Open Access
ARTICLE
Seeded Transfer Learning for Enhanced Attack Trace and Effective Deception
1 Department of Amity Institute of Information Technology (AIIT), Amity University, Noida, Uttar Pradesh, 201301, India
2 Department of Information Technology, Bhairab Ganguly College, Kolkata, West Bengal, 700056, India
* Corresponding Author: Jalaj Pateria. Email:
Journal of Cyber Security 2022, 4(4), 223-238. https://doi.org/10.32604/jcs.2023.040186
Received 08 March 2023; Accepted 27 April 2023; Issue published 10 August 2023
Abstract
Cyberattacks have reached their peak during COVID-19, and intruders urge to gain the upper hand in the cybersecurity battlefield, even gaining dominance. Now intruders are trying harder to elude behavior analysis techniques, which in turn gets organization security to come for a toss. This phenomenon is even more prevalent in agentless environments (IOT devices, mobile devices), where we do not have any access to edge devices and rely on packet data to predict any attack and its actors. In this paper, we shall be discussing enhancing the accuracy of anomalous behavior detection techniques for efficient threat intelligence and revamping deception using a unique machine learning model training technique termed “Seeded Transfer Learning”, in this technique data is reshaped into knowledge to fit to the target domain in small capsules of information in real-time or near real-time. In this method we shall be using seeds of data or real-time small data sequences to train machine learning models along with that sustaining on the ideology of positive transfer learning techniques where previous learning will be made more effective by taking advantage of a new training set. Comprehensive experiments are done on ANN-(Artificial Neural Network) and result revels best incremental performance from ∼91% to ∼97%. Experiment summarized that while training model on data seeds we have achieved great accuracy with limited computing resources and time, additionally model is trained on latest attack dataset which helped in identifying attacks effectively which in turns translates to effective defense against future unknow attacks.Keywords
Cite This Article
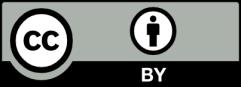
This work is licensed under a Creative Commons Attribution 4.0 International License , which permits unrestricted use, distribution, and reproduction in any medium, provided the original work is properly cited.