Open Access
ARTICLE
Multi-Label Chinese Comments Categorization: Comparison of Multi-Label Learning Algorithms
School of Computer and Software, Nanjing University of Information Science & Technology, Nanjing, 210044, China.
School of Information Technology, Deakin University, Victoria, Australia.
*Corresponding Author: Leiming Yan. Email: .
Journal of New Media 2019, 1(2), 51-61. https://doi.org/10.32604/jnm.2019.06238
Abstract
Multi-label text categorization refers to the problem of categorizing text through a multi-label learning algorithm. Text classification for Asian languages such as Chinese is different from work for other languages such as English which use spaces to separate words. Before classifying text, it is necessary to perform a word segmentation operation to convert a continuous language into a list of separate words and then convert it into a vector of a certain dimension. Generally, multi-label learning algorithms can be divided into two categories, problem transformation methods and adapted algorithms. This work will use customer's comments about some hotels as a training data set, which contains labels for all aspects of the hotel evaluation, aiming to analyze and compare the performance of various multi-label learning algorithms on Chinese text classification. The experiment involves three basic methods of problem transformation methods: Support Vector Machine, Random Forest, k-Nearest-Neighbor; and one adapted algorithm of Convolutional Neural Network. The experimental results show that the Support Vector Machine has better performance.Keywords
Cite This Article
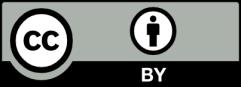
This work is licensed under a Creative Commons Attribution 4.0 International License , which permits unrestricted use, distribution, and reproduction in any medium, provided the original work is properly cited.