Open Access
ARTICLE
CenterPicker: An Automated Cryo-EM Single-Particle Picking Method Based on Center Point Detection
1 Key Laboratory of Intelligent Computing Information Processing, Ministry of Education, Computer Science College of Xiangtan University, Xiangtan, Hunan Province, 411100, China
2 Department of Computer Science, the University of Georgia, Athens, Georgia, USA
* Corresponding Author: Jianquan Ouyang. Email:
Journal of Cyber Security 2022, 4(2), 65-77. https://doi.org/10.32604/jcs.2022.028065
Received 01 May 2022; Accepted 02 June 2022; Issue published 04 July 2022
Abstract
Cryo-electron microscopy (cryo-EM) has become one of the mainstream techniques for determining the structures of proteins and macromolecular complexes, with prospects for development and significance. Researchers must select hundreds of thousands of particles from micrographs to acquire the database for single-particle cryo-EM reconstruction. However, existing particle picking methods cannot ensure that the particles are in the center of the bounding box because the signal-to-noise ratio (SNR) of micrographs is extremely low, thereby directly affecting the efficiency and accuracy of 3D reconstruction. We propose an automated particle-picking method (CenterPicker) based on particle center point detection to automatically select a large number of high-quality particles from low signal-to-noise, low-contrast refrigerated microscopy images. The method uses a fully convolutional neural network to generate a keypoint heatmap. The heatmap value represents the probability that a micrograph pixel belongs to a particle center area. CenterPicker can process images of any size and can directly predict the center point and size of the particle. The network implements multiscale feature fusion and introduces an attention mechanism to improve the feature fusion part to obtain more accurate selection results. We have conducted a detailed evaluation of CenterPicker on a range of datasets, and results indicate that it excels in single-particle picking tasks.Keywords
Cite This Article
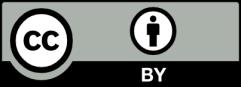
This work is licensed under a Creative Commons Attribution 4.0 International License , which permits unrestricted use, distribution, and reproduction in any medium, provided the original work is properly cited.