Open Access
ARTICLE
Build Gaussian Distribution Under Deep Features for Anomaly Detection and Localization
1 School of Computer & Software, Nanjing University of Information Science and Technology, Nanjing, 210044, China
2 College of Computer Science and Engineering, Chongqing University of Technology, Chongqing, 400054, China
* Corresponding Author: Mei Wang. Email:
Journal of New Media 2022, 4(4), 179-190. https://doi.org/10.32604/jnm.2022.032447
Received 18 May 2022; Accepted 20 June 2022; Issue published 12 December 2022
Abstract
Anomaly detection in images has attracted a lot of attention in the field of computer vision. It aims at identifying images that deviate from the norm and segmenting the defect within images. However, anomalous samples are difficult to collect comprehensively, and labeled data is costly to obtain in many practical scenarios. We proposes a simple framework for unsupervised anomaly detection. Specifically, the proposed method directly employs CNN pre-trained on ImageNet to extract deep features from normal images and reduce dimensionality based on Principal Components Analysis (PCA), then build the distribution of normal features via the multivariate Gaussian (MVG), and determine whether the test image is an abnormal image according to Mahalanobis distance. We further investigate which features are most effective in detecting anomalies. Extensive experiments on the MVTec anomaly detection dataset show that the proposed method achieves 98.6% AUROC in image-level anomaly detection and outperforms previous methods by a large margin.Keywords
Cite This Article
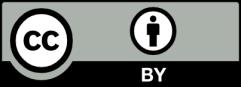