Open Access
ARTICLE
Credit Card Fraud Detection on Original European Credit Card Holder Dataset Using Ensemble Machine Learning Technique
Department of Electrical and Electronic Engineering, FETBE, UCSI University, Kuala Lumpur, 56000, Malaysia
* Corresponding Author: Yih Bing Chu. Email:
Journal of Cyber Security 2023, 5, 33-46. https://doi.org/10.32604/jcs.2023.045422
Received 26 August 2023; Accepted 09 October 2023; Issue published 03 November 2023
Abstract
The proliferation of digital payment methods facilitated by various online platforms and applications has led to a surge in financial fraud, particularly in credit card transactions. Advanced technologies such as machine learning have been widely employed to enhance the early detection and prevention of losses arising from potentially fraudulent activities. However, a prevalent approach in existing literature involves the use of extensive data sampling and feature selection algorithms as a precursor to subsequent investigations. While sampling techniques can significantly reduce computational time, the resulting dataset relies on generated data and the accuracy of the pre-processing machine learning models employed. Such datasets often lack true representativeness of real-world data, potentially introducing secondary issues that affect the precision of the results. For instance, under-sampling may result in the loss of critical information, while over-sampling can lead to overfitting machine learning models. In this paper, we proposed a classification study of credit card fraud using fundamental machine learning models without the application of any sampling techniques on all the features present in the original dataset. The results indicate that Support Vector Machine (SVM) consistently achieves classification performance exceeding 90% across various evaluation metrics. This discovery serves as a valuable reference for future research, encouraging comparative studies on original dataset without the reliance on sampling techniques. Furthermore, we explore hybrid machine learning techniques, such as ensemble learning constructed based on SVM, K-Nearest Neighbor (KNN) and decision tree, highlighting their potential advancements in the field. The study demonstrates that the proposed machine learning models yield promising results, suggesting that pre-processing the dataset with sampling algorithm or additional machine learning technique may not always be necessary. This research contributes to the field of credit card fraud detection by emphasizing the potential of employing machine learning models directly on original datasets, thereby simplifying the workflow and potentially improving the accuracy and efficiency of fraud detection systems.Keywords
Cite This Article
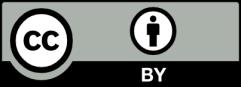
This work is licensed under a Creative Commons Attribution 4.0 International License , which permits unrestricted use, distribution, and reproduction in any medium, provided the original work is properly cited.