Guest Editors
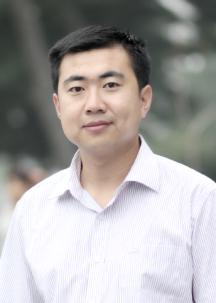
Prof. Dr. Qi Zhao, University of Science and Technology Liaoning, China. zhaoqi@lnu.edu.cn
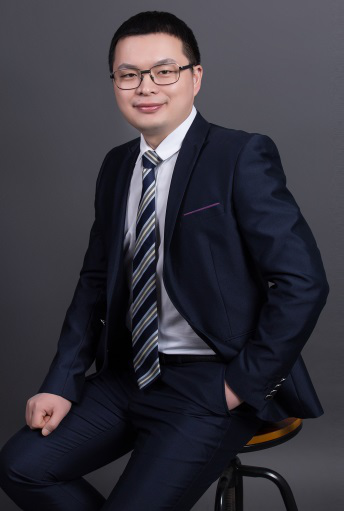
Prof. Dr. Liang Wang, Xuzhou Medical University, China. leonwang@xzhmu.edu.cn
Summary
Non-coding RNA (ncRNA) is an RNA molecule that does not encode a protein, but this does not mean that such RNAs do not contain information nor have function. Although it has been generally assumed that most genetic information is transacted by proteins, recent evidence suggests that the majority of the genomes of mammals and other complex organisms is in fact transcribed into ncRNAs, many of which are alternatively spliced and/or processed into smaller products. Abundant and functionally important types of ncRNAs include transfer RNAs (tRNAs) and ribosomal RNAs (rRNAs), as well as small RNAs such as microRNAs (miRNAs), siRNAs, piRNAs, snoRNAs, snRNAs, exRNAs, scaRNAs and the long ncRNAs (lncRNAs) such as Xist and HOTAIR. As is known, RNA regulatory networks determine most of our complex characteristics and play a significant role in disease and constitute an unexplored world of genetic variation both within and between species. Recent transcriptomic and bioinformatic studies suggest that the ncRNAs appear to comprise a hidden layer of internal signals that control various levels of gene expression in physiology and development, including chromatin architecture/epigenetic memory, transcription, RNA splicing, editing, translation and turnover. Furthermore, ncRNAs have been also revealed to contribute to diseases including cancer, autism, Alzheimer's and so on. Predicting ncRNA–disease associations could not only boost human disease diagnostic and prognostic, but also improve the new drug development.
However, little efforts have been attempted to understand and predict ncRNA–disease associations on a large scale until now. And the traditional methods are both expansive and time-consuming. In contrast to the traditional experimental approach, the aim of us is to assess these ncRNA-disease associations on a large-scale in human with the computational methods based on the big data accumulated by the previous experimental methods. To find associations between the ncRNAs and their corresponding diseases, various statistical and computational techniques could be employed. Meanwhile, benefitting from the rapid development of artificial intelligence, accumulating computational methods of analyses and prediction on large scale data have been developed to work for various fields related to data science. As the previous traditional experimental results revealed, the ncRNA-disease pairs appeared some laws to be associated with each other.
Therefore, it is feasible and necessary to build advanced intelligent algorithm or computational models to reveal the ncRNA-disease associations. Furthermore, with the help of the computational methods, the experimental analyses of associations between ncRNA and disease or other instances could be more convenient and accurate. We can look forward that more and more computational models on ncRNA-disease association prediction will be developed to promote the further experimental studies with our best efforts. Similar to the researches on ncRNA-disease association, the researches on ncRNA-protein interaction, function and structure of ncRNAs, and even drug effect associated with ncRNAs are all the fields that we will devote ourselves to. We hence invite investigators to contribute research article, reviews, and commentaries describing recent findings which use computational techniques for the research of ncRNAs.
Keywords
ncRNA-Disease Association Prediction, ncRNA-Protein Interaction Prediction, ncRNA Function Prediction, ncRNA Structure Prediction, ncRNA and Drug Effect
Published Papers