Open Access
ARTICLE
Employing a Backpropagation Neural Network for Predicting Fear of Cancer Recurrence among Non-Small Cell Lung Cancer Patients
1 National Cancer Center/National Clinical Research Center for Cancer (NCRCC)/Thoracic Surgery, Cancer Hospital, Chinese Academy of Medical Sciences & Peking Union Medical College, Beijing, 100021, China
2 Arrhythmia Diagnostic and Treatment Center, Qingdao Hiser Hospital Affiliated of Qingdao University (Qingdao Traditional Chinese Medicine Hospital), Qingdao, 266000, China
* Corresponding Authors: Hongjing Wang. Email: ; Lu Liu. Email:
# Co-first authors
(This article belongs to the Special Issue: Multidisciplinary Clinical Health Psychology for Cancer Experience
Psychologie clinique multidisciplinaire de la santé pour l'expérience du cancer)
Psycho-Oncologie 2024, 18(4), 305-316. https://doi.org/10.32604/po.2024.054098
Received 18 May 2024; Accepted 24 September 2024; Issue published 04 December 2024
Abstract
Objective: Non-small cell lung cancer (NSCLC) patients often experience significant fear of recurrence. To facilitate precise identification and appropriate management of this fear, this study aimed to compare the efficacy and accuracy of a Backpropagation Neural Network (BPNN) against logistic regression in modeling fear of cancer recurrence prediction. Methods: Data from 596 NSCLC patients, collected between September 2023 and December 2023 at the Cancer Hospital of the Chinese Academy of Medical Sciences, were analyzed. Nine clinically and statistically significant variables, identified via univariate logistic regression, were inputted into both BPNN and logistic regression models developed on a training set (N = 427) and validated on an independent set (N = 169). Model performances were assessed using Area Under the Receiver Operating Characteristic (ROC) Curve and Decision Curve Analysis (DCA) in both sets. Results: The BPNN model, incorporating nine selected variables, demonstrated superior performance over logistic regression in the training set (AUC = 0.842 vs. 0.711, p < 0.001) and validation set (0.7 vs. 0.675, p < 0.001). Conclusion: The BPNN model outperforms logistic regression in accurately predicting fear of cancer recurrence in NSCLC patients, offering an advanced approach for fear assessment.Keywords
The Global Cancer (GLOBOCAN) 2020 report by the WHO’s International Agency for Research on Cancer (IARC) highlights the increasing prevalence of cancer worldwide, with lung cancer being a major contributor to global cancer incidence and mortality. In 2020, lung cancer accounted for approximately 2.2 million new cases and 1.8 million deaths [1]. Non-small cell lung cancer (NSCLC) represents about 80% of primary malignant lung cancers [2]. Despite advancements in surgical techniques improving the 5-year survival rates for lung cancer patients [3], a significant proportion still faces the risk of postoperative recurrence and metastasis, resulting in considerable psychological distress [4,5].
This psychological burden, predominantly manifesting as Fear of Cancer Recurrence (FCR), is a prevalent concern among NSCLC patients, especially those in early stages post-surgery [6]. FCR affects not only the patients’ quality of life and survival duration but also has broader implications on healthcare resource utilization and compliance with medical treatments [7,8]. The National Comprehensive Cancer Network (NCCN) and other health organizations recognize the importance of addressing psychological issues in cancer care.
Hope theory suggests that fostering positive psychology in individuals can help them unlock their potential, build confidence and hope for the future, promote personal growth in a positive direction, and mitigate the negative effects of adverse events [9], Our previous study has shown that the FCR in lung cancer patients is negatively correlated with the level of hope, and FCR may trigger negative emotions such as tension and anger, which may affect the quality of life of patients and even develop into psychosomatic disorders, and this emotional state may reduce the level of hope of patients and make them pessimistic about the outcome of treatment [10].
Social support refers to a network of close relatives or friends who can assist patients during their illness [11]. Social support not only provides emotional support and financial assistance but also encourages patients to adopt a positive attitude towards treatment. Through social support, patients can effectively reduce negative stress and FCR caused by various adverse situations, enhance psychological resilience, and promote overall physical and mental health [12]. At the same time, studies have shown that the FCR scores of lung cancer chemotherapy patients are significantly negatively correlated with the social support scores, and social support, as a material and spiritual pillar, is conducive to patients’ positive and optimistic treatment of their illnesses, and this kind of support may help patients to better cope with difficulties in the course of treatment and to reduce the fear of recurrence of the disease [13], Research has also shown that there is a significant nonlinear dose-response relationship between social support, levels of hope, and FCR, indicating that these factors can influence each other [14]. Therefore, this study included the Hope Scale and the Social Support Scale to further explore their predictive effects on the FCR model.
Previous literature provides ample insight into factors predicting FCR in lung cancer patients. However, traditional predictive models like logistic regression often fail to capture the intricate relationships influencing FCR. To address this gap, our study employs the Backpropagation Neural Network (BPNN), an advanced method derived from artificial neural networks (ANN). BPNN’s self-organizing, self-learning, and adaptive features enable the effective identification of complex non-linear relationships between variables [15]. This approach offers a comprehensive understanding of FCR predictors and surpasses traditional methodologies in predicting the psychological state of NSCLC patients [16,17].
Our research aims to establish a BPNN-based predictive model for FCR in NSCLC patients. This innovative approach promises to enhance the precision of individual predictions, allowing healthcare professionals to develop targeted prevention and intervention strategies for patients at risk of FCR. By integrating this novel method into the realm of cancer care, our study seeks to contribute significantly to the field, promoting the application of artificial intelligence in medical practice.
To ensure the accuracy and generalization ability of the Back propagation Neural Network (BPNN) model, we followed Hecht-Nielsen’s (1987) guideline, which suggests that the sample size should be at least ten times the number of network weights. For our study, we expect to include 8 input variables, 5 neurons in the hidden layer, and 1 output neuron. The total number of weights W = (n × h) + (h × m) + h + m is calculated to be 51, thus, the minimum required sample size is N ≥ 510, To account for a potential 10% questionnaire loss rate, we adjust the sample size to be at least 567.
This study adopted a cross-sectional survey design, consecutively enrolling 616 patients who underwent surgical treatment for non-small cell lung cancer (NSCLC) at the Cancer Hospital of the Chinese Academy of Medical Sciences from September 2023 and December 2023. Inclusion criteria were: (1) age ≥18 years; (2) intraoperative frozen pathology confirming NSCLC; (3) informed consent and willingness to participate in the survey; (4) patients being aware of their diagnosis; (5) clear consciousness without communication barriers. Exclusion criteria were: (1) presence of other severe physical or psychological diseases; (2) cognitive impairment or mental illness; (3) language communication or comprehension barriers. This study was approved by the Ethics Committee of the Cancer Hospital of the Chinese Academy of Medical Sciences (approval number: 23/179–3921).
Designed by the researchers, it includes demographic and clinical data such as age, gender, educational level, long-term residence, average monthly family income, method of medical expenses payment, presence of children, whether children are adults, chemotherapy status, etc. The categorization of the elderly population is based on the National Health Commission reports to align with the demographics of the Chinese population.
Fear of progression questionnaire-short form (FoP-Q-SF)
Developed by Mehnert et al. [18] and translated into Chinese by Wu et al. [19]. This unidimensional scale consists of 12 items, with a score range of 12–60. A score >34 indicates psychological dysfunction. The Chinese version correlates well with the original (correlation coefficients range from 0.578 to 0.712), and has a good reliability with a Cronbach’s α coefficient of 0.883.
Developed by Herth [20] and adapted for Chinese patients by Zhao [21], HHI is widely used to measure hope levels in cancer patients. It comprises 12 items distributed across three subscales. The total score ranges from 12 to 48, with higher scores indicating higher levels of hope.
Social support rating scale (SSRS)
Developed by Xiao et al. [22], this scale has 10 items across three dimensions: objective support, subjective support, and social support utility. It is scored on a 4-point Likert scale, with higher scores indicating better social support. The scale has shown good reliability and validity.
Hope levels and social support, measured by the Herth Hope Index (HHI) and Social Support Rating Scale (SSRS), were included in this study not only because they are significant factors influencing FCR according to previous research, but also because they involve complex interactions and nonlinear relationships with FCR [14]. Neural network models like the Backpropagation Neural Network (BPNN) are particularly adept at handling these complexities. By integrating these variables into the BPNN model and comparing its performance with the traditional logistic regression model, we aimed to develop a more accurate and robust model for predicting FCR, ultimately improving patient care and management.
An electronic questionnaire survey was conducted using the “Wenjuanxing” platform. Researchers distributed the electronic questionnaires to post-surgery lung cancer patients who met the inclusion criteria. Data collectors received online training, which covered the study’s purpose and significance, the questionnaire and scale content, data collection methods, and precautions. Before the survey, each data collector explained the study’s purpose and significance to respondents in a uniform teaching language. The questionnaire’s introduction page provided standardized instructions, informing participants about the study’s anonymity, voluntary nature, lack of right or wrong answers, and privacy protection strategies. Participation was entirely voluntary and decided by the respondents.
The questionnaire settings allowed only one submission per IP address, with questions presented in a paginated format and marked as mandatory. To prevent interference among patients in the same ward, the questionnaires were completed separately. Survey distributors provided explanations for any questions that patients found difficult to understand. For patients with poor vision, caregivers completed the questionnaire based on the patients’ responses. Data were double-checked and exported through Excel. Out of 616 distributed questionnaires, 11 were excluded due to a completion time of less than 180 s, 3 for identical answers to all questions, and 6 from patients who had received preoperative neoadjuvant therapy. This resulted in 596 valid questionnaires, with an effective response rate of 96.8%. After collection, clinical data were verified and supplemented by cross-referencing with medical records from the hospital’s HIS information system to ensure accuracy.
Statistical analysis was conducted using SPSS 25.0 software. Demographic data were presented in frequencies and percentages. Univariate logistic regression analysis was employed for group comparisons, with a significance level set at p < 0.05 (two-sided). The BPNN model was built using a multilayer perceptron and ranked variables for importance. The number of nodes in the hidden layer of the BPNN model was determined through a trial method, selecting the number that minimized the difference between output and actual values. The model was used to classify and predict cancer recurrence fear based on general data, disease-related factors, and socio-psychological factors. The performance of logistic regression and neural network models was assessed using the area under the ROC curve and Decision Curve Analysis (DCA). Comparison of the AUC for both models was conducted using the paired AUC comparison method, i.e., the DeLong test.
General information of study participants
Among the 596 postoperative NSCLC patients, 40% were male with an average fear score of 28.16 ± 10, and 60% were female with a fear score of 30.57 ± 8.57, indicating a significantly higher fear of recurrence in females. Patients aged 18–35 years (8% of the cohort) had a fear score of 33.13 ± 8.33, those aged 36–60 years (59%) had a score of 30.68 ± 9.07, and those aged over 60 years (33%) scored 26.88 ± 9.13. ANOVA results showed younger patients had significantly higher fear levels. Other clinical and psychosocial factors, such as lower educational levels, having underage children, undergoing chemotherapy, and lower levels of hope, were significantly associated with higher fear levels. Detailed data are presented in Table 1.
Logistic regression analysis of fear of recurrence in NSCLC patients
Thirty clinical, pathological, and psychosocial indicators, including age, gender, disease duration, surgical technique, and level of hope, were analyzed using univariate logistic regression. Gender, age, monthly family income, whether children were adults, level of hope, and social support intensity showed statistically significant impacts on fear levels. These significant factors, along with clinically meaningful indicators from the ANOVA, were incorporated into a multivariate logistic regression model. Etailed data are presented in Table 2.
Construction of risk prediction model for postoperative fear of recurrence in NSCLC patients
Eight indicators identified as significant in ANOVA, logistic regression analysis, and clinical assessment were selected for constructing logistic prediction and BPNN models. The factors included gender, age, educational level, long-term residence, monthly family income, whether children were adults, level of hope, and social support intensity. In the BPNN model, fear of recurrence compensation was included in the output layer (No = 0, Yes = 1), with the aforementioned eight predictive variables in the input layer. A three-layer BP network was determined as most suitable for the model due to its capability for non-linear mapping and relatively short training time. Neural network nodes in the hidden layer were incrementally added, with weights adjusted systematically, selecting the number of nodes when the model reached optimum performance.
The importance scores of variables in the BPNN model were ranked and are presented in Table 3. A higher score indicates a greater influence of the corresponding variable on the model’s classification. The results showed that the level of hope, whether children were adults, social support, and family monthly income had a higher contribution to the model’s classification.
Comparison of predictive results between logistic regression and BPNN models
Comparisons between the logistic regression and BPNN models are listed in Table 4. In the training set, the logistic regression model had an AUC of 0.726 (95%CI 0.676–0.775), and the BPNN model had an AUC of 0.823 (95%CI 0.782–0.865), with BPNN significantly outperforming logistic regression (p < 0.001). In the validation set, the logistic regression model had an AUC of 0.711 (95%CI 0.630–0.792), and the BPNN model had an AUC of 0.843 (95%CI 0.778–0.907), again with BPNN showing significantly superior predictive performance (p < 0.001) (See Fig. 1 for details). DCA curve analysis revealed that the BPNN model provided greater clinical intervention benefits at various thresholds compared to logistic regression (See Fig. 2 for details).
Figure 1: (A) ROC for training set; (B) ROC for testing set.
Figure 2: (A) DCA curve in training set; (B) DCA curve in testing set.
Importance ranking of variables in the BPNN model
After a comprehensive literature review and subsequent logistic regression analysis, this study ultimately incorporated 8 significant variables into the model, based on general demographic and clinical data related to factors affecting FCR.
The relative importance scores of variables in the BPNN model indicate that the level of hope, whether children are underage and social support are much more significant than other factors such as gender, monthly income, levels of education, residence and age. Thus, this discussion will focus primarily on hope level, whether children are adults and social support.
Hope level as a significant influencing factor in cancer recurrence fear modeling
This study utilized the BPNN method to rank the importance of various factors in predicting the fear of recurrence in NSCLC patients. The analysis revealed that the level of hope had the highest importance score (0.418, normalized importance of 100%). This indicates that among all factors examined, the level of hope has the strongest predictive power for fear of recurrence. This is consistent with our previous research, which found that as levels of hope increase, they can transform into a protective factor, thereby reducing FCR. At this point, hope transitions from a risk factor to a protective factor, effectively lowering FCR levels. Moreover, no significant saturation effect was observed with the increase in hope levels [14].
Univariate analysis confirmed that the level of hope is an independent influencing factor for recurrence fear, consistent with previous research findings [23]. Hope is a positive motivational state, where higher levels of hope in patients are associated with lower levels of fear. Increased hope enhances patients’ belief and capability to combat cancer recurrence and strengthens their confidence in overcoming the disease. This aligns with findings reported in the literature [24]. Therefore, healthcare providers and family members should assist patients in enhancing their levels of hope, eliminating negative emotions, and establishing positive goals. For example, increasing patients’ levels of hope can be achieved through communication with cured patients, dissemination of new treatment methods, and sharing survival rates. This support is aimed at fostering an optimistic outlook towards the future, increasing patients’ self-acceptance, and bolstering their confidence in overcoming the disease.
Whether children are adults as a significant influencing factor in cancer recurrence fear modeling
The adulthood status of children ranks second only to hope level in importance among included factors, with an importance score of 0.171 (normalized importance of 41%). Univariate analysis shows that whether children are adults is an independent influencing factor for recurrence fear, consistent with previous research findings [25]. Patients with minor children experience higher levels of fear; they worry about potential disease recurrence, the timing of such recurrence and metastasis, and the availability of better treatment options thereafter. Primarily, their foremost concern following recurrence or metastasis is who will care for their underage children. Additionally, the inability to work normally during treatment and the significant financial burden it places on families heighten their concerns about the future costs of living and education for their children. Therefore, it is crucial for healthcare providers to pay more attention to this group, offering timely counseling, informing them about advanced treatment technologies, and devising more scientific and reasonable nursing measures. When choosing a treatment plan, the patient’s personal wishes should be respected. Efforts should be made to reduce the economic burden on patients and their families without compromising the effectiveness of the treatment. Moreover, mobilizing a robust social support system can provide substantial emotional and material support to patients and their children, alleviating fears associated with the caregivers’ illness and underage children.
Social support as a significant influencing factor in cancer recurrence fear modeling
Social support ranks third in importance among the factors considered, with an importance score of 0.13 (normalized importance of 31.2%). Patients with high levels of social support typically enjoy harmonious relationships with family, colleagues, and friends and can receive comfort, help, and care from various channels and community or organizational support, which contributes to lower levels of recurrence fear. Conversely, patients with lower social support levels may worry more about the lack of family and friends’ support and companionship if the cancer recurs. They fear being left without care and support for their future life, leading to higher levels of fear [26]. Therefore, it is recommended to mobilize a strong social support system, including healthcare professionals, family members, friends, and various social charitable organizations, to provide patients with more emotional and material comfort, encouragement, and support. This can enhance their confidence in overcoming the disease and reduce their fear of recurrence both now and in the future.
Predictive efficacy of the NSCLC postoperative fear of recurrence model
The study results indicate that the BPNN prediction model outperforms the logistic regression model. In the training set, the BPNN model achieved an Area Under the Curve (AUC) of 0.823 (95%CI: 0.782–0.865), which is superior to the logistic regression model’s AUC of 0.726 (95%CI: [insert CI here]). In the validation set, the BPNN model again showed better performance with an AUC of 0.843 (95%CI: 0.778–0.907), compared to the logistic regression model’s AUC of 0.711 (95%CI: 0.630–0.792). Across both training and validation sets, the BPNN model consistently demonstrated a larger area under the curve than the logistic regression model, exhibiting robust predictive capabilities and good calibration. These results suggest that the BPNN model, which leverages the flexibility of simulating human brain processes, has clinical significance and provides a more accurate prediction of recurrence fear in NSCLC patients. It effectively identifies the levels of FCR in early-stage NSCLC patients and is operable for practical use. Given its high level of recognition ability and precise data fitting advantages, the BPNN model can accurately screen patients at risk of recurrence fear. Overall, the BPNN model slightly outperforms our logistic regression model, making it a valuable tool in clinical settings.
FCR, a prevalent concern among patients with lung cancer, often leads to anxiety and depression. This emotional burden can disrupt communication with others, reducing social support and creating a vicious cycle that further exacerbates psychological distress [27]. Despite most participants in our study being diagnosed with early-stage non-small cell lung cancer, which typically has a lower recurrence risk, the mere diagnosis of cancer significantly overshadows details such as tumor stage or size. This overwhelming fear of recurrence persists throughout the treatment and survival journey, underscoring the need for precise prediction and timely intervention. Such measures are crucial not only for alleviating immediate psychological distress but also for breaking the cycle of reduced social support and communication barriers, thereby restoring both physiological and psychological quality of life.
The adoption of neural network models marks a significant advancement in refining the prediction of fear of recurrence, steering towards a more precise and individualized approach. These models, by incorporating time-dependent indicators like social support levels, enable ongoing assessments, thereby facilitating real-time monitoring of patients’ mental health. This dynamic approach not only aids in identifying patients who would benefit most from psychological interventions but also allows for constant evaluation of the effectiveness of these interventions. Adjustments in the strategies and intensity of interventions can be made in response to real-time feedback, significantly enhancing patient care. This method’s extensive clinical applicability could revolutionize how fear of recurrence is managed, although its implementation may pose challenges, including the need for robust data infrastructure and training for healthcare providers in interpreting complex model outputs.
Our study has several limitations. First, the data used was sourced from a single-center sample at the Thoracic Surgery Department of the Chinese Academy of Medical Sciences Cancer Hospital. Future studies could enhance the generalizability of our model through multicenter, large-sample surveys. Second, our study included nine predictive factors; future research could incorporate data and insights from multiple centers to identify additional significant factors and decisive outcomes.
This study reveals that the majority of NSCLC patients experience FCR. We identified nine statistically significant and clinically relevant predictive factors for FCR through univariate logistic regression analysis, including hope levels, whether children are adults, social support, and average family monthly income. Additionally, this study provides a proof of concept for using the Backpropagation Neural Network (BPNN) as a predictive model for FCR in NSCLC patients, which was compared with the traditional logistic regression prediction model. The results demonstrate that the BPNN model enhances the accuracy of individual predictions. Our research lays a theoretical foundation for AI-assisted prediction of risk factors associated with FCR. The BPNN model, built on nine relatively common risk factors, is easier to implement in routine clinical practice compared to more complex methods, making its results more generalizable.
Acknowledgement: We would like to express our deepest gratitude to all the patients who took part in this study and generously shared their time and experience!
Funding Statement: Supported by Beijing Hope Run Special Fund of Cancer Foundation of China (LC2022C05).
Author Contributions: The authors confirm contribution to the paper as follows: draft manuscript preparation: Man Liu; study conception and designand data collection: Lu Liu; analysis and interpretation of results: Zhuoheng Lv; Resources (equal): Hongjing Wang. All authors reviewed the results and approved the final version of the manuscript.
Availability of Data and Materials: Not applicable.
Ethics Approval: The study was independently approved by the Ethics committee of Cancer Hospital Chinese Academy of Medical Sciences (Approval No. 23/179-3921). All participants signed the informed consent in this study.
Conflicts of Interest: The authors declare that they have no conflicts of interest to report regarding the present study.
References
1. Sung H, Ferlay J, Siegel RL, Laversanne M, Soerjomataram I, Jemal A, et al. Global cancer statistics 2020: GLOBOCAN estimates of incidence and mortality worldwide for 36 cancers in 185 countries. CA: Cancer J Clin. 2021;71(3):209–49. [Google Scholar]
2. Polanski J, Jankowska-Polanska B, Rosinczuk J, Chabowski M, Szymanska-Chabowska A. Quality of life of patients with lung cancer. Onco Targets Ther. 2016;9:1023–8. [Google Scholar] [PubMed]
3. Yoo JE, Han K, Shin DW, Park SH, Cho IY, Yoon DW, et al. Conditional relative survival and competing mortality in patients who underwent surgery for lung cancer: a nationwide cohort study. Int J Cancer. 2021;148(3):626–36. doi:10.1002/ijc.v148.3. [Google Scholar] [CrossRef]
4. Jones SM, Ziebell R, Walker R, Nekhlyudov L, Rabin BA, Nutt S, et al. Association of worry about cancer to benefit finding and functioning in long-term cancer survivors. Support Care Cancer. 2017;25:1417–22. doi:10.1007/s00520-016-3537-z. [Google Scholar] [PubMed] [CrossRef]
5. Gowing S, Baker L, Tran A, Zhang Z, Ahn H, Ivanovic J, et al. Correction to: postoperative adverse events are associated with oncologic recurrence following curative‐intent resection for lung cancer. Lung. 2020;198(6):983. doi:10.1007/s00408-020-00409-3. [Google Scholar] [PubMed] [CrossRef]
6. Mutsaers B, Jones G, Rutkowski N, Tomei C, Leclair CS, Petricone-Westwood D, et al. When fear of cancer recurrence becomes a clinical issue: a qualitative analysis of features associated with clinical fear of cancer recurrence. Support Care Cancer. 2016;24(10):4207–18. doi:10.1007/s00520-016-3248-5. [Google Scholar] [PubMed] [CrossRef]
7. Kim SJ, Kang D, Kim IR, Yoon SE, Kim WS, Butow PN, et al. Impact of fear of cancer recurrence on survival among lymphoma patients. Psycho-Oncology. 2020;29(2):364–72. doi:10.1002/pon.5265. [Google Scholar] [PubMed] [CrossRef]
8. Wang Q, Wei Q, Zhong Q, Ying X, Li D, Lin X, et al. Research on the current situation and influencing factors of fear of cancer recurrence in lung cancer patients. Nurs Manag China. 2020;20(10):1595–600 (In Chinese). [Google Scholar]
9. Du J, Lin L. Application of snyder’s hope theory combined with the satir model in the rehabilitation treatment of middle-aged and young stroke patients. West China Med J. 2021;36(12):1692–9 (In Chinese). doi:10.7507/1002-0179.202106074. [Google Scholar] [CrossRef]
10. Yang L, Li Y, Wang X, Xia C, Yang L, Li X, et al. Examining the role of resilience in the relationship between social support and fear of recurrence among patients with gastric cancer on chemotherapy: a cross-sectional study in Jiangsu, China. BMJ Open. 2024;16:4(6):e078679. doi:10.1136/bmjopen-2023-078679. [Google Scholar] [PubMed] [CrossRef]
11. Zeilani RS, Younis WY, Albusoul R, Hasanien A, Hamdan-Mansour A. The buffering role of social support on the severity of physical symptoms among patients living with cancer. Int J Palliat Nurs. 2023;29(5):204–15. doi:10.12968/ijpn.2023.29.5.204. [Google Scholar] [PubMed] [CrossRef]
12. Zhong M, She F, Wang W, Ding L, Wang A. The mediating effects of resilience on perceived social support and fear of cancer recurrence in glioma patients. Psychol Res Behav Manag. 2022;15:2027–33. doi:10.2147/PRBM.S374408. [Google Scholar] [PubMed] [CrossRef]
13. Zheng W, Hu M, Liu Y. Social support can alleviate the fear of cancer recurrence in postoperative patients with lung carcinoma. Am J Trans Res. 2022;14(7):4804–11. [Google Scholar] [PubMed]
14. Liu M, Liu L, Lv Z, Mao Y, Liu Y. Investigating the determinants of fear of cancer recurrence in patients with early-stage non-small cell lung cancer: insights from a restricted cubic spline model. Cancer Med. 2024;13:e7406. doi:10.1002/cam4.v13.12. [Google Scholar] [CrossRef]
15. Ben SJ, Dörner M, Günther MP, von Känel R, Euler S. Proof of concept: predicting distress in cancer patients using back propagation neural network (BPNN). Heliyon. 2023;9(8):e18328. doi:10.1016/j.heliyon.2023.e18328. [Google Scholar] [PubMed] [CrossRef]
16. Guo Z, Dong J, Yao F, Wang Y, Wang H, Wang C, et al. Application of BP neural network in the study of hospitalization costs in patients with schizophrenia. Henan J Prev Med. 2022;33(11):820–5. doi:10.13515/j.cnki.hnjpm.1006-8414.2022.11.005. [Google Scholar] [CrossRef]
17. Dwivedi C, Rao J. Multilingual sentiment analysis for detecting mental health problems using a hybrid algorithm combining RNN and Bi-LSTM. In: Proceedings of the 2023 7th International Conference on I-SMAC (IoT in Social, Mobile, Analytics and Cloud)(I-SMAC), 2023 October 12–13; New York, NY, USA. [Google Scholar]
18. Mehnert A, Herschbach P, Berg P, Henrich G, Koch U. Fear of progression in breast cancer patients--validation of the short form of the Fear of Progression Questionnaire (FoP-Q-SF). Z Fur Psychosom Medi Psychoth. 2006;52(3):274–88. [Google Scholar]
19. Wu Q, Ye Z, Li L, Liu P. Sinicization and reliability and validity analysis of simplified scale of fear of disease progression in cancer patients. Chin J Nurs. 2015;50(12):1515–9 (In Chinese). [Google Scholar]
20. Herth K. Enhancing hope in people with a first recurrence of cancer. J Adv Nurs. 2000;32(6):1431–41. doi:10.1046/j.1365-2648.2000.01619.x. [Google Scholar] [PubMed] [CrossRef]
21. Zhang Y. A study on the relationship between hope levels and anxiety and depressive mood in maintenance hemodialysis patients. Soochow University: Suzhou, China; 2011. [Google Scholar]
22. Xiao S. The theoretical basis and research application of the Social Support Rating Scale. J Clin Psychiatr. 1994;4(2):98–100. [Google Scholar]
23. Liu M, Liu L, Zhang S, Li T, Ma F, Liu Y. Fear of cancer recurrence and hope level in patients receiving surgery for non-small cell lung cancer: a study on the mediating role of social support. Support Care Cancer. 2022;30(11):9453–60. doi:10.1007/s00520-022-07318-6. [Google Scholar] [PubMed] [CrossRef]
24. Bedaso A, Adams J, Peng W, Sibbritt D. The mediational role of social support in the relationship between stress and antenatal anxiety and depressive symptoms among Australian women: a mediational analysis. Reprod Health. 2021;18:1–10. [Google Scholar]
25. Wang D, Zheng W, Yan T, Duan H, Hou S. Fear of recurrence in gastric cancer patients and its association with depression. Modern Prev Med. 2018;45(20):3824–7. [Google Scholar]
26. Calderon C, Gustems M, Galán-Moral R, Muñoz-Sánchez MM, Ostios-García L, Jiménez-Fonseca P. Fear of recurrence in advanced cancer patients: sociodemographic, clinical, and psychological correlates. Cancers. 2024;23:16(5):909. doi:10.3390/cancers16050909. [Google Scholar] [PubMed] [CrossRef]
27. Sun H, Yang Y, Zhang J, Li T, Wang H, Garg S, et al. Fear of cancer recurrence, anxiety and depressive symptoms in adolescent and young adult cancer patients. Neuropsychiatr Dis Treat. 2019;15:857–65. doi:10.2147/NDT. [Google Scholar] [CrossRef]
Cite This Article
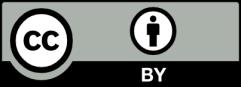
This work is licensed under a Creative Commons Attribution 4.0 International License , which permits unrestricted use, distribution, and reproduction in any medium, provided the original work is properly cited.