Open Access
ARTICLE
Low-Dose CT Image Denoising Based on Improved WGAN-gp
School of Computer Science and Technology, Nanjing Tech University, Nanjing, 211816, China.
Electrical and Computer Engineering, University of Rochester, Rochester, 14620, NY, USA.
*Corresponding Author: Xiaoli Li. Email: .
Journal of New Media 2019, 1(2), 75-85. https://doi.org/10.32604/jnm.2019.06259
Abstract
In order to improve the quality of low-dose computational tomography (CT) images, the paper proposes an improved image denoising approach based on WGAN-gp with Wasserstein distance. For improving the training and the convergence efficiency, the given method introduces the gradient penalty term to WGAN network. The novel perceptual loss is introduced to make the texture information of the low-dose images sensitive to the diagnostician eye. The experimental results show that compared with the state-of-art methods, the time complexity is reduced, and the visual quality of low-dose CT images is significantly improved.Keywords
Cite This Article
Citations
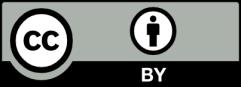
This work is licensed under a Creative Commons Attribution 4.0 International License , which permits unrestricted use, distribution, and reproduction in any medium, provided the original work is properly cited.