generation EGFR inhibitors in lung cancer. Nature Medicine 25: 111–118. https://doi.org/10.1038/s41591-018-0264-7 [Google Scholar] [PubMed] [CrossRef]
Shen W, Tong D, Chen J, Li H, Hu Z et al. (2022). Silencing oncogene cell division cycle associated 5 induces apoptosis and G1 phase arrest of non-small cell lung cancer cells via p53-p21 signaling pathway. Journal of Clinical Laboratory Analysis 36: e24396. https://doi.org/10.1002/jcla.24396 [Google Scholar] [PubMed] [CrossRef]
Shi S, Wu T, Ma Z, Zhang X, Xu K, Tian Q, Gao L, Yin X, Xu S, Yang S (2022). Serum-derived extracellular vesicles promote the growth and metastasis of non-small cell lung cancer by delivering the m6A methylation regulator HNRNPC through the regulation of DLGAP5. Journal of Cancer Research and Clinical Oncology 149: 4639–4651. https://doi.org/10.1007/s00432-022-04375-6 [Google Scholar] [PubMed] [CrossRef]
Succony L, Rassl DM, Barker AP, McCaughan FM, Rintoul RC (2021). Adenocarcinoma spectrum lesions of the lung: Detection, pathology and treatment strategies. Cancer Treatment Reviews 99: 102237. https://doi.org/10.1016/j.ctrv.2021.102237 [Google Scholar] [PubMed] [CrossRef]
Sun H, Zhang H, Yan Y, Li Y, Che G, Zhou C, Nicot C, Ma H (2022). NCAPG promotes the oncogenesis and progression of non-small cell lung cancer cells through upregulating LGALS1 expression. Molecular Cancer 21: 55. https://doi.org/10.1186/s12943-022-01533-9 [Google Scholar] [PubMed] [CrossRef]
Sung H, Ferlay J, Siegel RL, Laversanne M, Soerjomataram I, Jemal A, Bray F (2021). Global cancer statistics 2020: GLOBOCAN estimates of incidence and mortality worldwide for 36 cancers in 185 countries. CA: A Cancer Journal for Clinicians 71: 209–249. https://doi.org/10.3322/caac.21660 [Google Scholar] [PubMed] [CrossRef]
Szklarczyk D, Gable AL, Nastou KC, Lyon D, Kirsch R et al. (2021). The STRING database in 2021: Customizable protein-protein networks, and functional characterization of user-uploaded gene/measurement sets. Nucleic Acids Research 49: D605–D612. https://doi.org/10.1093/nar/gkaa1074 [Google Scholar] [PubMed] [CrossRef]
Thorsson V, Gibbs DL, Brown SD, Wolf D, Bortone DS et al. (2018). The immune landscape of cancer. Immunity 48: 812–830.e14. https://doi.org/10.1016/j.immuni.2018.03.023 [Google Scholar] [PubMed] [CrossRef]
Tsou AP, Yang CW, Huang CYF, Yu RCT, Lee YCG et al. (2003). Identification of a novel cell cycle regulated gene, HURP, overexpressed in human hepatocellular carcinoma. Oncogene 22: 298–307. https://doi.org/10.1038/sj.onc.1206129 [Google Scholar] [PubMed] [CrossRef]
Wang Q, Chen Y, Feng H, Zhang B, Wang H (2018). Prognostic and predictive value of HURP in non‐small cell lung cancer. Oncology Reports 39: 1682–1692. https://doi.org/10.3892/or.2018.6280 [Google Scholar] [PubMed] [CrossRef]
Wang J, Liu D, Sun Z, Ye T, Li J, Zeng B, Zhao Q, Rosie Xing H (2021). Autophagy augments the self-renewal of lung cancer stem cells by the degradation of ubiquitinated p53. Cell Death & Disease 12: 98. https://doi.org/10.1038/s41419-021-03392-6 [Google Scholar] [PubMed] [CrossRef]
Wherry EJ (2011). T cell exhaustion. Nature Immunology 12: 492–499. https://doi.org/10.1038/ni.2035 [Google Scholar] [PubMed] [CrossRef]
Wong J, Fang G (2006). HURP controls spindle dynamics to promote proper interkinetochore tension and efficient kinetochore capture. The Journal of Cell Biology 173: 879–891. https://doi.org/10.1083/jcb.200511132 [Google Scholar] [PubMed] [CrossRef]
Ye F, Tan L, Yang Q, Xia Y, Deng LW, Murata-Hori M, Liou YC (2011). HURP regulates chromosome congression by modulating kinesin Kif18A function. Current Biology 21: 1584–1591. https://doi.org/10.1016/j.cub.2011.08.024 [Google Scholar] [PubMed] [CrossRef]
You J, Wang X, Wang J, Yuan B, Zhang Y (2017). DDX59 promotes DNA replication in lung adenocarcinoma. Cell Death Discovery 3: 16095. https://doi.org/10.1038/cddiscovery.2016.95 [Google Scholar] [PubMed] [CrossRef]
Yuan H, Yan M, Zhang G, Liu W, Deng C et al. (2019). CancerSEA: A cancer single-cell state atlas. Nucleic Acids Research 47: D900–D908. https://doi.org/10.1093/nar/gky939 [Google Scholar] [PubMed] [CrossRef]
Zhang X, Pan Y, Fu H, Zhang J (2018). Nucleolar and spindle associated protein 1 (NUSAP1) inhibits cell proliferation and enhances susceptibility to epirubicin in invasive breast cancer cells by regulating cyclin D kinase (CDK1) and DLGAP5 expression. Medical Science Monitor 24: 8553–8564. https://doi.org/10.12659/MSM.910364 [Google Scholar] [PubMed] [CrossRef]
Cite This Article
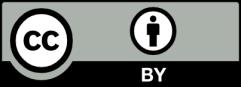
This work is licensed under a Creative Commons Attribution 4.0 International License , which permits unrestricted use, distribution, and reproduction in any medium, provided the original work is properly cited.