Open Access
ARTICLE
A Comparative Performance Analysis of Machine Learning Models for Intrusion Detection Classification
1 School of Electronics and Control Engineering, Chang’an University, Xi’an, 710000, China
2 School of Information Engineering, Chang’an University, Xi’an, 710000, China
* Corresponding Author: Amna Khatoon. Email:
Journal of Cyber Security 2024, 6, 1-23. https://doi.org/10.32604/jcs.2023.046915
Received 19 October 2023; Accepted 21 November 2023; Issue published 03 January 2024
Abstract
The importance of cybersecurity in contemporary society cannot be inflated, given the substantial impact of networks on various aspects of daily life. Traditional cybersecurity measures, such as anti-virus software and firewalls, safeguard networks against potential threats. In network security, using Intrusion Detection Systems (IDSs) is vital for effectively monitoring the various software and hardware components inside a given network. However, they may encounter difficulties when it comes to detecting solitary attacks. Machine Learning (ML) models are implemented in intrusion detection widely because of the high accuracy. The present work aims to assess the performance of machine learning algorithms in the context of intrusion detection, providing valuable insights into their efficacy and potential for enhancing cybersecurity measures. The main objective is to compare the performance of the well-known ML models using the UNSW-NB15 dataset. The performance of the models is discussed in detail with a comparison using evaluation metrics and computational performance.Keywords
Cite This Article
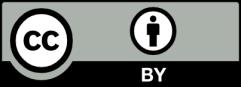