Open Access
REVIEW
Multi-Aspect Critical Assessment of Applying Digital Elevation Models in Environmental Hazard Mapping
1 Faculty of Civil Engineering, Damascus University, P.O. Box 30621, Damascus, Syria
2 Research Institute of Sciences and Engineering, University of Sharjah, P.O. Box 27272, Sharjah, United Arab Emirates
3 Surveying Engineering Department, and Environmental Engineering, Lebanese International University, P.O. Box 146404, Beirut, Lebanon
* Corresponding Author: Maan Habib. Email:
Revue Internationale de Géomatique 2024, 33, 247-271. https://doi.org/10.32604/rig.2024.053857
Received 11 May 2024; Accepted 08 July 2024; Issue published 07 August 2024
Abstract
Digital elevation models (DEMs) are essential tools in environmental science, particularly for hazard assessments and landscape analyses. However, their application across multiple environmental hazards simultaneously remains in need for a multi-aspect critical assessment to promote their effectiveness in comprehensive risk management. This paper aims to review and critically assess the application of DEMs in mapping and managing specific environmental hazards, namely floods, landslides, and coastal erosion. In this regard, it seeks to promote their utility of hazard maps as key tools in disaster risk reduction and environmental planning by employing high-resolution DEMs integrated with advanced geographic information systems. The findings offer valuable insights into optimizing DEM technology for environmental management, contributing to safer and more resilient communities. The paper addresses an important gap in the geospatial analysis of natural hazards and serves as a foundational reference for future advancements in the field, emphasizing its importance to academic researchers and practical stakeholders in environmental and disaster management.Keywords
Integrating digital elevation models (DEMs) into environmental hazard mapping represents a pivotal development in geospatial sciences [1–4]. DEMs are essential tools for accurately assessing and managing environmental hazards such as floods [5,6], landslides [7–9], and coastal erosion [10–13]. They are widely used in environmental-related studies, particularly in hydrological and hydraulic models, where the high resolution of these models significantly affects the accuracy of hazard assessments [13–16]. Their importance is underscored by the increased frequency of these hazards, driven by climate change and urban expansion. DEMs are fundamental in determining landscapes and analyzing topography and are instrumental in enhancing the precision and utility of hazard maps, which are vital tools in disaster risk reduction [17–20]. DEMs provide fundamental elevation data that enable researchers to identify regions susceptible to environmental hazards by determining watershed boundaries, identifying flood plains, highlighting seismic affected areas, and recognizing steep slopes that can predispose areas to landslides [21–23]. Recent studies have emphasized the role of DEM resolution in the performance of machine learning models for predicting flood probabilities, indicating that finer resolutions can substantially improve prediction accuracy [24–27]. In addition, the technological advancements in DEMs have expanded their application in environmental management [28–30]. This includes the fusion of multiple DEM sources to enhance model accuracy, which has proven essential in refining the precision of hazard maps crucial for disaster risk planning [31,32]. In addition, integrating DEMs with geographic information systems (GIS) has revolutionized environmental planning and hazard management, allowing for more detailed and accurate mapping and significantly enhancing disaster preparedness and response strategies [9,33–35]. The use of LiDAR-derived DEMs has been highlighted for its effectiveness in flood applications, providing detailed and accurate topographical data that improve flood risk assessments [36,37]. Integrating UAV-derived DEMs demonstrates substantial potential, particularly in small-scale hazard mapping and monitoring geo-hazards in challenging terrains [38,39].
Although the utility of DEMs in hazard mapping is well-established, existing literature reveals several current problems. One significant issue is the gap in the simultaneous application across various types of environmental hazards. Most studies focus on a single type of hazard, leading to deficiency in comprehensive risk assessments that integrate high-resolution DEMs with other geospatial technologies across multiple hazard types. This lack of integration limits the ability to provide a holistic view of environmental risks, which is crucial for effective disaster preparedness. In addition, there is a gap in the application of machine learning techniques in enhancing DEM-based environmental hazard mapping. Machine learning methods can significantly improve the predictive accuracy of hazard maps by learning complex patterns from large and diverse datasets that traditional methods cannot capture. These techniques can refine the resolution and accuracy of DEMs, optimize hazard prediction models, and facilitate the development of more robust and comprehensive hazard assessment tools [40–42].
The potential for machine learning in this context is vast, as it can analyze the interactions between multiple hazard types and their environmental impacts more efficiently than conventional statistical methods. For instance, Su et al. [43] demonstrated the application of deep learning for assessing earthquake disaster chains, suggesting that similar methodologies can enhance multi-hazard models by integrating various data types and sources, including high-resolution DEMs. In addition, the integration of DEMs with other geospatial technologies, such as remote sensing and GIS, has been shown to effectively map hazards such as floods, landslides, and soil erosion, but the simultaneous use of these technologies across multiple hazard scenarios remains underexplored [44–47]. These gaps present significant opportunities for future research, where the development of integrated, multi-hazard assessment models can significantly increase the accuracy and efficiency of hazard maps, thus improving disaster risk management strategies. This study aims to review and critically assess the application of DEMs in mapping and managing specific environmental hazards, focusing on floods, landslides, and coastal erosion. The study seeks to enhance the accuracy and utility of hazard maps by employing high-resolution DEMs integrated with advanced GIS and innovative machine learning techniques. These improved maps are expected to provide more detailed and precise hazard assessments, crucial for effective disaster risk reduction and strategic environmental planning. In addition, the study aims to develop a robust framework that utilizes the combined capabilities of high-resolution DEMs, GIS, and machine learning to create a multi-hazard assessment tool, identifying potential hazard zones with greater precision and predicting the severity and probable impact of these hazards under various environmental scenarios. This approach intends to fill existing gaps in current hazard mapping practices, which often do not simultaneously consider multiple types of hazards or fully utilize the potential of modern technological advancements. The findings offer valuable insights into optimizing DEM technology for environmental management, contributing to safer and more resilient communities. This research addresses an important gap in the geospatial analysis of natural hazards and serves as a foundational reference for future advancements in the field, emphasizing its importance to academic researchers and practical stakeholders in environmental and disaster management.
A bibliometric assessment was conducted to evaluate the existing literature on the application of DEMs in environmental hazard mapping. A search was performed on the Web of Science platform, yielding a total of 2110 documents relevant to the study. The temporal distribution of publications from 2000 to 2023 is illustrated in Fig. 1, showing a steady increase in research activity over the years, with growth after 2010. The analysis of keyword co-occurrence within these publications reveals the most frequently used terms, including “hazard,” “GIS,” “model,” “LIDAR,” “remote sensing,” “uncertainty,” “risk,” “earthquake,” “vulnerability,” “climate change,” “deformation,” “landslide,” “evolution,” “susceptibility,” and “logistic regression,” as depicted in Fig. 2. These keywords highlight the multidisciplinary nature of the research and the integration of various technologies and methodologies in the field.
Figure 1: Number of publications related to DEM applications in environmental hazard mapping over the period between 2000 and 2023
Figure 2: Co-occurrence of keywords in research related to DEM applications in environmental hazard mapping
Additionally, the assessment identified the journals that published the most research on DEM applications in environmental hazard mapping. The prominent journals include Natural Hazards and Earth System Sciences, Natural Hazards, Geomorphology, Remote Sensing, Landslides, Environmental Earth Sciences, Geomatics Natural Hazards and Risk, and ISPRS International Journal of Geo-Information, as shown in Fig. 3. These journals are pivotal in disseminating advancements and findings related to DEM applications in hazard mapping. Citation analysis by country, as presented in Fig. 4, indicates that China, the USA, Italy, Germany, England, France, India, and Switzerland are the leading contributors to this research domain. This geographical distribution underscores the global interest and collaboration in utilizing DEMs for environmental hazard assessment and management. The bibliometric assessment demonstrates the evolving and expanding body of knowledge in the application of DEMs for environmental hazard mapping, emphasizing the importance of interdisciplinary approaches and international collaboration in advancing this critical field.
Figure 3: Journals with most publications of research related to DEM applications in environmental hazard mapping
Figure 4: Citation analysis by country in research related to DEM applications in environmental hazard mapping
This section outlines the materials and methods employed in the study to evaluate the effectiveness of DEMs in environmental hazard mapping. The methodology is designed to explore the integration of high-resolution DEMs with advanced GIS and machine learning techniques to enhance the accuracy and utility of hazard maps. Fig. 5a illustrates the integration process of DEMs, GIS, and machine learning in creating a comprehensive multi-hazard assessment tool. The framework is divided into several components, each representing a step in the process from data acquisition to hazard mitigation and planning. However, Fig. 5b describes the general approach applied in this study. This includes a step-by-step depiction of the operational workflow, which helps in understanding the systematic application of integrated technologies and methodologies to address the complexities of environmental hazard mapping and provides a clear roadmap for achieving the research objectives.
Figure 5: Comprehensive framework and methodology for multi-hazard assessment: (a) Conceptual framework and (b) Schematic illustration of the research methodology
3.1 Data Collection and Sources
The successful application of DEMs in environmental hazard mapping fundamentally depends on the accuracy of the elevation data. DEMs are pivotal in the mapping process, providing detailed representations of surface terrains essential for identifying and analyzing various environmental hazards. The precision of these models is crucial; even minor inaccuracies in elevation data can lead to significant errors in hazard identification and subsequent mitigation strategies [48,49]. Therefore, selecting high-quality DEMs, characterized by their resolution, accuracy, and frequency of updates, is imperative for maintaining the integrity of hazard mapping efforts. This section investigates the diverse data sources used in hazard mapping, detailing their inherent characteristics such as spatial resolution, data collection methodologies, and both vertical and horizontal accuracy. It also examines the coverage of each dataset. The application of each DEM in multi-hazard assessments is discussed, indicating how their unique attributes meet the specific demands of different environmental hazards, including floods, landslides, and coastal erosion. This study aims to underscore the vital role of accurate and detailed elevation data in supporting the capacity for effective environmental and disaster management through refined hazard mapping techniques by exploring these aspects. Table 1 lists key factors in developing effective DEMs for environmental hazard mapping.
Algorithm 1 depicts a pseudocode for applying DEMs in hazard analysis. The procedure begins with Data Acquisition, where elevation data is collected from various sources. Following the acquisition, the Data Preprocessing phase involves filtering and smoothing the data to eliminate noise and correct errors, which is vital for maintaining the DEM’s accuracy and reliability. In the Construct DEM phase, a grid structure is created at the desired resolution, and elevation values are assigned to each grid cell. For cells lacking data, interpolation methods are employed to estimate values, ensuring a complete and continuous elevation model. Terrain Analysis follows, calculating essential terrain attributes crucial for assessing the terrain’s susceptibility to hazards. Integrate Additional Datasets involves incorporating additional data such as hydrological, geological, and land use information into the DEM. Hazard-specific modeling then applies risk models based on the DEM and additional data to define hazard zones. Finally, Validation and Update tests the DEM against historical data to ensure accuracy.
The accuracy evaluation of DEMs is conducted by comparing the elevation data from the DEMs to ground control points (GCPs) collected using differential GPS. This process involves statistical measures such as root mean square error (RMSE) and mean absolute error (MAE) to quantify the differences [13,50]. High-resolution DEMs from sources such as satellite imagery and aerial surveys are pre-processed for spatial resolution and coordinate systems consistency. The resulting accuracy metrics provide a quantitative basis for assessing the reliability of the DEMs in representing the actual terrain, ensuring the precision required for effective environmental hazard mapping. The generated hazard maps are also validated using historical hazard event data and field observations. The spatial extent and severity of the hazards, as predicted by the DEMs, are compared to the actual events to assess the predictive capability of the models.
Table 2 compares various DEMs, examining how their source, characteristics such as spatial resolution, and data collection methods impact the accuracy of hazard assessment. It highlights the trade-offs between high-resolution DEMs, such as those from LiDAR, which provide detailed data for precise modeling but may be costly and less available.
Table 3 categorizes different natural hazards, explains how DEMs are applied for each hazard type, outlines key methodologies used, and discusses the benefits and challenges of employing DEMs in these settings. It also emphasizes the practical significance of DEMs in enhancing hazard mapping and mitigation efforts. Fundamental DEM analysis techniques include slope stability analysis for identifying areas prone to landslides and hydrological modeling for delineating floodplains and analyzing water flow dynamics. DEMs are also instrumental in tsunami inundation mapping, providing detailed coastal elevation profiles to predict the inland travel of tsunami waves [57].
Probabilistic risk assessment (PRA) is a systematic and comprehensive methodology employed to evaluate risks associated with safety-critical systems [58,59]. It calculates the probability of adverse events and their potential impacts to enhance decision-making and boost safety and operational integrity. The process begins with hazard identification, utilizing tools such as failure mode and effects analysis (FMEA) or hazard and operability study (HAZOP) to systematically detect and categorize possible risks. Event tree analysis (ETA) is then employed to explore different accident scenarios. This technique starts with an initiating event and follows possible progression paths, incorporating system responses and safety measures. Each path represents events that can lead to a specific outcome. Simultaneously, fault tree analysis (FTA) analyzes the probability of a particular adverse event by constructing a logical diagram that lays out various mechanical or human failures that could lead to the undesired event. This helps identify root causes and system vulnerabilities. Probability determination is then performed, calculating the likelihood of different failure scenarios using statistical data, historical failure rates, and expert judgments. This phase often involves complex mathematical models. The results support risk quantification, combining the probability analysis with the potential consequences of each event, typically expressed as a risk index to prioritize risks and evaluate mitigation strategies. Based on the findings, mitigation and decision-support strategies are developed to reduce the likelihood or impact of hazardous events. PRA provides objective data crucial for making informed decisions on risk management. Finally, continuous review and update are essential as systems and environments evolve with regular monitoring and refinement of risk models to maintain the relevance and effectiveness of the assessment in risk management.
Applying DEMs in environmental hazard mapping has caused significant advances in managing and assessing various natural hazards. This section introduces a series of case studies that illustrate the practical application of DEMs in mapping various environmental hazards. Each case study focuses on a specific type of hazard, including seismic events, floods, landslides, and coastal erosion, and illustrates how integrating DEMs with GIS and machine learning techniques can enhance hazard assessment and planning [60,61]. This structured approach details the methodology and findings from each case study and connects them under the broader theme of improving hazard preparedness through technological innovation. The following case studies underline the versatility and effectiveness of DEMs in environmental hazard assessment across different contexts.
Manfreda et al. [62] developed a DEM-based method to estimate flood inundation depth. Al-Areeq et al. [63] demonstrated using DEMs for flood hazard analysis in complex terrains such as Jeddah, Saudi Arabia, enhancing the understanding of flood dynamics in urban settings. Sulaiman et al. [64] explored the extraction of DEMs using remote sensing and GIS for flood risk assessment, providing insights into the practical applications of these technologies in urban planning. Niyongabire et al. [65] indicated that DEMs in GIS are used for flood susceptibility mapping in Bujumbura City, highlighting the critical role of geospatial technologies in urban disaster risk management. Meena et al. [66] highlighted the effect of spatial resolution of DEMs on the accuracy of landslide susceptibility maps. Rabby et al. [8] evaluated DEM effects in landslide mapping in Rangamati District, Bangladesh. Brock et al. [9] investigated the impact of the resolution and quality of DEMs on the performance of landslide susceptibility models and indicated that it heavily relies on the quality of the underlying DEMs.
Jibson et al. [67,68] developed methodologies for digital probabilistic seismic landslide hazard mapping, enhancing risk management strategies in seismically active regions. In addition, Li et al. [69] employed Monte Carlo simulations for probabilistic seismic landslide hazard mapping. They reported that advancements in computational techniques with reliable DEM data can significantly improve hazard prediction and risk assessment. Haneberg [70] discussed the effects of DEM errors on seismic slope stability calculations, marking a pivotal study in understanding the impact of DEM errors on seismic hazard assessments. Ahmad et al. [71] conducted a comprehensive seismic hazard assessment of Syria, incorporating seismicity, DEMs, slope data, active faults, and GIS. They illustrated the comprehensive approach required to assess seismic risks effectively. Theilen-Willige et al. [72] performed seismic hazard analysis along the Koyna Dam area in India, exhibiting the effective integration of remote sensing and GIS with DEMs to understand and mitigate seismic hazards. Marfai et al. [73] utilized high-resolution UAV-derived DEMs to plan tsunami vertical evacuation routes in Indonesia, demonstrating the importance of precise elevation data in coastal hazard planning. Demirkesen et al. [74] conducted a coastal flood risk analysis in Izmir, Turkey, utilizing Landsat-7 ETM+ imagery and SRTM DEM. They demonstrated the enhancement of hazard analysis by integrating DEMs with other geospatial data.
These case studies collectively illustrate the critical role of DEMs in environmental hazard mapping. They underscore the necessity for high-resolution and accurate DEMs and demonstrate the diverse applications of this technology across various types of environmental hazards. Each study contributes to a broader understanding and application of DEMs in risk reduction, emphasizing their importance to academic researchers and practical stakeholders in environmental and disaster management.
4 Critical Analysis of DEM Applications in Hazard Mapping
This section presents a critical analysis to explore the applications, benefits, challenges, and practical implications of using DEMs in environmental hazard mapping, highlighting critical areas for improvement and future research. The case studies highlighted numerous applications of DEMs across different environmental hazards [21,63]. Each case study demonstrated the specific capabilities of DEMs in hazard mapping and revealed the improvements in hazard preparedness enabled by technological advancements. The effectiveness of DEMs can vary significantly based on the resolution, quality, and the specific environmental hazard being assessed [75]. Although high-resolution DEMs offer greater detail and accuracy, they require substantial computational resources and are more expensive. Lower-resolution DEMs, while less costly and computationally demanding, cannot provide sufficient detail for all types of hazard assessments, particularly in complex urban or densely vegetated areas. Table 4 categorizes strategic measures to enhance the application of DEMs in environmental hazard mapping. Each category addresses specific aspects, from technical challenges and operational needs to capacity building and policy support, ensuring a comprehensive strategy for implementing DEM technology in hazard assessments.
The critical role of DEMs in environmental hazard mapping carries significant implications for environmental management. Initially, the ability of DEMs to provide detailed topographical data aids decision-makers in planning and implementing effective mitigation strategies, such as flood barriers or landslide warning systems [76,77]. These tools are essential for reducing the potential impact of natural disasters on vulnerable communities and infrastructures. In addition, integrating DEMs with GIS and machine learning opens up new methods for predictive hazard modeling, allowing for more proactive management strategies. This approach helps in immediate hazard response and long-term planning for climate change impacts, such as sea-level rise and increased frequency of extreme weather events.
DEMs are crucial in hydrological modeling, particularly in flood simulation and management. They provide detailed information about land surface and riverbed elevations, essential for modeling water flow paths and accumulation zones [5,6]. High-resolution DEMs enable accurate delineation of floodplains and identification of flood-prone areas, facilitating effective emergency planning and community alerts. For example, integrating DEMs with GIS and real-time meteorological data can enhance predictive models, allowing for dynamic flood risk assessments that support timely and targeted responses [78]. The role of DEMs in landslide risk assessment is primarily related to their ability to provide detailed data on terrain slope, aspect, and elevation, all critical factors in determining landslide susceptibility. Scientists can predict potential landslide hotspots by analyzing these terrain parameters, especially in mountainous regions where natural disasters frequently occur. This predictive capability supports land use planning and infrastructure development, aiming to mitigate the impacts of landslides on human settlements and critical transport routes. In seismic hazard assessment, DEMs are employed to model ground shaking and to identify fault zones where earthquakes are likely to occur. Elevation data contribute to the understanding of tectonic settings and the mapping of seismic risk areas [69,79]. This information is vital for urban planning and the design of earthquake-resistant structures, which are critical in minimizing human and economic losses during seismic events. DEMs facilitate the monitoring and prediction of coastal erosion, providing essential data that helps understand how waves and tidal patterns affect shoreline changes [80,81]. This application is critical in the context of rising sea levels and increased storm activity associated with climate change. Coastal managers use DEMs to plan protective measures, such as sea walls and restored habitats, to prevent loss of land and protect coastal communities.
Despite their advantages, the application of DEMs in environmental hazard mapping is limited by several factors. The resolution and coverage of DEM data cannot adequately capture detailed terrain features crucial for accurate analyses. In addition, the static nature of DEMs means they can quickly become outdated as landscapes change. Low-resolution DEMs struggle to capture essential landform details that impact water flow, slope stability, or seismic wave propagation. High-resolution DEMs are often inaccessible in many parts of the world, particularly in developing countries, due to the prohibitive data acquisition and processing costs. Environmental conditions are inherently dynamic; thus, landscapes evolve due to natural processes and human activities, necessitating frequent DEM updates that are impossible due to logistical and financial constraints. Although DEMs are valuable tools, their effectiveness is enhanced when integrated with other geographic and environmental data [82]. This process can be complex and resource-intensive, requiring sophisticated GIS and computational modeling skills. In addition, managing and processing high-resolution DEM demands considerable computational power and technical expertise, which can be challenging, especially in resource-limited environments. However, as natural disasters become more frequent and intense, incorporating climate variables into DEM analyses is essential for accurate hazard assessments. Climate change affects temperature, precipitation, sea level rise, and extreme weather events, altering landscapes and natural hazards such as floods, landslides, and coastal erosion [83,84]. Integrating climate change projections with DEM data can assess future scenarios and regional impacts. Table 5 provides a comprehensive view of the advantages, challenges, opportunities for improvement, and potential risks associated with DEM applications in hazard mapping.
However, addressing these challenges involves several practical implications crucial for maximizing the accuracy and effectiveness of DEMs in environmental hazard mapping and ensuring they remain relevant over time. Enhanced data acquisition and sharing through advanced remote sensing and aerial survey technologies such as drones and satellite-based LiDAR are essential. These technologies provide more frequent updates and higher resolutions, and fostering global cooperation in data sharing can help overcome data availability limitations, especially in under-resourced regions. Developing adaptive models incorporating real-time data and feedback mechanisms can maintain the relevance of DEMs as landscapes change. Integrating DEMs with other environmental and social data through comprehensive GIS platforms can offer a more holistic view of hazard risks. Significant investment in technology and training is necessary to enhance the potential of DEMs. This includes building computational infrastructure and training local experts in advanced GIS and modeling techniques. In addition, continued research and development into new methodologies, including machine learning and artificial intelligence, can improve the accuracy and utility of DEMs, enhancing their predictive power in hazard management. Fig. 6 shows the interaction between the various factors influencing DEM application in environmental hazard mapping.
Figure 6: Critical analysis of DEM applications in environmental hazard mapping
Accordingly, DEMs have limitations that affect hazard mapping despite their significant utility. The quality and availability of high-resolution DEMs are often restricted by costs and logistical challenges, compromising accuracy in data-scarce regions. In addition, DEMs are static, necessitating frequent updates to capture recent changes due to natural events or human activities. Integrating DEMs with other geospatial data is complex and resource-intensive, requiring advanced computational resources and specialized technical expertise. To overcome these limitations, future work should focus on improving data acquisition through technologies such as drones and satellite-based LiDAR and developing adaptive models that integrate real-time data. Enhancing machine learning applications can also increase predictive accuracy.
5 Soft Computing Techniques for Multi-Hazard Mapping
Soft computing encompasses a variety of methodologies, including fuzzy logic, neural networks, genetic algorithms, and machine learning, which are particularly effective in enhancing the precision of hazard management [85,86]. These techniques integrate diverse data types such as experimental, remote sensing, topographic maps, and socio-economic datasets [24,87]. Such integration is essential for comprehensive hazard analysis, facilitating the management of the inherent uncertainty and variability of hazards due to changing climatic conditions and geographical transformations [88,89]. Fig. 7 highlights the benefits of integrating soft computing techniques into hazard analysis. These methods improve the accuracy and efficiency of solutions across various hazard mapping applications. Fig. 8 illustrates two problem-solving strategies in computer science and artificial intelligence: hard and soft computing techniques. Each approach has benefits and usages, frequently combined to achieve the best results. Hard computing relies on mathematical logic and accurate and deterministic techniques, which need well-defined issues and yield exact and reliable solutions. However, soft computing offers a more adaptable method that assumes the nuances of imprecision and uncertainty. This adaptability makes it well-suited for addressing complex issues in real-world scenarios.
Figure 7: Various soft computing methods
Figure 8: Hard and soft computing methods
Explainability in artificial intelligence (AI) models is crucial for their acceptance and effectiveness in multi-hazard mapping. Techniques such as shapley additive explanations (SHAP) and LIME (local interpretable model-agnostic explanations) provide insights into the variables influencing model predictions, enhancing trust and decision-making in hazard mitigation [85,90,91].
Previous studies have developed various methodologies for integrating machine learning in the process of multi-hazard mapping, such as the one given in Fig. 9 by Nachappa et al. [92]. One of the main benefits comes from the adaptability of neural networks and machine learning models that allows for continual learning from new data, improving hazard prediction models [93,94]. As a result, using soft computing in multi-hazard mapping improves the accuracy and reliability of hazard assessments and significantly contributes to developing informed and effective disaster risk reduction strategies. Previously, Kariminejad et al. [95–97] emphasized the role of geo-environmental modeling and deep learning methods in improving hazard mapping and management. Ullah et al. [98–100] further underscored the potential of machine learning techniques in multi-hazard susceptibility mapping, presenting substantial advancements in the field. Rahmati et al. [24] and Nachappa et al. [92] highlighted using machine learning in multi-hazard exposure mapping, providing a practical framework for assessing risks associated with various natural hazards. Despite these advantages, soft computing faces challenges such as computational complexity, the necessity for extensive training datasets, and difficulties in interpreting complex model outputs. However, future advancements in computational power and algorithm efficiency, along with improved data collection methods, are expected to enhance the applicability of soft computing in hazard management.
Figure 9: General procedure for multi-hazard mapping using DEM models integrated with machine learning techniques
This paper attempts to review and critically analyze existing literature on applying DEMs in multi-hazard assessments. It highlights the need for broader application of DEMs in multi-hazard assessments by integrating high-resolution DEMs with advanced methods such as machine learning techniques to enhance the precision and utility of hazard maps, which are essential for effective disaster risk reduction and strategic environmental planning. Based on the statements above, the following conclusions are drawn:
• Integrating DEMs across multiple hazard types, including floods, landslides, and coastal erosion, enables more comprehensive environmental risk assessments.
• Using high-resolution DEMs significantly improves the precision of hazard assessments, proving essential in accurately identifying and managing environmental risks.
• Machine learning techniques enhance hazard maps’ predictive accuracy by identifying complex patterns and interactions between various hazard types and environmental factors.
• Utilizing DEMs with GIS technology helps with environmental planning and hazard management, which allows for detailed and dynamic hazard mapping.
• The enhanced DEMs could facilitate better preparedness and response strategies in disaster management.
The review outlines several limitations in the current applications of DEMs, such as the challenges related to data resolution, update frequency, and the accessibility of high-resolution models, particularly in resource-limited areas. In order to address these limitations, it is recommended that DEMs be enhanced in resolution and accessibility to ensure they provide accurate and current data for hazard assessments. In addition, it recommends developing innovative methods to integrate real-time environmental data into DEMs to capture dynamic changes more effectively and improve the models’ predictive accuracy. Further exploration into expanding machine learning algorithms in conjunction with DEMs is also advised to bolster multi-hazard assessment capabilities. This critical review lays the foundation for future advancements in the field and underscores the importance of bridging these gaps to enhance DEM applications in environmental hazard mapping.
Acknowledgement: The authors would like to thank the editors and reviewers for their review and recommendations.
Funding Statement: The authors received no specific funding for this study.
Author Contributions: The authors confirm their contribution to the paper as follows: study conception and design: Maan Habib and Ahed Habib; data collection: Mohammad Abboud; analysis and interpretation of results: Maan Habib, Mohammad Abboud and Ahed Habib; draft manuscript preparation: Maan Habib. All authors reviewed the results and approved the final version of the manuscript.
Availability of Data and Materials: The authors confirm that the data supporting the findings of this study are available within the article and supplementary material.
Conflicts of Interest: The authors declare that they have no conflicts of interest to report regarding the present study.
References
1. Bishop MP, James LA, Shroder Jr JF, Walsh SJ. Geospatial technologies and digital geomorphological mapping: concepts, issues and research. Geomorphology. 2012;137(1):5–26. [Google Scholar]
2. Saleem N, Huq ME, Twumasi NYD, Javed A, Sajjad A. Parameters derived from and/or used with digital elevation models (DEMs) for landslide susceptibility mapping and landslide risk assessment: a review. ISPRS Int J Geo-Inf. 2019;8(12):545. [Google Scholar]
3. Kumar P, Debele SE, Sahani J, Rawat N, Marti-Cardona B, Alfieri SM, et al. An overview of monitoring methods for assessing the performance of nature-based solutions against natural hazards. Earth-Sci Rev. 2021;217:103603. [Google Scholar]
4. Taloor AK, Sharma S, Jamwal J, Kumar S. Quantitative and qualitative study of the Tawi basin: inferences from digital elevation model (DEM) using geospatial technology. Quat Sci Adv. 2024;14:100182. [Google Scholar]
5. McClean F, Dawson R, Kilsby C. Implications of using global digital elevation models for flood risk analysis in cities. Water Resour Res. 2020;56(10):1–15. [Google Scholar]
6. Agrawal R, Singh SK, Kanga S, Sajan B, Meraj G, Kumar P. Advancing flood risk assessment through integrated hazard mapping: a Google earth engine-based approach for comprehensive scientific analysis and decision support. J Clim. Change. 2024;10(1):47–60. [Google Scholar]
7. Yalcin A, Bulut F. Landslide susceptibility mapping using GIS and digital photogrammetric techniques: a case study from Ardesen (NE-Turkey). Nat Hazards. 2007;41(1):201–26. [Google Scholar]
8. Rabby YW, Ishtiaque A, Rahman MS. Evaluating the effects of digital elevation models in landslide susceptibility mapping in Rangamati District, Bangladesh. Remote Sens. 2020;12(17):2718. [Google Scholar]
9. Brock J, Schratz P, Petschko H, Muenchow J, Micu M, Brenning A. The performance of landslide susceptibility models critically depends on the quality of digital elevation models. Geomat. Nat Hazards Risk. 2020;11(1):1075–92. [Google Scholar]
10. Li R, Liu JK, Felus Y. Spatial modeling and analysis for shoreline change detection and coastal erosion monitoring. Mar Geod. 2001;24(1):1–12. [Google Scholar]
11. Eakins BW, Grothe PR. Challenges in building coastal digital elevation models. J Coast Res. 2014;30(5):942–53. doi:10.2112/JCOASTRES-D-13-00192.1. [Google Scholar] [CrossRef]
12. Stephens SA, Paulik R, Reeve G, Wadhwa S, Popovich B, Shand T, et al. Future changes in built environment risk to coastal flooding, permanent inundation and coastal erosion hazards. J Mar Sci Eng. 2021;9(9):1011. doi:10.3390/jmse9091011. [Google Scholar] [CrossRef]
13. Nguyen BQ, Vo ND, Le MH, Nguyen QD, Lakshmi V, Bolten JD. Quantification of global digital elevation model (DEM)–A case study of the newly released NASADEM for a river basin in Central Vietnam. J Hydrol Reg Stud. 2023;45(8):101282. doi:10.1016/j.ejrh.2022.101282. [Google Scholar] [CrossRef]
14. Sampson CC, Smith AM, Bates PD, Neal JC, Trigg MA. Perspectives on open access high resolution digital elevation models to produce global flood hazard layers. Front Earth Sci. 2016;3:85. doi:10.3389/feart.2015.00085. [Google Scholar] [CrossRef]
15. Kovanič Ľ., Blistan P, Urban R, Štroner M, Blišťanová M, Bartoš K, et al. Analysis of the suitability of high-resolution DEM obtained using ALS and UAS (SfM) for the identification of changes and monitoring the development of selected geo-hazards in the Alpine environment—a case study in High Tatras Slovakia. Remote Sens. 2020;12(23):3901. doi:10.3390/rs12233901. [Google Scholar] [CrossRef]
16. Tran TND, Nguyen QB, Vo ND, Marshall R, Gourbesville P. Assessment of terrain scenario impacts on hydrological simulation with SWAT model. application to lai giang catchment, Vietnam. In: Advances in hydroinformatics: models for complex and global water issues–practices and expectations. Singapore: Springer Nature Singapore; 2022. p. 1205–22. [Google Scholar]
17. Franci F, Bitelli G, Mandanici E, Hadjimitsis D, Agapiou A. Satellite remote sensing and GIS-based multi-criteria analysis for flood hazard mapping. Nat Hazards. 2016;83(S1):31–51. doi:10.1007/s11069-016-2504-9. [Google Scholar] [CrossRef]
18. Gigović L, Pamučar D, Bajić Z, Drobnjak S. Application of GIS-interval rough AHP methodology for flood hazard mapping in urban areas. Water. 2017;9(6):360. doi:10.3390/w9060360. [Google Scholar] [CrossRef]
19. Habib M, Alzubi Y, Malkawi A, Awwad M. Impact of interpolation techniques on the accuracy of large-scale digital elevation model. Open Geosci. 2020;12(1):190–202. doi:10.1515/geo-2020-0012. [Google Scholar] [CrossRef]
20. Habib M, Okayli M. An overview of modern cartographic trends aligned with the ICA’s perspective. Rev Int Géomat. 2023;32:1–16. doi:10.32604/rig.2023.043399. [Google Scholar] [CrossRef]
21. Manfreda S, Di Leo M, Sole A. Detection of flood-prone areas using digital elevation models. J Hydrol Eng. 2011;16(10):781–90. doi:10.1061/(ASCE)HE.1943-5584.0000367. [Google Scholar] [CrossRef]
22. Ibrahim M, Al-Mashaqbah A, Koch B, Datta P. An evaluation of available digital elevation models (DEMs) for geomorphological feature analysis. Environ Earth Sci. 2020;79(13):336. [Google Scholar]
23. Pourghasemi HR, Gayen A, Edalat M, Zarafshar M, Tiefenbacher JP. Is multi-hazard mapping effective in assessing natural hazards and integrated watershed management? Geosci Front. 2020;11(4):1203–17. [Google Scholar]
24. Rahmati O, Yousefi S, Kalantari Z, Uuemaa E, Teimurian T, Keesstra S, et al. Multi-hazard exposure mapping using machine learning techniques: a case study from Iran. Remote Sens. 2019;11(6):1943. [Google Scholar]
25. Achour Y, Pourghasemi HR. How do machine learning techniques help in increasing accuracy of landslide susceptibility maps? Geosci Front. 2020;11(3):871–83. [Google Scholar]
26. Javidan N, Kavian A, Pourghasemi HR, Conoscenti C, Jafarian Z, Rodrigo-Comino J. Evaluation of multi-hazard map produced using MaxEnt machine learning technique. Sci Rep. 2021;11(1):6496. [Google Scholar] [PubMed]
27. Avand M, Kuriqi A, Khazaei M, Ghorbanzadeh O. DEM resolution effects on machine learning performance for flood probability mapping. J Hydro-Environ Res. 2022;40:1–16. [Google Scholar]
28. MacMillan RA, Jones RK, McNabb DH. Defining a hierarchy of spatial entities for environmental analysis and modeling using digital elevation models (DEMs). Comput Environ Urban Syst. 2004;28(3):175–200. [Google Scholar]
29. Coveney S, Roberts K. Lightweight UAV digital elevation models and orthoimagery for environmental applications: data accuracy evaluation and potential for river flood risk modelling. Int J Remote Sens. 2017;38(8–10):3159–80. [Google Scholar]
30. Habib M. Quantifying topographic ruggedness using principal component analysis. Adv Civ Eng. 2021;2021:1–20. [Google Scholar]
31. Fathololoumi S, Vaezi AR, Alavipanah SK, Ghorbani A, Saurette D, Biswas A. Improved digital soil mapping with multitemporal remotely sensed satellite data fusion: a case study in Iran. Sci Total Environ. 2020;721:137703. [Google Scholar] [PubMed]
32. Okolie CJ, Smit JL. A systematic review and meta-analysis of digital elevation model (DEM) fusion: preprocessing, methods and applications. ISPRS J Photogramm Remote Sens. 2022;188:1–29. [Google Scholar]
33. Lee S, Choi U. Development of GIS-based geological hazard information system and its application for landslide analysis in Korea. Geosci J. 2003;7:243–52. [Google Scholar]
34. Alaghmand S, bin Abdullah R, Abustan I, Vosoogh B. GIS-based river flood hazard mapping in urban area (a case study in Kayu Ara River Basin, Malaysia). Int J Eng Technol. 2010;2(6):488–500. [Google Scholar]
35. Alarifi SS, Abdelkareem M, Abdalla F, Alotaibi M. Flash flood hazard mapping using remote sensing and GIS techniques in southwestern Saudi Arabia. Sustainability. 2022;14(21):14145. [Google Scholar]
36. Liu X. Airborne LiDAR for DEM generation: some critical issues. Prog Phys Geogr. 2008;32(1):31–49. [Google Scholar]
37. Muhadi NA, Abdullah AF, Bejo SK, Mahadi MR, Mijic A. The use of LiDAR-derived DEM in flood applications: a review. Remote Sens. 2020;12(14):2308. [Google Scholar]
38. Gomez C, Purdie H. UAV-based photogrammetry and geocomputing for hazards and disaster risk monitoring-a review. Geoenviron Disasters. 2016;3:1–11. [Google Scholar]
39. Annis A, Nardi F, Petroselli A, Apollonio C, Arcangeletti E, Tauro F, et al. UAV-DEMs for small-scale flood hazard mapping. Water. 2020;12(6):1717. [Google Scholar]
40. Kappes MS, Keiler M, von Elverfeldt K, Glade T. Challenges of analyzing multi-hazard risk: a review. Nat Hazards. 2012;64:1925–58. [Google Scholar]
41. Han L, Zhang J, Zhang Y, Ma Q, Alu S, Lang Q. Hazard assessment of earthquake disaster chains based on a Bayesian network model and ArcGIS. ISPRS Int J Geo-Inf. 2019;8(5):210. [Google Scholar]
42. Linardos V, Drakaki M, Tzionas P, Karnavas YL. Machine learning in disaster management: recent developments in methods and applications. Mach Learn Knowl Extr. 2022;4(2):446–473. [Google Scholar]
43. Su Y, Rong G, Ma Y, Chi J, Liu X, Zhang J, et al. Hazard assessment of earthquake disaster chains based on deep learning–a case study of Mao county, sichuan province. Front Earth Sci. 2022;9:683903. [Google Scholar]
44. Gournellos T, Evelpidou N, Vassilopoulos A. Developing an erosion risk map using soft computing methods (case study at Sifnos island). Nat Hazards. 2004;31:63–83. [Google Scholar]
45. Mallick J, Alashker Y, Mohammad SA, Ahmed M, Hasan MA. Risk assessment of soil erosion in semi-arid mountainous watershed in Saudi Arabia by RUSLE model coupled with remote sensing and GIS. Geocarto Int. 2014;29(8):915–40. [Google Scholar]
46. Chowdhuri I, Pal SC, Saha A, Chakrabortty R, Roy P. Evaluation of different DEMs for gully erosion susceptibility mapping using in-situ field measurement and validation. Ecol Inform. 2021;65:101425. [Google Scholar]
47. Negash DA, Moisa MB, Merga BB, Sedeta F, Gemeda DO. Soil erosion risk assessment for prioritization of sub-watershed: the case of Chogo Watershed, Horo Guduru Wollega, Ethiopia. Environ Earth Sci. 2021;80(17):589. [Google Scholar]
48. Wechsler SP. Uncertainties associated with digital elevation models for hydrologic applications: a review. Hydrol Earth Syst Sci. 2007;11(4):1481–500. [Google Scholar]
49. Bhuyian MN, Kalyanapu A. Accounting digital elevation uncertainty for flood consequence assessment. J Flood Risk Manage. 2018;11:S1051–S1062. [Google Scholar]
50. Salekin S, Lad P, Morgenroth J, Dickinson Y, Meason DF. Uncertainty in primary and secondary topographic attributes caused by digital elevation model spatial resolution. Catena. 2023;231:107320. [Google Scholar]
51. Farr TG, Rosen PA, Caro E, Crippen R, Duren R, Hensley S, et al. The shuttle radar topography mission. Rev Geophys. 2007;45(2). [Google Scholar]
52. Tachikawa T, Kaku M, Iwasaki A, Gesch DB, Oimoen MJ, Zhang Z, et al. ASTER global digital elevation model version 2-summary of validation results. NASA; 2011. p. 1–33. [Google Scholar]
53. Hodgson ME, Bresnahan P. Accuracy of airborne lidar-derived elevation. Photogramm Eng Remote Sens. 2004;70(3):331–9. [Google Scholar]
54. Rosenqvist A, Shimada M, Ito N, Watanabe M. ALOS PALSAR: a pathfinder mission for global-scale monitoring of the environment. IEEE Trans Geosci Remote Sens. 2007;45(11):3307–16. [Google Scholar]
55. Baltsavias EP. Airborne laser scanning: basic relations and formulas. ISPRS J Photogramm Remote Sens. 1999;54(2–3):199–214. [Google Scholar]
56. Nex F, Remondino F. UAV for 3D mapping applications: a review. Appl Geomatics. 2014;6:1–15. [Google Scholar]
57. Zerger A. Digital elevation modelling for natural hazard risk assessment. Aust J Emerg Manage. 1999;13(4):61–5. [Google Scholar]
58. Stamatelatos M, Dezfuli H, Apostolakis G, Everline C, Guarro S, Mathias D, et al. Probabilistic risk assessment procedures guide for NASA managers and practitioners. NASA; 2011. HQ-STI-11-213. [Google Scholar]
59. Kwag S, Gupta A. Probabilistic risk assessment framework for structural systems under multiple hazards using Bayesian statistics. Nucl Eng Des. 2017;315:20–34. [Google Scholar]
60. Van de Sande B, Lansen J, Hoyng C. Sensitivity of coastal flood risk assessments to digital elevation models. Water. 2012;4(3):568–79. [Google Scholar]
61. Xu K, Fang J, Fang Y, Sun Q, Wu C, Liu M. The importance of digital elevation model selection in flood simulation and a proposed method to reduce DEM errors: a case study in Shanghai. Int J Disaster Risk Sci. 2021;12:890–902. [Google Scholar]
62. Manfreda S, Samela C. A digital elevation model based method for a rapid estimation of flood inundation depth. J Flood Risk Manage. 2019;12:1–27. [Google Scholar]
63. Al-Areeq AM, Sharif HO, Abba SI, Chowdhury S, Al-Suwaiyan M, Benaafi M, et al. Digital elevation model for flood hazards analysis in complex terrain: case study from Jeddah, Saudi Arabia. Int J Appl Earth Obs Geoinf. 2023;119:103330. [Google Scholar]
64. Sulaiman NA, Husain F, Hashim KA, Ma’arof I, Samad AM. A study on the extraction of digital elevation model (DEM) using remote sensing and geographic information system (GIS) for flood risk assessment. In: 2012 IEEE International Conference on Control System, Computing and Engineering, 2012; Malaysia; p. 568–73. [Google Scholar]
65. Niyongabire E, Hassan R, Elhassan E, Mehdi M. Use of digital elevation model in a GIS for flood susceptibility mapping: case of Bujumbura City. In: Proceedings of the 6th International Conference on Cartography and GIS, 2016; Albena: Bulgaria; p. 241–8. [Google Scholar]
66. Meena SR, Gudiyangada Nachappa T. Impact of spatial resolution of digital elevation model on landslide susceptibility mapping: a case study in Kullu Valley Himalayas. Geosci. 2019;9(8):360. [Google Scholar]
67. Jibson RW, Harp EL, Michael JA. A method for producing digital probabilistic seismic landslide hazard maps: an example from the Los Angeles, California area. US Geol Surv. 1998;1:98–113. [Google Scholar]
68. Jibson RW, Harp EL, Michael JA. A method for producing digital probabilistic seismic landslide hazard maps. Eng Geol. 2000;58(3–4):271–89. [Google Scholar]
69. Li C, Wang G, He J, Wang Y. A novel approach to probabilistic seismic landslide hazard mapping using Monte Carlo simulations. Eng Geol. 2022;301:106616. [Google Scholar]
70. Haneberg WC. Effects of digital elevation model errors on spatially distributed seismic slope stability calculations: an example from Seattle. Washington Environ Eng Geosci. 2006;12(3):247–60. [Google Scholar]
71. Ahmad RA, Singh RP, Adris A. Seismic hazard assessment of Syria using seismicity, DEM, slope, active faults and GIS. Remote Sens Appl: Soc Environ. 2017;6:59–70. [Google Scholar]
72. Theilen-Willige B, Aher SP, Gawali PB, Venkata LB. Seismic hazard analysis along Koyna Dam area, western Maharashtra, India: a contribution of remote sensing and GIS. Geosci. 2016;6(2):20. [Google Scholar]
73. Marfai MA, Khakim N, Fatchurohman H, Salma AD. Planning tsunami vertical evacuation routes using high-resolution UAV digital elevation model: case study in Drini coastal area, Java, Indonesia. Arab J Geosci. 2021;14:1–13. [Google Scholar]
74. Demirkesen AC, Evrendilek F, Berberoglu SÜ, Kilic S. Coastal flood risk analysis using Landsat-7 ETM+ imagery and SRTM DEM: a case study of Izmir. Turkey Environ Monit Assess. 2007;131:293–300. [Google Scholar] [PubMed]
75. Xafoulis N, Kontos Y, Farsirotou E, Kotsopoulos S, Perifanos K, Alamanis N, et al. Evaluation of various resolution DEMs in flood risk assessment and practical rules for flood mapping in data-scarce geospatial areas: a case study in Thessaly. Greece Hydrology. 2023;10(4):91. [Google Scholar]
76. Iqbal A, Mondal MS, Veerbeek W, Khan MSA, Hakvoort H. Effectiveness of UAV-based DTM and satellite-based DEMs for local-level flood modeling in Jamuna floodplain. J Flood Risk Manage. 2023;16(4):1–19. [Google Scholar]
77. Okoli J, Nahazanan H, Nahas F, Kalantar B, Shafri HZM, Khuzaimah Z. High-resolution lidar-derived DEM for landslide susceptibility assessment using AHP and fuzzy logic in Serdang, Malaysia. Geosciences. 2023;13(2):34. [Google Scholar]
78. Reshma R, Devi NN, Kuiry SN. Real-time urban flood modeling: exploring the sub-grid approach for accurate simulation and hazard analysis. Nat Hazards. 2024;2024:1–39. [Google Scholar]
79. Malakar S, Rai AK, Gupta AK. Earthquake risk mapping in the Himalayas by integrated analytical hierarchy process, entropy with neural network. Nat Hazards. 2023;116(1):951–75. [Google Scholar]
80. Vitousek S, Buscombe D, Vos K, Barnard PL, Ritchie AC, Warrick JA. The future of coastal monitoring through satellite remote sensing. Cambridge Prisms: Coast Futures; 2023. [Google Scholar]
81. Savastano S, Gomes da Silva P, Sánchez JM, Tort AG, Payo A, Pattle ME, et al. Assessment of shoreline change from SAR satellite imagery in three tidally controlled coastal environments. J Mar Sci Eng. 2024;12(1):163. [Google Scholar]
82. Bohat N, Joshi V. Evaluation of morphometric characteristics of Mandakini River watershed, Uttarakhand, India using cartosat-DEM in GIS environment. Proc Indian Natl Sci Acad. 2024;90(1):91–101. [Google Scholar]
83. Chathuranika IM, Gunathilake MB, Baddewela PK, Sachinthanie E, Babel MS, Shrestha S, et al. Comparison of two hydrological models, HEC-HMS and SWAT in runoff estimation: application to Huai Bang Sai tropical watershed. Thailand Fluids. 2022;7(8):267. [Google Scholar]
84. Ukumo TY, Abebe A, Lohani TK, Edamo ML. Flood hazard mapping and analysis under climate change using hydro-dynamic model and RCPs emission scenario in Woybo River catchment of Ethiopia. World J Eng. 2023;20(3):559–76. [Google Scholar]
85. Antzoulatos G, Kouloglou IO, Bakratsas M, Moumtzidou A, Gialampoukidis I, Karakostas A, et al. Flood hazard and risk mapping by applying an explainable machine learning framework using satellite imagery and GIS data. Sustainability. 2022;14(6):3251. [Google Scholar]
86. Habib A, Youssefi I, Kunt MM. Identification of pulse-like ground motions using artificial neural network. Earthquake Eng Eng Vib. 2022;21(4):899–912. [Google Scholar]
87. Habib A, Yildirim U. Estimating mechanical and dynamic properties of rubberized concrete using machine learning techniques: a comprehensive study. Eng Comput. 2022;39(8):3129–78. [Google Scholar]
88. Eini M, Kaboli HS, Rashidian M, Hedayat H. Hazard and vulnerability in urban flood risk mapping: machine learning techniques and considering the role of urban districts. Int J Disaster Risk Reduct. 2020;50:101687. [Google Scholar]
89. Hamdy O, Gaber H, Abdalzaher MS, Elhadidy M. Identifying exposure of urban area to certain seismic hazard using machine learning and GIS: a case study of greater Cairo. Sustainability. 2022;14(17):10722. [Google Scholar]
90. Habib A, Yildirim U, Habib M. Applying kernel principal component analysis for enhanced multivariable regression modeling of rubberized concrete properties. Arab J Sci Eng. 2023;48(4):5383–96. doi:10.1007/s13369-022-07435-8. [Google Scholar] [CrossRef]
91. Madhushani C, Dananjaya K, Ekanayake IU, Meddage DPP, Kantamaneni K, Rathnayake U. Modeling streamflow in non-gauged watersheds with sparse data considering physiographic, dynamic climate, and anthropogenic factors using explainable soft computing techniques. J Hydrol. 2024;631(17):130846. doi:10.1016/j.jhydrol.2024.130846. [Google Scholar] [CrossRef]
92. Nachappa TG, Ghorbanzadeh O, Gholamnia K, Blaschke T. Multi-hazard exposure mapping using machine learning for the State of Salzburg. Austria Remote Sens. 2020;12(17):2757. doi:10.3390/rs12172757. [Google Scholar] [CrossRef]
93. Habib A, Alnaemi A, Habib M. Developing a framework for integrating blockchain technology into earthquake risk mitigation and disaster management strategies of smart cities. Smart Sustain Built Environ. 2024. doi:10.1108/SASBE-12-2023-0376. [Google Scholar] [CrossRef]
94. Wang L, Zhang X, Li Q, Zhang M, Su H, Zhu J, et al. Incorporating neuro-inspired adaptability for continual learning in artificial intelligence. Nat Mach Intell. 2023;5(12):1356–68. doi:10.1038/s42256-023-00747-w. [Google Scholar] [CrossRef]
95. Kariminejad N, Pourghasemi HR, Hosseinalizadeh M. Analytical techniques for mapping multi-hazard with geo-environmental modeling approaches and UAV images. Sci Rep. 2022;12(1):14946. doi:10.1038/s41598-022-18757-w. [Google Scholar] [PubMed] [CrossRef]
96. Mahendra RS, Mohanty PC, Bisoyi H, Kumar TS, Nayak S. Assessment and management of coastal multi-hazard vulnerability along the Cuddalore-Villupuram, east coast of India using geospatial techniques. Ocean Coast Manage. 2011;54(4):302–11. [Google Scholar]
97. Ruiz-Lendínez JJ, Ariza-López FJ, Reinoso-Gordo JF, Ureña-Cámara MA, Quesada-Real FJ. Deep learning methods applied to digital elevation models: state of the art. Geocarto Int. 2023;38(1):2252389. [Google Scholar]
98. Ullah K, Wang Y, Fang Z, Wang L, Rahman M. Multi-hazard susceptibility mapping based on convolutional neural networks. Geosci Front. 2022;13(5):101425. [Google Scholar]
99. Cao J, Zhang Z, Du J, Zhang L, Song Y, Sun G. Multi-geohazards susceptibility mapping based on machine learning—a case study in Jiuzhaigou China. Nat Hazards. 2020;102:851–71. [Google Scholar]
100. Pourghasemi HR, Kariminejad N, Amiri M, Edalat M, Zarafshar M, Blaschke T, et al. Assessing and mapping multi-hazard risk susceptibility using a machine learning technique. Sci Rep. 2020;10(1):3203. [Google Scholar] [PubMed]
Cite This Article
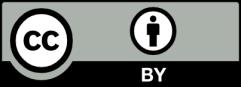
This work is licensed under a Creative Commons Attribution 4.0 International License , which permits unrestricted use, distribution, and reproduction in any medium, provided the original work is properly cited.