Open Access
ARTICLE
Génération de cartes tactiles photoréalistes pour personnes déficientes visuelles par apprentissage profond
1. LIMOS UMR 6158, Université Clermont Auvergne, 1 rue Chebarde,
TSA 60125 CS 60026 63178 Aubière cedex, France
2. LASTIG, Univ Gustave Eiffel, ENSG, IGN, 73 av. de Paris, 94165 Saint-Mandé
cedex, France
Revue Internationale de Géomatique 2020, 30(1), 105-126. https://doi.org/10.3166/rig.2020.00104
RÉSUMÉ
Les cartes tactiles photoréalistes sont un des outils mobilisés par les personnes en situation de déficience visuelle pour appréhender leur environnement urbain proche, notamment dans le cadre de la mobilité, pour la traversée de carrefours par exemple. Ces cartes sont aujourd’hui principalement fabriquées artisanalement. Dans cet article, nous proposons une approche permettant de produire une segmentation sémantique d’une imagerie aérienne de précision, étape centrale de cette fabrication. Les différents éléments d’intérêt tels que trottoirs, passages piétons, ou îlots centraux sont ainsi localisés et tracés dans l’espace urbain. Nous présentons en particulier comment l’augmentation de cette imagerie par des données vectorielles issues d’OpenStreetMap permet d’obtenir par une technique d’apprentissage profond (réseau adverse génératif conditionnel) des résultats significatifs. Après avoir présenté les enjeux de ce travail et un état de l’art des techniques existantes, nous détaillons l’approche proposée, et nous étudions les résultats obtenus, en comparant en particulier les segmentations obtenues sans et avec enrichissement par données vectorielles. Les résultats sont très prometteurs.Abstract
Photo-realistic tactile maps are one of the tools used by visually impaired people to understand their immediate urban environment, particularly in the context of mobility, for crossing crossroads for example. These maps are nowadays mainly hand-made. In this article, we propose an approach to produce a semantic segmentation of precision aerial imagery, a central step in this manufacturing process. The different elements of interest such as sidewalks, pedestrian crossings, or central islands are thus located and traced in the urban space. We present in particular how the augmentation of this imagery by vector data from OpenStreetMap leads to significant results using a deep learning technique (conditional generative adversarial network). After presenting the stakes of this work and a state of the art of existing techniques, we detail the proposed approach, and we study the results obtained, in particular by comparing the segmentations obtained without and with enrichment by vector data. The results are very promising.MOTS CLÉS
Keywords
Cite This Article
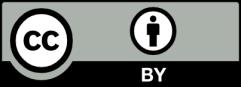
This work is licensed under a Creative Commons Attribution 4.0 International License , which permits unrestricted use, distribution, and reproduction in any medium, provided the original work is properly cited.