Open Access
ARTICLE
Support Vector Machine (SVM) and Object Based Classification in Earth Linear Features Extraction: A Comparison
1 School of Geomatics Science and Natural Resources, College of Built Environment, Universiti Teknologi MARA, Shah Alam, 40450, Malaysia
2 Institute for Biodiversity and Sustainable Development, Universiti Teknologi MARA, Shah Alam, 40450, Malaysia
3 School of Geomatics Science and Natural Resources, College of Built Environment, Universiti Teknologi MARA, Perlis, 02600, Malaysia
4 Department of Geomatic Engineering, Artvin Coruh University, Artvin, 08000, Türkiye
5 College of Agriculture and Natural Resources, Central Bicol State University of Agriculture, Pili, Camarines Sur, 4418, Philippines
6 GRAB Driver Center Kota Kinabalu (GDC KK), Riverson, Kota Kinabalu, 88100, Sabah
* Corresponding Author: Siti Aekbal Salleh. Email:
Revue Internationale de Géomatique 2024, 33, 183-199. https://doi.org/10.32604/rig.2024.050723
Received 15 February 2024; Accepted 14 May 2024; Issue published 27 June 2024
Abstract
Due to the spectral and spatial properties of pervious and impervious surfaces, image classification and information extraction in detailed, small-scale mapping of urban surface materials is quite difficult and complex. Emerging methods and innovations in image classification have centred on object-based classification techniques and various segmentation techniques, which are fundamental to this approach. Consequently, the purpose of this study is to determine which classification method is most suitable for extracting linear features in terms of techniques and performance by comparing two classification methods, pixel-based approach and object-based approach, using WorldView-2 satellite imagery to specifically highlight linear features such as roads, building edges, and road dividers. Two applied algorithms, including support vector machines (SVM) and ruled-based, were evaluated using two distinct software. A comparison of the results reveals that the object-based classification has a higher overall resolution than the pixel-based classification. The output of rule-based classification was satisfactory, with an overall accuracy of 88.6% (ENVI) and 92.2% (e-Cognition). The SVM classification result contained misclassified impervious surfaces and other urban features, as well as mixed objects. This classification achieved an overall accuracy of 75.1%. Nonetheless, this study provides an excellent overview for understanding the differences in their performances on the same data, as well as a comparison of the software employed.Graphic Abstract
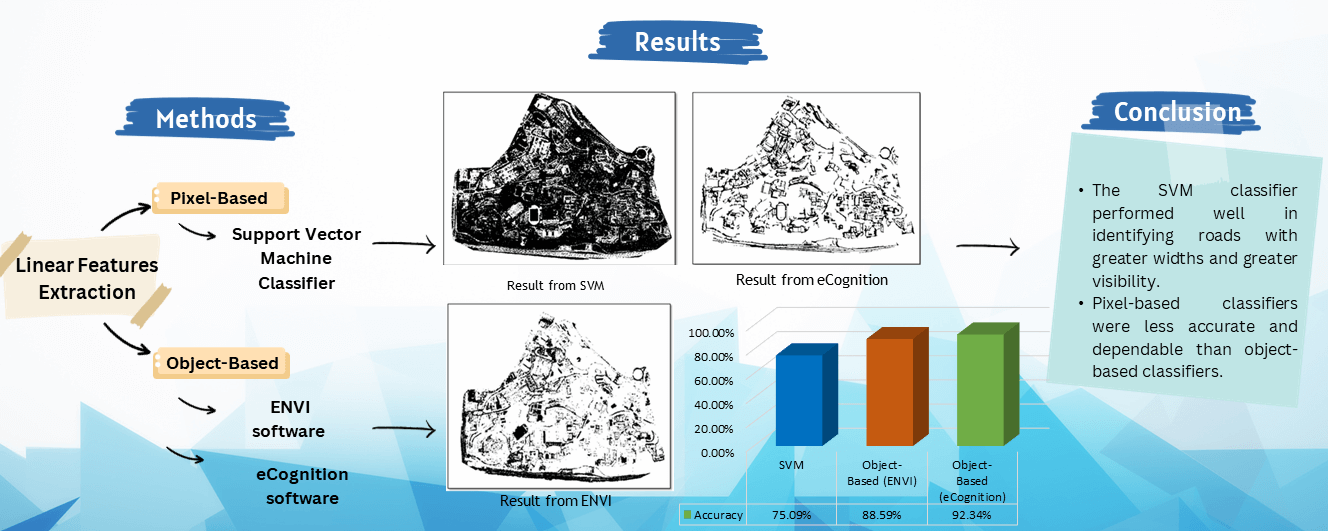
Keywords
Cite This Article
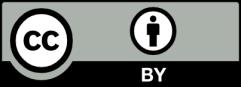
This work is licensed under a Creative Commons Attribution 4.0 International License , which permits unrestricted use, distribution, and reproduction in any medium, provided the original work is properly cited.