Open Access
ARTICLE
Semi-Supervised Medical Image Segmentation Based on Generative Adversarial Network
1 Hunan Applied Technology University, Changde, 415000, China
2 Central South University of Forestry and Technology, Changsha, 410004, China
3 The Second Xiangya Hospital of Central South University, Changsha, 410011, China
* Corresponding Author: Jiaohua Qin. Email:
Journal of New Media 2022, 4(3), 155-164. https://doi.org/10.32604/jnm.2022.031113
Received 10 April 2022; Accepted 11 May 2022; Issue published 13 June 2022
Abstract
At present, segmentation for medical image is mainly based on fully supervised model training, which consumes a lot of time and labor for dataset labeling. To address this issue, we propose a semi-supervised medical image segmentation model based on a generative adversarial network framework for automated segmentation of arteries. The network is mainly composed of two parts: a segmentation network for medical image segmentation and a discriminant network for evaluating segmentation results. In the initial stage of network training, a fully supervised training method is adopted to make the segmentation network and the discrimination network have certain segmentation and discrimination capabilities. Then a semi-supervised method is adopted to train the model, in which the discriminant network will generate pseudo-labels on the results of the segmentation for semi-supervised training of the segmentation network. The proposed method can use a small part of annotated dataset to realize the segmentation of medical images and effectively solve the problem of insufficient medical image annotation data.Keywords
Cite This Article
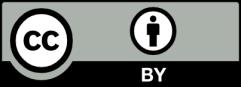
This work is licensed under a Creative Commons Attribution 4.0 International License , which permits unrestricted use, distribution, and reproduction in any medium, provided the original work is properly cited.