Open Access
ARTICLE
A Two-Stage Highly Robust Text Steganalysis Model
1 School of Computer and Software, Nanjing University of Information Science and Technology, Nanjing, 210044, China
2 Guangxi Key Laboratory of Cryptography and Information Security, Guilin, 541004, China
3 College of Information Science and Technology, College of Cyber Security, Jinan University, Guangzhou, 510632, China
* Corresponding Author: Zhangjie Fu. Email:
Journal of Cyber Security 2020, 2(4), 183-190. https://doi.org/10.32604/jcs.2020.015010
Received 02 November 2020; Accepted 10 November 2020; Issue published 07 December 2020
Abstract
With the development of natural language processing, deep learning, and other technologies, text steganography is rapidly developing. However, adversarial attack methods have emerged that gives text steganography the ability to actively spoof steganalysis. If terrorists use the text steganography method to spread terrorist messages, it will greatly disturb social stability. Steganalysis methods, especially those for resisting adversarial attacks, need to be further improved. In this paper, we propose a two-stage highly robust model for text steganalysis. The proposed method analyzes and extracts anomalous features at both intra-sentential and inter-sentential levels. In the first phase, every sentence is first transformed into word vectors. To obtain a high dimensional sentence vector, we use Bi-LSTM to obtain feature information for all words in the sentence while retaining strong correlations. In the second phase, we input multiple sentences vectors into the GNN, from which we extract inter-sentential anomaly features and make a judgment as to whether the text contains secret messages. In addition, to improve the robustness of the model, we add adversarial examples to the training set to improve the robustness and generalization of the steganalysis model. Theoretically, our proposed method is more robust and more accurate in detection compared to existing methods.Keywords
Cite This Article
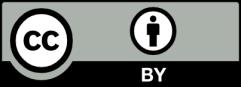