Open Access
ARTICLE
Large-Scale KPI Anomaly Detection Based on Ensemble Learning and Clustering
1 National University of Defense Technology, Changsha, 410000, China
2 Hunan University, Changsha, 410000, China
3 Hunan Agricultural University, Changsha, 410000, China
4 Dreamland Software Development Co., Ltd., Changsha, 410000, China
* Corresponding Author: Fang Liu. Email:
Journal of Cyber Security 2020, 2(4), 157-166. https://doi.org/10.32604/jcs.2020.011169
Received 12 September 2020; Accepted 19 October 2020; Issue published 07 December 2020
Abstract
Anomaly detection using KPI (Key Performance Indicator) is critical for Internet-based services to maintain high service availability. However, given the velocity, volume, and diversified nature of monitoring data, it is difficult to obtain enough labelled data to build an accurate anomaly detection model for using supervised machine leaning methods. In this paper, we propose an automatic and generic transfer learning strategy: Detecting anomalies on a new KPI by using pretrained model on existing selected labelled KPI. Our approach, called KADT (KPI Anomaly Detection based on Transfer Learning), integrates KPI clustering and model pretrained techniques. KPI clustering is used to obtain the similarity of different KPI data's distribution, and applied transfer knowledge from source dataset to the target dataset by model pretrained technique. In our evaluation using real-world KPIs from large Internet-based services, the clustering algorithm used to detect various KPI curve pattern achieve the best classification effect and accuracy More importantly, further evaluation on 30 KPIs shows that KADT can significantly reduce the time overhead of the model training with little loss of accuracy.Keywords
Cite This Article
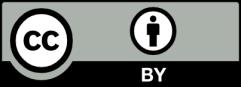
This work is licensed under a Creative Commons Attribution 4.0 International License , which permits unrestricted use, distribution, and reproduction in any medium, provided the original work is properly cited.