Open Access
ARTICLE
Phishing Scam Detection on Ethereum via Mining Trading Information
1 Engineering Research Center of Digital Forensics, Ministry of Education, Nanjing University of Information Science and Technology, Nanjing, China
2 The State Key Laboratory of Integrated Services Networks, Xidian University, Xi’an, Shaanxi, China
* Corresponding Author: Zhangjie Fu. Email:
Journal of Cyber Security 2022, 4(3), 189-200. https://doi.org/10.32604/jcs.2022.038401
Received 01 July 2022; Accepted 30 September 2022; Issue published 01 February 2023
Abstract
As a typical representative of web 2.0, Ethereum has significantly boosted the development of blockchain finance. However, due to the anonymity and financial attributes of Ethereum, the number of phishing scams is increasing rapidly and causing massive losses, which poses a serious threat to blockchain financial security. Phishing scam address identification enables to detect phishing scam addresses and alerts users to reduce losses. However, there are three primary challenges in phishing scam address recognition task: 1) the lack of publicly available large datasets of phishing scam address transactions; 2) the use of multi-order transaction information requires a large number of queries and computations; and 3) the extraction of phishing scam address features relies on machine learning methods excessively, which leads to the loss of practical meaning and is harmful to the research of phishing scam addresses. This paper proposes a systematic phishing scam address recognition scheme, to simultaneously overcome the three challenges in phishing scam address recognition. In this paper, a systematic phishing scam address recognition scheme is proposed to addresses these issues. Specifically, due to the insufficient number of address tagged in the existing publicly available Ethereum phishing scam address transaction dataset, we first construct a transaction dataset involving over 10000 tagged addresses. To the best of our knowledge, this is the largest dataset of tagged addresses for Ethereum phishing scam detection. Then, we design a new heuristic rule to implement feature extraction of address nodes by analysing the traditional financial involved accounts combined with information specific to Ethernet transactions. After that, a novel adaptive feature importance filtering method is designed to adaptively adjust the filtering threshold based on the final classification results, which reduce the feature dimensionality while ensuring a certain detection performance. Finally, random forest is used to classify whether the addresses is a phishing scam address or not. Extensive experiments on real Ethereum datasets show that our approach (98.89% Precision, 98.35% Recall, 98.62% F1) achieves state-of-the-art performance.Keywords
Cite This Article
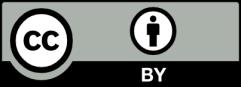
This work is licensed under a Creative Commons Attribution 4.0 International License , which permits unrestricted use, distribution, and reproduction in any medium, provided the original work is properly cited.