Open Access
ARTICLE
Associations between Social Media Use and Sleep Quality in China: Exploring the Mediating Role of Social Media Addiction
1 School of Journalism and Communication, Nanjing Normal University, Nanjing, 210097, China
2 School of Journalism and Communication, Jinan University, Guangzhou, 510632, China
* Corresponding Author: Hao Gao. Email:
(This article belongs to the Special Issue: Social Stress, Adversity, and Mental Health in Transitional China)
International Journal of Mental Health Promotion 2024, 26(5), 361-376. https://doi.org/10.32604/ijmhp.2024.049606
Received 12 January 2024; Accepted 22 March 2024; Issue published 30 May 2024
Abstract
Sleep quality is closely linked to people’s health, and during the COVID-19 pandemic, the sleep patterns of residents in China were notably poor. The lockdown in China led to an increase in social media use, prompting questions about its impact on sleep. Therefore, this study investigates the association between social media use and sleep quality among Chinese residents during the COVID-19 outbreak, highlighting the potential mediating role of social media addiction. Data were collected via questionnaires through a cross-sectional survey with 779 valid responses. Variance analysis was used to test for differences in social media use among different demographic variables. Bivariate correlation analysis was employed to explore the relationships between variables, while regression analysis investigated the correlations between various media factors and sleep quality. Additionally, Bootstrap sampling was utilized to analyze the potential mediating influence of social media addiction in the relationship between social media use and sleep. The study's findings reveal a significant correlation between social media use, particularly before bedtime, and sleep quality (p < 0.01), with pre-sleep activity notably linked to poorer overall sleep scores (β = 0.141, p = 0.004). Although the daily use of social media did not directly impact most individuals’ sleep quality, specific platforms like news apps, short video apps, dating apps, and content community platforms were associated with higher levels of social media addiction, subsequently negatively affecting sleep quality. Specifically, the use of news apps (B = 0.068, 95% CI [0.000, 0.019]), short video apps (B = 0.112, 95% CI [0.001, 0.031]), dating apps (B = 0.147, 95% CI [0.000, 0.028]), and content community platforms (B = 0.106, 95% CI [0.001, 0.028]) was found to increase the risk of social media addiction, subsequently leading to adverse effects on sleep quality. The study underscores a notable link between social media use and sleep quality, suggesting that mindful social media habits, particularly before bedtime, and reducing addiction-associated apps could enhance sleep quality.Keywords
Good sleep quality is widely recognized as a predictor of physical and mental health, happiness, and overall vitality [1]. However, in today's fast-paced society, insufficient sleep is a common and pressing health issue [2], with insomnia being the most prevalent sleep disorder, imposing a significant burden on sufferers [3]. Studies have shown that insomnia is related to subjective well-being [4] and has a causal relationship with diseases such as depression [5]. However, research indicates that sleep problems were widespread during the COVID-19 pandemic [6,7], affecting about 40% of the general population and healthcare workers, with an even higher incidence rate among COVID-19 patients [8]. Sleep directly affects immune maintenance and response, and a decline in sleep quality can impair the immune system [9]. The immune system is closely related to the progression of viral infections [10], forming a vicious cycle. Therefore, paying attention to the factors related to poor sleep quality during the COVID-19 pandemic is necessary.
Studies indicate that there is a correlation between the use of social media and sleep quality [11,12]. Irregular use of social media has been found to affect sleep quality [13], while reducing the use of social media to a certain extent may improve sleep quality and increase individual happiness [14]. Notably, during the COVID-19 pandemic, social media users significantly increased [15], and people increasingly used social media to pass the time [16]. Some studies have pointed out that residents’ sleep patterns changed during this period, with bedtime and wake-up times being delayed, nighttime sleep duration decreasing, and daytime napping time increasing, leading to an overall decline in sleep quality [17]. The government implemented strict lockdown measures in China to combat the pandemic, further promoting social media use [18]. The “2022 China Sleep Quality Report” also showed that unhealthy sleep concepts and behaviors are shared among the Chinese population [19]. Therefore, although the COVID-19 pandemic can be seen as a particular period that exacerbates existing social trends, it also reveals a broader phenomenon—the relationship between social media use and sleep quality. This finding prompts us to explore the potential connection between the two further. Based on this, the study poses the research question: How is the use of social media related to the sleep quality of the Chinese population?
Specifically, the use of social media is a complex phenomenon involving multiple dimensions, including the frequency of use, duration, and the social media platforms preferred by users [20]. Existing research reveals that social media use periods (such as weekdays and weekends) have varying degrees of impact on sleep quality [12]. In particular, the habit of using social media before bedtime has received widespread attention, with studies indicating that individuals who frequently use social media before bed are more likely to suffer from a decline in sleep quality [21]. For instance, nighttime social media use may lead to pre-sleep anxiety or depression [22], and the blue light from social media use before bed may also disrupt the sleep cycle [23]. Based on this, we propose Research Hypothesis 1: The use of social media before bedtime is related to the sleep quality of the Chinese population.
Although existing research has explored the relationship between specific types of social media platforms and sleep quality, these studies have primarily focused on specific, widely used social media platforms, such as Facebook [24], Instagram, and WhatsApp [25], and often have not fully considered the diversity of social media and its potential differential impacts on sleep. Additionally, previous studies have mostly adopted a macro perspective [14,26] without delving into the specific effects of different types of social media applications—such as news and information, short videos, dating apps, and content community platforms—on sleep quality. Given this, this study aims to refine the classification of social media further to more accurately explore the relationship between the use of different types of social media and sleep quality. Based on this, the study proposes Research Hypothesis 2: The use of different types of social media is related to the sleep quality of the Chinese population.
The use of social media can also lead to social media addiction [27]. Social media addiction is defined as a loss of control over social media use, with individuals heavily relying on social media for gratification or to escape reality [28]. Social media addiction not only poses a threat to individuals’ psychological health but also affects sleep quality [29,30]. Based on these observations, we further hypothesize that social media addiction, as an extreme form of excessive social media use, may play a mediating role, forming a bridge between social media use and sleep quality. Specifically, excessive use of social media may first induce addictive behaviors, which then lead to more frequent and later use times, ultimately affecting sleep quality. Based on this, this study proposes Research Hypothesis 3: Social media addiction mediates the relationship between social media use and sleep quality (Fig. 1).
Figure 1: Simplified mediation model diagram for social media use, addiction, and sleep quality.
Given the high prevalence of social media in China and its central role in daily life, this study aims to delve into the potential link between social media use and sleep quality, focusing on social media addiction as a possible mediating variable. Although existing literature has already made preliminary explorations into the broad impacts of social media use, in-depth studies on the relationship between social media use and sleep quality within China’s specific cultural and societal context remain relatively scarce. By systematically examining and analyzing the interactions between social media usage habits, addictive behaviors, and sleep quality, this study hopes to contribute new theoretical and empirical data to the academic discussion in this field, providing a theoretical basis for public health and mental health professionals to improve sleep quality and develop targeted intervention strategies.
This study conducted a survey based on the population structure from China’s Seventh National Population Census, with the survey taking place from December 01 to December 15, 2021. Data from 812 Chinese citizens were collected online through the Tencent Questionnaire platform (https://wj.qq.com/index.html). Tencent Questionnaire is a professional survey service company that provides targeted quantitative questionnaire distribution and collection services for various organizations, including consulting/research institutions, enterprises, universities, and government entities. Its database contains over 3 million verified personal records, enabling precise, efficient, and low-cost survey research. Additionally, existing academic research has proven the reliability and effectiveness of the Tencent Questionnaire in data collection, further strengthening its application value in the academic and research fields [31–33].
In the preliminary phase of this survey, this study has been performed in accordance with the principles stated in the Declaration of Helsinki and has received ethical approval from the Biomedical Research Ethics Committee of Nanjing Normal University (IRB Number: NNU2022060054). All participants signed the informed consent in this study. After excluding participants who did not complete the questionnaire, data from 779 participants were ultimately selected for subsequent analysis. To ensure the scientific rigor of data collection and the validity of the sample, we employed strict methodological measures to ensure the sample’s accuracy and data quality. By conducting statistical tests on these collected data, we further validated the representativeness of the sample and the reliability of the obtained data, which collectively ensured the validity and scientific integrity of the research findings.
Variable selection
Independent variables
Social media use
Social media use is defined as the specific consumption of digital media or the Internet. It allows the audience to connect and interact with each other and their familiar friends through real-time communication or social media websites [34]. In this study, frequency of media use refers to the frequency of social media use. Previous studies have evaluated two dimensions of social media use: time and frequency [35]. Due to the self-assessment survey method adopted by this questionnaire, it is difficult for each participant to answer their social media use time. Therefore, this study uses the participants’ self-rated social media use frequency as their social media use level. In the option setting, this study uses the Likert seven-point scale, from 1 (never) to 7 (always), to measure the participant’s frequency of using social media. At the same time, this study further subdivides social media use from the use time and application type.
(1) Period
This study divides the frequency of social media use into two parts based on the period: daytime social media use frequency and pre-sleep media use frequency. Specifically, because this study has differentiated various types of social media, respondents may have different preferences for social media. The sum or average of the frequency of use of mixed social media may not represent the respondent’s actual use frequency. Therefore, this study takes the highest value of daytime and pre-sleep social media use frequency in the questionnaire as the analysis data.
(2) APP type
In terms of social media type, this study selected six of the most mainstream social media platforms in China: a. Instant messaging app (WeChat, QQ); b. News app (Toutiao); c. Short videos app (Douyin,Kuaishou); d. Dating app (Momo, Tantan, Tinder); e. Content community platform (Weibo, Douban, Zhihu, Little Red Book); f. Consumer review website (Dianping). Considering the differences in users’ media usage preferences in the data processing, the data with the highest frequency of use in each category was selected as the analysis data. Additionally, based on the differentiation of software type, this study further differentiates the frequency of use into daytime and pre-sleep use frequency.
Social media addiction can be seen as Internet addiction [36], characterized by excessive and irrational use of social media, and impacts the addicts’ daily lives [35,37]. This study uses a set of items adapted from The Bergen Social Media Addiction Scale (BSMAS) [38] to assess the level of social media addiction among the respondents. Over the past few years, the psychometric properties of the BSMAS have been investigated in multiple areas, including Hong Kong and Taiwan [39], and have shown good reliability and validity [40]. To further substantiate the structural validity of the BSMAS in our study, a CFA was conducted. The CFA model fit indices for the BSMAS demonstrated satisfactory results, with χ2/df = 2.454, CFI = 0.953, TLI = 0.954, and RMSEA = 0.098. Each item in the scale represents one of the six core elements of addiction (significance, emotional regulation, tolerance, withdrawal, conflict, and relapse [41]). The Likert seven-point scale, from 1 (strongly disagree) to 7 (strongly agree), is used for the option settings, with a higher score indicating a higher likelihood of social media addiction. In the statistical process, this study uses the average score of six questions to represent each participant’s problematic social media use. The internal consistency of the social media addiction scale is good (α = 0.776).
In a study on sleep quality, the Pittsburgh Sleep Quality Index (PSQI) developed by Dr. Buysse and others at the University of Pittsburgh in 1989 was chosen to measure the participants’ sleep quality over the past month [42]. The PSQI includes seven measurement indicators with 19 specific measurement items to assess sleep quality and interference factors, scoring each item 0–3 and standardizing the scores to get the total sleep quality score, which ranges from 1 to 19. An evaluation level can be assigned based on the score [43], with 0–5 being very good, 6–10 being good, 11–15 being average, and 16–21 being poor. The CFA results yielded the following model fit indices: χ2/df = 2.362, CFI = 0.91, TLI = 0.92, RMSEA = 0.098, further supporting the reliability and validity of the PSQI for assessing sleep quality in our sample. The internal consistency of PSQI in this study was good (α = 0.785).
In previous empirical studies, demographic variables such as gender [44,45], age [46], education level [47], monthly income [48], and location [49] are associated with sleep quality. Thus, in this study, these demographic variables are set as control variables (Suppl. Table 1).
According to the calculations in G* Power 3.1 [50], a minimum sample size of 115 would be required to draw a statistical conclusion from a linear multiple regression analysis based on the number of predictors, with a significance level of 0.05, a power of 0.95, and an effect size of 0.15 as referenced from the previous study [51]. Variance analysis was used to test for differences in social media use among different demographic variables. Bivariate correlation was used to explore relationships between variables, regression analysis was used to examine the effects of various media factors on sleep quality, and Bootstrap sampling was used to analyze the mediating role of social media addiction between social media use and sleep quality. IBM SPSS Statistics 26.0 was used to process all encoded data, and the statistical significance level was set at p < 0.05.
Descriptive statistical analysis
This study sampled based on the population structure of the 7th population census in China and distributed the questionnaire. Participants came from 31 provinces, autonomous regions, and municipalities directly under the central government in Mainland China. Finally, 779 valid samples were obtained, and the sample’s demographic characteristics are shown in Suppl. Table 1.
The participants’ final PSQI score was calculated by summing each sub-item of PSQI and rating the participants based on their scores. Regarding PSQI ratings, the highest proportion of participants was rated as good, accounting for 65.1%. The next was general, accounting for 18.7%. The proportion of participants rated as very good or poor was relatively small, accounting for 15% and 1.2%, respectively (Suppl. Table 2).
From a gender and urban-rural area perspective (Suppl. Tables 3 and 4), the variance results show significant differences (p < 0.01) in PSQI scores between genders regarding sleep quality, onset time, and final sleep time score. Significant differences (p < 0.01) exist in the final sleep quality scores and daytime dysfunction in urban-rural areas. Regarding social media use, there are substantial differences (p < 0.01) between different genders in the daytime and pre-sleep content community and consumer review type of social media use. Significant differences exist (p < 0.01) in the daytime and pre-sleep use of social media, daytime and pre-sleep use of instant messaging apps, daytime and pre-sleep use of news apps, and pre-sleep content community platform use when comparing urban and rural areas. Using paired t-test to study the differences in the data, as seen from Suppl. Table 5, a total of 6 pairs all show differences (p < 0.05).
Bivariate correlation analysis
The results of the bivariate correlation analysis between social media use time, types of social media use, and factors affecting sleep quality are presented in Suppl. Tables 6–8. Age, income, pre-sleep social media use, and social media addiction were significantly related to PSQI (Pittsburgh Sleep Quality Index). There was a significant relationship between the daytime use of the news apps and PSQI scores; pre-sleep use of instant messaging apps and news apps was significantly related to PSQI scores.
Multilevel regression analysis
The results of the multilevel regression analysis show that the F-value changed significantly (p < 0.05) after adding the variables of social media addiction, daytime social media use, and pre-sleep social media use in model 1 (Suppl. Table 9). It means that the variables have explanatory significance for the model. The findings from Suppl.Table 10 reveal a significant positive correlation between pre-sleep social media use and the PSQI score (t = 2.877, p = 0.004).
After adding social media addiction, daytime use of instant messaging app, daytime use of news app, daytime use of short videos app, daytime use of dating app, daytime use of content community platform, and daytime use of consumer reviews website to Model 1 (Suppl. Table 10), the F-value change did not show significance (detF = 1.710, detP = 0.103). It indicates that adding these variables to the model did not have an explanatory meaning.
Based on Model 1, this study added social media addiction, pre-sleep use of instant messaging apps, pre-sleep use of news apps, pre-sleep use of short video apps, pre-sleep use of dating apps, pre-sleep use of content community platforms, and pre-sleep use of consumer reviews website (Suppl. Table 11). The change in F-value did not show significance (detF = 1.929, detP = 0.062), meaning that adding the variables did not have explanatory significance for the model.
To further explore the relationship between media use, media addiction, and sleep quality, this study identifies media addiction as a potential mediator in the impact of media use on sleep quality. This study adopts the product coefficient method to test the mediating effect. As the statistical efficiency of the product coefficient method is superior to the causal step method [40], the coefficient product method is increasingly used by more and more researchers. The coefficient product method is divided into two categories, one based on the normal distribution of the mediating effect’s sampling distribution, the Sobel test method, and the other based on the non-normal distribution of the mediating effect’s sampling distribution, the asymmetric confidence interval method, which includes the Bootstrap method and the distribution of the Product. Mackinnon et al. found through simulation studies that the Bootstrap method had the highest statistical efficiency in mediating effect analysis [52]. Therefore, this study uses the Bootstrap sampling method to test significance.
Suppl. Table 12 displays the models involved in mediating effect analysis, mainly including the regression model of independent variables to mediating variables (Model 2) and the regression model of independent variables to dependent variables (when there is no mediating variable, see Model 1).
Suppl. Table 12 reveals a full mediation effect between social media addiction and the relationship between daytime use of news apps, short video apps, dating apps, content community platforms, and sleep quality. This suggests that while daytime media use does not directly correlate with sleep quality in the general population, the use of news apps, short videos, dating apps, and content community platforms is associated with sleep quality through the mediation of social media addiction. In essence, daytime engagement with these apps is associated with an increased likelihood of addiction, which in turn is related to sleep quality (Fig. 2).
Figure 2: Mediation effect model of the relationship between social media use, addiction, and sleep quality (Note: **p < 0.01).
This study investigated the correlation between social media use and sleep quality and explored the mediating role of social media addiction, revealing three key findings.
Firstly, we found a significant correlation between pre-sleep social media use and poorer sleep quality. It indicates that individuals who use social media more before bedtime have higher sleep quality scores, reflecting poorer sleep quality. It suggests frequent exposure to social media before bedtime may disrupt standard sleep patterns. This result is consistent with previous research findings [53,54]. It may be because the use of social media before sleep can directly affect users’ sleep through emotional stimulation. Content on social media, such as emotionally charged messages and affective communication, may trigger psychological stimulation in users, activating emotional, cognitive, or physiological responses [55], mainly when used before bedtime, it is more likely to lead to emotional or cognitive arousal, which in turn can cause insomnia [56]. During the COVID-19 pandemic, the frequency and intensity of such affective communication may have increased, and the plethora of negative information on social media may further induce users’ negative emotions, affecting sleep quality [57,58]. Additionally, social media use may lead to sleep procrastination and interruptions. Due to the constant interactivity of social media, users may wait for social interactions when they should be sleeping [59], leading to delayed bedtime and disruption of sleep rhythms [60]. Meanwhile, notifications from social media at night may disturb sleep, causing interruptions [61] further affecting sleep quality [62,63]. However, it is noteworthy that the research data revealed no direct association between daytime social media use and the overall sleep quality score. Previous studies often did not differentiate the time of day of social media use, typically treating it as a whole [64] or primarily focusing on nighttime and pre-sleep usage [65,66]. This study differentiated between social media use during the day and at night, establishing a clear link between the use of social media before bedtime and the quality of sleep. However, it did not find a similar correlation with social media use during daytime hours. This distinction may reflect differences in the impact of daytime and nighttime social media use on individuals. Daytime social media use may not affect sleep quality due to its lesser impact on individuals’ physiological and psychological states. In contrast, nighttime use may involve more emotional content and social interaction, which may more easily provoke psychological activity at night and significantly correlate with sleep quality [67,68]. It suggests that considering the specific context of usage time when exploring the relationship between social media use and sleep quality may help to more accurately understand their interaction and provide a basis for strategies to promote good sleep quality.
Secondly, there is a significant relationship between daytime use of news apps and sleep quality (PSQI score); simultaneously, using real-time communication apps and news apps before bedtime significantly correlates with sleep quality scores. This indicates that various social media applications may be linked to sleep quality through different mechanisms. Previous studies only analyzed a few of the most popular social media platforms. For example, frequent use of Facebook may lead to addiction among college students, negatively affecting their daily lives, health, and sleep quality [69,70]. Although these studies provide valuable insights into the psychosocial impact of social media use, the specificity of their analyses may limit the broad applicability of their results. These findings may not be applicable, particularly in a socio-cultural context like China, where western social media like Facebook are less prevalent. Furthermore, focusing research on a single social media platform may not fully reveal the comprehensive impact of different social media functions on users’ health and sleep quality. Platforms like Facebook encompass a wide range of functions, including real-time messaging, social networking, etc., and these different functions may have varying impacts on users. Our study adopted a segmented approach by classifying social media based on primary functions to explore the association between different functional categories of social media use and sleep quality. Specifically, daytime use of news apps may lead to information overload [71] and an increased state of psychological arousal [72] due to their rapid update of a vast amount of information and negative news that can trigger emotional responses, affecting the preparation for sleep at night and possibly leading to difficulty falling asleep. Using news apps before bedtime may directly stimulate strong emotional reactions such as anxiety, fear, or sadness, especially during the pandemic when the processing of related information is more likely to trigger such intense emotional responses [57,58]. Using real-time communication social media before bedtime may increase the pressure of social interaction, particularly the expectation of immediate replies, which may make users feel the need to stay online, experiencing pressure and guilt when unable to respond immediately [73], triggering the fear of missing out (FOMO) [74], thereby increasing pre-sleep psychological activity and negatively impacting sleep quality. Additionally, using these two types of social media may lead to increased cognitive load, especially when dealing with large amounts of information and engaging in social interaction [75]. This cognitive burden keeps the brain active, making it difficult to transition smoothly into the sleep state [76], affecting the sleep quality. Therefore, our data supports the view that different modes and types of social media use may indirectly affect sleep quality through different mechanisms by affecting psychological arousal, emotional responses, social interaction pressure, and cognitive load.
The third notable observation from this study is the significant association found between the daytime use of specific types of social media applications—including news, short video, dating apps, and content community platforms—and sleep quality, mediated by social media addiction. This finding underscores the potential mediating role of social media addiction in the relationship between daytime social media use and sleep quality. Additionally, this study also found a direct negative association between pre-sleep social media use and sleep quality, while daytime social media use is not only directly related to sleep quality but also associated with sleep quality through the mediating path of social media addiction. These results may indicate that the daytime engagement with applications such as news, short videos, dating apps, and content community platforms is associated with the development of addictive behaviors, which in turn is associated with sleep quality. The results of this study may suggest that during the day, the use of applications such as news, short videos, dating apps, and content community platforms can facilitate the formation of addictive behaviors, which in turn negatively affects users’ sleep quality. Influenced by the evolution of the era and the pandemic, the Chinese public bears enormous pressure [77], which may lead them to rely more on social media such as news, short videos, dating apps, and content communities to alleviate anxiety and regulate negative emotions [78–80], thus forming a dependency on social media. This dependency on social media formed during the day may affect nighttime sleep quality through continuous psychological stress, anxiety, or other psychophysiological mechanisms [81]. Alternatively, social media addiction may induce pre-sleep social media use [82], directly reducing sleep time [83], leading to overuse [84,85], and affecting sleep quality. Furthermore, social media addiction may also bring about sleep disorders and negative sleep attitudes [86], negatively impacting the perception of sleep quality [87] and resulting in poor sleep quality assessments. Compared to existing studies, this research not only explores the relationship between social media use and sleep quality within the specific context of China and examines the mediating role of social media addiction but also provides a more refined analysis by segmenting the types of social media applications.
This research provides vital insights into the relationship between social media usage habits and sleep quality. Our analysis reveals a significant positive correlation between pre-sleep social media use and poorer sleep quality, aligning with the common expectation that using social media before bed may disrupt sleep. Moreover, the study delves further into social media addiction as a potential mediating mechanism, indicating that daytime use of specific types of social media applications (such as news apps, short video apps, dating apps, and content community platforms) may contribute to adverse effects on sleep quality by fostering social media addiction.
These findings are essential for public health policymakers, researchers, and social media users. They underscore the necessity of further research into the relationship between social media use and sleep disturbances, considering the specific context of individuals’ social media usage habits. Additionally, the results of this study can support the development and implementation of targeted interventions aimed at reducing the behavior of using social media before bedtime to improve sleep quality.
Limitations and Future Directions
Although this study has offered significant insights into the relationship between social media use and sleep quality, it is limited by its cross-sectional research design, which does not allow for the determination of causality between the variables. Therefore, our conclusions should be interpreted as revealing correlations rather than causations. The discovery of these correlations highlights the need for future research, mainly through longitudinal research designs and experimental methods, to further explore the potential impact of social media use habits on sleep quality. It will validate these preliminary findings and investigate the underlying causal mechanisms. Future research should include more control variables to accurately capture the relationship between social media use and sleep quality, thereby overcoming the limitations identified in this study and elucidating the dynamics of this relationship.
Furthermore, this research was conducted during the COVID-19 pandemic to explore the association between social media use and sleep quality. We recognize that the stress and anxiety caused by the pandemic are also significant factors affecting sleep quality. Therefore, while this study provides preliminary insights into the potential impact of social media use on sleep quality during this particular period, we also emphasize the need for further research to explore the persistence of this relationship in different contexts and consider other potential influencing factors. Future studies should explore the relationship between social media use and sleep quality after relaxing global pandemic control measures to provide a more comprehensive understanding.
This study identified an association between pre-sleep social media use and decreased sleep quality. Frequent interaction with specific types of social media, such as news and information apps, short video platforms, social networking apps, and content community platforms, is associated with an increased likelihood of addiction, which is in turn associated with decreased sleep quality. This adds to the evidence regarding the impact of social media on individuals’ daily lives. However, the mediating role of social media addiction in the relationship between social media use and sleep quality suggests a complex interaction, with the specific effects of addiction on sleep quality warranting further study.
In practical terms, based on the in-depth analysis of this study, it is clear that raising public awareness about the potential impact of social media use (especially before bedtime) on sleep quality through educational activities and public health campaigns is not a sufficient solution. The core challenge lies in how to coach users to adopt healthier strategies for coping with emotions and stress rather than relying on social media for diversion, particularly when faced with negative news and information. Public organizations and other relevant bodies must prioritize and promote educational resources and guidance on emotional management and stress relief. The widespread adoption of effective emotional regulation strategies and stress management techniques can assist individuals in handling the stresses and emotional fluctuations of daily life in healthier ways and reduce social media addictive behaviors and their negative impact on sleep quality. Therefore, comprehensive intervention measures should include, but not be limited to, mental health education, emotional regulation skill training, and strategies promoting healthy social media usage habits to improve overall public sleep quality and well-being.
Acknowledgement: We extend our heartfelt appreciation to those who completed the online survey. Our sincere thanks also go to the editors and reviewers for their diligent work in assessing our manuscript and providing constructive comments.
Funding Statement: The authors received no specific funding for this study.
Author Contributions: The authors confirm contribution to the paper as follows: study conception and design: Hao Gao; data collection: Hao Gao, Yijie Ye; analysis and interpretation of results: Yijie Ye, Liujiang Ye; draft manuscript preparation: Yijie Ye, Han Wang. All authors reviewed the results and approved the final version of the manuscript.
Availability of Data and Materials: The data supporting this research are available from the authors on reasonable request.
Ethics Approval: This study has been performed in accordance with the principles stated in the Declaration of Helsinki and has received ethical approval from the Biomedical Research Ethics Committee of Nanjing Normal University (IRB Number: NNU2022060054). All participants signed the informed consent in this study.
Conflicts of Interest: The authors declare that they have no conflicts of interest to report regarding the present study.
References
1. Ohayon M, Wickwire EM, Hirshkowitz M, Albert SM, Avidan A, Daly FJ, et al. National Sleep Foundation’s sleep quality recommendations: first report. Sleep Health. 2017 Feb;3(1):6–19. doi:10.1016/j.sleh.2016.11.006 [Google Scholar] [PubMed] [CrossRef]
2. Chattu VK, Manzar MD, Kumary S, Burman D, Spence DW, Pandi-Perumal SR. The global problem of insufficient sleep and its serious public health implications. Healthcare. 2019 Mar;7(1):1. doi:10.3390/healthcare7010001 [Google Scholar] [PubMed] [CrossRef]
3. Morin CM, Benca R. Chronic insomnia. Lancet. 2012;379(9821):1129–41. doi:10.1016/S0140-6736(11)60750-2 [Google Scholar] [PubMed] [CrossRef]
4. Abiddine FZE, Aljaberi MA, Gadelrab HF, Lin CY, Muhammed A. Mediated effects of insomnia in the association between problematic social media use and subjective well-being among university students during COVID-19 pandemic. Sleep Epidemiol. 2022 Dec;2(6):100030. doi:10.1016/j.sleepe.2022.100030 [Google Scholar] [PubMed] [CrossRef]
5. Lustberg L, Reynolds CFIII. Depression and insomnia: questions of cause and effect. Sleep Med Rev. 2000;4(3):253–62. doi:10.1053/smrv.1999.0075 [Google Scholar] [PubMed] [CrossRef]
6. Altena E, Baglioni C, Espie CA, Ellis J, Gavriloff D, Holzinger B, et al. Dealing with sleep problems during home confinement due to the COVID-19 outbreak: practical recommendations from a task force of the European CBT-I Academy. J Sleep Res. 2020;29(4):e13052. doi:10.1111/jsr.13052 [Google Scholar] [PubMed] [CrossRef]
7. Pinto J, van Zeller M, Amorim P, Pimentel A, Dantas P, Eusébio E, et al. Sleep quality in times of COVID-19 pandemic. Sleep Med. 2020 Oct;74:81–5. doi:10.1016/j.sleep.2020.07.012 [Google Scholar] [PubMed] [CrossRef]
8. Jahrami H, BaHammam AS, Bragazzi NL, Saif Z, Faris M, Vitiello MV. Sleep problems during the COVID-19 pandemic by population: a systematic review and meta-analysis. J Clin Sleep Med. 2021 Feb;17(2):299–313. doi:10.5664/jcsm.8930 [Google Scholar] [PubMed] [CrossRef]
9. Ono BHVS, Souza JC. Sleep and immunity in times of COVID-19. Rev Assoc Med Bras. 2020;66:143–7. doi:10.1590/1806-9282.66.S2.143 [Google Scholar] [PubMed] [CrossRef]
10. Yang L, Liu S, Liu J, Zhang Z, Wan X, Huang B, Chen Y, Zhang Y. COVID-19: immunopathogenesis and immunotherapeutics. Signal Transduct Target Ther. 2020 Jul 25;5(1):128. doi:10.1038/s41392-020-00243-2 [Google Scholar] [PubMed] [CrossRef]
11. Alonzo R, Hussain JR, Stranges S, Anderson KK. Interplay between social media use, sleep quality, and mental health in youth: a systematic review. Sleep Med Rev. 2021 Apr;56:101414. doi:10.1016/j.sleep.2019.11.1017. [Google Scholar] [CrossRef]
12. Garett R, Liu S, Young SD. The relationship between social media use and sleep quality among undergraduate students. Inform Commun Soc. 2018 Feb;21(2):163–73. doi:10.1080/1369118X.2016.1266374 [Google Scholar] [PubMed] [CrossRef]
13. Adiwibawa DN, Munandar TA, Ruqayyah S, Anulus A. Hubungan penggunaan media sosial dengan kejadian insomnia pada mahasiswa fakultas kedokteran universitas islam al-azhar angkatan 2021. Nusantara Hasana J. 2023;2(11):118–29. doi:10.59003/nhj.v2i11.835. [Google Scholar] [CrossRef]
14. Graham S, Mason A, Riordan B, Winter T, Scarf D. Taking a break from social media improves wellbeing through sleep quality. Cyberpsychol Behav Soc Netw. 2021 Jun 1;24(6):421–5. doi:10.1089/cyber.2020.0217 [Google Scholar] [PubMed] [CrossRef]
15. Hannahcurrey. Digital 2022: another year of bumper growth; We Are Social UK. 2023. Available from: https://wearesocial.com/uk/blog/2022/01/digital-2022-another-year-of-bumper-growth-2/. [Accessed 2023]. [Google Scholar]
16. Kashif M, Aziz-Ur-Rehman MK, Javed MK. Social media addiction due to coronavirus. Int J Med Sci Clin Res Rev. 2020;3(4):331–6. [Google Scholar]
17. Gupta R, Grover SR, Basu A, Krishnan V, Tripathi A, Subramanyam A, et al. Changes in sleep pattern and sleep quality during COVID-19 lockdown. Indian J Psychiatry. 2020;62(4):370–8. doi:10.4103/psychiatry.IndianJPsychiatry_523_20 [Google Scholar] [PubMed] [CrossRef]
18. Ni MY, Yang L, Leung CMC, Li N, Yao XI, Wang Y, et al. Mental health, risk factors, and social media use during the COVID-19 epidemic and cordon sanitaire among the community and health professionals in Wuhan, China: cross-sectional survey. JMIR Ment Health. 2020;7(5):e19009. doi:10.2196/19009 [Google Scholar] [PubMed] [CrossRef]
19. China Sleep Research Association. “China sleep study report (2022)” released, revealing the current state of Chinese sleep. Available from: http://www.zgsmyjh.org/nd.jsp?id=777#skeyword=%E7%9D%A1%E7%9C%A0%E8%B4%A8%E9%87%8F&_np=0_35. [Accessed 2022]. [Google Scholar]
20. Atske S. Social media use in 2021. Pew res center: internet, sci & tech; 2022. Available from: https://www.pewresearch.org/internet/2021/04/07/social-media-use-in-2021/. [Accessed 2023]. [Google Scholar]
21. Xu X, Zhu R, Sharma Mk, Zhao Y. The influence of social media on sleep quality: a study of undergraduate students in Chongqing. China J Nurs Care. 2015;4(253):2167–1168. doi:10.4172/2167-1168.1000253. [Google Scholar] [CrossRef]
22. Woods HC, Scott H. Sleepyteens: social media use in adolescence is associated with poor sleep quality, anxiety, depression and low self-esteem. J Adolesc. 2016;51:41–9. doi:10.1016/j.adolescence.2016.05.008 [Google Scholar] [PubMed] [CrossRef]
23. Chang A-M, Aeschbach D, Duffy JF, Czeisler CA. Evening use of light-emitting eReaders negatively affects sleep, circadian timing, and next-morning alertness. Proc Natl Acad Sci. 2015;112(4):1232–7. doi:10.1073/pnas.1418490112 [Google Scholar] [PubMed] [CrossRef]
24. Wang TJ, Kang CL, Tsai JL, Song WT, Lien ASY. Social media (Facebook) improper use and the influence of sleeping quality in Taiwan’s university students. Sci Prog. 2021;104(2). doi:10.1177/00368504211011878 [Google Scholar] [PubMed] [CrossRef]
25. Gaya AR, Brum R, Brites K, Gaya A, Schneiders LB, Angelo M, et al. Electronic device and social network use and sleep outcomes among adolescents: the EHDLA study. BMC Public Health. 2023;23(1):1–11. doi:10.1186/s12889-023-15579-x [Google Scholar] [PubMed] [CrossRef]
26. Siebers T, Beyens I, Baumgartner S, Valkenburg PM. Adolescents’ digital nightlife: the comparative effects of day-and nighttime smartphone use on sleep quality; 2023. doi:10.31234/osf.io/cdg73. [Google Scholar] [CrossRef]
27. Samaha M, Hawi NS. Relationships among smartphone addiction, stress, academic performance, and satisfaction with life. Comput Hum Behav. 2016;57:321–5. doi:10.1016/j.chb.2015.12.045. [Google Scholar] [CrossRef]
28. Kuss DJ, Griffiths MD. Online social networking and addiction—a review of the psychological literature. Int J Environ Res Public Health. 2011;8(9):3528–52. doi:10.3390/ijerph8093528 [Google Scholar] [PubMed] [CrossRef]
29. Malaeb D, Salameh P, Barbar S, Awad E, Haddad C, Hallit R, et al. Problematic social media use and mental health (depression, anxiety, and insomnia) among Lebanese adults: any mediating effect of stress? Perspect Psychiatr Care. 2021;57(2):539–49. doi:10.1111/ppc.12576 [Google Scholar] [PubMed] [CrossRef]
30. Zhu X, Zheng T, Ding L, Zhang X, Li Z, Jiang H. Exploring associations between social media addiction, social media fatigue, fear of missing out and sleep quality among university students: a cross-section study. PLoS One. 2023;18(10):e0292429. doi:10.1371/journal.pone.0292429 [Google Scholar] [PubMed] [CrossRef]
31. Tian Y, Zhang H, Jiang Y, Yang Y. Understanding trust and perceived risk in sharing accommodation: an extended elaboration likelihood model and moderated by risk attitude. J Hosp Mark Manag. 2022;31(3):348–68. doi:10.1080/19368623.2022.1986190. [Google Scholar] [CrossRef]
32. Zhu C, Fong LHN, Gao H, Liu CYN. When TikTok meets celebrity: an investigation of how celebrity attachment influences visit intention. Curr Issues Tour. 2023;26(17):2762–76. doi:10.1080/13683500.2022.2097058. [Google Scholar] [CrossRef]
33. Xue D, Liu T, Chen X, Liu X, Chao M. Data on media use and mental health during the outbreak of COVID-19 in China. Data in Brief. 2021;35:106765. doi:10.1016/j.dib.2021.106765 [Google Scholar] [PubMed] [CrossRef]
34. Correa T, Hinsley AW, de Zúñiga HG. Who interacts on the Web?: the intersection of users’ personality and social media use. Comput Hum Behav. 2010 Mar;26(2):247–53. doi:10.1016/j.chb.2009.09.003. [Google Scholar] [CrossRef]
35. Shensa A, Escobar-Viera CG, Sidani JE, Bowman ND, Marshal MP, Primack BA. Problematic social media use and depressive symptoms among U.S. young adults: a nationally-representative study. Soc Sci Med. 2017 Jun;182:150–7. doi:10.1016/j.socscimed.2017.03.061 [Google Scholar] [PubMed] [CrossRef]
36. Griffiths M. Internet addiction-time to be taken seriously? Addict Res. 2000 Jan;8(5):413–8. doi:10.3109/16066350009005587. [Google Scholar] [CrossRef]
37. Torsheim TCS, Brunborg GS, Pallesen S. Development of a Facebook addiction scale. Psychol Rep. 2012 Apr;110(2):501–17. doi:10.2466/02.09.18.PR0.110.2.501-517 [Google Scholar] [PubMed] [CrossRef]
38. Andreassen CS, Pallesen S, Griffiths MD. The relationship between addictive use of social media, narcissism, and self-esteem: findings from a large national survey. Addict Behav. 2017 Jan;64:287–93. doi:10.1016/j.addbeh.2016.03.006 [Google Scholar] [PubMed] [CrossRef]
39. Leung H, Pakpour AH, Strong C, Lin YC, Tsai MC, Griffiths MD, et al. Measurement invariance across young adults from Hong Kong and Taiwan among three internet-related addiction scales: bergen social media addiction scale (BSMASsmartphone application-based addiction scale (SABASand internet gaming disorder scale-short form (IGDS-SF9) (study Part A). Addict Behav. 2020;101:105969. doi:10.1016/j.addbeh.2019.04.027 [Google Scholar] [PubMed] [CrossRef]
40. Margraf JJ. Addictive social media use during COVID-19 outbreak: validation of the Bergen Social Media Addiction Scale (BSMAS) and investigation of protective factors in nine countries. Curr Psychol. 2022;43:13022–40. [Google Scholar]
41. Griffiths M. A ‘components’ model of addiction within a biopsychosocial framework. J Subst Use. 2005;10(4):191–7. doi:10.1080/14659890500114359. [Google Scholar] [CrossRef]
42. Buysse DJ, Reynolds CFIII, Monk TH, Berman SR, Kupfer DJ. The Pittsburgh sleep quality index: a new instrument for psychiatric practice and research. Psychiat. Res. 1989 May;28(2):193–213. doi:10.1016/0165-1781(89)90047-4 [Google Scholar] [PubMed] [CrossRef]
43. Yunzhao T, lingling M, Daiqing L, Min Y, Yanjuan Z, Chenguang L, et al. Interaction of sleep quality and sleep duration on glycemic control in patients with type 2 diabetes mellitus. Chin Med J. 2014;127(20):3543–7. doi:10.3760/cma.j.issn.0366-6999.20141512 [Google Scholar] [PubMed] [CrossRef]
44. Kirchner H.L. S, Quan SF, Gottlieb DJ, Kapur V, Newman A. The effects of age, sex, ethnicity, and sleep-disordered breathing on sleep architecture. Arch Intern Med. 2004 Feb 23;164(4):406. doi:10.1001/archinte.164.4.406 [Google Scholar] [PubMed] [CrossRef]
45. Cole JC, Motivala SJ, Buysse DJ, Oxman MN, Levin MJ, Irwin MR. Validation of a 3-factor scoring model for the pittsburgh sleep quality index in older adults. Sleep. 2006 Jan;29(1):112–6. doi:10.1093/sleep/29.1.112 [Google Scholar] [PubMed] [CrossRef]
46. Madrid-Valero JJ, Martínez-Selva JM, Ribeiro do Couto B, Sánchez-Romera JF, Ordoñana JR. Age and gender effects on the prevalence of poor sleep quality in the adult population. Gac Sanit. 2017 Jan;31(1):18–22. doi:10.1016/j.gaceta.2016.05.013 [Google Scholar] [PubMed] [CrossRef]
47. Kotronoulas GC, Papadopoulou CN, Papapetrou A, Patiraki E. Psychometric evaluation and feasibility of the Greek Pittsburgh Sleep Quality Index (GR-PSQI) in patients with cancer receiving chemotherapy. Support Care Cancer. 2011;19:1831–40. doi:10.1007/s00520-010-1025-4 [Google Scholar] [PubMed] [CrossRef]
48. Patel NP, Grandner MA, Xie D, Branas CC, Gooneratne N. “Sleep disparity” in the population: poor sleep quality is strongly associated with poverty and ethnicity. BMC Public Health. 2010 Dec;10(1):475. doi:10.1186/1471-2458-10-475 [Google Scholar] [PubMed] [CrossRef]
49. Liao YJ, Kelly BC, Xie L, Xiang YT, Qi C, et al. Gender and regional differences in sleep quality and insomnia: aa general population-based study in hunan province of China. Sci Rep. 2017 Mar 6;7(1):43690. doi:10.1038/srep43690 [Google Scholar] [PubMed] [CrossRef]
50. Faul F, Erdfelder E, Lang AG, Buchner A. G* Power 3: a flexible statistical power analysis program for the social, behavioral, and biomedical sciences. Behav Res Methods. 2007;39(2):175–91. doi:10.3758/BF03193146 [Google Scholar] [PubMed] [CrossRef]
51. Demirci K, Akgönül M, Akpinar A. Relationship of smartphone use severity with sleep quality, depression, and anxiety in university students. J Behav Addict. 2015;4(2):85–92. doi:10.1556/2006.4.2015.010 [Google Scholar] [PubMed] [CrossRef]
52. MacKinnon DP, Lockwood CM, Williams J. Confidence limits for the indirect effect: distribution of the product and resampling methods. Multivar Behav Res. 2004;39(1):99–128. doi:10.1207/s15327906mbr3901_4 [Google Scholar] [PubMed] [CrossRef]
53. Levenson JC, Shensa A, Sidani JE, Colditz JB, Primack BA. The association between social media use and sleep disturbance among young adults. Prev Med. 2016;85:36–41. doi:10.1016/j.ypmed.2016.01.001 [Google Scholar] [PubMed] [CrossRef]
54. Shensa AJC, Sidani JE, Colditz JB, Primack BA. Social media use before bed and sleep disturbance among young adults in the United States: a nationally representative study. Sleep. 2017;40(9):zsx113. doi:10.1093/sleep/zsx113 [Google Scholar] [PubMed] [CrossRef]
55. Crowley SJ, Cain SW, Burns AC, Acebo C, Carskadon MA. Increased sensitivity of the circadian system to light in early/mid-puberty. J Clin Endocrinol Metab. 2015 Nov;100(11):4067–73. doi:10.1210/jc.2015-2775 [Google Scholar] [PubMed] [CrossRef]
56. Browman CP, Tepas DI. The effects of presleep activity on all-night sleep. Psychophysiology. 1976;13(6):536–40. doi:10.1111/j.1469-8986.1976.tb00876.x [Google Scholar] [PubMed] [CrossRef]
57. Kramer ADI, Guillory JE, Hancock JT. Experimental evidence of massive-scale emotional contagion through social networks. Proc Natl Acad Sci. 2014;111(24):8788–90. doi:10.1073/pnas.1320040111 [Google Scholar] [PubMed] [CrossRef]
58. Niederkrotenthaler T, Stack S, Till B, Sinyor M, Pirkis J, Garcia D, et al. Association of increased youth suicides in the United States with the release of 13 reasons why. JAMA Psychiat. 2019;76(9):933–40. doi:10.1001/jamapsychiatry.2019.0922 [Google Scholar] [PubMed] [CrossRef]
59. van den Bulck J. Television viewing, computer game playing, and Internet use and self-reported time to bed and time out of bed in secondary-school children. Sleep. 2004;27(1):101–4. doi:10.1093/sleep/27.1.101 [Google Scholar] [PubMed] [CrossRef]
60. Touitou Y, Touitou D, Reinberg A. Disruption of adolescents’ circadian clock: the vicious circle of media use, exposure to light at night, sleep loss and risk behaviors. J Physiol–Paris. 2016;110(4):467–79. doi:10.1016/j.jphysparis.2017.05.001 [Google Scholar] [PubMed] [CrossRef]
61. van den Bulck J. Text messaging as a cause of sleep interruption in adolescents, evidence from a cross-sectional study. J Sleep Res. 2003;12(3):263. doi:10.1046/j.1365-2869.2003.00362.x [Google Scholar] [PubMed] [CrossRef]
62. Chen H, Zhang G, Wang Z, Feng S, Li H. The associations between daytime physical activity, while-in-bed smartphone use, sleep delay, and sleep quality: a 24-h investigation among Chinese college students. Int J Environ Res Public Health. 2022;19(15):9693. doi:10.3390/ijerph19159693 [Google Scholar] [PubMed] [CrossRef]
63. Pandi-Perumal SR, Cardinali DP, Zaki NFW, Karthikeyan R, Spence DW, Reiter RJ, et al. Timing is everything: circadian rhythms and their role in the control of sleep. Front Neuroendocrinol. 2022;66:100978. doi:10.1016/j.yfrne.2022.100978 [Google Scholar] [PubMed] [CrossRef]
64. Xanidis N, Brignell CM. The association between the use of social network sites, sleep quality and cognitive function during the day. Comput Hum Behav. 2016;55:121–6. doi:10.1016/j.chb.2015.09.004. [Google Scholar] [CrossRef]
65. Bhat S, Pinto-Zipp G, Upadhyay H, Polos PG. “To sleep, perchance to tweet”: in-bed electronic social media use and its associations with insomnia, daytime sleepiness, mood, and sleep duration in adults. Sleep Health. 2018;4(2):166–73. doi:10.1016/j.sleh.2017.12.004 [Google Scholar] [PubMed] [CrossRef]
66. Chuang H.L. HT, Kuo CP, Yeh TP, Liao WC. Electronic device use before bedtime and sleep quality among university students. Healthcare. 2021;9(9):1091. doi:10.3390/healthcare9091091 [Google Scholar] [PubMed] [CrossRef]
67. Greenall EAG. Catastrophic worry in primary insomnia. J Behav Ther Exp Psychiat. 2003;34(1):11–23. doi:10.1016/s0005-7916(03)00003-x [Google Scholar] [PubMed] [CrossRef]
68. Gregory AM, Willis TA, Wiggs L, Harvey AG, STEPS team. Presleep arousal and sleep disturbances in children. Sleep. 2008;31(12):1745–7. doi:10.1093/sleep/31.12.1745 [Google Scholar] [PubMed] [CrossRef]
69. Zaremohzzabieh Z, Samah BA, Omar SZ, Bolong J, Kamarudin NA. Addictive Facebook use among university students. Asi Social Sci. 2014;10(6):107–16. doi:10.5539/ass.v10n6p107. [Google Scholar] [CrossRef]
70. Huang FY, Huang DH, Lin HY, Chiu SL. Analysis of the psychological traits, Facebook usage, and Facebook addiction model of Taiwanese university students. Telemat Inform. 2014;31(4):597–606. doi:10.1016/j.tele.2014.01.001. [Google Scholar] [CrossRef]
71. Bawden D, Robinson L. The dark side of information: overload, anxiety and other paradoxes and pathologies. J Inf Sci. 2009;35(2):180–91. doi:10.1177/0165551508095781. [Google Scholar] [CrossRef]
72. Keib K, Espina C, Lee YI, Wojdynski BW, Choi D, Bang H. Picture this: the influence of emotionally valenced images, on attention, selection, and sharing of social media news. Media Psychol. 2018;21(2):202–21. doi:10.1080/15213269.2017.1378108. [Google Scholar] [CrossRef]
73. Skierkowski D, Wood RM. To text or not to text? The importance of text messaging among college-aged youth. Comput Hum Behav. 2012;28(2):744–56. doi:10.1016/j.chb.2011.11.023. [Google Scholar] [CrossRef]
74. Thomée S, Dellve L, Härenstam A, Hagberg M. Perceived connections between information and communication technology use and mental symptoms among young adults—a qualitative study. BMC Public Health. 2010;10:1–14. doi:10.1186/1471-2458-10-66 [Google Scholar] [PubMed] [CrossRef]
75. Islam AKMN, Laato S, Talukder S, Sutinen E. Misinformation sharing and social media fatigue during COVID-19: an affordance and cognitive load perspective. Technol Forecast Soc Change. 2020;159:120201. doi:10.1016/j.techfore.2020.120201 [Google Scholar] [PubMed] [CrossRef]
76. Saper CB, Fuller PM, Pedersen NP, Lu J, Scammell TE. Sleep state switching. Neuron. 2010;68(6):1023–42. doi:10.1016/j.neuron.2010.11.032 [Google Scholar] [PubMed] [CrossRef]
77. Cao W, Fang Z, Hou G, Han M, Xu X, Dong J, et al. The psychological impact of the COVID-19 epidemic on college students in China. Psychiat Res. 2020;287:112934. doi:10.1016/j.psychres.2020.112934 [Google Scholar] [PubMed] [CrossRef]
78. Lavoie JAA, Pychyl TA. Cyberslacking and the procrastination superhighway: a web-based survey of online procrastination, attitudes, and emotion. Soc Sci Comput Rev. 2001;19(4):431–44. doi:10.1177/089443930101900403. [Google Scholar] [CrossRef]
79. Velezmoro R, Lacefield K, Roberti JW. Perceived stress, sensation seeking, and college students’ abuse of the Internet. Comput Hum Behav. 2010;26(6):1526–30. doi:10.1016/j.chb.2010.05.020. [Google Scholar] [CrossRef]
80. Caplan SE. Relations among loneliness, social anxiety, and problematic internet use. Cyberpsychol Behav. 2007 Apr;10(2):234–42. doi:10.1089/cpb.2006.9963 [Google Scholar] [PubMed] [CrossRef]
81. Nakshine VS, Thute P, Khatib MN, Sarkar B. Increased screen time as a cause of declining physical, psychological health, and sleep patterns: a literary review. Cureus. 2022;14(10):e30051. doi:10.7759/cureus.30051 [Google Scholar] [PubMed] [CrossRef]
82. Woods HC, Scott H. Sleepyteens: social media use in adolescence is associated with poor sleep quality, anxiety, depression and low self-esteem. J Adolesc. 2016 Aug;51(1):41–9. doi:10.1016/j.adolescence.2016.05.008 [Google Scholar] [PubMed] [CrossRef]
83. Eroğlu O, Yıldırım Y. Examining the relationship between the purpose of using social media networks addiction and sleep quality. Turk J Sports Sci. 2017;1(1):1–10. doi:10.51960/jitte.901629. [Google Scholar] [CrossRef]
84. Khan MM. Adverse effects of excessive mobile phone use. Int J Occup Med Environ Health. 2008;21(4):289–93. doi:10.2478/v10001-008-0028-6 [Google Scholar] [PubMed] [CrossRef]
85. Kalak N, Gerber M, Kirov R, Mikoteit T, Yordanova J, Pühse U, et al. Daily morning running for 3 weeks improved sleep and psychological functioning in healthy adolescents compared with controls. J Adolesc Health. 2012;51(6):615–22. doi:10.1016/j.jadohealth.2012.02.020 [Google Scholar] [PubMed] [CrossRef]
86. Gaultney J.F. HD, Ruggiero AR. Direct and indirect associations of sleep knowledge and attitudes with objective and subjective sleep duration and quality via sleep hygiene. J Prim Prev. 2018;39:555–70. doi:10.1007/s10935-018-0526-7 [Google Scholar] [PubMed] [CrossRef]
87. Vernon L, Barber BL, Modecki KL. Adolescent problematic social networking and school experiences: the mediating effects of sleep disruptions and sleep quality. Cyberpsychol Behav Soc Netw. 2015 Jul;18(7):386–92. doi:10.1089/cyber.2015.0107 [Google Scholar] [PubMed] [CrossRef]
Supplementary Materials
Cite This Article
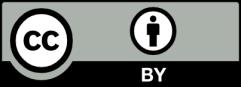
This work is licensed under a Creative Commons Attribution 4.0 International License , which permits unrestricted use, distribution, and reproduction in any medium, provided the original work is properly cited.