Open Access
ARTICLE
Group Decision-Making Based on GIS and MultiCriteria Analysis for Assessing Land Suitability for Agriculture
Algerian Space Agency, Centre des Techniques Spatiales, Arzew, 31200, Algeria
* Corresponding Author: Abdelkader Mendas. Email:
Revue Internationale de Géomatique 2024, 33, 383-398. https://doi.org/10.32604/rig.2024.055321
Received 23 June 2024; Accepted 04 September 2024; Issue published 25 September 2024
Abstract
The main purpose of this work is to propose a methodology that considers the multicriteria and multi-actor aspects for assessing land suitability for agriculture. This involves offering a group spatial decision-making approach. The members of a multidisciplinary team can decide on the relative importance of the criteria and the ranking of alternatives. Each member provides his judgment and contributes in a distinct and identifiable manner to find a compromise solution. Twelve criteria (easily available water reserve, cation exchange capacity, electric conductivity, potential of hydrogen (pH), drainage, permeability, active limestone, soil texture, soil useful depth, slopes, labor availability, and proximity to roads) grouped into four factors (agronomy, planning and socio-economy, land enhancement and improvement, conservation of soils and environmental protection) were selected in this study. The methodology consists of calculating the initial criteria weights using the AHP method. The final weights are obtained using the Consensual Convergence Model (CCM), and the decision-maker’s performance is aggregated using the ELECTRE Tri method. All the required processing methods were integrated into a GIS environment. The methodological developments were motivated by an application to the suitability of land for durum wheat cultivation in a study area in Mleta, Algeria, which is comprised of 74 land units. Every criterion was classified from the best to the poor based on its values and used for assessing land suitability for agriculture. The land units were assigned to different predefined classes. The final results are presented as a map produced according to the optimistic procedure of ELECTRE Tri. The greatest contribution of this research lies in integrating group decision-making in multicriteria spatial decisions, particularly the land suitability for agriculture, which has never been previously addressed. The consistency of the obtained map confirms the methods’ effectiveness.Keywords
Agriculture is a key component of national economic development. It differs from other sectors by the importance of soils as a production factor. Agricultural development remains linked to the type of agriculture to be promoted. The agricultural sector must rationally use the available natural resources to achieve efficient wheat farming with high yields. Sustainable agriculture is a complex concept involving many aspects as socio-economy and ecology. It aims to increase the productivity of the soil and reduce the negative effects of agricultural practices on climate, soil, water, environment, and human health. It focuses on the needs, knowledge, skills, and socio-cultural values of the local people. It makes it possible to produce more than one product in a small area with high efficiency. It also contributes to the creation of new employment. The soil plays an important role in the sustainable agriculture. It should be enriched with natural fertilizers which are healthier for plants, water, air, and people than chemical fertilizers [1]. Algeria depends on others for its food supplies. To improve the yield, the rational use of natural resources and the enhancement of existing productive potential, Algeria has introduced since the 2000s a policy designed to improve national food security, to develop some priority agricultural sectors such as cereals, and to improve land through various national plans, in particular, the National Agricultural Development Program (PNDA 2000–2010) that became in 2002 the National Plan of Agricultural and Rural Development (PNDAR), the Policy of Agricultural and Rural Renewal (PRAR 2010–2014), and the FELAHA Plan (2014–2020) [2]. Algeria has deployed substantial public investments into the agricultural sector to support enhancement programs and development projects, and further steps have been taken to promote wheat production to increase local production and thus reduce imports. To increase yields and reduce food dependency and costs, there is a need to utilize the land in a balanced way, taking into account its agricultural suitability and the intended objectives, and to extend the areas allocated to agriculture in the hope of obtaining better production and meeting consumer demands. In other words, it is crucial to utilize available agricultural lands appropriately according to the suitable crop and to take into account the opinions of all experts in the domain. Assessing land suitability for agriculture is a spatial decision problem that includes many criteria and multiple actors (experts, stakeholders). To achieve optimal decision-making, the opinion of each participant in the team and the importance of each criterion involved in this decision must be evaluated and then included in the decision process [3]. Achieving a consensus within this team on the relative importance of different criteria can be difficult indecision-making. Multicriteria Decision Making (MCDM) allows the consideration of multiple divergent criteria to reach acceptable compromises. Spatial decision-making problems have the characteristics of multicriteria issues, which means multicriteria evaluation is essential. This research aims to take into account the multicriteria and multi-actor aspects for assessing the suitability of land for agriculture, showing their importance in spatial decision support and actively involving all participants in the decision-making. It is about providing the main decision-maker with a scientific approach that enables him to make scientifically-based group decisions using objectively derived information. This will avoid contradictory decisions. Thus, the innovation of this research lies in integrating group decision-making in multicriteria spatial decisions, particularly the land suitability for agriculture, which has never been previously addressed. The implementation of the adopted methodological approach requires adequate decision-making methods and a CCM integrated into GIS software. An application was conducted to evaluate the suitability of lands for agriculture of durum wheat.
In most multicriteria problems, there are potential actions (alternatives), criteria (of unequal importance), and decision-maker performances. In this work, a methodological approach based on GIS, MCDA and CCM has been proposed. This approach will facilitate the land suitability assessment for agriculture [4,5] when many criteria and various participants are taken into account. The importance of different criteria expressed by several experts (agronomists, hydrologists, economists, and planners) is assessed in the first step and then introduced into a consensus convergence model. The second step is to provide decision-makers with a scientific approach that helps them to make a decision based on scientific principles using derived information. Applications were carried out on the plain of Mleta in Algeria and the main outputs are presented.
Values of criteria concerning the soil environment are measured by specific techniques in a hydro-agricultural study conducted by (SCETTunisia/ENHYD-Algeria). Insofar as the main objective of this research is to show the contribution of group decision-making, GIS, and MultiCriteria Decision Making to assess land suitability for agriculture, values of criteria were taken from the sub-mentioned study. Every criterion was classified (from the best to the poor based on its values) and used for assessing land suitability for agriculture.
The main components of the adopted method are included in the following scheme (Fig. 1).
Figure 1: General flowchart of the adopted methodology
The initial criteria weights will be calculated using the AHP (Analytic Hierarchy Process) method. The consensual criteria weights will be determined using the CCM. Each land unit represents an alternative which is evaluated against the criteria. These values, the weights of criteria, and all the other performances of decision makers as thresholds are registered in a database into QGIS (Quantum Geographic Information System). Their aggregation will be used according to the ELECTRE Tri method. The results will be presented as maps using the functionalities of QGIS. QGIS is a free, open-source software that allows users to create, edit, visualize, analyze, and publish geospatial information. This software offers many free online resources and maps available to download. It accepts many vector file formats and there are a variety of plug-ins for potential use. Plug-ins are extra applications that can be downloaded to complete a specific task that is not easily accomplished otherwise [6].
The Analytic Hierarchy Process (AHP), proposed by Saaty [7], is up to single criterion approaches of synthesis, neglecting all incomparability. It consists of seeking a function that allows the calculation of a corresponding value for each option, then the best-rated one is selected. AHP makes it possible to decompose complex problems into hierarchical levels such as goals, factors, and criteria (Fig. 2), and it makes a criteria pairwise comparison based on the Saaty scale to identify the best alternative. AHP can help to make the most appropriate decision based on a synthesis of the comparison outcomes. Its theory utilizes expert information to measure the priority of judgments, showing how much one factor dominates the other for a given feature [7,8]. Therefore, the AHP requires priority values obtained from weightings and validated by the measurement of consistency to give preferences between several criteria and to rank alternatives by successive pairwise comparisons [9]. It is a multicriteria decision-making method allowing the interpretation of data and information. The use of AHP for the weight calculation is highly suitable because it allows us to check the weight consistency through a consistency ratio (CR) [10]. If CR is greater than 0.1, then the used data needs to be checked, and additional information should be added to improve consistency.
Figure 2: Hierarchical representation of the decision problem
ELECTRE Tri allows the allocation of the alternatives to predefined classes (categories) through a partial aggregation approach to decision-maker performances. It belongs to the sorting problem [11]. Each alternative is compared to the profiles (alternatives of reference), and a value is determined. The alternative is allocated to a class by comparing its evaluation against all criteria with the profiles defining the boundaries of classes [12]. Two procedures of assignment are available: the first one compares the alternative successively to the alternatives of reference in an ascending order, and the second one compares the alternative successively to the alternatives of reference in a descending order. When the alternative evaluation is between the two profiles of a class, both procedures allocate this alternative to that class. When an alternative is not comparable to the alternatives of reference, the pessimistic procedure allocates this alternative to a lower class than the optimistic one [12,13]. The method of ELECTRE Tri uses an outranking relation to allow the comparison between an alternative and an alternative of reference. This relation needs the calculation of various indices (For more details see [14]).
In this study, AHP was used for criteria weight calculation, and ELECTRE Tri was used for the aggregation of the decision-makers performances.
2.2 Consensus Convergence Model (CCM)
In this research, the CCM developed by Regan et al. [15] is used. It consists of grouping the individual criteria weights to form a consensual weight for a group of participants in decision-making. There are two types of weights: criteria weights pim and respect weights wij that each participant i expresses for all other participants j [16]. It is supposed that participants in the decision have opinions about the expertise and rationality of the other participants [17] and that n members have initial criterion weightings P10; P20; ...; Pn0. Each participant assigns a respect weight for himself and for the other participants (at least a positive weight to one other participant) [18]. As the weight of respect increases, so does the importance of the participant’s expertise j [15]. The original criterion weight is updated to incorporate the views of other participants according to their weights of respect. The new criterion weight of participant i is as follows [15]:
W: weights of respect,
P: initial criterion weight for each member.
Criterion weight of state-one = WP
Criterion weight of state-two = W2P
Criterion weight of state-m = WmP
When m increases, the criteria weights converge to a single number which becomes the consensual criterion weight [15].
The algorithms of AHP, CCM, and ELECTRE Tri are implemented into a GIS environment using the Python language to provide a spatial decision support system [19]. That system is developed independently of the area and the crop type [20]. GIS technologies allow the outcomes to be visualized as a map [21] which contains an attribute table with all the necessary information on the land units, the criteria, the constraints, and the calculated indices of AHP, CCM, and ELECTRE Tri [22]. Ultimately, each land unit will be assessed based on these indices.
The criteria needed to identify the lands suitable for agriculture are derived from factors related to agronomy, planning and socio-economy, land enhancement and improvement, and soil conservation and environment protection [23]. Twelve (12) criteria required for durum wheat (Triticum durum Desf.) were selected in consultation with agriculture experts (Table 1) and their weights were calculated according to the method of AHP. Additional criteria, where data are available, can be integrated into the developed system to improve land suitability for durum wheat agriculture.
The criteria weights are calculated using the AHP method proposed by Saaty [7]. This method makes it possible to break down complex problems into hierarchical levels and to make pairwise comparisons between criteria based on a weighting scale (Saaty scale) to identify the best alternative. This method is based on priority values derived from weights and validated through coherence measurements. The use of AHP for calculating the weights is particularly suitable because it allows us to check the weight consistency via a consistency ratio (CR) using the following equation [10]:
where:
CI: Consistency Index. It is calculated as follows [7]:
λmax: Maximum Eigenvalue. It is calculated by following Saaty’s method [10].
n: number of factors or criteria.
RI: Random Index, given by Saaty [8].
CR measures the decision maker’s consistency when rating the criteria. If it is greater than 0.1, then the decision maker should repeat the pairwise comparison, re-examine the data used for calculating the weights, and incorporate additional information until CR is less than 0.1 [10].
Potential alternatives are represented by land units to be assessed using MCDM procedures. Key information about agronomy, planning, soils, environment, and socio-economy is stored in the feature attribute table of GIS. The study area includes seventy-four (74) land units. Sixty-three (63) land units will be assessed, while eleven (11) are considered outside assessment [21].
The concept of MCDM and GIS has been used by several authors to develop decision-support approaches for evaluating land suitability for agricultural activities. Martine [24] explored the application of GIS-Multi-criteria analysis in modeling future land use scenarios for resource planning and management. Getachew et al. [25] combined GIS and MCDM to identify permissible areas suitable for rice crop production. Munkhdulam et al. [26] developed an approach based on MCDM and GIS for preparing a cropland suitability map based on comprehensive landscape principles, including topography, soil properties, vegetation, climate, and socio-economic factors. Parvin et al. [27] combined MCDM and GIS for the use of urban treated wastewater in agriculture. Maulana et al. [28] conducted a study to evaluate land suitability by integrating MCDM, GIS, and augmented Reality. Mathenge et al. [29] used GIS to improve agriculture sustainability. Khater et al. [30] applied GIS techniques to determine the optimum site to collect the residues to reduce cost and increase the benefits. Topuz et al. [31] proposed an approach based on AHP and GIS for creating a land use suitability map. Isaac et al. [32] created a semi-detailed land suitability map using georeferenced soil survey data and spatial environmental data to assist agricultural development planners and decision-makers. Nungula et al. [33] analyzed the appropriateness of land for sunflower production using GIS and AHP. Yaman [34] developed a GIS-based multi-criteria decision-making approach for identifying optimal sites for installing wind power plants through the integration of AHP and GIS. Nikolić et al. [35] modeled the wildfire susceptibility in different climate zones in Montenegro using GIS-MCDA. The innovation of this study lies in the development of an approach integrating group decision-making, GIS, and multicriteria decision-making to assess land suitability for agriculture, which has never been previously addressed. The integration of different methods of multicriteria analysis into a GIS provides users with a familiar and powerful environment for geospatial analysis enhancing usability and accessibility. Additionally, the cartographic visualization of results facilitates the understanding of the alternatives’ ranking.
To test the developed system, the plain of Mleta in Algeria (Fig. 3) has been selected because most of the necessary data are available. The main data are derived from a hydro-agricultural study conducted by SCET-Tunisia/ENHYD-Algeria [36] and a soil survey carried out by ANRH-Algeria. For geographical data the datum of “Nord Sahara 1959” and the projection system of UTM (Universal Transverse Mercator) Zone 30N are used. Covering an area of about 10,000 ha, the plain is situated about 20 kilometers from Oran. It is easily accessible via National Roads N°3 and National Road N°4. Additionally, several other roads of Wilaya cross or surround the plain [20].
Figure 3: Study area location. Adapted with permission of Reference [20]. Copyright © 2020, Springer Nature B.V.
The main data used in this study comes from a hydro-agricultural study conducted by (SCETTunisia/ENHYD-Algeria) [36]. These data and other performances of decision-makers are registered in a Database in QGIS. The land units are evaluated according to the ELECTRE Tri method and then assigned to predefined classes to produce the land suitability map.
The AHP method proposed by Saaty [7] was used for the calculation of the initial criteria weights. To avoid burdening the document, the detailed weights obtained from a single expert (agronomist) are reported. Four experts participated in determining the criteria importance: Expert1: agronomist; Expert2: hydrologist; Expert3: socio-economist and Expert4: planner.
Table 2 indicates the factors comparison using the Saaty scale [7] and Table 3 contains their relative importance according to the agronomist expert.
Table 4 shows the agronomic criteria comparison using the Saaty scale and Table 5 illustrates the relative importance according to the agronomist expert.
The same process is applied to all other criteria of factors (planning and socio-economy, land enhancement and improvement, conservation of soils, and protection of the environment). The initial weights for the agronomist expert’s criteria are represented in Table 6.
Using the same method, the factors and criteria weights for the other experts were obtained (Tables 7 and 8).
The selected consensus convergence model was applied to incorporate the different participants’ opinions in the decision-making process and to achieve final (consensual) weights. This model was adapted to use a respect weight based on the difference in criteria weights assigned by the group members. Its advantage is to place importance on the different opinions behind the criteria weight assignments rather than the weights of individual assignments [15]. The selected method allows the implementation of the consensus convergence model after the application of AHP. Its primary purpose is to use the CCM to aggregate the weights assigned by the four experts (agronomist, hydrologist, socio-economist, and planner) for each criterion used. Let pi0 be the initial criterion weight assigned by expert i. This expert must assign a weighting wij to each other expert j (including himself) based on the differences between the pi0 value of expert i and the pj0 values held by the other experts.
The main properties of the weights of respect are as follows [18]:
– Each member gives the highest weight to himself.
– Higher weights are assigned to the team members with similar values of pi0.
– Lower weights are assigned to members with more disparate values of pi0.
To address these issues, the weights of respect of the “Soil texture” criterion are calculated using Formula (2).
The criterion weight was calculated for each team member and then used in Formula (1). Criteria weights were updated following Formula (3).
This procedure was repeated until all values of the column P (Formula (3)) became identical. Consensual criteria weights were achieved by iterating Formula (3) [15]. For the criteria “Soil texture”, the different weights of respect obtained using Formula (2) and the various criteria weights determined using AHP were used, and the criterion’s consensual weight converged towards 0.098. The consensual weights obtained for all criteria selected in this study are reported in Table 9.
The performance aggregation using ELECTRE Tri uses the following discrimination thresholds [37]: indifference threshold q, preference threshold p, veto threshold v, and cutting threshold λ. The evaluation of these thresholds largely depends on the stakeholders’ experience in decision-making. Thus, based on each criterion definition and the specialists’ experience in the field, the cutting threshold λ is settled at 0.7 [12,20], then preference, indifference, and veto thresholds have been set for each criterion. The optimistic procedure of ELECTTE Tri has been selected to aggregate decision-maker performances and to assign land units to different predefined classes. This procedure is more appropriate for the assessment of land suitability for agriculture [22]. A map has been produced according to the optimistic procedure (Fig. 4). Since a GIS-AMCD-CCM full integration was used, the data exchange between the two software (QGIS and ELECTRE Tri) is done automatically. The results obtained in this research remain experimental.
Figure 4: Land suitability map for durum wheat agriculture
Given the diversity of views among members involved in the decision-making process, especially regarding the relative importance of criteria in this study, it is unlikely that the negotiation will lead to consensual weights of criteria. Therefore, this situation is ideal for applying a consensus convergence model which is simple and has considerable mathematical power to reveal the negotiation structure [38]. If each group member gives positive weight to other members and this process is iterated, the team members will reach a consensus. In this study, a method developed by Regan et al. [15] was used to determine respect weights which are employed in a consensus convergence model. This model can be combined with an MCDM method using weights assigned by decision group members. According to the optimistic procedure selected in this research, 31 units are allocated to Class S1 (Very suitable), 29 units to S2 (Moderately suitable), and only 3 units are identified as marginally suitable (Class S3). The outcomes of this study were compared to results obtained in a previous study conducted by Mendas et al. [20] using multi-criteria methods with a single decision-maker. This comparison shows that 40 units remain allocated to the same suitability class. Ranking differences are due to the use of consensus weights which reflect the reality better, since the opinions of several experts in the field were taken into consideration which has increased the accuracy of the results.
The work carried out in this study defines a methodology that assists in spatial decision-making in a multi-criteria and multi-decision-makers context. This approach is based on a multi-criteria analysis process for spatial decision support, which involves establishing a list of variants to be evaluated, and defining an exhaustive series of criteria and weighting them. To incorporate the group decision aspect, it was necessary to express the individual materiality of criteria using the AHP method, involving different participants in the decision-making process to determine the final criteria weights using a CCM for achieving a consensus on complex decision problems. The decision-makers performances have been aggregated using the ELECTRE Tri method. The necessary algorithms have been implemented into a GIS environment. These methods are well-anchored and easily implemented. The proposed methodology enables the multitude of stakeholders from different fields in a decision-making process to be taken into account to reach a consensual decision. In spatial decisions, several experts are involved. These experts do not need to be present during the evaluation. Each of them can perform the analysis independently, and when all the experts finish, the data coming from each expert are aggregated to get the final analytical result. This can prevent participants in the decision to be influenced by their colleagues’ decisions. The identification of lands suitable for agriculture has demonstrated the power of multi-criteria and multi-decision-maker analysis methods in decision support. Thus, the use of the CCM greatly contributes to the improvement of spatial decisions.
The obtained outcomes do not present a definitive classification, but rather a support tool available to the decision-maker to consolidate his decision-making. Applications carried out have also demonstrated the need to use GIS during this type of work for preparing data and displaying results. In this study, all needed data and information were identified and organized. The study provided an opportunity to obtain a soil capability map for the durum wheat according to the optimistic procedure of the ELECTRE Tri method. The thresholds required for ELECTRE Tri make it possible to account for the imperfections characterizing data. Another strength of this method lies in the decision maker’s preferences aggregation mode and the potential variants assignment to earmarked categories. The allocation of the land units to predefined classes in terms of suitability highlights the areas most suitable for durum wheat cultivation, which helps users to exploit the available land rationally for enhancing agricultural production. In practice, a good agriculture suitability analysis requires the availability of data from various sectors. In a future perspective, we propose to study and analyze the sensitivity and robustness of the multicriteria methods used in spatial decision-making and to verify the stability of the obtained results.
Acknowledgement: We are highly thankful to the anonymous referees for their helpful comments and suggestions in revising the manuscript and also, to the concerned departments for providing the necessary information and data needed for the research.
Funding Statement: The authors received no specific funding for this study.
Author Contributions: Abdelkader Mendas contributed to the conceptualization of the research and preparation of the manuscript. Abdellah Mebrek contributed to the implementation of CCM and ELECTRE Tri into the GIS environment and their application. Zohra Mekranfar contributed to the implementation of AHP into the GIS environment and its application. Abdelkader Mendas, Abdellah Mebrek, and Zohra Mekranfar contributed to the analysis and interpretation of results. All authors reviewed the results and approved the final version of the manuscript.
Availability of Data and Materials: Data used in this study are obtained from the hydro-agricultural improvements study (SCET-Tunisia/ENHYD-Algeria, 2007), the topographical map in 1/25000, the world population map (www.diva-gis.org, accessed on 10 August 2024) and ASTER GDEM.
Ethics Approval: Not applicable.
Conflicts of Interest: The authors declare that they have no conflicts of interest to report regarding the present study.
References
1. Mehmet Tuğrul K. Soil management in sustainable agriculture. In: Mirza Hasanuzzaman Sher-e-Bangla Agricultural University, editor. Sustainable crop production. Bangladesh: IntechOpen; 2020. doi:10.5772/intechopen.88319. [Google Scholar] [CrossRef]
2. Bessaoud O, Pellissier JP, Rolland JP, Khechimi W. Rapport de synthèse sur l’agriculture en Algérie. Montpellier: CIHEAM-IAMM; 2019. p. 82. Report No.: Hal-02137632. [Google Scholar]
3. Malczewski JA. GIS-based approach to multiple criteria group decision-making. Int J Geogr Inf Sci. 1996;10(8):955–71. doi:10.1080/02693799608902119. [Google Scholar] [CrossRef]
4. Jafari S, Zaredar N. Land suitability analysis using multi attribute decision making approach. Int J Environ Sci Dev. 2010;1(5):441–45. doi:10.7763/IJESD.2010.V1.85. [Google Scholar] [CrossRef]
5. Prakash TN. Land suitability analysis for agricultural crops: a fuzzy multicriteria decision making approach (Thesis). ITC: Netherlands; 2003. [Google Scholar]
6. Palino G, Coastal SE. Coastal and marine extension program. Publication 3269 (POD-10-21) MASGP-18-038. USA: Mississippi State University; 2021. [Google Scholar]
7. Saaty TL. Scaling method for priorities in hierarchical structures. J Math Psychol. 1977;15(3):234–81. doi:10.1016/0022-2496(77)90033-5. [Google Scholar] [CrossRef]
8. Saaty TL. Decision-making with the analytic hierarchy process. Int J Serv Sci. 2008;1(1):83–98. doi:10.1504/IJSSCI.2008.017590. [Google Scholar] [CrossRef]
9. Roy B, Vincke P. Relational systems of preferences with one or more pseudo-criteria: some new concepts and results. Manage Sci. 1984;30(11):1323–34. [Google Scholar]
10. Saaty TL. Decision-making with the analytic hierarchy process. Sci Iran. 2002;9(3):215–29. [Google Scholar]
11. Almeida-Dias J, Figueira JR, Roy B. ELECTRE Tri-C: a multiple criteria sorting method based on characteristic reference actions. Eur J Oper Res. 2010;204(3):565–80. [Google Scholar]
12. Mousseau V, Slowinski R, Zielniewicz P. ELECTRE Tri 2.0a, Methodological guide and user’s manual. Paris: LAMSADE; 1999. Report No.: 111. [Google Scholar]
13. Schärlig A. Pratiquer ELECTRE et Promethee : un complément à décider sur plusieurs critères (Practicing ELECTRE and Promethee : a complement to make decision in multiple criteria). Lausanne: Presses Polytechniques et Universitaires Romandes; 1996. [Google Scholar]
14. Maystre LY, Pictet J, Simos J. Méthodes multicritères ELECTRE. Description, conseils pratiques et cas d’application à la gestion environnementale. Lausanne: Presses Polytechniques et Universitaires Romandes; 1994. [Google Scholar]
15. Regan HM, Colyvan M, Markovchick-Nicholls L. A formal model for consensus and negotiation in environmental management. J Environ Manage. 2006;80(2):167–76. [Google Scholar] [PubMed]
16. Lehrer K, Wagner C. Rational consensus in science and society. Dordrecht: Reidel; 1981. [Google Scholar]
17. Du Z, Yu S, Luo H, Lin X. Consensus convergence in large-group social network environment: coordination between trust relationship and opinion similarity. Knowl-Based Syst. 2021;217(3):106828. [Google Scholar]
18. Yaniv I. Receiving other people’s advice: influence and benefit. Organ Behav Hum Decis Process. 2004;93(1):1–13. [Google Scholar]
19. Mendas A, Hamadouche MA, Nechniche H, Djilali A. Elaboration d’un système d’aide à la décision spatiale-Application à la dangerosité de l’infrastructure routière (Development of a spatial decision support system. Application to the road infrastructure danger). J Decis Syst. 2007;16(3):369–91. [Google Scholar]
20. Mendas A, Delali A. Integration of multicriteria decision analysis in GIS to develop land suitability for agriculture: application to durum wheat cultivation in the region of Mleta in Algeria. Comput Electron Agric. 2012;83(5):117–26. [Google Scholar]
21. Mendas A, Delali A, Khalfallah M, Likou L, Gacemi MA, Boukrentach H, et al. Improvement of land suitability assessment for agriculture-application in Algeria. Arab J Geosci. 2014;7(2):435–45. [Google Scholar]
22. Mendas A, Mebrek A, Mekranfar Z. Comparison between two multicriteria methods for assessing land suitability for agriculture: application in the area of Mleta in western part of Algeria. Environ Dev Sustain. 2021;23(6):9076–89. [Google Scholar]
23. Food and Agriculture Organization of the United Nations. Land evaluation: towards a revised framework. Rome: FAO; 2007. Report No.: 6. [Google Scholar]
24. Martine N. GIS and multi-criteria decision analysis for land use resource planning. J Geogr Inf Syst. 2012;4(4):341–8. [Google Scholar]
25. Ayehu GT, Besufekad SA. Land suitability analysis for rice production: a GIS based multi-criteria decision approach. Am J Geogr Inf Syst. 2015;4(3):95–104. [Google Scholar]
26. Otgonbayar M, Atzberger C, Chambers J, Amarsaikhan D, Böck S, Tsogtbayar J. Land suitability evaluation for agricultural cropland in mongolia using the spatial MCDM method and AHP based GIS. J Geosci Environ Prot. 2017;5(9):238–63. doi:10.4236/gep.2017.59017. [Google Scholar] [CrossRef]
27. Parvin Z, Mahdi Z, Hossein K. A model for the use of urban treated wastewater in agriculture using multiple criteria decision making (MCDM) and geographic information system (GIS). Agric Water Manag. 2021;243(8):106490. doi:10.1016/j.agwat.2020.106490. [Google Scholar] [CrossRef]
28. Maulana H, Kanai H. Land suitability evaluation by integrating multi-criteria decision-making (MCDM), geographic information system (GIS) method, and augmented reality-GIS. KES Int Conf Intell Decis Technol. 2021. doi:10.1007/978-981-16-2765-1. [Google Scholar] [CrossRef]
29. Mathenge M, Sonneveld BGJS, Broerse JEW. Application of GIS in agriculture in promoting evidence-informed decision making for improving agriculture sustainability: a systematic review. Sustainability. 2022;14(16):9974. doi:10.3390/su14169974. [Google Scholar] [CrossRef]
30. Khater ESG, Ali SA, Afify MT, Bayomy MA, Abbas RS. Using geographic information systems (GIS) to determine the suitable site for collecting agricultural residues. Sci Rep. 2022;12(1):14567. doi:10.1038/s41598-022-18850-0. [Google Scholar] [PubMed] [CrossRef]
31. Topuz M, Deniz M. Application of GIS and AHP for land use suitability analysis: case of Demirci district (Turkey). Humanit Soc Sci Commun. 2023;10(1):115. doi:10.1057/s41599-023-01609-x. [Google Scholar] [CrossRef]
32. Isaac AJB, Boniface HM, Mawazo JS, Pio KD. Land suitability evaluation for rainfed maize production using geospatial technologies in Nzara and Yambio counties, South Sudan. J Soil Sci Environ Manage. 2023;14(1):1–13. [Google Scholar]
33. Nungula EZ, Mugwe J, Massawe BHJ, Seleiman MF, Ali N, Gitari HI. GIS-AHP-based approach in land evaluation and suitability assessment for sunflower (Helianthus annus) production. Cogent Food Agric. 2024;10(1):2309831. doi:10.1080/23311932.2024.2309831. [Google Scholar] [CrossRef]
34. Yaman A. A GIS-based multi-criteria decision-making approach (GIS-MCDM) for the determination of the most appropriate site selection of an onshore wind farm in Adana, Turkey. Clean Techn Environ Policy. 2024. doi:10.1007/s10098-024-02866-3. [Google Scholar] [CrossRef]
35. Nikolić G, Vujović F, Golijanin J, Šiljeg A, Valjarević A. Modelling of wildfire susceptibility in different climate zones in montenegro using GIS-MCDA. Atmosphere. 2023;14(6):929. doi:10.3390/atmos14060929. [Google Scholar] [CrossRef]
36. SCET-Tunisia, ENHYD-Algéria. Hydro-agricultural improvements study of Mleta–Oran, Algeria. Algeria: National Office of Irrigation and Drainage; 2007. [Google Scholar]
37. Bouyssou D, Roy B. La notion de seuils de discrimination en analyse multicritère. INFOR. 1987;25(4):302–13 (In French). doi:10.1080/03155986.1987.11732046. [Google Scholar] [CrossRef]
38. Lehrer K. Conflict and consensus. Initiative: the Udall center for public policy. Initiative. 1993;5(2):11–3. [Google Scholar]
Cite This Article
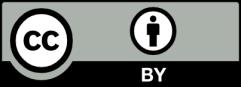
This work is licensed under a Creative Commons Attribution 4.0 International License , which permits unrestricted use, distribution, and reproduction in any medium, provided the original work is properly cited.