Open Access
ARTICLE
Data-Efficient Image Transformers for Robust Malware Family Classification
1 Department of Computer Science, Kwame Nkrumah University of Science and Technology, Kumasi, AK-315-1475, Ghana
2 Department of Mathematics, Kwame Nkrumah University of Science and Technology, Kumasi, AK-315-1475, Ghana
* Corresponding Author: Boadu Nkrumah. Email:
Journal of Cyber Security 2024, 6, 131-153. https://doi.org/10.32604/jcs.2024.053954
Received 14 May 2024; Accepted 21 August 2024; Issue published 17 December 2024
Abstract
The changing nature of malware poses a cybersecurity threat, resulting in significant financial losses each year. However, traditional antivirus tools for detecting malware based on signatures are ineffective against disguised variations as they have low levels of accuracy. This study introduces Data Efficient Image Transformer-Malware Classifier (DeiT-MC), a system for classifying malware that utilizes Data-Efficient Image Transformers. DeiT-MC treats malware samples as visual data and integrates a newly developed Hybrid GridBay Optimizer (HGBO) for hyperparameter optimization and better model performance under varying malware scenarios. With HGBO, DeiT-MC outperforms the state-of-the-art techniques with a strong accuracy rate of 94% on the MaleViS and 92% on MalNet-Image Tiny datasets. Therefore, this work presents DeiT-MC as a promising and robust solution for classifying malware families using image analysis techniques and visualization approaches.Keywords
Cite This Article
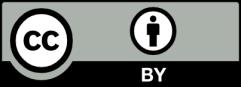
This work is licensed under a Creative Commons Attribution 4.0 International License , which permits unrestricted use, distribution, and reproduction in any medium, provided the original work is properly cited.