Open Access
ARTICLE
Harnessing ML and GIS for Seismic Vulnerability Assessment and Risk Prioritization
1 Faculty of Technology, Center for Environmental Planning and Technology (CEPT) University, Kasturbhai Lalbhai Campus, Navrangpura, Ahmedabad, 380009, India
2 Tata Institute of Social Science, VN Purav Marg, Deonar, Mumbai, 400088, India
* Corresponding Authors: Dhwanilnath Gharekhan. Email: ,
(This article belongs to the Special Issue: Applications of Artificial Intelligence in Geomatics for Environmental Monitoring)
Revue Internationale de Géomatique 2024, 33, 111-134. https://doi.org/10.32604/rig.2024.051788
Received 15 March 2024; Accepted 14 April 2024; Issue published 15 May 2024
Abstract
Seismic vulnerability modeling plays a crucial role in seismic risk assessment, aiding decision-makers in pinpointing areas and structures most prone to earthquake damage. While machine learning (ML) algorithms and Geographic Information Systems (GIS) have emerged as promising tools for seismic vulnerability modeling, there remains a notable gap in comprehensive geospatial studies focused on India. Previous studies in seismic vulnerability modeling have primarily focused on specific regions or countries, often overlooking the unique challenges and characteristics of India. In this study, we introduce a novel approach to seismic vulnerability modeling, leveraging ML and GIS to address these gaps. Employing Artificial Neural Networks (ANN) and Random Forest algorithms, we predict damage intensity values for earthquake events based on various factors such as location, depth, land cover, proximity to major roads, rivers, soil type, population density, and distance from fault lines. A case study in the Satara district of Maharashtra underscores the effectiveness of our model in identifying vulnerable buildings and enhancing seismic risk assessment at a local level. This innovative approach not only fills the gap in existing research by providing predictive modeling for seismic damage intensity but also offers a valuable tool for disaster management and urban planning decision-makers.Keywords
Cite This Article
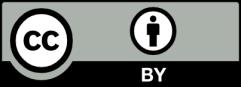
This work is licensed under a Creative Commons Attribution 4.0 International License , which permits unrestricted use, distribution, and reproduction in any medium, provided the original work is properly cited.