Open Access
ARTICLE
A Hybrid Algorithm Based on PSO and GA for Feature Selection
1
Nanjing University of Information Science and Technology, Nanjing, 210044, China
2
Department of Mathematics, Faculty of Science, New Valley University, El-Kharja, 72511, Egypt
3
Jiangsu Key Laboratory of Data Science and Smart Software, Jinling Institute of Technology, Nanjing, 211169, China
* Corresponding Author: Yu Xue. Email:
Journal of Cyber Security 2021, 3(2), 117-124. https://doi.org/10.32604/jcs.2021.017018
Received 21 January 2021; Accepted 14 July 2021; Issue published 02 August 2021
Abstract
One of the main problems of machine learning and data mining is to develop a basic model with a few features, to reduce the algorithms involved in classification’s computational complexity. In this paper, the collection of features has an essential importance in the classification process to be able minimize computational time, which decreases data size and increases the precision and effectiveness of specific machine learning activities. Due to its superiority to conventional optimization methods, several metaheuristics have been used to resolve FS issues. This is why hybrid metaheuristics help increase the search and convergence rate of the critical algorithms. A modern hybrid selection algorithm combining the two algorithms; the genetic algorithm (GA) and the Particle Swarm Optimization (PSO) to enhance search capabilities is developed in this paper. The efficacy of our proposed method is illustrated in a series of simulation phases, using the UCI learning array as a benchmark dataset.Keywords
Cite This Article
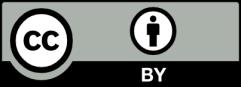
This work is licensed under a Creative Commons Attribution 4.0 International License , which permits unrestricted use, distribution, and reproduction in any medium, provided the original work is properly cited.