Open Access
REVIEW
Review of Unsupervised Person Re-Identification
School of Computer & Software, Nanjing University of Information Science and Technology, Nanjing, 210044, China
* Corresponding Author:Yang Dai. Email:
Journal of New Media 2021, 3(4), 129-136. https://doi.org/10.32604/jnm.2021.023981
Received 30 September 2021; Accepted 15 October 2021; Issue published 05 November 2021
Abstract
Person re-identification (re-ID) aims to match images of the same pedestrian across different cameras. It plays an important role in the field of security and surveillance. Although it has been studied for many years, it is still considered as an unsolved problem. Since the rise of deep learning, the accuracy of supervised person re-ID on public datasets has reached the highest level. However, these methods are difficult to apply to real-life scenarios because a large number of labeled training data is required in this situation. Pedestrian identity labeling, especially cross-camera pedestrian identity labeling, is heavy and expensive. Why we cannot apply the pre-trained model directly to the unseen camera network? Due to the existence of domain bias between source and target environment, the accuracy on target dataset is always low. For example, the model trained on the mall needs to adapt to the new environment of airport obviously. Recently, some researches have been proposed to solve this problem, including clustering-based methods, GAN-based methods, co-training methods and unsupervised domain adaptation methods.Keywords
Cite This Article
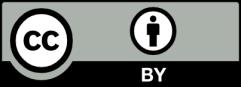
This work is licensed under a Creative Commons Attribution 4.0 International License , which permits unrestricted use, distribution, and reproduction in any medium, provided the original work is properly cited.