Open Access
ARTICLE
Using Machine Learning to Determine the Efficacy of Socio-Economic Indicators as Predictors for Flood Risk in London
1 Department of Earth Science and Engineering, Imperial College London, London, SW7 1NE, UK
2 Centre for Environmental Policy, Imperial College London, London, SW7 1NE, UK
3 Nature Based Solutions Initiative (NBSI), School of Geography and Environment, Oxford University, Oxford, SW7 2UA, UK
* Corresponding Author: Minerva Singh. Email:
Revue Internationale de Géomatique 2024, 33, 427-443. https://doi.org/10.32604/rig.2024.055752
Received 06 July 2024; Accepted 12 September 2024; Issue published 11 October 2024
Abstract
This study examines how socio-economic characteristics predict flood risk in London, England, using machine learning algorithms. The socio-economic variables considered included race, employment, crime and poverty measures. A stacked generalization (SG) model combines random forest (RF), support vector machine (SVM), and XGBoost. Binary classification issues employ RF as the basis model and SVM as the meta-model. In multiclass classification problems, RF and SVM are base models while XGBoost is meta-model. The study utilizes flood risk labels for London areas and census data to train these models. This study found that SVM performs well in binary classifications with an accuracy rate of 0.60 and an area under the curve of 0.62. XGBoost outperforms other multiclass classification methods with 0.62 accuracy. Multiclass algorithms may perform similarly to binary classification jobs due to reduced data complexity when combining classes. The statistical significance of the result underscores their robustness, respectively. The findings reveal a significant correlation between flood risk and socio-economic factors, emphasizing the importance of these variables in predicting flood susceptibility. These results have important implications for disaster relief management and future research should focus on refining these models to improve predictive accuracy and exploring socio-economic factors.Keywords
Cite This Article
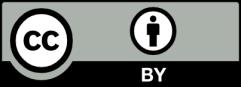
This work is licensed under a Creative Commons Attribution 4.0 International License , which permits unrestricted use, distribution, and reproduction in any medium, provided the original work is properly cited.