Open Access
ARTICLE
Empirical Analysis of Neural Networks-Based Models for Phishing Website Classification Using Diverse Datasets
Department of Computer Science, City University of Science and Information Technology, Peshawar, Pakistan
* Corresponding Author: Saifullah Jan. Email:
Journal of Cyber Security 2023, 5, 47-66. https://doi.org/10.32604/jcs.2023.045579
Received 31 August 2023; Accepted 21 November 2023; Issue published 28 December 2023
Abstract
Phishing attacks pose a significant security threat by masquerading as trustworthy entities to steal sensitive information, a problem that persists despite user awareness. This study addresses the pressing issue of phishing attacks on websites and assesses the performance of three prominent Machine Learning (ML) models—Artificial Neural Networks (ANN), Convolutional Neural Networks (CNN), and Long Short-Term Memory (LSTM)—utilizing authentic datasets sourced from Kaggle and Mendeley repositories. Extensive experimentation and analysis reveal that the CNN model achieves a better accuracy of 98%. On the other hand, LSTM shows the lowest accuracy of 96%. These findings underscore the potential of ML techniques in enhancing phishing detection systems and bolstering cybersecurity measures against evolving phishing tactics, offering a promising avenue for safeguarding sensitive information and online security.Keywords
Cite This Article
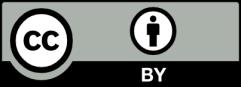
This work is licensed under a Creative Commons Attribution 4.0 International License , which permits unrestricted use, distribution, and reproduction in any medium, provided the original work is properly cited.