Open Access
ARTICLE
Retrospective Analysis of Postprandial Glucose-Response Data Collected in a Free-Living Environment
1 Converspeech Limited Liability Company, Palo Alto, 94301, Canada
2 Tastermonial, Palo Alto, 95014, Canada
3 Nourishable, Litchfield, 03052, America
4 Aventure AB Company, Lund, 26151, Sweden
* Corresponding Author: Colleen Crangle. Email:
Journal of Intelligent Medicine and Healthcare 2022, 1(2), 91-102. https://doi.org/10.32604/jimh.2022.038379
Received 10 November 2022; Accepted 12 December 2022; Issue published 05 January 2023
Abstract
Postprandial glucose responses provide vital information on an individual’s risk of major diet-related chronic diseases. This study features digital health technology, namely Continuous Glucose Monitoring (CGM) sensors, along with mobile devices (iPhones running an app) used to collect data from individuals and their environment, specifically nutritional information on what they eat and drink. The paper presents a retrospective analysis of data collected during an investigation into the use of a functional drink taken as a supplement with a standardized meal to reduce postprandial responses to that meal. Given that there are consequential differences between individuals in their postprandial glucose responses, these real-world data are analyzed at the level of the individual. The aim of the study was to assess the feasibility of collecting real-world data on supplements and postprandial glucose responses outside of a controlled laboratory environment, synthesizing the data from the different sources, and producing individualized results. Of the 40 individuals invited to conduct 6 tests, 3 with and 3 without the supplement, 19 provided from 1 to 6 valid tests, producing 0 to 6 comparisons each. Four individuals contributed at least 5 comparisons each, the minimum number required for the exact 1-sided Wilcoxon signed-rank test. The conclusion is that a real-world study of supplements and postprandial glucose responses is feasible, with an increase in the number of tests requested of each individual, a simplification of the testing protocol, and steps taken to reduce the number of invalid tests.Keywords
Metabolic health in American adults is critically poor. Fewer than one-third of normal-weight adults are metabolically healthy, and in overweight and obese individuals only 8% and 0.5%, respectively [1]. Diet has the greatest influence on a person’s glucose levels, with elevated postprandial glucose responses an independent risk factor for a variety of diet-related chronic diseases and, in its association with obesity, many other negative health consequences [2–5]. Two developments have converged to enable data collection in real-world settings (that is, outside laboratories) on postprandial glucose responses.
• Continuous Glucose Monitoring devices, with improved levels of accuracy and reliability, now let individuals collect real-world data on their glucose responses under free-living conditions [6,7].
• Comprehensive nutrition and ingredient databases (such the USDA FoodData Central) provide immediate access to nutrient profile data on packaged foods being consumed in the home [8].
Numerous studies, including large randomized controlled trials, have shown how using CGMs can improve glycemic control in people with type 1 or 2 diabetes [9–11] and, more recently, in people without diagnosed diabetes [12]. The use of CGMs to monitor postprandial glucose responses is also increasingly finding its way into nutrition guidance [13,14].
This study is a retrospective analysis of data collected during an investigation into the use of a functional drink taken as a supplement with a meal to reduce postprandial glucose responses [6,15]. Individuals wore CGM devices in a free-living environment (their homes) and used a mobile app (Tastermonial) to synchronize data from the CGM device with data on the food or supplement they were testing. The purpose was to determine the feasibility of conducting such tests in the individuals’ own homes and to assess the quality and value of data collected.
This retrospective analysis is important for two reasons. First, even though it is now well established that interventions targeting elevated postprandial glucose responses significantly reduce the risk of metabolic-related conditions, that is not the end of the story [16,17]. Evidence is growing that there are consequential differences in individuals’ postprandial glucose responses [2,18]. It is no longer accepted that the same food eaten by different people produces a glucose response similar enough that characteristics of the food itself can be used as a guide to that individual’s postprandial responses. It is crucial that postprandial glucose responses be studied at the level of the individual.
Second, real-world data are increasingly needed to understand glucose responses outside the laboratory in the individual’s regular living environment. Real-world studies have grown significantly in the past 6 years in the USA since the publication of the Food and Drug Administration’s (FDA) 21st Century Cures Act [19,20]. Typically, real-world studies use data from several sources. This study uses digital health technology (CGM sensors) and mobile devices (iPhones running an app) to collect data in the individual’s home setting. This retrospective analysis will provide important insight into how to design real-world studies of intelligent systems that synthesize physiological sensor data (from CGMs) and data obtained from the environment (the food eaten at home) to provide insights at the individual level to address diet-related disorders.
The Tastermonial mobile app (available on Apple iPhones) allows individuals to log what they are eating by scanning the product’s barcode and have the nutritional information of that logged food or drink be synchronized with the glucose readings from the CGM they are wearing (FreeStyle Libre 14-day). In an open-label comparison of a standardized meal with and without a supplement, individuals from Tastermonial’s community of users were invited to be testers using their CGMs with meals and supplements mailed to their homes.
Participants. The testers were male or female, not diagnosed with type-1 or type-2 diabetes, nor taking any medication that affected blood glucose. Excluded were anyone under 18 or over 72, any females who were pregnant and anyone with an underlying health condition that warranted non-participation.
The standardized meal was the Beviva Purpo all-in-one cereal from Beviva Foods, with 36 g total carbohydrates, 8 g fiber (28 g net carbohydrates) in each serving. The supplement was a functional drink (Good Idea) containing amino acids and chromium (Good Idea, Good Idea Inc. CA [6,15]). Good Idea is a zero-carbohydrate drink that tastes like ordinary flavored mineral water. Testers could choose any out of three flavors. Free samples of the meal and drink were shipped to each individual along with instructions on how to conduct the tests.
Instructions. All tests were to be conducted at breakfast after an overnight fast of at least 8 h. Individuals were told to consume the entire cereal meal and functional drink within 10–15 min, with 1/3 of the drink being consumed before starting the meal and the rest during the meal. They were also told to refrain from exercise for the two hours before and after the test and, except for plain water or other unflavored flat beverages such as plain tea or coffee, not to eat or drink anything else for the 2 h before and after the test. Two double servings of the cereal with and without the supplement and one single serving with and without were to be tested. At the start of each test, the individual was to use the Tastermonial app to scan the barcode on the cereal (and the barcode on the supplement as well on days 3, 5, and 7) so that the starting time of the meal would be logged and the glucose readings of the 2 h that followed could be noted as the postprandial glucose response period for each test.
The test period was to last 14 days. On day 1, a new CGM was to be applied and no further action taken. On days 2 and 4, double servings of the cereal meal were consumed and on days 3 and 5 the cereal meal was taken with the supplement as instructed. On day 6, individuals consumed a single serving of the cereal alone and on day 7 a single serving together with the supplement. These 6 tests could be conducted all within the first 7 days or they could be spread out over the span of the 14-day CGM.
Test Validity. To ensure that each test was conducted according to the instructions (that is, on an empty stomach), the glucose value at the start of each test was checked to see if it was within what is considered the normal range (70–100 mg/dL). If it was, the test was deemed valid and included in the analysis. If not, the test was excluded from analysis.
The primary outcome in this retrospective analysis was the change in postprandial peak glucose values for the meals alone and the meals with the supplement, with separate comparisons made between single-serving and double-serving tests and the data analyzed at the individual level. Full compliance with the protocol would yield 5 comparisons, 4 from the double-serving tests and one from the single. The peak value is the maximum increase measured in the 2 h after the start of the test. Secondary outcomes were the proportion of comparisons that showed a reduction for an individual and that average reduction itself.
Metrics obtained from the CGM devices. The glucose value at the start of a meal was subtracted from the maximum postprandial glucose value to give Mi for meal i eaten alone (i = 1, …, 3) and MSj for meal j consumed with the supplement (i = 1, …, 3), values that gave in each instance the postprandial peak (the highest glucose concentration increase within the 2-h period after the start of the meal). Paired comparisons were made for each individual of their Mi and MSj values and the differences Mi − MSj calculated. See sample comparison in Fig. 1.
Figure 1: Sample postprandial glucose peaks for meal with the supplement (MS) and without (M)
Statistical Analysis. The Wilcoxon signed-rank test was used to evaluate the changes in the postprandial peak glucose values for each individual [21]. That is, pairs of related with- and without-supplement increases (Mi and MSj) were compared and the differences Mi − MSj calculated. The Wilcoxon signed-rank test is suitable for small sample sizes and for data that cannot be assumed to be normally distributed. For small sample sizes, an exact test is used to determine the significance of Wilcoxon’s W. For the secondary outcomes, Spearman’s Rank Correlation Coefficient (rho) was used to compare across all individuals the proportion of reductions in postprandial glucose peaks and those average reductions.
Ethics Statement. The CGM data of four individuals were analyzed retrospectively. A CGM device has a sensor that measures the interstitial fluid glucose concentrations, a reliable proxy for blood glucose, for 14 days. For the CGMs used in this analysis, the glucose data were measured at 15-min intervals and the sensor had to be scanned (using an app or a companion Freestyle reader) at least once every 8 h. The device is small, about the size of a quarter coin in US currency, with the individual typically hardly aware of the sensor. The individuals were not in a controlled environment; they carried out their daily activities independently with the CGM device attached to their upper arm. No IRB approval was obtained for the study but all individuals gave written informed consent for the use of their data.
Of the 40 individuals invited to join the test, 26 (16 female, 10 male) completed at least some tests. There were 8/26 testers who were overweight (body mass index (BMI) >= 25). Average age was 41 (range 19–67). The average fasting glucose value was 93 (SD 16) mg/dL, with 7/26 of the testers having a value over 100 mg/dL. Of the 26, 19 provided from 1 to 6 valid tests, with the number of comparisons then varying from 0 to 6 (some conducted only 2-serving tests, yielding an extra comparison). Four individuals contributed at least 5 comparisons each, the minimum number required for the exact 1-sided Wilcoxon signed-rank test (see Table 1).
Table 2 shows demographic and glucose peak data for the 4 individuals who provided at least 5 comparisons. Table 3 has data for all 19 individuals.
For the 4 individuals with enough comparisons, skewness and kurtosis were all greater than 2, as would be expected in data that are not normally distributed. Of these 4 individuals, only individual V62 had results for which the null hypothesis could be rejected at the 95% alpha level. See Table 4.
Across all testers, 51% (30/59) of the comparisons entailed reductions in the peak postprandial glucose value with the supplement. The average reduction was 14.9 (SD 13.7). For the 29/59 comparisons that did not show a reduction, one was a difference of zero, and the average increase for the remaining 28 was 12.7 mg/dL (SD 11.63). Across all testers, there was a strong positive correlation between the proportion of reductions for the individual and the individual’s average reduction, rho (17) = 0.62, P = 0.004. That is, those who had a higher proportion of reductions had a higher average reduction. This correlation was calculated simply to assess reasonableness of the results. Taking into account only those individuals for whom there were 5 or more comparisons, there was a stronger correlation but with so few data points not one that was statistically significant, rho (2) = 0.75, p = 0.25.
This study explored the feasibility of conducting real-world studies of postprandial glucose responses that are analyzed at the level of the individual. Such analysis is important because of the now widely acknowledged fact that there are differences between individuals in their postprandial glucose responses to the same food. Furthermore, individualized results open up the possibility of individualized action plans for health improvement. The study also set out to guide the design of a full individual-level (single-subject or n-of-1) study [22,23] in free-living environments with CMGs and nutrition data collection. N-of-1 studies explore variability in individuals’ responses in an objective way while simultaneously leading to an informed decision about the best course of action for an individual using their own data. The number of measures taken on an individual, not the number of individuals being studied, enables statistically and clinically meaningful conclusions to be drawn.
A majority (15/19) of those completing at least some tests had difficulty completing enough tests to yield sufficient comparisons. Limitations in the test instructions and/or the mobile app interface could have made the tests difficult to complete, as well as possible boredom to the test meal when repeated many times in a row. The instructions have already been reworked for greater clarity and the food logging interface in the app is being simplified in preparation for a focus-group study.
Testing both single servings and double servings reduced the number of comparisons that could be made. There is currently not enough evidence to suggest that both serving sizes need to be tested on each individual. The individual’s typical serving size may yield the most relevant data; that is, if someone would typically eat two servings of the cereal then they would do only double-serving comparisons.
Of the tests completed by the 19 testers, 20% (21/105) were deemed invalid. There were several reasons, including out-of-range glucose values at the start of a test meal. No reports were given of suspected faulty sensors. In follow-up studies, it may make sense to check that the glucose level at the start of each test is within the individual’s nocturnal glucose range (that is, average glucose values from 1 am to 6 am each morning from Day 2 till Day 14 of the sensor), as opposed to checking that it is within the range considered normal [24].
Overall, the limiting factor in this study was the number of valid meals that could be compared. Although the Wilcoxon signed-rank test could be run for four of the testers, their small sample sizes (of 5 and 6 repeated meals) preclude strong conclusions. If n = 8 comparable meals were tested, 4 with and 4 without the supplement, and allowing for 10% of the tests to be invalid, approximately 14 comparisons (4 * 4 * 0.9) would be generated for each person. To permit stratification for factors such as BMI, age, sex and average fasting glucose value–and possibly over-the-counter herbal formulations or oral contraceptive individuals may be taking–a greater number of testers would likely be needed, however.
A legitimate question is what reduction (or lack of reduction) should be considered of clinical or practical importance in assessing postprandial glucose differences. There’s some indication that for the peak 2-h value, a reduction of 0.60 mmol/L (around 10 mg/dL) or a reduction in the range 3%–12% is meaningful at the group level, but very little data is available for clinically significant 2-h postprandial differences at the individual level [25,26]. Using a cutoff of 10 mg/dL, the individual results in this study do not change appreciably, but there are too few data points to draw strong conclusions.
One tester (Fn1) had what appear to be anomalous results, with a clear increase in the 2-h peak value with the supplement for 4 out of 5 comparisons. Non-adherence to the study protocol must be considered a possible cause. No possible explanation could be found in the tester’s full set of CGM data. The coefficient of variation was 17%, compared to 14%, 18% and 19% for the other 3 individual-data testers. In identifying the percentage of spikes >30 mg/dL across all CGM data for a tester using a moving median filter of window size 10, Fn1 was not shown to be substantially different (1.49% vs. 1.94%, 0.41% and 2.41%) [27]. Fn1 also had stable glucose levels before all tests. Of interest is the fact that tester Fn1 was the only participant who reported taking oral contraceptives. A follow-up study that included other testers on oral contraceptives could possibly show similar results, or point to some other, as yet unknown, reason for Fn1’s results.
This retrospective analysis at the level of the individual suggests that a full n-of-1 study could provide important information. We know that individuals, whether diagnosed with diabetes or not, vary in the extent to which they understand metabolic health and vary in their interest and curiosity about the topic [28–30]. With growing evidence that individuals also vary in their postprandial glucose responses [2,18] and given the global increase in metabolic diseases, the time is ripe for studies that provide a more personalized view of postprandial glucose responses in free-living conditions.
Funding Statement: The authors received no specific funding for this study.
Conflicts of Interest: The authors declare that they have no conflicts of interest to report regarding the present study.
References
1. J. Araújo, J. W. Cai and J. Stevens, “Prevalence of optimal metabolic health in American adults: National health and nutrition examination survey 2009–2016,” Metabolic Syndrome and Related Disorders, vol. 17, no. 1, pp. 46–52, 2019. [Google Scholar]
2. D. Zeevi, T. Korem, N. Zmora, D. Israeli, D. Rothschild et al., “Personalized nutrition by prediction of glycemic responses,” Cell, vol. 163, no. 5, pp. 1079–1094, 2015. [Google Scholar]
3. B. Gallwitz, “Implications of postprandial glucose and weight control in people with type 2 diabetes: Understanding and implementing the international diabetes federation guidelines,” Diabetes Care, vol. 32, no. 2, pp. S322–S325, 2009. [Google Scholar]
4. H. Haller, “The clinical importance of postprandial glucose,” Diabetes Research and Clinical Practice, vol. 40, pp. S43–S49, 1998. [Google Scholar]
5. A. W. Barclay, P. Petocz, J. McMillan-Price, V. M. Flood, T. Prvan et al., “Glycemic index, glycemic load, and chronic disease risk--a meta-analysis of observational studies,” The American Journal of Clinical Nutrition, vol. 87, no. 3, pp. 627–637, 2008. [Google Scholar]
6. A. Samigullin, P. M. Humpert and E. Östman, “Continuous glucose monitoring as a close to real life alternative to meal studies-a pilot study with a functional drink containing amino acids and chromium,” Frontiers in Medical Technology, vol. 4, pp. 1–7, 2022. [Google Scholar]
7. S. T. Bailey and S. Alva, “Landscape of continuous glucose monitoring (CMG) and integrated CMG: Accuracy considerations,” Diabetes Technology & Therapeutics, vol. 23, no. 3, pp. S5–S11, 2021. [Google Scholar]
8. B. Larrick, A. Kretser and K. McKillop, “Update on “a partnership for public health: USDA global branded food products database”,” Journal of Food Composition and Analysis, vol. 105, pp. 104250, 2022. [Google Scholar]
9. T. Haak, H. Hanaire, R. Ajjan, N. Hermanns, J. Riveline et al., “Use of flash glucose sensing technology for 12 months as a replacement for blood glucose monitoring in insulin-treated type 2 diabetes,” Diabetes Therapy, vol. 8, no. 3, pp. 573–586, 2017. [Google Scholar]
10. M. Lind, W. Polonsky and I. B. Hirsch, “Continuous glucose monitoring vs. conventional therapy for glycemic control in adults with type 1 diabetes treated with multiple daily insulin injections: The GOLD randomized clinical trial,” Jama, vol. 317, no. 4, pp. 379–387, 2017. [Google Scholar]
11. P. Bolinder, R. Antuna, P. Geelhoed-Duijvestijn, J. Kröger and R. Weitgasser, “Novel glucose-sensing technology and hypoglycemia in type 1 diabetes: A multicentre, non-masked, randomised controlled trial,” The Lancet, vol. 388, no. 10057, pp. 2254–2263, 2016. [Google Scholar]
12. E. Selvin, D. Wang, O. Tang, M. Minotti, J. B. Echouffo-Tcheugui et al., “Glucose patterns in very old adults: A pilot study in a community-based population,” Diabetes Technology & Therapeutics, vol. 23, no. 11, pp. 737–744, 2021. [Google Scholar]
13. J. Kröger, T. Siemgund, O. Schubert-Olesen, W. Keuthage, M. Lettmann et al., “AGP and nutrition-analysing postprandial glucose courses with CGM,” Diabetes Research and Clinical Practice, vol. 174, pp. 108738, 2021. [Google Scholar]
14. N. J. Schork and L. H. Goetz, “Single-subject studies in translational nutrition research,” Annual Review of Nutrition, vol. 37, pp. 395–422, 2017. [Google Scholar]
15. E. Östman, A. Samigullin, L. Heyman-Lindén, K. Andersson, I. Björck et al., “A novel nutritional supplement containing amino acids and chromium decreases postprandial glucose response in a randomized, double-blind, placebo-controlled study,” PLoS One, vol. 15, no. 6, pp. e0234237, 2020. [Google Scholar]
16. N. M. J. Hanssen, M. J. Kraakman, M. C. Flynn, P. R. Nagareddy, C. G. Schalkwijk et al., “Postprandial glucose spikes, an important contributor to cardiovascular disease in diabetes?,” Frontiers in Cardiovascular Medicine, vol. 18, no. 7, pp. 570553, 2020. [Google Scholar]
17. E. Bonora and M. Muggeo, “Postprandial blood glucose as a risk factor for cardiovascular disease in type II diabetes: The epidemiological evidence,” Diabetologia, vol. 44, pp. 2107–2114, 2001. [Google Scholar]
18. C. Noecker and E. Borenstein, “Getting personal about nutrition,” Trends in Molecular Medicine, vol. 22, no. 2, pp. 83–85, 2016. [Google Scholar]
19. J. Concato and J. Corrigan-Curay, “Real-world evidence-where are we now?,” The New England Journal of Medicine, vol. 386, no. 18, pp. 1680–1682, 2022. [Google Scholar]
20. F. Schad and A. Thronicke, “Real-world evidence-current developments and perspectives,” International Journal of Environmental Research and Public Health, vol. 19, no. 16, pp. 10159, 2022. [Google Scholar]
21. D. Rey and M. Neuhäuser, “Wilcoxon-signed-rank test,” in International Encyclopedia of Statistical Science, Berlin, Heidelberg: Springer, pp. 1658–1659, 2011. [Google Scholar]
22. F. Vitali, Q. Li, A. G. Schissler, J. Berghout, C. Kenost et al., “Developing a `personalome' for precision medicine: Emerging methods that compute interpretable effect sizes from single-subject transcriptomes,” Briefings in Bioinformatics, vol. 20, no. 3, pp. 789–805, 2019. [Google Scholar]
23. F. Klaassen, C. M. Zedelius, H. Veling, H. Aarts and H. Hoijtink, “All for one or some for all? Evaluating informative hypotheses using multiple N = 1 studies,” Behavior Research Methods, vol. 50, no. 6, pp. 2276–2291, 2018. [Google Scholar]
24. L. Vu, S. Kefayati, T. Idé, V. Pavuluri, G. Jackson et al., “Predicting nocturnal hypoglycemia from continuous glucose monitoring data with extended prediction horizon,” American Medical Informatics Association, vol. 2019, pp. 874–882, 2020. [Google Scholar]
25. Z. Li, L. Zhao, L. Yu and J. Yang, “Head-to-head comparison of the hypoglycemic efficacy and safety between dipeptidyl peptidase-4 inhibitors and α-glucosidase inhibitors in patients with type 2 diabetes mellitus: A meta-analysis of randomized controlled trials,” Frontiers in Pharmacology, vol. 10, pp. 777, 2019. [Google Scholar]
26. D. W. Davis, J. W. Navalta, G. R. McGinnis, R. Serafica, K. Izuora et al., “Effects of acute dietary polyphenols and post-meal physical activity on postprandial metabolism in adults with features of the metabolic syndrome,” Nutrients, vol. 12, no. 4, pp. 1120, 2020. [Google Scholar]
27. E. G. Dana and A. Jennifer, “Identification of spikes in time series,” Epidemiologic Methods, vol. 8, no. 1, pp. 20180005, 2019. [Google Scholar]
28. C. E. Crangle, C. Bradley, P. F. Carlin, R. J. Esterhay, R. Harper et al., “Exploring patient information needs in type 2 diabetes: A cross sectional study of questions,” PloS One, vol. 13, no. 11, pp. e0203429, 2018. [Google Scholar]
29. C. E. Crangle, C. Bradley, P. F. Carlin, R. J. Esterhay, R. Harper et al., “Soliciting and responding to patients’ questions about diabetes through online sources,” Diabetes Technology & Therapeutics, vol. 19, no. 3, pp. 194–199, 2017. [Google Scholar]
30. C. E. Crangle, C. Bradley, P. F. Carlin, R. J. Esterhay, R. Harper et al., “Consumer questions about diabetes: A health literacy assessment,” in Poster Session Presented at: 8th Annual Health Literacy Research Conf., Bethesda, MD, USA, pp. 12–13, 2019. [Google Scholar]
Cite This Article
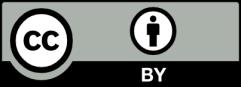