Open Access
ARTICLE
Securing Web by Predicting Malicious URLs
School of Computer Science, University of Hertfordshire, Hatfield, AL10 9AB, UK
* Corresponding Author: Meenakshi Megavarnam. Email:
Journal of Cyber Security 2024, 6, 117-130. https://doi.org/10.32604/jcs.2024.048332
Received 05 December 2023; Accepted 08 November 2024; Issue published 06 December 2024
Abstract
A URL (Uniform Resource Locator) is used to locate a digital resource. With this URL, an attacker can perform a variety of attacks, which can lead to serious consequences for both individuals and organizations. Therefore, attackers create malicious URLs to gain access to an organization’s systems or sensitive information. It is crucial to secure individuals and organizations against these malicious URLs. A combination of machine learning and deep learning was used to predict malicious URLs. This research contributes significantly to the field of cybersecurity by proposing a model that seamlessly integrates the accuracy of machine learning with the swiftness of deep learning. The strategic fusion of Random Forest (RF) and Multilayer Perceptron (MLP) with an accuracy of 81% represents a noteworthy advancement, offering a balanced solution for robust cybersecurity. This study found that by combining RF and MLP, an efficient model was developed with an accuracy of 81% and a training time of 33.78 s.Keywords
Cite This Article
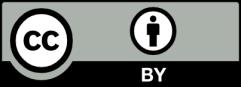
This work is licensed under a Creative Commons Attribution 4.0 International License , which permits unrestricted use, distribution, and reproduction in any medium, provided the original work is properly cited.