Open Access
ARTICLE
Delineating Groundwater Potential Zones Using Geospatial and Analytical Hierarchy Process Techniques in the Upper Omo-Gibe Basin, Ethiopia
1 Department of Geology and Environmental Geosciences, University of Dayton, Dayton, OH 45469, USA
2 Hanley Sustainability Institute, University of Dayton, Dayton, OH 45469, USA
3 Institute of Geophysics, Space Science and Astronomy, Addis Ababa University, Addis Ababa, 1000, Ethiopia
* Corresponding Author: Yonas Hagos. Email:
Revue Internationale de Géomatique 2024, 33, 399-425. https://doi.org/10.32604/rig.2024.053975
Received 15 May 2024; Accepted 28 August 2024; Issue published 27 September 2024
Abstract
In regions with unpredictable rainfall and limited water supply, it’s crucial to pinpoint areas with high potential for groundwater and find the best spots for groundwater resource development. This study utilizes the Analytic Hierarchy Process (AHP) in combination with Geographic Information Systems (GIS) to evaluate the potential groundwater zones in the Gombora watershed within the Omo Gibe basin in Ethiopia. Combining these two tools provided a detailed map showing potential groundwater areas. These zones are determined based on various thematic maps containing information about geology, soil texture, lineament density, slope, land use, and drainage density. The AHP method combines these data layers by assigning weights to each layer based on its importance for groundwater recharge. These weighted layers are then overlaid using a GIS platform to produce a conclusive map of potential groundwater areas. The groundwater potential within the watershed was qualitatively divided into five categories with area coverages of very good (1.6%), good (7.4%), moderate (21.4%), poor (51.6%), and very poor (17.9%) of the watershed area. The accuracy of the groundwater potential zones was evaluated using the receiver operating characteristic (ROC) curve and the area under the curve (AUC), producing good results (AUC = 75.5%). This research has shown that integrating AHP with GIS can effectively pinpoint potential groundwater zones. Additionally, the findings could play a key role in determining suitable locations for new groundwater wells and supplying valuable insights to decision-makers to aid in planning and implementing sustainable strategies for managing groundwater resources in the watershed.Keywords
Around half of the world’s population relies on groundwater as their primary source of freshwater. Agriculture, industry, and ecosystems depend on groundwater [1,2]. Nevertheless, in various regions worldwide, including Ethiopia, groundwater resources face severe threats stemming from overuse, rapid population growth, pollution, and the effects of climate change [3]. Over 80% of Ethiopia’s water supply comes from groundwater [4], and with increasing demand and climate change affecting its availability, exploration, and development of groundwater resources are essential to meet these evolving demands and ensure a dependable water supply [5–8]. A crucial aspect of effective groundwater management involves identifying and estimating groundwater potential [9,10]. This process includes evaluating the quantity and quality of groundwater resources and identifying the areas with the highest potential for groundwater development [9]. To ensure the long-term sustainability of groundwater resources, policymakers and stakeholders can use groundwater potential assessment to promote groundwater development and improve effective groundwater management [8,11,12]. Therefore, identifying sites with high groundwater potential in Ethiopia will support groundwater development and help address the critical water shortage for drinking, irrigation, and industry [9,13].
Geospatial technology provides a more efficient and cost-effective method for identifying groundwater potential zones compared with the traditional approaches. Traditional methods rely on ground surveys using geophysical, geological, and hydrogeological tools, which can be expensive, time-consuming [14–18], and often qualitative and subjective [19]. In contrast, geospatial tools allow for quick and affordable data analysis and modeling in various geoscience fields [20,21]. The geospatial mapping of groundwater potential has been used in several regions of the world, including China [22], Ethiopia [23], and India [24]. These techniques involve integrating different thematic layers such as land use, soil texture and depth, rainfall, and slope [25–28] to identify potential groundwater zones. By integrating multiple data sources, geospatial technology generates quantitative results that can be utilized for decision-making [19,25]. This approach is valuable for identifying and mapping potential groundwater zones, which is beneficial for the sustainable management of groundwater resources.
Numerous researchers have definitively utilized various techniques to define and map groundwater potential zones. For instance, some researchers have used machine learning techniques such as random forest (RF) [29–32], maximum entropy (ME) [33,34], probabilistic models such as artificial neural network model [35–39], certainty factor [40], decision tree [41,42], evidential belief function [43–45], frequency ratio [22,33,46–50], logistic regression [51–54], multi-criteria decision analysis [8,55–57], Shannon’s entropy [58,59], and weights-of-evidence [43,60,61]. Prior to employing expensive surveying methods, remote sensing, and GIS are used to economically identify potential groundwater locations [62]. To that end, Multi-Criteria Decision Analysis (MCDA) has recently been used in various research to evaluate groundwater potential zones [63–65]. According to Uc castillo et al. [66] and Doke et al. [67], the Analytical Hierarchy Process (AHP) is the most well-known and often applied technique used to define groundwater prospecting zones.
In the study area, the groundwater potential is poorly known and hasn’t been well-studied. Thus, the main objective of this study is to map groundwater potential zones in the Gombora watershed using the combination of geospatial and AHP techniques. The study’s outcome could provide valuable information for decision-makers, policymakers, and water resource planners to make effective and sustainable groundwater resource management.
2.1 Description of the Study Area
The Gombora watershed is located between 7°22′15′′ and 7°41′10′′ N latitude and 37°32′30′′ and 37°52′40′′ E longitude in Ethiopia. It covers an area of 614.7 km2 and is part of the Omo Gibe basin. The altitude of the area ranges from 998 meters above sea level at the Omo-Gibe River valley to 2817 m above sea level at peak Shonkola. The elevation generally decreases from east to west, with lower elevations in the western part of the watershed and higher elevations in the north and southeastern areas Fig. 1.
Figure 1: Location of the study area in relation to river basins in Ethiopia and the Omo-Gibe River basin
In the study watershed, there are four different geological units: Ignimbrite, Rhyolite and Ignimbrite, Pumice, tuff and ignimbrite, and Pre-rift volcanics, which cover 52.7, 125.1, 397.1, and 39.8 km2, respectively. With an aerial extent of 456.3 km2, light clay (Humic Nitisols) dominates the soil texture/type, followed by sandy clay loam (Chromic Luvisols), which covers 120.0 km2, and clay loam (Lithic Leptosols), which covers 38.3 km2 of the watershed. Cropland (392.9 km2) built area (155.0 km2), rangeland (34.6 km2), and forest land spanning an extent of 32.1 km2 of the total area make up the four land-use groups that characterize the research region. So far, the study area has been distinguished by slope gradients ranging from 0–409.5 in percent rise. The drainage density of the watershed ranges from 0 to 1.7 km/km2.
In the Gombora watershed, groundwater is primarily used for residential purposes, though occasionally, farmers use it to make up the difference in irrigation needs in various areas of the watershed. Even though several wells have been dug to help meet domestic water needs, a severe water shortage still requires immediate action. Thus, assessment of groundwater potential is essential to alleviate the water shortage and meet significant growing needs for irrigation and residential use.
The investigation utilized a variety of data and software (Table 1). A digital elevation model (DEM) with a resolution of 12.5 m was used to outline the study watershed boundary and produce slope and drainage density maps using ArcGIS. The lineament density map of the study area was generated using a Landsat 8 Operational Land Imager (OLI) satellite image obtained from the USGS website. Lineaments were automatically extracted using the line module of PCI Geomatica. The Geological Survey of Ethiopia provided the geological information, and the data from the Ministry of Water and Energy (MoWE) and Harmonized World Soil Database (HWSD) were used to prepare a soil map of the research area. The 10 m resolution land use/cover map for 2022 was acquired from https://livingatlas.arcgis.com/landcover/ (accessed on 24 July 2023). Finally, to validate the model’s output water well and spring location, the water well yield of the existing wells was collected from the zonal, regional, and federal water resources offices.
The factors impacting groundwater occurrence and flow were given weight after the thematic maps were created using ArcGIS 10.3.1 software. Engineers, geologists, hydrologists, and GIS experts provided input for the reclassification of factors and their sub-criteria in ArcGIS, and it was based on that input as well as previous literature [8,12,57,68,69]. Then, using weighted index overlay analysis, a groundwater potential map was created (Fig. 2).
Figure 2: Workflow of groundwater potential zone analysis for Gombora watershed
The geology of an area is crucial for assessing groundwater potential because it affects the porosity and permeability of rock formations. These factors directly influence the amount of water that can be stored and extracted from an aquifer or underground water source [8,12,71]. The ability of different rock types to hold water and allow its movement depends on their level of permeability and porosity [8,72]. For example, sandstones and fractured limestone are well-suited for groundwater storage and yield higher well outputs due to their high levels of porosity and permeability. Conversely, impermeable rock types such as volcanic rocks, metamorphic rocks, and some sedimentary rocks do not allow water to move through them easily and are therefore unsuitable as aquifers [73]. Identifying the most suitable drilling locations requires utilizing geological data to evaluate groundwater potential [74]. It is essential to have a comprehensive knowledge of geology to identify the most favorable hydrological conditions, reduce drilling costs, and improve sustainability and management practices in the use of groundwater resources [75].
The Gombora watershed is dominated by volcanic rocks (Fig. 3a). Four lithologic units are exposed in the area dominated by the Upper Miocene-Pliocene Nazareth group in the central part of the study area. The Nazareth group comprised of pumice, tuff, ash flows, ignimbrites, rhyolite, and trachyte. The northeastern low-lying areas along the Omo-Gibe River are characterized by alkaline to sub-alkaline Oligocene pre-rift volcanics with basaltic and rhyolitic composition. The northeastern part of the study area is dominated by pyroclastic flow deposits, ignimbrite, composed of a poorly sorted mixture of rock and pumice fragments and crystals in a volcanic ash groundmass. The rocks exposed in the southern part of the study area are mapped as a peralkaline silicic undifferentiated rock with rhyolites and ignimbrites. Generally, Ignimbrites are characterized as low-density and high-porosity rocks. However, their porosity and permeability can vary depending on factors such as the degree of welding and the presence of fractures or faults. Highly welded ignimbrites may have low porosity and permeability, while less welded and highly fractured ignimbrites may have higher porosity and permeability. The central and northeastern parts of the study are mainly comprised of unwelded tuff and pumice deposits, which might lead to high porosity and permeability. The spatial distribution of different rock types could control the degree and nature of groundwater recharge in the study area.
Figure 3: Geology (a); Lineament density (b); Slope (c); and Soil texture (d) maps of the study area
Lineament density is the quantity of linear geological structures on the surface that are expressions of underlying geological structures, such as faults and fractures, present in each area [76]. These lineaments can act as channels for subterranean groundwater movement, enhancing the likelihood of groundwater availability. In hydrogeological research and decision-making processes related to managing groundwater resources, the density of lineaments can substantially impact groundwater flow and potential assessment [8]. Due to the presence of natural fractures that allow water to pass through rocks and soils, areas with high lineament density have enhanced permeability [22,77–80]. Thus, areas with a high lineament density typically have higher rates of groundwater recharge and outflow [8,81,82]. Lineament density has been employed in several studies to evaluate the potential and vulnerability of groundwater [8,12,69].
To create the lineament density map, we utilized a Landsat 8 (OLI) satellite image captured on 19 February 2022. The line module of PCI Geomatica was employed to generate the lineament map [69]. Lineament density (Ld), which was estimated (Eq. (1)) to generate a lineament density map, is defined as the total length of lineaments per unit area [8,12,69,83].
where
Considering its significant impact on various aspects such as rainfall infiltration, runoff speed, and groundwater potential, the land slope is a crucial factor in assessing groundwater potential [71,73,74,81,84]. Due to limited infiltration and recharge, a high-slope location would have poor recharge prospects and weak groundwater potential [85]. On the other hand, gentle slopes provide great potential for groundwater recharging and high levels of precipitation infiltration [86]. The slope of an area impacts infiltration capacity, runoff retention on the soil surface, and runoff speed [87]. Flat lands are excellent for groundwater recharge capacity because they favor high infiltration rates and less surface runoff generation. On the contrary, there is less time for stormwater to penetrate in places with steep slopes, and rainfall is easily converted to runoff and runs down the slope quickly [88]. As a result, these locations have low groundwater potential.
The slope percentage map of the study area (Fig. 3c) was prepared from DEM with 12.5 m resolution using a spatial analysis tool in ArcGIS 10.3.1. The slope percentage of the study area varies between 0 and 409.5 %. The area under examination has been divided into five slope categories. About 21.8% of the landscape is under the gentle slope (S < 5%) category. In contrast, the moderate (5% < S ≤ 10%), moderately steep (10% < S ≤ 15%), very steep slope (15% < S ≤ 25%), and escarpment (S > 25%) covers about 29.4%, 18.8%, 18.3%, and 11.7% of the total area of the watershed, respectively.
It is crucial to consider soil texture when assessing groundwater potential [7,8,71,89]. The distribution of soil particles, including sand, silt, and clay, significantly affects groundwater recharge and storage. Clay soils have low porosity, which hinders rain percolation and increases surface runoff, while sandy soils, with their high porosity and permeability, allow water to percolate quickly [71]. Additionally, the composition, structure, and other physical characteristics of soil directly influence groundwater potential by affecting the retention and transmission of water [74].
Thus, soil plays a crucial role in determining groundwater potential due to its impact on water retention, infiltration, and transmission rates [12,71,74]. The research area features three primary soil types: sandy clay loam (Chromic Luvisols) prevalent in the southern and northern regions, clay loam (Lithic Leptosols) in the central low-lying areas, and light clay (Humic Nitisols) in the western part (see Fig. 3d). These soils demonstrate varying levels of infiltration, categorized as low to moderate based on the USDA-Soil Conservation Service classification system [90] and the Environmental Research Agency standards [91].
Land utilization and coverage play a vital role in assessing the potential of groundwater [57,92–95]. Land use and cover pertains to the utilization of land area in a specific region, encompassing forests, grasslands, urban areas, agricultural lands, and bodies of water [96,97]. Different types of land use and land cover (LULC) can have varying effects on groundwater recharge rates [94,98–100]. This link between groundwater availability and land use/cover is important for two main reasons. The amount of rainfall that percolates into the soil and replenishes groundwater aquifers is first affected by vegetation and ground cover [101]. Infiltration and groundwater recharge are promoted and enhanced by dense vegetation and ground cover [102]. Second, human activities can affect groundwater recharge, including land clearing and construction of infrastructures and facilities like roads, industrial parks, and storage sites [99,103].
The land cover map with a 10 m resolution was downloaded from the https://livingatlas.arcgis.com/landcover/ website for January 2022, accessed on 24 July 2023. The study area comprises four different types of land covers (Fig. 4a), with forestland, rangeland, farmland, and built area covering, respectively, 5.2%, 5.6%, 63.9%, and 25.2% of the total area.
Figure 4: Land use/cover (a) and drainage density (b) maps of the study area
The density and distribution of stream channels in a watershed are known as drainage density. It is calculated as the total length of the stream channels per unit area of a drainage basin [24,57]. Drainage density controls the flow of water over the ground surface, influencing surface runoff, infiltration, permeability, and subsequent recharge to the underlying aquifer [84,104,105]. Consequently, when evaluating groundwater potential zones, drainage density is taken into consideration [23,56]. Drainage density is inversely related to the permeability of a landscape and determines water movement. Thus, the groundwater recharge rate is higher for areas with low drainage density, whereas it gets lower with increasing drainage density [80]. Al-Ozeer et al. [106] and Raikwar et al. [107] show zones with low drainage density to have good groundwater potential, whereas Raikwar et al. [107] show areas with high drainage density to experience higher runoff.
To determine the drainage density of the Gombora watershed, we utilized a digital elevation model with a 12.5 m resolution on the ArcGIS 10.3.1 platform. As per Horton [108], drainage density was calculated by dividing the total length of the channels in a drainage basin by the size of the watershed (Eq. (2)).
where Dd is the drainage density, n is the number of streams, L is the stream length (km), and A is the drainage basin (km).
The Gombora watershed exhibits a drainage density ranging from 0 to 12.3 km−1, as depicted in Fig. 4b. The study area has been categorized into five drainage density classes based on their significance for groundwater storage: very poor (Dd > 12.3 km−1), poor (9.3 < Dd ≤ 12.3 km−1), good (6.2 < Dd ≤ 9.3 km−1), very good (3.1 < Dd ≤ 6.2 km−1), and excellent (Dd < 3.1 km−1). Approximately 54.5% and 30.5% of the area fall within the excellent (Dd < 3.1 km−1) and very good (3.1 < Dd ≤ 6.2 km−1) drainage density classes, respectively, indicating their significance for groundwater storage.
The data necessary for the study was collected from multiple sources (Table 1), and the potential groundwater zone was determined utilizing the Weighted Overlay tool within the ArcGIS Spatial Analyst Toolbox. This tool is grounded in the principles of Multi-Criteria Decision Analysis (MCDA). MCDA is a systematic approach used to weigh and assess diverse data about a particular issue with multiple criteria, enabling the identification of optimal solutions through comprehensive evaluation [109,110]. The methodology involves a transparent process of appraising and ranking alternatives, facilitating rational, transparent, and justifiable decision-making. The effectiveness of the approach lies in the collaboration of various stakeholders to reach a consensus on the most favorable course of action.
The groundwater potential zone was established and weighted using key parameters [8,12,68,69,111]. Six variables were chosen to define the groundwater potential zones in this study: geology, lineament density, slope, soil texture, land use/cover, and drainage density. These variables have varying effects on the identification of groundwater potential zones. The weight of each factor was determined by its impact on runoff speed, groundwater potential, aquifer recharge potential, and flow direction [8,12,57,69,112]. The reclassified and weighted factor maps are then overlaid to create the final groundwater potential zone map using the Weighted Overlay tool of the Spatial Analyst Toolbox in the ArcGIS 10.3.1 environment.
The analytical hierarchy process, also known as AHP, is an approach used to determine the normalized weights of the distinct themes and their various levels. In addition, it can be used to select the best option from a list of options. It was created in the 1980s by Dr. Thomas Saaty and has since gained widespread acceptance in industries like business, engineering, and social sciences. AHP entails dissecting difficult choices into smaller, more manageable components and methodically weighing the alternatives. It employs mathematical calculations to give decision criterion weights, simplifying alternatives based on the effect of the criteria on the specific case study.
The AHP process entails several processes, such as defining the issue at hand, listing potential solutions, determining evaluation criteria, and allocating weights to the criteria [57,112]. The judgment matrices are then created for each level/class of criteria in AHP, which assign weights to each one and assess each one relative importance using Saaty’s scalar from 1 to 9. It is done by reviewing literature, field observation, and expert judgment. On a scale of 1 to 9, the parameters used to zone groundwater potential areas are ranked, with 1 denoting equal importance and 9 denoting that one component is more crucial than the others [113,114]. One is less significant than the other when using the reciprocal of 1 to 9 (1/1 and 1/9) (Table 2). After assigning weights to the selected factors, the fundamental processes of establishing the indicator’s weight and consistency ratio (CR) were completed.
Step 1-Establishment of judgment matrices (P) by pair-wise comparison.
where n denotes the nth row, and m denotes the mth column elements of the judgment matrix.
The pairwise comparison between each thematic layer obtained based on the methods employed by several researchers in different case studies (Tables 3 and 4) [23,24,57,74,84,85,112,115,116].
Step 2-Calculation of normalized weight
The matrix will be normalized in this stage by adding the values in each column. The column sum is then divided by each entry’s normalized score to produce the result. Each column’s total is 1 (Tables 5 and 6).
where the geometric mean of the ith row of the judgment matrices is calculated as:
Step 3-Calculate a consistency ratio (CR) to verify the coherence of the judgments. The consistency ratio should now be calculated, and its value verified. This is being done to ensure that the initial preference ratings were accurate.
Consistency index (CI) is denoted as follows:
where max is the eigen value of the judgment matrix and it is calculated as:
where W is the weight vector (column). Random index (RI) can be obtained from standard tables (Table 7) [114]. In practical terms, a CR of 0.1 or less is thought to be acceptable. Any greater value at any level means that the verdicts need to be reviewed. The consistency ratio in this study is 0.02, which is acceptable (Table 8).
According to their significance in determining the groundwater potential zone, weight values were allocated for each factor and their subsequent classes, with the most significant factor having the highest weight and vice versa (Table 3). The weighted average of the factors in this study is 33.1% for geology, 26.2% for lineament density, 17.9% for slope, 11.2% for soil texture, 7.0% for land use, and 4.6% for drainage density (Table 9).
2.5 Delineation of Groundwater Potential Zones
Weights were assigned to the factors influencing the presence and movement of groundwater after thematic maps were created (Table 10). The groundwater prospective map was created using GIS environment-weighted index overlay analysis. A weighted linear combination approach was used to determine the groundwater potential index [117] (Eq. (9)). In general, the study procedure adopted was shown in the flowchart (Fig. 2).
where GWPI is the groundwater potential index; G is the score of geology; Ld is the score of lineament density; Sg is the score of slope gradient; S is the score of soil texture; Lu is the score of LULC; and Dd is the score of drainage density, and where the subscripts w and r refer to the weight of a theme and the rate of individual features of a theme, respectively.
According to the GWPI values, the groundwater potential zones can be classified as being either very good, good, moderate, poor, or very poor [56,57,68,118–120]. The outcome of the ArcGIS overlay analysis at the Gombora watershed quantifies the proportion of different groundwater potential zones as 1.6% (very good), 7.4% (good), 21.4% (moderate), 51.6% (bad), and 17.9% (very poor) (Fig. 5).
Figure 5: Groundwater potential zones class and their area coverage
As shown in Fig. 6, by giving high relative importance to the geology of the region and less importance to drainage density layers (Table 9), the northwestern, central, and northern part of the watershed is delineated as good and very good groundwater potential zones based on the AHP model. Those areas with good to very good groundwater potential could be due to the high porous and permeable rock media and high liniment density that favor groundwater recharge, storage, and movement. Moreover, these areas are characterized as sandy clay loam and flat slopes, which are the fundamental factors for groundwater potential prospecting. The poor to very poor potential zones are mainly distributed in areas having steep slopes, low lineament density, covered by clay soil, and high drainage density. This study clearly shows the influence of geologic features, lineament density, and slope in the groundwater potential zone delineation.
Figure 6: Groundwater potential zones, well yield in l/s, location of existing well and springs of the study area
The results of this study attest to the use of GIS and AHP techniques to delineate groundwater potential zones and provide a cost-effective assessment for further detailed investigation of groundwater development in Ethiopia. Similar geospatial and AHP methods were also successfully used in mapping groundwater potential in other parts of Ethiopia. For example, Duguma et al. [121] in the Guder watershed, Abay basin; Kabeto et al. [8] in the West Arsi Zone; Burayu [23] in the Didessa Sub-Basin, western Ethiopia; Seifu et al. [122] in the Fafen-Jerer sub-basin; Melese et al. [123] in the Muga watershed found that GIS and AHP techniques were efficient and useful in delineating and mapping groundwater potential zone. Overall, these studies show that GIS and AHP techniques are effective in identifying groundwater potential zones in Ethiopia. The results of this study are consistent with the findings of other studies, which have identified very poor to very good potential groundwater zones in different parts of the watershed. However, it is important to note that the methods and results of these studies may vary depending on the study area and the data used. Therefore, it is crucial to conduct site-specific studies to assess groundwater potential zones accurately.
Assessing groundwater potential is crucial for achieving Sustainable Development Goals (SDGs) 6, 12, and 13. Identifying viable groundwater resources allows us to guarantee access to clean water and sanitation (SDG 6), promote sustainable water use, reduce water waste (SDG 12), and improve climate resilience by mitigating the impacts of droughts and floods (SDG 13). This knowledge empowers us to make informed decisions for long-term water security and environmental sustainability. The proper management of water resources can be aided by the identification of groundwater potential zones [122]. The results of this investigation in delineating the groundwater potential zones in the Gombora watershed have significant ramifications for water resource management. Previous related research on Ethiopia’s groundwater potential zone mapping indicated that it could help groundwater well location, water management, better planning, and sustainable development [7,124,125]. Identifying groundwater potential zones may also assist in increasing water access. Water resource managers could focus on high groundwater zones and ensure water availability and sustainability of water resources [126]. Communities can obtain clean and safe drinking water, water for irrigation, and other uses by drilling wells in these areas. Furthermore, locating groundwater potential zones might aid in better urban planning. For instance, identifying the groundwater potential zones serves as a valuable tool when selecting suitable sites for new development to support the community and prevent overusing the area’s groundwater supplies. Thus, identifying groundwater potential zones would promote sustainable development.
The fundamental constraints of the groundwater potential zone assessment utilizing GIS coupled with AHP are not well outlined in most prior studies; however, the AHP method has some limitations. As an illustration, the AHP is a heuristic method that ranks criteria based on knowledge, which introduces subjectivity and prejudice [67]. Another drawback is that the input data, such as thematic layers and remote sensing data, may vary in quality and resolution, affecting the accuracy of the final map is [71]. AHP is a popular and well-known GIS-based technique for defining groundwater potential zones, but it might not be the most accurate [67], and the result might depend on available input data. This research defined the groundwater potential zone map using only six contributing parameters. To obtain better results, however, additional factors should be considered, including geomorphology, rainfall, curvature, the thickness of aquifers, and other datasets. Additional fieldwork and other relevant investigations, such as geophysical and hydrogeological, should be carried out to understand the area’s potential for groundwater overall. Regardless, the outcome of this study demonstrates that integrating GIS and AHP techniques can provide an effective first-order assessment of the groundwater potential zone in the Gombora watershed of the Omo-Gibe basin.
3.1 Groundwater Map Validation
Validating a groundwater map entail confirming the veracity of the data that went into its creation. This is accomplished by gathering field information on well locations, well discharge, and groundwater levels, and comparing it to the information used to produce the map [127]. Validation is done to make sure the groundwater map is accurate and gives decision-makers and stakeholders relevant information (Fig. 7).
Figure 7: Image of some Artesian well and springs around the Gombora watershed (Source taken by authors during field work)
Receiver Operating Characteristic (ROC) curve analysis was used in this study of groundwater potential zone assessment in the Gombora watershed to compare the locations of existing groundwater wells and validate the model output created by integrating GIS and MCDA [20]. The classification performance of a binary classifier is depicted graphically by the ROC curve. At various classification thresholds, it plots the true positive rate (TPR) along the y-axis and the false positive rate (FPR) along the x-axis. ROC curves are frequently employed in statistics, machine learning, and medical imaging applications. They assist academics and industry professionals in evaluating the performance of several models, choosing the best thresholds, and determining which model is best suited for a particular categorization challenge (Fig. 8).
Figure 8: ROC curve used for validation
The total performance of the classifier is frequently summarized using the area under the curve (AUC) measure. According to Naghibi et al. [59], the relationship between AUC and prediction accuracy is as follows: Excellent (0.9–1), Very Good (0.8–0.9), Good (0.7–0.8), Satisfactory (0.6–0.7), and Unsatisfactory (0.5–0.6) are all acceptable grades. A higher AUC implies better performance.
The ROC’s area under the curve (AUC) for this study is 0.747, which is a good performance (Fig. 9). In addition to the AUC curve value, we use the ground truth locations of high yield artesian wells and springs to validate the model output with the actual condition (Fig. 7).
Figure 9: ROC curve used for validation
The utilization of GIS combined with AHP for assessing groundwater potential zones has grown in popularity due to its efficiency in terms of time and cost-effectiveness as well as reduced labor-intensive requirements. This integration of AHP and geospatial technology has improved the precision and effectiveness of Groundwater potential zone identifications. The identification of groundwater potential zones can aid in more effective water resource management, better planning, increased water access, and sustainable development. In this study at the Gombora watershed, Omo Gibe basin, Ethiopia, a qualitative analysis was conducted to evaluate the groundwater potential zones using GIS and AHP techniques. Geology, lineament density, slope, soil texture, land use/cover, and drainage density were some of the six influencing thematic layers used to construct the delineated groundwater potential zone. Geology, with a weight of 33.1%, was found to be the most crucial factor in identifying a groundwater potential zone, followed by lineament density, slope, soil texture, land use, and drainage density, which had weights of 26.2%, 17.9%, 11.2%, 7.0%, and 4.6%, respectively.
A groundwater potential zones map was created after the obtained data were analyzed using the analytical hierarchy method and mapped using geographic information system techniques. According to the output of the model for groundwater potential zones, 1.6%, 7.4%, 21.4%, 51.6%, and 17.9% of the watershed are, respectively, in very good, good, moderate, poor, and very poor groundwater potential zones. The AUC value for this study was discovered to be 0.745, indicating good prediction capability of the AHP approach. Therefore, groundwater development activities can be carried out to increase the productivity of supplemental irrigation and household usage in such high groundwater prospect zones of the landscape. The methodology used in this study, which can be applied in other regions of the country, offers valuable insights for water resource planners in identifying possible groundwater development areas. To increase the accuracy of the groundwater potential evaluation, future research should add more data layers such as geomorphology, rainfall, curvature, and aquifer thickness. Additionally, the application of machine learning algorithms such as random forest and support vector machines may increase the accuracy of the groundwater potential evaluation. Furthermore, future studies could compare alternative weighting methods to identify which method offers the most accurate groundwater potential assessment, as well as study the impact of climate change on groundwater potential.
Acknowledgement: This research is supported by the National Science Foundation—Hydrologic Science Program. We would like to express our sincere gratitude to the Hadya Zone Water, Mine, and Energy Department Officer Melese Haile and Abayneh Selase who helped us in the data collection process and provided us existing well data’s. We would also like to extend our appreciation to our driver Mesay Gelanew who provided us with safe and reliable transportation during our fieldwork. Their assistance was invaluable in ensuring the success of our research project. Thank you for your contributions to our work!
Funding Statement: This research is supported by National Science Foundation Grant EAR 2132353.
Author Contributions: Conceptualization, Yonas Hagos, Zelalem Bedaso and Mulugeta Kebede; methodology, Yonas Hagos and Zelalem Bedaso; software, Yonas Hagos and Zelalem Bedaso; validation, Yonas Hagos and Zelalem Bedaso; formal analysis, Yonas Hagos and Zelalem Bedaso; investigation, Zelalem Bedaso, Yonas Hagos and Mulugeta Kebede; resources, Zelalem Bedaso, Yonas Hagos and Mulugeta Kebede; writing original draft preparation, Yonas Hagos; writing review and editing, Zelalem Bedaso and Mulugeta Kebede; visualization, Yonas Hagos and Zelalem Bedaso; supervision, Zelalem Bedaso and Mulugeta Kebede. All authors reviewed the results and approved the final version of the manuscript.
Availability of Data and Materials: The data that support the findings of this study are available from the corresponding author upon reasonable request.
Ethics Approval: Not applicable.
Conflicts of Interest: The authors declare that they have no conflicts of interest to report regarding the present study.
References
1. Nampak H, Pradhan B, Abd Manap M. Application of GIS based data driven evidential belief function model to predict groundwater potential zonation. J Hydrol. 2014;513:283–300. [Google Scholar]
2. Manap MA, Sulaiman WNA, Ramli MF, Pradhan B, Surip N. A knowledge-driven GIS modeling technique for groundwater potential mapping at the Upper Langat Basin. Malaysia Arab J Geosci. 2013;6:1621–37. [Google Scholar]
3. Sarkar S, Mukherjee A, Senapati B, Duttagupta S. Predicting potential climate change impacts on groundwater nitrate pollution and risk in an intensely cultivated area of South Asia. ACS Environ Au. 2022;2(6):556–76. doi:10.1021/acsenvironau.2c00042. [Google Scholar] [PubMed] [CrossRef]
4. Mengistu TD, Chung IM, Chang SW, Yifru BA, Kim MG, Lee J, et al. Challenges and prospects of advancing groundwater research in Ethiopian aquifers: a review. Sustainability. 2021;13(20):11500. doi:10.3390/su132011500. [Google Scholar] [CrossRef]
5. Godebo TR, Jeuland MA, Paul CJ, Belachew DL, McCornick PG. Water quality threats, perceptions of climate change and behavioral responses among farmers in the Ethiopian Rift Valley. Climate. 2021;9(6):92. doi:10.3390/cli9060092. [Google Scholar] [CrossRef]
6. Lin X. Promoting the sustainable utilization of groundwater resources in Ethiopia using the integrated groundwater footprint index (Honors Scholar Theses). University of Connecticut: USA; 2020. [Google Scholar]
7. Chatterjee S, Dutta S. Assessment of groundwater potential zone for sustainable water resource management in south-western part of Birbhum District, West Bengal. Appl Water Sci. 2022;12(3):40. doi:10.1007/s13201-021-01549-4. [Google Scholar] [CrossRef]
8. Kabeto J, Adeba D, Regasa MS, Leta MK. Groundwater potential assessment using GIS and remote sensing techniques: case study of West Arsi Zone, Ethiopia. Water. 2022;14(12):1838. doi:10.3390/w14121838. [Google Scholar] [CrossRef]
9. Kedir EG. Ground water potential assessment and sustainable management. Environ Monit Assess. 2023;195(7):891. doi:10.1007/s10661-023-11521-1. [Google Scholar] [PubMed] [CrossRef]
10. Priya U, Iqbal MA, Salam MA, Nur-E-Alam M, Uddin MF, Islam ARMT, et al. Sustainable groundwater potential zoning with integrating GIS, remote sensing, and AHP model: a case from North-Central Bangladesh. Sustainability. 2022;14(9):5640. doi:10.3390/su14095640. [Google Scholar] [CrossRef]
11. Derdour A, Benkaddour Y, Bendahou B. Application of remote sensing and GIS to assess groundwater potential in the transboundary watershed of the Chott-El-Gharbi (Algerian-Moroccan border). Appl Water Sci. 2022;12(6):136. doi:10.1007/s13201-022-01663-x. [Google Scholar] [CrossRef]
12. Andualem TG, Demeke GG. Groundwater potential assessment using GIS and remote sensing: a case study of Guna tana landscape, upper Blue Nile Basin, Ethiopia. J Hydrol: Reg Stud. 2019;24:100610. [Google Scholar]
13. Gorelick SM, Zheng C. Global change and the groundwater management challenge. Water Resour Res. 2015;51(5):3031–51. doi:10.1002/2014WR016825. [Google Scholar] [CrossRef]
14. Asoka A, Wada Y, Fishman R, Mishra V. Strong linkage between precipitation intensity and monsoon season groundwater recharge in India. Geophys Res Lett. 2018;45(11):5536–44. [Google Scholar]
15. Mukherjee S, Aadhar S, Stone D, Mishra V. Increase in extreme precipitation events under anthropogenic warming in India. Weather Clim Extrem. 2018;20:45–53. [Google Scholar]
16. Roxy MK, Ghosh S, Pathak A, Athulya R, Mujumdar M, Murtugudde R, et al. A threefold rise in widespread extreme rain events over central India. Nat Commun. 2017;8(1):708. doi:10.1038/s41467-017-00744-9. [Google Scholar] [PubMed] [CrossRef]
17. Senanayake IP, Dissanayake DMDOK, Mayadunna BB, Weerasekera WL. An approach to delineate groundwater recharge potential sites in Ambalantota, Sri Lanka using GIS techniques. Geosci Front. 2016;7(1):115–24. doi:10.1016/j.gsf.2015.03.002. [Google Scholar] [CrossRef]
18. Singh P, Thakur JK, Kumar S. Delineating groundwater potential zones in a hard-rock terrain using geospatial tool. Hydrol Sci J. 2013;58(1):213–23. doi:10.1080/02626667.2012.745644. [Google Scholar] [CrossRef]
19. Ibrahim-Bathis K, Ahmed SA. Geospatial technology for delineating groundwater potential zones in Doddahalla watershed of Chitradurga district, India. Egypt J Remote Sens Space Sci. 2016;19(2):223–34. doi:10.1016/j.ejrs.2016.06.002. [Google Scholar] [CrossRef]
20. Moghaddam DD, Rezaei M, Pourghasemi HR, Pourtaghie ZS, Pradhan B. Groundwater spring potential mapping using bivariate statistical model and GIS in the Taleghan watershed, Iran. Arab J Geosci. 2015;8(2):913–29. doi:10.1007/s12517-013-1161-5. [Google Scholar] [CrossRef]
21. Russo TA, Fisher AT, Lockwood BS. Assessment of managed aquifer recharge site suitability using a GIS and modeling. Groundwater. 2015;53(3):389–400. doi:10.1111/gwat.12213. [Google Scholar] [CrossRef]
22. Li S, Abdelkareem M, Al-Arifi N. Mapping groundwater prospective areas using remote sensing and GIS-Based data driven frequency ratio techniques and detecting land cover changes in the yellow river basin, China. Land. 2023;12(4):771. doi:10.3390/land12040771. [Google Scholar] [CrossRef]
23. Burayu DG. Identification of groundwater potential zones using AHP, GIS and RS integration: a case study of didessa sub-basin, western Ethiopia. Remote Sens Land. 2022;6:1–15. doi:10.21523/gcj1.2022060101. [Google Scholar] [CrossRef]
24. Arulbalaji P, Padmalal D, Sreelash K. GIS and AHP techniques based delineation of groundwater potential zones: a case study from southern Western Ghats, India. Sci Rep. 2019;9(1):51. 2082. doi:10.1038/s41598-019-38567-x. [Google Scholar] [PubMed] [CrossRef]
25. Danso SY, Ma Y. Geospatial techniques for groundwater potential zones delineation in a coastal municipality, Ghana. Egypt J Remote Sens Space Sci. 2023;26(1):75–84. doi:10.1016/j.ejrs.2022.12.004. [Google Scholar] [CrossRef]
26. Ajayi OG, Nwadialor IJ, Odumosu JO, Adetunji OO, Abdulwasiu IO. Assessment and delineation of groundwater potential zones using integrated geospatial techniques and analytic hierarchy process. Appl Water Sci. 2022;12(12):276. doi:10.1007/s13201-022-01802-4. [Google Scholar] [CrossRef]
27. Masoud AM, Pham QB, Alezabawy AK, El-Magd SAA. Efficiency of geospatial technology and multi-criteria decision analysis for groundwater potential mapping in a Semi-Arid region. Water. 2022;14(6):882. doi:10.3390/w14060882. [Google Scholar] [CrossRef]
28. Morgan H, Hussien HM, Madani A, Nassar T. Delineating groundwater potential zones in hyper-arid regions using the applications of remote sensing and GIS modeling in the eastern desert, Egypt. Sustainability. 2022;14(24):16942. doi:10.3390/su142416942. [Google Scholar] [CrossRef]
29. Madani A, Niyazi B. Groundwater potential mapping using remote sensing and random forest machine learning model: a case study from lower part of Wadi Yalamlam, Western Saudi Arabia. Sustainability. 2023;15(3):2772. doi:10.3390/su15032772. [Google Scholar] [CrossRef]
30. Morgan H, Madani A, Hussien HM, Nassar T. Using an ensemble machine learning model to delineate groundwater potential zones in desert fringes of East Esna-Idfu area, Nile valley, Upper Egypt. Geosci Lett. 2023;10(1):9. doi:10.1186/s40562-023-00261-2. [Google Scholar] [CrossRef]
31. Thanh NN, Chotpantarat S, Trung NH, Ngu NH. Mapping groundwater potential zones in Kanchanaburi Province, Thailand by integrating of analytic hierarchy process, frequency ratio, and random forest. Ecol Indic. 2022;145(2):109591. doi:10.1016/j.ecolind.2022.109591. [Google Scholar] [CrossRef]
32. Rahmati O, Pourghasemi HR, Melesse AM. Application of GIS-based data driven random forest and maximum entropy models for groundwater potential mapping: a case study at Mehran Region, Iran. Catena. 2016;137(1):360–72. doi:10.1016/j.catena.2015.10.010. [Google Scholar] [CrossRef]
33. Al-Abadi AM, Al-Temmeme AA, Al-Ghanimy MA. A GIS-based combining of frequency ratio and index of entropy approaches for mapping groundwater availability zones at Badra-Al Al-Gharbi-Teeb areas, Iraq. Sustain Water Resour Manag. 2016;2(3):265–83. doi:10.1007/s40899-016-0056-5. [Google Scholar] [CrossRef]
34. Salvacion AR. Groundwater potential mapping using maximum entropy. In: Water resources management and sustainability. Singapore: Springer Nature Singapore; 2022. p. 239–56. [Google Scholar]
35. Xu H, Yang X, Wang D, Hu Y, Shi Y, Cheng Z, et al. Predicting groundwater potential assessment in water-deficient islands based on convolutional neural networks. Egypt J Remote Sens Space Sci. 2022;25(4):1013–23. doi:10.1016/j.ejrs.2022.11.002. [Google Scholar] [CrossRef]
36. Tamiru H, Wagari M. Comparison of ANN model and GIS tools for delineation of groundwater potential zones, Fincha Catchment, Abay Basin, Ethiopia. Geocarto Int. 2022;37(23):6736–54. doi:10.1080/10106049.2021.1946171. [Google Scholar] [CrossRef]
37. Nguyen PT, Ha DH, Jaafari A, Nguyen HD, Van Phong T, Al-Ansari N, et al. Groundwater potential mapping combining artificial neural network and real AdaBoost ensemble technique: the DakNong province case-study, Vietnam. Int J Environ Res Public Health. 2020b;17(7):2473. doi:10.3390/ijerph17072473. [Google Scholar] [PubMed] [CrossRef]
38. Lee S, Hong SM, Jung HS. GIS-based groundwater potential mapping using artificial neural network and support vector machine models: the case of Boryeong city in Korea. Geocarto Int. 2018;33(8):847–61. doi:10.1080/10106049.2017.1303091. [Google Scholar] [CrossRef]
39. Lee S, Kim YS, Oh HJ. Application of a weights-of-evidence method and GIS to regional groundwater productivity potential mapping. J Environ Manag. 2012;96(1):91–105. doi:10.1016/j.jenvman.2011.09.016. [Google Scholar] [CrossRef]
40. Razandi Y, Pourghasemi HR, Neisani NS, Rahmati O. Application of analytical hierarchy process, frequency ratio, and certainty factor models for groundwater potential mapping using GIS. Earth Sci Inform. 2015;8(4):867–83. doi:10.1007/s12145-015-0220-8. [Google Scholar] [CrossRef]
41. Chen W, Wang Z, Wang G, Ning Z, Lian B, Li S, et al. Optimizing rotation forest-based decision tree algorithms for groundwater potential mapping. Water. 2023;15(12):2287. doi:10.3390/w15122287. [Google Scholar] [CrossRef]
42. Lee S, Lee CW. Application of decision tree model to groundwater productivity-potential mapping. Sustainability. 2015;7(10):13416–32. doi:10.3390/su71013416. [Google Scholar] [CrossRef]
43. Li Y, Abdelkareem M, Al-Arifi N. Mapping potential water resource areas using GIS-based frequency ratio and evidential belief function. Water. 2023;15(3):480. doi:10.3390/w15030480. [Google Scholar] [CrossRef]
44. Tahmassebipoor N, Rahmati O, Noormohamadi F, Lee S. Spatial analysis of groundwater potential using weights-of-evidence and evidential belief function models and remote sensing. Arab J Geosci. 2016;9(1):1–18. doi:10.1007/s12517-015-2166-z. [Google Scholar] [CrossRef]
45. Park I, Kim Y, Lee S. Groundwater productivity potential mapping using evidential belief function. Groundwater. 2014;52(S1):201–7. doi:10.1111/gwat.12197. [Google Scholar] [CrossRef]
46. Pawar U, Suppawimut W, Rathnayake U. Mapping of groundwater potential zones in a drought prone Marathwada Region using frequency ratio and statistical index methods. India Results Eng. 2024;22(4):101994. doi:10.1016/j.rineng.2024.101994. [Google Scholar] [CrossRef]
47. Abdekareem M, Abdalla F, Al-Arifi N, Bamousa AO, El-Baz F. Using remote sensing and GIS-based frequency ratio technique for revealing groundwater prospective areas at Wadi Al Hamdh watershed, Saudi Arabia. Water. 2023;15(6):1154. doi:10.3390/w15061154. [Google Scholar] [CrossRef]
48. Maity B, Mallick SK, Das P, Rudra S. Comparative analysis of groundwater potentiality zone using fuzzy AHP, frequency ratio and Bayesian weights of evidence methods. Appl Water Sci. 2022;12(4):63. doi:10.1007/s13201-022-01591-w. [Google Scholar] [CrossRef]
49. Muavhi N, Thamaga KH, Mutoti MI. Mapping groundwater potential zones using relative frequency ratio, analytic hierarchy process and their hybrid models: case of Nzhelele-Makhado area in South Africa. Geocarto Int. 2022;37(21):6311–30. doi:10.1080/10106049.2021.1936212. [Google Scholar] [CrossRef]
50. Guru B, Seshan K, Bera S. Frequency ratio model for groundwater potential mapping and its sustainable management in cold desert. India J King Saud Univ-Sci. 2017;29(3):333–47. doi:10.1016/j.jksus.2016.08.003. [Google Scholar] [CrossRef]
51. Nguyen PT, Ha DH, Avand M, Jaafari A, Nguyen HD, Al-Ansari N, et al. Soft computing ensemble models based on logistic regression for groundwater potential mapping. Appl Sci. 2020a;10(7):2469. doi:10.3390/app10072469. [Google Scholar] [CrossRef]
52. Chen W, Li H, Hou E, Wang S, Wang G, Panahi M, et al. GIS-based groundwater potential analysis using novel ensemble weights-of-evidence with logistic regression and functional tree models. Sci Total Environ. 2018;634:853–67. [Google Scholar] [PubMed]
53. Park S, Hamm SY, Jeon HT, Kim J. Evaluation of logistic regression and multivariate adaptive regression spline models for groundwater potential mapping using R and GIS. Sustainability. 2017;9(7):1157. doi:10.3390/su9071157. [Google Scholar] [CrossRef]
54. Ozdemir A. Using a binary logistic regression method and GIS for evaluating and mapping the groundwater spring potential in the Sultan Mountains (Aksehir, Turkey). J Hydrol. 2011;405(1–2):123–36. doi:10.1016/j.jhydrol.2011.05.015. [Google Scholar] [CrossRef]
55. Ghanim AA, Al-Areeq AM, Benaafi M, Al-Suwaiyan MS, Aghbari AAA, Alyami M. Mapping groundwater potential zones in the habawnah basin of Southern Saudi Arabia: an AHP- and GIS-based approach. Sustainability. 2023;15(13):10075. doi:10.3390/su151310075. [Google Scholar] [CrossRef]
56. Sathiyamoorthy M, Masilamani US, Chadee AA, Golla SD, Aldagheiri M, Sihag P, et al. Sustainability of groundwater potential zones in coastal areas of Cuddalore District, Tamil Nadu, South India using integrated approach of remote sensing, GIS and AHP techniques. Sustainability. 2023;15(6):5339. doi:10.3390/su15065339. [Google Scholar] [CrossRef]
57. Hagos YG, Andualem TG. Geospatial and multi-criteria decision approach of groundwater potential zone identification in Cuma sub-basin, Southern Ethiopia. Heliyon. 2021;7(9):e07963. doi:10.1016/j.heliyon.2021.e07963. [Google Scholar] [PubMed] [CrossRef]
58. Khoshtinat S, Aminnejad B, Hassanzadeh Y, Ahmadi H. Groundwater potential assessment of the Sero plain using bivariate models of the frequency ratio, Shannon entropy and evidential belief function. J Earth Sys Sci. 2019;128(6):1–16. doi:10.1007/s12040-019-1155-0. [Google Scholar] [CrossRef]
59. Naghibi SA, Pourghasemi HR, Pourtaghi ZS, Rezaei A. Groundwater qanat potential mapping using frequency ratio and Shannon’s entropy models in the Moghan watershed. Iran Earth Sci Inform. 2015;8(1):171–86. doi:10.1007/s12145-014-0145-7. [Google Scholar] [CrossRef]
60. Lee S, Song KY, Kim Y, Park I. Regional groundwater productivity potential mapping using a geographic information system (GIS) based artificial neural network model. Hydrogeol J. 2012;20(8):1511–27. doi:10.1007/s10040-012-0894-7. [Google Scholar] [CrossRef]
61. Rehman A, Islam F, Tariq A, Ul Islam I, Brian JD, Bibi T, et al. Groundwater potential zone mapping using GIS and Remote Sensing based models for sustainable groundwater management. Geocarto Int. 2024;39(1):2306275. doi:10.1080/10106049.2024.2306275. [Google Scholar] [CrossRef]
62. Hinton JC. GIS and remote sensing integration for environmental applications. Int J Geogr Inf Syst. 1996;10(7):877–90. [Google Scholar]
63. Alrawi I, Chen J, Othman AA. Groundwater potential zone mapping: integration of multi-criteria decision analysis (MCDA) and GIS techniques for the Al-Qalamoun Region in Syria. ISPRS Int J Geo Inf. 2022;11(12):603. doi:10.3390/ijgi11120603. [Google Scholar] [CrossRef]
64. Ejepu JS, Jimoh MO, Abdullahi S, Mba MA. Groundwater exploration using multi criteria decision analysis and analytic hierarchy process in Federal Capital Territory, Abuja, central Nigeria. Int J Geosci. 2022;13(1):33–53. doi:10.4236/ijg.2022.131003. [Google Scholar] [CrossRef]
65. Ghosh A, Adhikary PP, Bera B, Bhunia GS, Shit PK. Assessment of groundwater potential zone using MCDA and AHP techniques: case study from a tropical river basin of India. Appl Water Sci. 2022;12(3):37. doi:10.1007/s13201-021-01548-5. [Google Scholar] [CrossRef]
66. Uc Castillo JL, Martínez Cruz DA, Ramos Leal JA, Tuxpan Vargas J, Rodríguez Tapia SA, Marín Celestino AE. Delineation of groundwater potential zones (GWPZs) in a semi-arid basin through remote sensing, GIS, and AHP approaches. Water. 2022;14(13):2138. doi:10.3390/w14132138. [Google Scholar] [CrossRef]
67. Doke AB, Zolekar RB, Patel H, Das S. Geospatial mapping of groundwater potential zones using multi-criteria decision-making AHP approach in a hardrock basaltic terrain in India. Ecol Indic. 2021;127(3):107685. doi:10.1016/j.ecolind.2021.107685. [Google Scholar] [CrossRef]
68. Ahmad I, Dar MA, Andualem TG, Teka AH. Groundwater development using geographic information system. Appl Geomat. 2020a;12(1):73–82. doi:10.1007/s12518-019-00283-6. [Google Scholar] [CrossRef]
69. Tolche AD. Groundwater potential mapping using geospatial techniques: a case study of Dhungeta-Ramis sub-basin, Ethiopia. Geol Ecol Landsc. 2021;5(1):65–80. doi:10.1080/24749508.2020.1728882. [Google Scholar] [CrossRef]
70. Karra K, Kontgis C, Statman-Weil Z, Mazzariello JC, Mathis M, Brumby SP. Global land use/land cover with Sentinel 2 and deep learning. In: 2021 IEEE International Geoscience and Remote Sensing Symposium IGARSS, 2021 Jul; Brussels, Belgium: IEEE; p. 4704–7. [Google Scholar]
71. Jhariya DC, Khan R, Mondal KC, Kumar T, I. K, Singh VK. Assessment of groundwater potential zone using GIS-based multi-influencing factor (MIF), multi-criteria decision analysis (MCDA) and electrical resistivity survey techniques in Raipur city, Chhattisgarh, India. AQUA—Water Infrastruct, Ecosyst Soc. 2021;70(3):375–400. doi:10.2166/aqua.2021.129. [Google Scholar] [CrossRef]
72. Nigate F, Van Camp M, Yenehun A, Belay AS, Walraevens K. Recharge-discharge relations of groundwater in volcanic terrain of semi-humid tropical highlands of Ethiopia: the case of Infranz Springs, in the Upper Blue Nile. Water. 2020;12(3):853. doi:10.3390/w12030853. [Google Scholar] [CrossRef]
73. Abdullateef L, Tijani MN, Nuru NA, John S, Mustapha A. Assessment of groundwater recharge potential in a typical geological transition zone in Bauchi, NE-Nigeria using remote sensing/GIS and MCDA approaches. Heliyon. 2021;7(4):e06762. doi:10.1016/j.heliyon.2021.e06762. [Google Scholar] [PubMed] [CrossRef]
74. Guduru JU, Jilo NB. Groundwater potential zone assessment using integrated analytical hierarchy process-geospatial driven in a GIS environment in Gobele watershed, Wabe Shebele river basin, Ethiopia. J Hydrol: Reg Stud. 2022;44(7):101218. doi:10.1016/j.ejrh.2022.101218. [Google Scholar] [CrossRef]
75. Konikow LF, Bredehoeft JD. Groundwater resource development: effect and sustainability. Canada: USGS Publication Warehouse; 2020. [Google Scholar]
76. Murasingh S, Jha R. Identification of groundwater potential zones using remote sensing and GIS in a mine area of Odisha. In: National Conference on Recent Approaches to Water Resource Management, 2013; Dhanbad, India. doi:10.13140/RG.2.1.3374.6644. [Google Scholar] [CrossRef]
77. Manyoe IN, Hutagalung R. The extraction and analysis of lineament density from digital elevation model (dem) in libungo geothermal area, gorontalo. IOP Conf Ser: Earth Environ Sci. 2022;1089:012012. doi:10.1088/1755-1315/1089/1/012012. [Google Scholar] [CrossRef]
78. Kanagaraj G, Suganthi S, Elango L, Magesh NS. Assessment of groundwater potential zones in Vellore district, Tamil Nadu, India using geospatial techniques. Earth Sci Inform. 2019;12(2):211–23. doi:10.1007/s12145-018-0363-5. [Google Scholar] [CrossRef]
79. Abdullah TO, Ali SS, Al-Ansari NA, Knutsson S. Groundwater vulnerability mapping using lineament density on standard DRASTIC model: case study in Halabja Saidsadiq Basin, Kurdistan Region, Iraq. Engineering. 2015;7(10):644. doi:10.4236/eng.2015.710057. [Google Scholar] [CrossRef]
80. Sar N, Khan A, Chatterjee S, Das A. Hydrologic delineation of ground water potential zones using geospatial technique for Keleghai river basin. India Model Earth Syst Environ. 2015;1(3):1–15. doi:10.1007/s40808-015-0024-3. [Google Scholar] [CrossRef]
81. Hussein AA, Govindu V, Nigusse AGM. Evaluation of groundwater potential using geospatial techniques. Appl Water Sci. 2017;7(5):2447–61. doi:10.1007/s13201-016-0433-0. [Google Scholar] [CrossRef]
82. Rajaveni SP, Brindha K, Elango L. Geological and geomorphological controls on groundwater occurrence in a hard rock region. Appl Water Sci. 2017;7(3):1377–89. doi:10.1007/s13201-015-0327-6. [Google Scholar] [CrossRef]
83. Yeh HF, Cheng YS, Lin HI, Lee CH. Mapping groundwater recharge potential zone using a GIS approach in Hualian River, Taiwan. Sustain Environ Res. 2016;26(1):33–43. doi:10.1016/j.serj.2015.09.005. [Google Scholar] [CrossRef]
84. Ifediegwu SI. Assessment of groundwater potential zones using GIS and AHP techniques: a case study of the Lafia district, Nasarawa State. Nigeria Appl Water Sci. 2022;12(1):10. doi:10.1007/s13201-021-01556-5. [Google Scholar] [CrossRef]
85. Kadam AK, Umrikar BN, Sankhua RN. Assessment of recharge potential zones for groundwater development and management using geospatial and MCDA technologies in semiarid region of Western India. SN Appl Sci. 2020;2(2):1–11. doi:10.1007/s42452-020-2079-7. [Google Scholar] [CrossRef]
86. Maqsoom A, Aslam B, Khalid N, Ullah F, Anysz H, Almaliki AH, et al. Delineating groundwater recharge potential through remote sensing and geographical information systems. Water. 2022;14(11):1824. doi:10.3390/w14111824. [Google Scholar] [CrossRef]
87. Jourgholami M, Karami S, Tavankar F, Lo Monaco A, Picchio R. Effects of slope gradient on runoff and sediment yield on machine-induced compacted soil in temperate forests. Forests. 2020;12(1):49. doi:10.3390/f12010049. [Google Scholar] [CrossRef]
88. Rinderer M, Van Meerveld HJ, Seibert J. Topographic controls on shallow groundwater levels in a steep, prealpine catchment: when are the TWI assumptions valid? Water Resour Res. 2014;50(7):6067–80. doi:10.1002/2013WR015009. [Google Scholar] [CrossRef]
89. Thapa R, Gupta S, Guin S, Kaur H. Assessment of groundwater potential zones using multi-influencing factor (MIF) and GIS: a case study from Birbhum district, West Bengal. Appl Water Sci. 2017;7(7):4117–31. doi:10.1007/s13201-017-0571-z. [Google Scholar] [CrossRef]
90. USDA-SCS. Soil survey of travis county texas agricultural experiment station. Washington: USDA Soil Conservation Service; 1974. [Google Scholar]
91. Ethiopian Road Authority (ERA). Drainage design manual. Addis Ababa, Ethiopia; 2013. [Google Scholar]
92. Mengistu TD, Chung IM, Kim MG, Chang SW, Lee JE. Impacts and implications of land use land cover dynamics on groundwater recharge and surface runoff in East African Watershed. Water. 2022;14(13):2068. doi:10.3390/w14132068. [Google Scholar] [CrossRef]
93. Mensah JK, Ofosu EA, Yidana SM, Akpoti K, Kabo-bah AT. Integrated modeling of hydrological processes and groundwater recharge based on land use land cover, and climate changes: a systematic review. Environ Adv. 2022;8(7):100224. doi:10.1016/j.envadv.2022.100224. [Google Scholar] [CrossRef]
94. Owuor SO, Butterbach-Bahl K, Guzha AC, Rufino MC, Pelster DE, Díaz-Pinés E, et al. Groundwater recharge rates and surface runoff response to land use and land cover changes in semi-arid environments. Ecol Process. 2016;5(1):1–21. doi:10.1186/s13717-016-0060-6. [Google Scholar] [CrossRef]
95. Lerner DN, Harris B. The relationship between land use and groundwater resources and quality. Land Use Policy. 2009;26(3):S265–73. doi:10.1016/j.landusepol.2009.09.005. [Google Scholar] [CrossRef]
96. Potapov P, Hansen MC, Pickens A, Hernandez-Serna A, Tyukavina A, Turubanova S, et al. The global 2000-2020 land cover and land use change dataset derived from the Landsat archive: first results. Front Remote Sens. 2022;3:856903. doi:10.3389/frsen.2022.856903. [Google Scholar] [CrossRef]
97. Winkler K, Fuchs R, Rounsevell M, Herold M. Global land use changes are four times greater than previously estimated. Nat Commun. 2021;12(1):2501. doi:10.1038/s41467-021-22702-2. [Google Scholar] [PubMed] [CrossRef]
98. Salem A, Abduljaleel Y, Dezső J, Lóczy D. Integrated assessment of the impact of land use changes on groundwater recharge and groundwater level in the Drava floodplain, Hungary. Sci Rep. 2023;13(1):5061. doi:10.1038/s41598-022-21259-4. [Google Scholar] [PubMed] [CrossRef]
99. Siddik MS, Tulip SS, Rahman A, Islam MN, Haghighi AT, Mustafa SMT. The impact of land use and land cover change on groundwater recharge in northwestern Bangladesh. J Environ Manag. 2022;315(69):115130. doi:10.1016/j.jenvman.2022.115130. [Google Scholar] [CrossRef]
100. Abdelaziz KK, Nicaise Y, Séguis L, Ouattara I, Moussa O, Auguste K, et al. Influence of land use land cover change on groundwater recharge in the continental terminal area of Abidjan, ivory coast. J Water Resour Prot. 2020;12(5):431–53. doi:10.4236/jwarp.2020.125026. [Google Scholar] [CrossRef]
101. Ilstedt U, Bayala Tobella J, Bazié HR, Bayala J, Verbeeten E, Nyberg G, et al. Intermediate tree cover can maximize groundwater recharge in the seasonally dry tropics. Sci Rep. 2016;6(1):21930. doi:10.1038/srep21930. [Google Scholar] [PubMed] [CrossRef]
102. Brauman KA, Freyberg DL, Daily GC. Land cover effects on groundwater recharge in the tropics: ecohydrologic mechanisms. Ecohydrology. 2012;5(4):435–44. doi:10.1002/eco.236. [Google Scholar] [CrossRef]
103. Wang W, Chen Y, Chen Y, Wang W, Zhang T, Qin J. Groundwater dynamic influenced by intense anthropogenic activities in a dried-upriver oasis of central Asia. Hydrol Res. 2022;53(4):532–46. doi:10.2166/nh.2022.049. [Google Scholar] [CrossRef]
104. Vasileva T. An assessment of potential groundwater recharge zones in Bulgaria. Geologica Balcanica. 2019;48(1):43–61. doi:10.52321/geolbalc.48.1.43. [Google Scholar] [CrossRef]
105. Cotton CA. The control of drainage density. N Z J Geol Geophys. 1964;7(2):348–52. doi:10.1080/00288306.1964.10420180. [Google Scholar] [CrossRef]
106. Al-Ozeer AZ, Al-Abadi AM, Hussain TA, Fryar AE, Pradhan B, Alamri A, et al. Modeling of groundwater potential using cloud computing platform: a case study from Nineveh plain, Northern Iraq. Water. 2021;13(23):3330. doi:10.3390/w13233330. [Google Scholar] [CrossRef]
107. Raikwar V, Pagare P. Analitical study of stream frequency and drainage density of upper narmada basin: a remote sensing and GIS approach. J Glob Resour. 2019;6(1):179–82. doi:10.46587/jgr.2019.v06i01.028. [Google Scholar] [CrossRef]
108. Horton RE. Drainage-basin characteristics. Trans, Am Geophys Union. 1932;13(1):350–61. doi:10.1029/TR013i001p00350. [Google Scholar] [CrossRef]
109. Anteneh ZL, Alemu MM, Bawoke GT, Kehali AT, Fenta MC, Desta MT. Appraising groundwater potential zones using geospatial and multi-criteria decision analysis (MCDA) techniques in Andasa-Tul watershed, Upper Blue Nile basin, Ethiopia. Environ Earth Sci. 2022;81(1):1–20. doi:10.1007/s12665-021-10083-0. [Google Scholar] [CrossRef]
110. Bonilla Valverde JP, Blank C, Roidt M, Schneider L, Stefan C. Application of a GIS multi-criteria decision analysis for the identification of intrinsic suitable sites in Costa Rica for the application of managed aquifer recharge (MAR) through spreading methods. Water. 2016;8(9):391. doi:10.3390/W8090391. [Google Scholar] [CrossRef]
111. Çelik R. Evaluation of groundwater potential by GIS-based multicriteria decision making as a spatial prediction tool: case study in the Tigris River Batman-Hasankeyf Sub-Basin, Turkey. Water. 2019;11(12):2630. doi:10.3390/w11122630. [Google Scholar] [CrossRef]
112. Ahmad I, Dar MA, Andualem TG, Teka AH. GIS-based multi-criteria evaluation of groundwater potential of the Beshilo River basin, Ethiopia. J Afr Earth Sci. 2020b;164(1):103747. doi:10.1016/j.jafrearsci.2019.103747. [Google Scholar] [CrossRef]
113. Saaty TL. The analytic network process. In: Decision making with the analytic network process. International series in operations research & management science. Boston, MA: Springer; 2006. vol. 95. doi: 10.1007/0-387-33987-6_1. [Google Scholar] [CrossRef]
114. Saaty TL. The analytic hierarchy process: planning, priority setting, resource allocation. New York: McGraw-Hill International Book Co.; 1980. [Google Scholar]
115. Hagos YG, Andualem TG, Mengie MA, Ayele WT, Malede DA. Suitable dam site identification using GIS-based MCDA: a case study of Chemoga watershed, Ethiopia. Appl Water Sci. 2022;12(4):69. doi:10.1007/s13201-022-01592-9. [Google Scholar] [CrossRef]
116. Andualem TG, Hagos YG, Kefale A, Zelalem B. Soil erosion-prone area identification using multi-criteria decision analysis in Ethiopian highlands. Model Earth Syst Environ. 2020;6(3):1407–18. doi:10.1007/s40808-020-00757-2. [Google Scholar] [CrossRef]
117. Malczewski J. GIS and multicriteria decision analysis. New York: John Wiley & Sons; 1999. [Google Scholar]
118. Pande CB, Moharir KN, Panneerselvam B, Singh SK, Elbeltagi A, Pham QB, et al. Delineation of groundwater potential zones for sustainable development and planning using analytical hierarchy process (AHP), and MIF techniques. Appl Water Sci. 2021;11(12):186. doi:10.1007/s13201-021-01522-1. [Google Scholar] [CrossRef]
119. Ajay Kumar V, Mondal NC, Ahmed S. Identification of groundwater potential zones using RS, GIS and AHP techniques: a case study in a part of Deccan volcanic province (DVP), Maharashtra, India. J Indian Soc Remote Sens. 2020;48(3):497–511. doi:10.1007/s12524-019-01086-3. [Google Scholar] [CrossRef]
120. Machiwal D, Jha MK, Mal BC. Assessment of groundwater potential in a semi-arid region of India using remote sensing, GIS and MCDM techniques. Water Resour Manag. 2011;25(5):1359–86. doi:10.1007/s11269-010-9749-y. [Google Scholar] [CrossRef]
121. Duguma TA, Duguma GA. Assessment of groundwater potential zones of upper Blue Nile River Basin using multi-influencing factors under GIS and RS environment: a case study on guder watersheds, Abay basin, Oromia region, Ethiopia. Geofluids. 2022;2022(1):1–26. doi:10.1155/2022/1172039 2022. [Google Scholar] [CrossRef]
122. Seifu TK, Ayenew T, Woldesenbet TA, Alemayehu T. Identification of groundwater potential sites in the drought-prone area using geospatial techniques at Fafen-Jerer sub-basin, Ethiopia. Geol Ecol Landsc, 2022;8:1–13. [Google Scholar]
123. Melese T, Belay T. Groundwater potential zone mapping using analytical hierarchy process and GIS in Muga Watershed, Abay Basin, Ethiopia. Glob Chall. 2022;6(1):2100068. doi:10.1002/gch2.202100068. [Google Scholar] [PubMed] [CrossRef]
124. Duguma TA. RS and GIS analysis of the groundwater potential zones in the Upper Blue Nile River Basin, Ethiopia. J Hydrol: Reg Stud. 2023;46(3):101344. doi:10.1016/j.ejrh.2023.101344. [Google Scholar] [CrossRef]
125. Pal S, Kundu S, Mahato S. Groundwater potential zones for sustainable management plans in a river basin of India and Bangladesh. J Clean Prod. 2020;257(13):120311. doi:10.1016/j.jclepro.2020.120311. [Google Scholar] [CrossRef]
126. Ashwini K, Verma RK, Sriharsha S, Chourasiya S, Singh A. Delineation of groundwater potential zone for sustainable water resources management using remote sensing-GIS and analytic hierarchy approach in the state of Jharkhand. India Groundwater Sustain Dev. 2023;21:100908. doi:10.1016/j.gsd.2023.100908. [Google Scholar] [CrossRef]
127. Ojo JS, Olorunfemi MO, Akintorinwa OJ, Bayode S, Omosuyi GO, Akinluyi FO. GIS integrated geomorphological, geological and geoelectrical assessment of the groundwater potential of Akure Metropolis, southwest Nigeria. J Earth Sci Geotech Eng. 2015;5(14):85–101. [Google Scholar]
Cite This Article
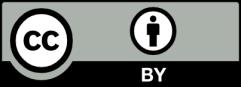
This work is licensed under a Creative Commons Attribution 4.0 International License , which permits unrestricted use, distribution, and reproduction in any medium, provided the original work is properly cited.