Open Access
ARTICLE
Net Effect of Short-Term Smoking Cessation on Mental Health Changes: Inverse Probability of Treatment Weighting and Difference-in-Differences Method
1 Busan Eastern Branch of Korea Association of Health Promotion, Busan, 47734, Republic of Korea
2 Department of Biomedical Health Science, Graduate School, Dong-Eui University, Busan, 47340, Republic of Korea
3 Department of Healthcare Management, Dong-Eui University, Busan, 47340, Republic of Korea
* Corresponding Author: Il-Su Park. Email:
International Journal of Mental Health Promotion 2024, 26(9), 745-755. https://doi.org/10.32604/ijmhp.2024.054630
Received 03 June 2024; Accepted 12 August 2024; Issue published 20 September 2024
Abstract
Background: In response to the need for research clearly demonstrating the net effect of smoking cessation on mental health status, considering the causal relationship between smoking cessation and changes in mental health status, this study was undertaken. Thus, this study aimed to examine the net effect of short-term smoking cessation on five specific mental health metrics and the overall mental health status score. Methods: We used data from the first wave of the Korean Health Panel (KHP) Survey (2011–2013, 2016–2018) and focused on men aged 19 years and older, to explore the effects of smoking cessation. We compared mental health status before and after cessation among current non-smokers who had quit smoking and continuous smokers. We ensured homogeneity between the two groups via inverse probability of treatment weighting (IPTW) and employed a difference-in-differences (DID) analysis to assess changes in mental health status. This study controlled for factors that could influence mental health and explored the net effect of short-term smoking cessation through difference-in-differences model using multiple regression analysis. Results: The results showed that short-term smoking cessation significantly alleviated mental and physical stress (p < 0.1), reduced experiences of frustration (p < 0.01), and improved the overall mental health status score (p < 0.1). Conclusion: Thus, these results highlight a meaningful link between positive health behavior changes, such as smoking cessation, and enhanced mental health. Moreover, the application of temporal variables further improved the understanding of the causal relationship between health behaviors and mental health.Keywords
According to the 2020 Organisation for Economic Co-operation and Development (OECD) Health Statistics, the daily smoking rate among those aged 15 years and older was on a decline annually in most countries. South Korea experienced a significant reduction from 22.9% in 2010 to 16.4% in 2019, which marked a 6.5%p decrease over 10 years. This rate of decline was considerably higher than that in other nations. However, the smoking rate in South Korea remained at the overall average level, which indicated a continuous need for efforts to reduce smoking rates [1]. Numerous studies have reported that smoking increased the risk of premature death owing to various diseases, such as heart attacks, strokes, lung cancer, and laryngeal cancer. Furthermore, increased incidence of cardiovascular diseases and cancer raised mortality and fatality rates nationwide, leading to substantial socio-economic costs [2]. In South Korea, direct smoking resulted in 58,036 deaths in 2019, with the socio-economic cost estimated to be USD 10.5 billion [3]. Thus, smoking is an unhealthy lifestyle habit that incurs high socioeconomic costs and has become an important national health and social issue.
People engage in smoking owing to the strong addictive nature of and habituation to nicotine [4]. Furthermore, they are exposed to physical illnesses and mental health issues, such as stress, depression, and suicide. Nicotine dependency follows a mechanism where nicotine reaches the brain from the pulmonary capillaries within approximately 10–19 s, induces dopamine secretion, and stimulates the brain’s reward circuitry. This process makes smokers feel better and plays a major role in their pursuit of smoking behavior [5]. Tolerance to nicotine can develop in just a day, thereby leading to rapid dependency [6]. Furthermore, the subsequent drop in blood nicotine levels causes tolerance, craving, and withdrawal symptoms, which prompts people to smoke again to eliminate these symptoms [5]. This cycle suggests a significant influence of mental and psychological factors on nicotine addiction.
Recent social changes such as information overload, family dissolution, and a competitive society are posing significant threats to individual stress and other mental health [7]. According to the Ministry of Health and Welfare (MOHW), one in four Korean adults (25.4%) has experienced a mental health issue, such as depression or anxiety disorder, at least once in their lifetime [8]. Therefore, to avoid or alleviate these mental health problems, individuals, especially those in their 30 and 40 s [7], often turn to smoking [9] or drinking as a method of stress relief. The National Institute on Drug Abuse (NIDA) found that the smoking rate among American adults with mental health issues was 28.2% compared to 15.8% among those without in 2019. This indicated that individuals with mental health issues were 1.8 times more likely to smoke [10]. Smokers with mental health problems exhibited higher levels of nicotine addiction and faced difficulties in quitting [11]. A study compared smokers’ and non-smokers’ mental health and revealed that heavy smokers, who smoked more than 20 cigarettes a day, experienced 1.9 and 1.7 times more stress and prolonged feelings of depression, respectively. Furthermore, they were more than twice as likely to have suicidal thoughts than non-smokers [12]. A systematic review and meta-analysis investigated smoking cessation for mental health improvement and suggested that while withdrawal symptoms from quitting smoking were commonly believed to exacerbate anxiety and stress, no clear scientific evidence supported this belief. This study also highlighted the need for further research to overcome the causality issues related to the temporal relationship between quitting smoking and improved mental health [13].
Previous research indicated that smokers generally had poorer mental health than non-smokers. Furthermore, the extent of smoking also influenced this disparity. However, whether the difference in mental health status was solely due to smoking or whether changes in mental health status influence smoking behavior or the amount smoked remains unclear. Research on the causal relationships between these factors is lacking, which indicates the need for further studies.
This study utilized survey data from the Korean Health Panel (KHP), a representative large-scale longitudinal study in the Korean healthcare sector, to generate generalized findings. Furthermore, this study aimed to identify the net effects of short-term smoking cessation on mental health and compared mental health status before and after cessation among current non-smokers who had quit smoking and continuous smokers. This minimized selection bias among smokers via a clear research design that established a temporal cause-and-effect relationship. This study had some specific objectives.
First, this study aimed to understand the changes and differences in mental health over time between current non-smokers and persistent smokers. Therefore, this study employed a difference-in-differences (DID) method to compare the mental health status of current non-smokers before and after quitting smoking with that of persistent smokers at the same time points.
Second, this study aimed to ascertain the net effect of smoking cessation on mental health status through changes induced by cessation. We employed a DID model using multiple regression which was controlled for general characteristics that affected mental health.
This study was exempt from ethical approval from Dong-eui University Institutional Review Board (IRB number: DIRB-202203-HR-W-15). The KIHASA and NHIS obtained informed consent from all participants involved in the KHP. The overall research framework of this study is shown in Fig. 1, and the specific details are as follows.
Figure 1: Conceptual framework.
This study utilized annual data from the Korean Health Panelfor from 2011–2013 and 2016–2018, overseen by the Korea Institute for Health and Social Affairs (KIHASA) and National Health Insurance Service (NHIS). Data from 2014 to 2015 were excluded owing to the absence of mental health metrics. The KHP aims to provide foundational data on healthcare utilization, medical expenditures, and health status and behaviors through an annual survey conducted nationwide since 2008. KHP is a panel survey conducted under professional investigators according to the Computer Assisted Personal Interviewing (CAPI) method. Hence, the data represented a significant longitudinal dataset in the healthcare field. Six years of data from 2011 to 2013 and 2016 to 2018 were vertically integrated (17,035 individuals based on the 2011 data). In addition, the integrated data were restructured so that each case could be considered even if the smoking status of the same individual was different. This was to minimize information loss and enable panel analysis. Consequently, individuals aged <19 years, females, lifetime non-smokers, and those who either did not respond to the mental health-related questions or dropped out were excluded, which totaled 13,852. Hence, data from 3813 participants were analyzed. In particular, the reason for excluding females from this study was that the number of female smokers was very small, and data availability was poor. Subjects were further categorized based on their smoking status in the year preceding the reference year (t − 1) and reference year (t). Each case was analyzed over six years to create a dataset of 7272 subjects. Construction of this dataset allowed the identification of changes over time within the same individuals, which indicated whether they currently had the characteristics of non-smokers or continuous smokers. Table 1 presents the classification of the groups. The smoking status variable was derived from KHP’s current smoking question: “Do you currently smoke?” Individuals who were currently smoking were categorized as “current smokers,” and those who were not were categorized as “current non-smokers.”
Table 2 presents an example of the participant composition used in the panel analysis. If an individual with a specific Personal ID, designated as A, was surveyed from 2011 to 2013, A in 2012 (reference year) and 2013 (reference year) they were the same individual. However, they could exhibit characteristics of continuous smokers (smoked in 2011 → smoked in 2012) and current non-smokers (smoked in 2012 → quit in 2013) and were thus differentiated as separate cases. Consequently, although the total number of individuals was 3183, reorganizing the data to suit the objectives of the panel analysis yielded 7272 cases.
Research variables for the propensity score calculation
This study constructed study and control groups based on smoking status in the reference year. To calculate the propensity score, the probability of belonging to the study group (smoking status = 1) based on baseline characteristics, the variables were organized, as shown in Table 3. Independent variables used to calculate the propensity score were selected from the factors that affected smoking status presented in other studies based on their applicability to the KHP data: age [14], household composition [15–18], economic activity [19,20], and alcohol consumption [21–24]. In particular, the alcohol consumption variable was created using KHP’s question asking about the individuals’ lifetime drinking status: “How often have you drank alcohol in the past year?” If they never consumed alcohol in their lifetime or in one year, I was coded as “non-drink” otherwise they were coded as “drinker”.
Research variables for DID method
Table 4 presents the configuration and measurement of variables for the DID model to discern the net effect of smoking cessation.
Dependent variables for DID method
Dependent variables were selected to represent mental health status, and included five items (mental and physical stress, frustration, unmet basic needs, anxiety regarding the future, and stress due to tasks) and the BEPSI-K score, which was the average score of these five items. All five items were rated on a 5-point Likert scale (that ranged from 1 “not at all” to 5 “always”). Responses that included “other” (6), “do not know/no response” (−9), and “not applicable” (−1) were excluded. To enhance readability, scores from the Korean Health Panel mental health survey items were inverted, which indicated that lower scores represented better mental health states. The Brief Encounter Psychosocial Instrument (BEPSI) was developed by Frank et al. in 1988 [25]. Its Korean version, the BEPSI-K, adapted by Bae et al. [26] in 1996, with only five closed-ended items, aligned with the mental health evaluation domain of the Korean Health Panel. Comprehensive mental health scores were measured via the BEPSI-K scoring method, which involved summing the scores of five items and dividing it by five.
Independent variables for DID method
Independent variables included group and time variables and interaction terms. The group variables comprised the study (current non-smokers = 1) and control groups (persistent smokers = 0). Time variables indicated whether there was a significant difference in mental health between the two groups in the previous year (t − 1) and reference year (t). Effects of smoking cessation could be identified via the interaction of group and time variables.
Control variables for DID method
Control variables were selected based on previous studies as factors that influenced mental health: age groups [7], household composition [27–29], residential area [30,31], level of education [32], household income [33], perceived health status [34], and alcohol consumption [35,36]. Age groups were divided into youth (19–29), middle-aged (30–49), senior (50–64), and older adults (>65 years). Household composition was categorized into “single-person” and “multi-person” households. Residential areas were classified as “metropolitan” and “non-metropolitan.” Level of education was classified as “high school graduate or less” and “college graduate or higher.” Household income was categorized into quintiles from “first” to “fifth.” Perceived health status was classified as “good,” “average,” or “poor.” Alcohol consumption was rated as “yes” or “no.”
Data from the KHP over six years were utilized to analyze how the mental health status of current non-smokers compared to persistent smokers changed from the before reference year via a DID method. To address selection bias and endogeneity, a method combining inverse probability of treatment weighting (IPTW) was employed. Subsequently, a DID model incorporating control variables was conducted to precisely assess the effect of smoking cessation on mental health changes (Fig. 1). All statistical analyses were performed via SAS software (version 9.4; Institute Inc., Cary, NC, USA).
Inverse probability of treatment weighting
To mitigate the issues that arose from selection bias in quasi-experimental designs, various statistical methods were proposed. Of these, the propensity score method stood out. This method included detailed techniques, such as matching, weighting, subclassification, and the recently discussed double propensity score adjustment for causal effect estimation [37]. Propensity scores represented the conditional probability of participants belonging to a study group given the observed variables. In this study, propensity scores and inverse probability weights were calculated using the Psmatch Procedure in SAS, which employed a logistic regression model. Inverse probability of treatment weighting had the advantage of using almost the original sample size and structured data [38]. However, if outliers existed within the study and control groups, some weights may become extremely large, which could potentially impact the analysis results [39]. Thus, in this study, “stabilized weights” were used to prevent an inaccurate estimation of the smoking cessation effect, utilizing the Psmatch Procedure in SAS with ‘weight = atewgt (stabilize = yes)’ [40].
Difference-in-differences (DID) model
The DID method served as an analytical approach to determine the effects of policies or systems and compared the pre-and post-intervention differences between the study and control groups, also considering the chronological changes. This study applied the DID model and incorporated the IPTW into the current non-smoking and persistent smoking groups. We aimed to assess the effect of smoking cessation on changes in mental health status using a DID model, comparing outcomes post-smoking cessation with those who continued smoking. The DID model facilitated the understanding of impact of smoking cessation on mental health as it considered differences across groups and over time.
Even with the minimization of bias between the groups via the DID method, complete elimination of differences in observable characteristics between current nonsmokers and continuous smokers was challenging. Thus, the full verification of causal effects was limited. Therefore, the multiple regression analysis employed the DID introduced control variables that represented individual characteristics and factors that affected mental health. This approach aimed to accurately determine the causal relationship between independent and dependent variables and assess the significant impact of smoking cessation on mental health, controlled for these variables.
Selection of two comparison groups
In this study, 14,529 cases were selected (7257 and 7272 in the study and control groups, respectively). To verify the balance between the current non-smoking and persistent smoking groups before and after the propensity score inverse probability weights were applied, the standardized mean difference (SMD) was calculated. Furthermore, the percentage bias reduction (PBR) was examined.
After the application of inverse probability weighting, the SMDs were all below 0.1, which confirmed a balance between the two groups [41] (Fig. 2). Furthermore, the PBR, which represented the bias reduction rate, showed that all items were above 90%, which indicated that the mean differences in the variables between the groups decreased by more than 90% after weighting. This demonstrated that the two groups were balanced related to factors other than smoking and non-smoking. This essentially eliminated bias in variables that could affect the causal relationship before examining the impact of smoking cessation on mental health status (Table 5).
Figure 2: Standardized mean differences before and after applying IPTW.
Effect of smoking cessation on mental health changes
Table 6 presents the effects of smoking cessation on mental health changes using descriptive statistical analysis, including differences between comparison groups, temporal differences, and differences when both are considered together. The overall mental health status of the current non-smokers improved significantly compared to persistent smokers, except stress due to tasks, with a statistically significant smoking cessation effect observed in frustration experiences (p < 0.05).
We examined the results for experiences of frustration among the five mental health items and found that current non-smokers experienced a decrease in frustration by 0.054 in the negative direction after they quit smoking. Conversely, persistent smokers saw an increase in frustration by 0.002 in the positive direction if they continued smoking. The DID was −0.056, which indicated a greater reduction in frustration experiences after smoking cessation (p < 0.05). Among the five mental health items, three (mental and physical stress, unmet basic needs, and anxiety regarding the future), along with the BEPSI-K scores, showed that both groups experienced a gradual overall improvement in mental health status. However, the DID values were negative, which indicated a more positive change in mental health after smoking cessation than continuing smoking, although these differences were not statistically significant. Conversely, the DID for stress due to tasks increased by 0.014 in a positive direction, which suggested an increase in stress due to tasks after smoking cessation. However, this increase was not statistically significant. Table 7 presents the DID model using the multiple regression analysis. It aimed to discern the net effect of short-term smoking cessation on mental health, controlled for factors—such as general characteristics that influenced mental health—and incorporated a group variable, time variable, and combined interaction variable as independent variables. Statistically significant reductions were observed in the five mental health scores (mental and physical stress, frustration experiences, unmet basic needs, anxiety regarding the future, and stress due to tasks) and average score on the BEPSI-K among the current non-smoking group, compared to the persistent smoking group. This indicated an improved mental health status among the current non-smoking group. Regarding differences between the comparison periods, statistical significance was observed for stress due to tasks, which suggested a significant reduction in task-related stress over time, in both the current non-smoking and persistent smoking groups. When controlling for factors that affected mental health and considering differences in both the group and the passage of time, the analysis of the interaction terms showed a net positive effect of short-term smoking cessation in frustration experiences, as indicated by previous DID results, and mental and physical stress (Coefficient −0.060, p < 0.1), frustration experiences (Coefficient −0.067, p < 0.01), and the BEPSI-K scores (Coefficient −0.040, p < 0.1).
This study aimed to determine the impact of short-term smoking cessation on the mental health status of men. Mental health status was assessed via the BEPSI-K score, which was calculated by averaging the scores of five items: mental and physical stress, frustration experiences, unmet basic needs, anxiety regarding the future, and stress due to tasks. This study utilized data from the first wave of the KHP, employed six years of data, and included a final analysis with 3183 male individuals aged 19 years and older. Furthermore, we excluded lifelong non-smokers and those who did not respond to mental health items (7272 instances). To eliminate selection bias between the groups, IPTW was applied, and 7257 and 7272 instances were selected for the study and control groups, respectively. To analyze changes in mental health due to smoking cessation, the DID method was conducted, which considered both the difference between the groups and before and after smoking cessation.
Descriptive statistical analysis of DID reveal a statistically significant difference in frustration experiences (p < 0.05), which indicated an improvement in mental health status regarding frustration experiences after short-term smoking cessation compared with persistent smoking.
The DID model showed statistically significant differences in the net effects of short-term smoking cessation on mental and physical stress (p < 0.1), frustration experiences (p < 0.01), and the BEPSI-K scores (p < 0.1). These findings were consistent with those of previous studies, which indicated that long-term smoking cessation reduced stress, depression, and anxiety and positively impacted the mental health of those who quit smoking compared with those who continued smoking [13,22]. Moreover, compared with non-smokers, smokers were more sensitive to stress due to their lack of emotional regulation skills, which led to a higher incidence of stress-induced headaches, anxiety, tension, and fatigue [42]. Considering the smoking-related indicators from the regional health statistics in 2022 [43], which showed a smoking cessation attempt rate of 40.9% within the last year, continuous smokers could feel frustrated due to failed cessation attempts. Conversely, stress related to tasks increased after cessation, a result that did not consider direct factors of job stress, such as job, organizational, and environmental factors, according to the National Institute for Occupational Safety and Health (NIOSH), which suggested that the work environment and organizational atmosphere could influence task-related stress.
Nevertheless, this study has some limitations. First, even if a smoker successfully quit smoking, maintaining lifelong cessation is challenging. Previous research [44] indicated that the highest relapse rate within less than six months after cessation was 66.7%. Since the study design defined current cessation based on a short period that ranged from several days to a maximum of one year, it was difficult to understand the impact of long-term cessation on mental health changes. Hence, further research on the effects of long-term cessation is warranted. Second, the study did not consider the daily average smoking amount during the smoking period or other factors, such as nicotine dependence, that could affect cessation [45,46]. Hence, future research should consider nicotine dependence and its impact on mental health changes due to cessation. In addition, when a direct measurement of nicotine dependence is not available, using the smoking amount such as “Pack-years” as a proxy variable may be a good alternative. Third, this study was limited to males. The reason for this is that the number of female smokers who participated in the KHP and those selected according to the design of this study were not sufficient to achieve the study’s purpose. Thus, only males were included to present the study results more clearly.
Despite these limitations, these findings were significant in identifying the net effect of smoking cessation on mental health changes through a regression analysis via inverse probability weighting and double differences, which reduced selection bias between the groups. The results demonstrated that cessation positively affected mental health changes, regardless of specific situations. This was a significant contribution to overcoming the limitations of previous research on the relationship and causality between smoking cessation and mental health.
Acknowledgement: This article was based on the first author’s doctoral dissertation at Dong-eui University in South Korea. Furthermore, we appreciate the administrative support from the Korea Association of Health Promotion.
Funding Statement: The authors received no specific funding for this study.
Author Contributions: The authors confirm contribution to the paper as follows: study conception and design: Il-Su Park, Ji-Su Park; data collection: Ji-Su Park, Tae-Hyeon Lee; methodology: Il-Su Park, Ji-Su Park, Tae-Hyeon Lee; analysis and interpretation of results: Il-Su Park, Ji-Su Park, Tae-Hyeon Lee; writing-review and editing: Il-Su Park, Ji-Su Park. All authors reviewed the results and approved the final version of the manuscript.
Availability of Data and Materials: Data are available on the official KHP website (https://www.khp.re.kr:444/web/data/data.do, accessed on 15 July 2024).
Ethics Approval: Only de-identified data from the Korean Health Panel (KHP) were provided to the researchers by the Korea Institute for Health and Social Affairs (KIHASA) and National Health Insurance Service (NHIS) in compliance with the Personal Information Protection Act and Statistical Act to protect the identity of the participants. And this study was exempt from ethical approval from Dong-Eui University Institutional Review Board (IRB number: DIRB-202203-HR-W-15). The KIHASA and NHIS obtained informed consent from all participants involved in the KHP.
Conflicts of Interest: The authors declare that they have no conflicts of interest to report regarding the present study.
References
1. Ministry of Health and Welfare. OECD Health Statistics 2022. Available from: https://www.oecd-ilibrary.org/social-issues-migration-health/data/oecd-health-statistics_health-data-en. [Accessed 2023]. [Google Scholar]
2. Lee EH, Park SK, Ko KP, Cho HI, Chang SH, Shin HR, et al. Cigarette smoking and mortality in the Korean Multi-Center Cancer Cohort (KMCC) study. J Prev Med Public Health. 2010;43(2):151–8. doi:10.3961/jpmph.2010.43.2.151. [Google Scholar] [PubMed] [CrossRef]
3. Korea Disease Control and Prevention Agency. Study on attributable mortality and socioeconomic burden of smoking and second-hand smoking in South Korea. Available from: https://www.prism.go.kr/homepage/entire/researchDetail.do?researchId=1351000-201900110&menuNo=I0000002. [Accessed 2023]. [Google Scholar]
4. Kim HM, Park KS. Effect of smoking type, smoking cessation-related psychological factors and nicotine dependence on smoking cessation in working female smokers. J Health Info Stat. 2022;47(2):111–7. doi:10.21032/jhis.2022.47.2.111. [Google Scholar] [CrossRef]
5. Korea Disease Control and Prevention Agency. Trends in smoking rates and determinants of smoking initiation. Available from: https://www.kdca.go.kr/contents.es?mid=a20205010601. [Accessed 2023]. [Google Scholar]
6. Moon JH, Linton JA, Choi J, Kim J, Lee J, Jo Y. Correlation between smoking and depression in Korean adult group: the Korean national health and nutrition examination survey (2014). Korean J Fam Pract. 2019;9(4):383–8. doi:10.21215/kjfp.2019.9.4.383. [Google Scholar] [CrossRef]
7. Yun HS. The relationships between smoking and mental health among Korean by age group. Korean J Health Educ Promot. 2018;35(1):41–54. doi:10.14367/kjhep.2018.35.1.41. [Google Scholar] [CrossRef]
8. Ministry of Health and Welfare. The survey of mental disorders in Korea. Available from: https://www.mohw.go.kr/board.es?mid=a10107010100&bid=0046&act=view&list_no=357650&tag=&cg_code=&list_depth=1. [Accessed 2023]. [Google Scholar]
9. Kang HC. A study on the relationship with nicotine dependence and mental health-focusing on SCL-90-R (Symptom Checklist-90-Revision). J Oriental Neuropsych. 2014;25(1):55–62. doi:10.7231/jon.2014.25.1.055. [Google Scholar] [CrossRef]
10. National Institute on Drug Abuse (NIDA). Do people with mental illness and substance use disorders use tobacco more often? Tobacco, Nicotine, and E-Cigarettes Research Report. Available from: https://nida.nih.gov/publications/research-reports/tobacco-nicotine-e-cigarettes/do-people-mental-illness-substance-use-disorders-use-tobacco-more-often. [Accessed 2023]. [Google Scholar]
11. Joe K, Lee K, Kim D. Smoking and smoking cessation in the patients with major psychiatric disorders. J Korean Soc Res Nicotine Tob. 2010;1:96–106. doi:10.25055/JKSRNT.2010.1.2.96. [Google Scholar] [CrossRef]
12. Kwon HJ, Kim YJ, Kim HS. The association between smoking amount and mental health. J Korean Soc Wellness. 2016;11(3):297–305. doi:10.21097/ksw.2016.08.11.3.297. [Google Scholar] [CrossRef]
13. Taylor GM, Lindson N, Farley A, Leinberger-Jabari A, Sawyer K, Te Water Naudé R, et al. Smoking cessation for improving mental health. Cochrane Database Syst Rev. 2021;3(3):CD013522. doi:10.1002/14651858.CD013522.pub2. [Google Scholar] [PubMed] [CrossRef]
14. Brown-Rice KA, Furr S, Jorgensen M. Analyzing Greek members alcohol consumption by gender and the impact of alcohol education interventions. J Alcohol Drug Educ. 2015;59(1):19–38. [Google Scholar]
15. Davis MA, Moritz DJ, Neuhaus JM, Barclay JD, Gee L. Living arrangements, changes in living arrangements, and survival among community dwelling older adults. Am J Public Health. 1997;87(3):371–77. doi:10.2105/AJPH.87.3.371. [Google Scholar] [PubMed] [CrossRef]
16. Umberson D. Relationships between adult children and their parents: psychological consequences for both generations. J Marriage Fam. 1992;54(3):664–74. doi:10.2307/353252. [Google Scholar] [CrossRef]
17. Kim JG. The impact of family type on health behavior of elderly people. J Welf Aged. 2011;51:35–56. doi:10.21194/kjgsw.51.201103.35. [Google Scholar] [CrossRef]
18. Lee YS. Marital status, health behaviors and health status for middle-aged men and women in Korea. Korea J Popul Stud. 2012;35(2):103–31. [Google Scholar]
19. Augustson M, Marcus S. Use of the current population survey to characterize subpopulations of continued smokers: a national perspective on the hardcore smoker phenomenon. Nicotine Tob Res. 2004;6(4):621–29. doi:10.1080/14622200410001727876. [Google Scholar] [PubMed] [CrossRef]
20. Ferketich AK, Gallus S, Colombo P, Pacifici R, Zuccaro P, La Vecchia C. Hardcore smoking among Italian men and women. Eur J Cancer Prev. 2009;18(2):100–5. doi:10.1097/CEJ.0b013e32830c8d98. [Google Scholar] [PubMed] [CrossRef]
21. Kim KA. A study on the factors related to smoking and smoking conditions among college students in some area. J Korea Acad-Ind Coop Soc. 2016;17(8):465–76. doi:10.5762/KAIS.2016.17.8.465. [Google Scholar] [CrossRef]
22. Kim MK, Shin KA. A study of the smoking and drinking behaviors among university and college students. J Korean Soc Integr Med. 2015;3(4):23–7. doi:10.15268/ksim.2015.3.4.023. [Google Scholar] [CrossRef]
23. Yang KM, Park CS, Jang JH. Smoking, alcohol consumption and dietary behavior of college students in the Kyungsan area. J Korean Home Econ Assoc. 2004;42(6):55–72. [Google Scholar]
24. Little HJ. Behavioral mechanisms underlying the link between smoking and drinking. Alcohol Res Health. 2000;24(4):215–24. [Google Scholar] [PubMed]
25. Frank SH, Zyzanski SJ. Stress in the clinical setting: the brief encounter psychosocial instrument. J Fam Pract. 1988;26(5):533–39. [Google Scholar] [PubMed]
26. Bae JM, Jeong EK, Yoo TW, Huh BY, Kim CH. A quick measurement of stress in outpatient clinic setting. J Korean Acad Fam Med. 1992;13:809–20. [Google Scholar]
27. Ansseau M, Fischler B, Dierick M, Albert A, Leyman S, Mignon A. Socioeconomic correlates of generalized anxiety disorder and major depression in primary care: the GADIS II study (Generalized Anxiety and Depression Impact Survey II). Depress Anxiety. 2008;25(6):506–13. doi:10.1002/(ISSN)1520-6394. [Google Scholar] [CrossRef]
28. Park E. A study on social support and depression by gender among adults. Korean J Women Health Nurs. 2011;17(2):169–77. doi:10.4069/kjwhn.2011.17.2.169. [Google Scholar] [PubMed] [CrossRef]
29. Sung JM. Effects of family and household’s economic factors on depression: panel regression analysis using fixed-effect model. J Soc Sci. 2013;24(2):207–28. doi:10.16881/jss.2013.04.24.2.207. [Google Scholar] [CrossRef]
30. Chun JD. Comparisons of health status and health behaviors among the elderly in urban and rural areas (Master’s Thesis). Chosun University: South Korea; 2011. doi: 10.5393/JAMCH.2013.38.3.182. [Google Scholar] [CrossRef]
31. Choi YH, Chae JH. A study on relationship between level of cigarette consumption, eating and mental-psychological state among adolescents: based on 2013 Korea Youth Risk Behavior Survey. J Korea Acad-Ind Coop Soc. 2015;16(11):7321–8. doi:10.5762/KAIS.2015.16.11.7321. [Google Scholar] [CrossRef]
32. Kim JS, Shin KR. A study on depression, stress, and social support in adult women. Taehan Kanho Hakhoe Chi. 2004;34(2):352–61. doi:10.4040/jkan.2004.34.2.352. [Google Scholar] [PubMed] [CrossRef]
33. Kahng SK, Kwon TY. A study on the relationship between depressive symptoms and alcohol use-focusing on “Tension Reduction Theory” and “Intoxication Theory”. Korean Assoc Soc Welf Stud. 2008;36:253–80. doi:10.16999/kasws.2008.36.253. [Google Scholar] [CrossRef]
34. Hwang BD, Kim YJ. The relevance of self-rated health level and mental health. Korean Soc Heal Serv Manage. 2022;16(3):51–62. doi:10.12811/kshsm.2022.16.3.051. [Google Scholar] [CrossRef]
35. Lee YC, Im BH. Associated factors of problem drinking grade and comparison of health behavior by drinking grade among Korean men and women. Korean Soc Alcohol Sci Heal Behav. 2010;11(2):107–23. [Google Scholar]
36. Ryu JS, Kang KH, Lee JH. Factors of problem drinking among Korean adults. Korean Soc Alcohol Sci Heal Behav. 2011;12(1):29–42. [Google Scholar]
37. Jang HJ, Jung H, Min KS. Comparison of propensity score methods for causal inference: matching, weighting, subclassification, and double propensity score adjustment. J Curric Eval. 2019;22(2):269–91. doi:10.29221/jce.2019.22.2.269. [Google Scholar] [CrossRef]
38. Seo TW, Lee JY. A comparison of propensity weight trimming and winsorizing methods in propensity score weighting analysis. In: Proceeding of Korean Statistical Society Spring Conference 2013, 2013 May 24–25; Incheon, South Korea; Korean Stat Soc; p. 77. [Google Scholar]
39. Lee JS. Methods for controlling confounders using propensity score in mediation analysis. Korean Surv Res Soc. 2020;21(3):61–86. doi:10.20997/SR.21.3.3. [Google Scholar] [CrossRef]
40. Robins JM, Hernan MA, Brumback B. Marginal structural models and causal inference in epidemiology. Epidemiology. 2000;11(5):550–60. doi:10.1097/00001648-200009000-00011. [Google Scholar] [PubMed] [CrossRef]
41. Jeon SY, Yang JY, Lee HS. Statistical mistakes commonly made when writing medical articles. J Korean Soc Radiol. 2023;84(4):866–78. doi:10.3348/jksr.2022.0108. [Google Scholar] [PubMed] [CrossRef]
42. al’Absi M, Nakajima M, Grabowski J. Stress response dysregulation and stress-induced analgesia in nicotine dependent men and woman. Biol Psychol. 2013;93(1):1–8. doi:10.1016/j.biopsycho.2012.12.007. [Google Scholar] [PubMed] [CrossRef]
43. Korea Disease Control and Prevention Agency. Community health statistics at a glance; 2022. Available from: https://chs.kdca.go.kr/chs/stats/statsMain.do. [Accessed 2023]. [Google Scholar]
44. Kim HS, Bae SS. Factors associated with relapse to smoking behavior using health belief model. J Agr Med Commun Heal. 2011;36(2):87–100. doi:10.5393/JAMCH.2011.36.2.087. [Google Scholar] [CrossRef]
45. Lee ES, Seo HG. The factors associated with successful smoking cessation in Korea. Korean J Fam Med. 2007;28(1):39–44. [Google Scholar]
46. Eum YH, Kim HJ, Bak S, Lee SH, Kim J, Park SH, et al. Factors related to the success of smoking cessation: a retrospective cohort study in Korea. Tob Induc Dis. 2022;20:15. doi:10.18332/tid/144272. [Google Scholar] [PubMed] [CrossRef]
Cite This Article
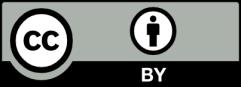
This work is licensed under a Creative Commons Attribution 4.0 International License , which permits unrestricted use, distribution, and reproduction in any medium, provided the original work is properly cited.