Open Access
ARTICLE
The Relationships between the Short Video Addiction, Self-Regulated Learning, and Learning Well-Being of Chinese Undergraduate Students
1 Faculty of Education, Beijing Normal University, Beijing, 100875, China
2 National Institute of Vocational Education, Beijing Normal University, Beijing, 100875, China
3 Faculty of Educational Administration, Beijing Institute of Education, Beijing, 100120, China
4 School of Maritime, Hainan Vocational University of Science and Technology, Haikou, 571126, China
5 Graduate Institute of Technological & Vocational Education, National Taipei University of Technology, Taipei, 106344, Taiwan
* Corresponding Author: Yuting Cui. Email:
(This article belongs to the Special Issue: Understanding Technological Addiction and Its Impact on Well-Being: Implications for Policymakers)
International Journal of Mental Health Promotion 2024, 26(10), 805-815. https://doi.org/10.32604/ijmhp.2024.055814
Received 07 July 2024; Accepted 26 August 2024; Issue published 31 October 2024
Abstract
Background: With the global popularity of short videos, particularly among young people, short video addiction has become a worrying phenomenon that poses significant risks to individual health and adaptability. Self-regulated learning (SRL) strategies are key factors in predicting learning outcomes. This study, based on the SRL theory, uses short video addiction as the independent variable, SRL strategies as the mediating variable, and learning well-being as the outcome variable, aiming to reveal the relationships among short video addiction, self-regulated learning, and learning well-being among Chinese college students. Methods: Using a cross-sectional study design and applying the snowball sampling technique, an online survey was administered to Chinese undergraduate students. A total of 706 valid questionnaires were collected, with an effective response rate of 85.7%. The average age of the participants was 20.5 years. Results: The results of structural equation modeling indicate that 7 hypotheses were supported. Short video addiction was negatively correlated with self-regulated learning strategies (preparatory, performance, and appraisal strategy), while SRL strategies were positively correlated with learning well-being. Additionally, short video addiction had a mediating effect on learning well-being through the three types of SRL strategies. The three types of SRL strategies explained 39% of the variance in learning well-being. Conclusion: Previous research has typically focused on the impact of self-regulated learning strategies on media addiction or problematic media use. This study, based on the SRL model, highlights the negative issues caused by short video addiction and emphasizes the importance of cultivating self-regulation abilities and media literacy. Short video addiction stems from failures in trait self-regulation, which naturally impairs the ability to effectively engage in self-regulation during the learning process. This study confirms and underscores that the SRL model can serve as an effective theoretical framework for helping students prevent short video addiction, engage in high-quality learning, and consequently enhance their learning well-being.Keywords
Short videos have created a sensation in China, and in less than a decade have become an essential medium for social media, gaining popularity worldwide and exerting a powerful influence on young people. The typical duration of a short video is 15 s, allowing individuals to watch several short videos within one minute. At the same time, short videos are highly intrusive, as the software algorithms push curated content to users during their viewing process. This provides users with a sense of presence and immersion. Additionally, short video apps offer entertainment and social features such as viewing, liking, commenting, following, and chatting with friends. Therefore, short videos are generally considered a highly addictive or overused form of social media.
Short video addiction refers to the fact that short video users are unable to control the length of time they spend watching short videos, and may experience adverse effects on their lives or studies as a result of inappropriate use of short videos [1], although of course, short video addicts may not feel that they are experiencing addiction. In addition, excessive viewing of short videos can make people emotionally unstable, irritable, and indifferent to real-world matters. Nowadays, short video apps are growing in popularity, especially among young people, as a result of their strong appeal. Short video addiction has thus become a worrying phenomenon as it poses significant risks to individuals’ health and adaptability [2]. Undergraduates are among the primary users of short videos, and the impact of short video addiction on this demographic requires more attention [3]. Students addicted to short videos may feel that they cannot achieve quick academic rewards, leading to a loss of confidence in their studies and an inability to complete academic tasks on time [3].
As time passes, procrastinators begin to see short videos as a tool for procrastination, repeatedly watching them [4]. However, there is still no adequate explanation mechanism for the impact of short video addiction on learning, and more empirical studies are needed to explain the impact of short video addiction on students. Although there is no evidence yet proving a relationship between short video addiction and self-regulation, past research has found that smartphone addiction is associated with poorer self-regulation [5]. For example, problematic smartphone use may be a predictor of academic procrastination [6], which is an inability to self-regulate learning well. In addition, Iyiola et al. found that social media addiction has an impact on self-regulated learning (SRL) [7]. The theory of SRL states that learners can actively participate in the learning process, and are able to monitor, control, and regulate their cognition [8]. To succeed in higher education, learners are expected to engage in self-regulation [9]. Since SRL outcomes are influenced by both human and environmental factors, when students monitor their learning, they make judgments based on any information they have that indicates their current learning status. Therefore, students may not be able to use SRL strategies well if they are interrupted by addiction factors. The SRL theory suggests that students’ role in managing their learning, study habits, and academic engagement is active and purposeful. If they can effectively manage their time and proactively adopt learning strategies, they can improve their academic performance, whereas adoption of ineffective self-regulation strategies could result in negative learning outcomes [10]. Overall, SRL is defined as taking responsibility for one’s own learning [11]. Therefore, SRL is considered a key component in predicting learning outcomes.
Subjective well-being is one component of mental health [12]. Since well-being and learning are interrelated [13], students experiencing good well-being while learning will continue to maintain intrinsic motivation for learning. In the school environment, learning is the central goal and activity for both students and teachers. If students feel they have difficulties in learning, it will affect their academic health [14]. Therefore, students’ well-being is an important educational goal, and research on this topic is of significant importance [15]. Additionally, literature indicates that there is a small but significant negative relationship between social media and well-being; however, the conclusions about the impact of social media on well-being are not consistent [16]. Given the importance of student well-being, it is crucial to identify variables that may negatively affect well-being [15]. Therefore, exploring this issue will contribute to the field of research on internet addiction.
Excessive use of short videos fosters a short-term oriented mindset, seeking immediate pleasure and satisfaction [3]. Consequently, video addiction and issues related to short video addiction continue to be subjects of ongoing concern, and it remains urgent to expand related research, as the negative impacts on adolescents have not yet been fully explored [17]. Moreover, short video addiction is considered one of the most severe types of modern technology addiction, and has become a significant social issue for governments, researchers, and the public. Although some studies have begun to explore the impact of short videos on learning, current research on this topic is still quite scarce within the broader context of technology addiction. Thus, it is necessary to explore the antecedents which affect learning well-being.
Self-regulation in different domains seems to affect an individual’s well-being, but this has not been well established in the field of education [18]. Additionally, many studies have explored learners’ SRL, but few have simultaneously focused on its three phases [19]. Therefore, Panadero suggested that more detailed research should be conducted to understand the specific mechanisms of SRL [20]. Although the SRL model provides a very detailed picture of its processes, more precise understanding of SRL mechanisms is still needed. By understanding the mechanism of short video addiction in SRL, it will be possible to clearly explain the influential nature of short video addiction on the learning process. Thus, this study explored the relationships among the short video addiction, three types of SRL, and the learning well-being of young Chinese students based on SRL theory. To achieve these objectives, this study focused on Chinese undergraduate students and used short video addiction as the independent variable, SRL strategies as the mediating variable, and learning well-being as the outcome variable in order to establish and verify a hypothesized model.
Theoretical Basis, Hypotheses and Modelling
Pintrich developed a conceptual framework for studying SRL which includes phases such as forethought, planning, activation, monitoring, control, reaction, and reflection [21]. As learners increase their use of generative strategies and actively create meaning during the learning process, their knowledge generation improves [8]. Based on the effectiveness of SRL, various theoretical models have been developed. However, the most widely known self-regulation model, proposed by Zimmerman, divides self-regulation into three distinct phases [22]. The first, the earliest and most important phase in the learning process is the forethought phase. In this phase, learners analyze the learning task, set their learning goals, and work out ways to achieve them. In this study, we refer to these ways as preparatory strategies.
In the second, the performance phase, learners conduct the learning activities, actively participating in them while monitoring and adjusting their learning behaviors so as to make sure they achieve their learning goals. In this study, we refer to these behaviors as performance strategies.
The appraisal phase is the last stage of the learning process in which learners review and evaluate the effectiveness of their learning and their learning outcomes in order to expand their learning experience and knowledge. In this study, we refer to these behaviors as evaluation strategies. To sum up, SRL has been conceptualized as a process involving three phases: the preparatory, performance, and appraisal phases [23].
More specifically, emotion management and environmental structuring are considered as preparatory strategies, while task strategies and time management are performance strategies, and self-evaluation and help-seeking are evaluation strategies [24]. All these aspects jointly influence learners’ actual regulatory behaviors. Overall, self-regulation (or self-directed learning) refers to the self-generated thoughts, feelings, and behaviors that are systematically oriented and adjusted to influence one’s learning and motivation.
The relationship between short video addiction and self-regulated learning
SRL clearly outlines the dynamic characteristics of self-regulation in the learning process [25]. In the self-regulation optimization cycle, learners begin by planning their learning activities, then they start to learn by adopting cognitive strategies to perform the activities while monitoring their learning behavior [25]. However, without interaction between the individual and the environment, self-regulation behaviors would not occur. Although these situational factors may play a smaller role than individual processes, these interactions are crucial to the self-regulation process. Research indicates that smartphone addiction, by increasing negative learning strategies, tends to cause university students addicted to smartphones to manage their time poorly, which may increase their procrastination [10]. Additionally, students addicted to short videos may believe that they cannot obtain quick academic rewards, causing them to lose confidence in learning and in their inability to complete academic tasks on time [3]. In summary, the more severe the student’s short video addiction tendency, the poorer their SRL strategies may be. Therefore, this study explored the relationships between short video addiction and participants’ three types of SRL strategies, with the following hypotheses:
H1: Short video addiction is negatively correlated with the preparatory strategy.
H2: Short video addiction is negatively correlated with the performance strategy.
H3: Short video addiction is negatively correlated with the appraisal strategy.
The relationship between self-regulated learning and learning well-being
All theorists agree that students who engage in SRL actively and constructively participate in the process of meaning-making, and adjust their thoughts, feelings, and behaviors as needed to influence their learning and motivation [26]. At the same time, it is widely believed that learning generates positive emotional and psychological outcomes [12]. In other words, knowing how to learn and being able to explore the ways of learning directly affect people’s well-being [27]. Additionally, research has pointed out that SRL strategies positively predict students’ psychological well-being, highlighting the importance of conscious self-regulation in learning activities as a crucial resource for students’ mental health [28]. However, when an individual feels unable to function effectively in a specific environment, it negatively impacts their well-being [27]. Other studies have also found that goal-oriented self-regulation behaviors (i.e., planning, monitoring, controlling, and reflecting) are positively correlated with college students’ mental health [29]. From the above, it can be inferred that the better students are at utilizing SRL strategies, the more likely they are to achieve a high level of learning well-being. Therefore, this study explored the relationships between three types of SRL strategies and participants’ learning well-being, with the following hypotheses:
H4: The preparatory strategy is positively correlated with learning well-being.
H5: The performance strategy is positively correlated with learning well-being.
H6: The appraisal strategy is positively correlated with learning well-being.
The relationship between short video addiction and learning well-being
Pathological internet use behaviors negatively affect an individual’s important activities and well-being [30]. The reason may be that internet addiction is caused by the simple fact that users combine coping with internet technology to escape negative emotions in daily life and promote more positive emotions, which in turn reduces well-being and life satisfaction [31]. Additionally, studies have found a significant relationship between social media addiction and well-being. As the degree of social media addiction increases, well-being decreases [32]. This is because, when continuous internet use allows users to “deepen” their addictive behaviors, internet addiction only increases negative rather than positive emotions [31]. Previous research has found that internet addiction negatively impacts well-being [33]. Furthermore, growing evidence suggests that the use of short videos is closely related to the mental health of adolescents, with higher levels of depression, fear of missing out, and lower core self-evaluations found in those with high usage durations and many online friends [34]. Other studies have found that reducing social media addiction and online victimization has a positive indirect effect on subjective well-being [35]. From the above, it can be inferred that when students’ short video addiction tendency becomes more severe, it may indirectly affect their learning well-being. Therefore, this study explored the relationship between short video addiction and participants’ learning well-being, with the following hypothesis:
H7: Short video addiction is indirectly negatively correlated with learning well-being.
Self-regulation is the process of people actively monitoring, regulating, and guiding their activities using a variety of both cognitive and behavioral techniques, thereby allowing them to achieve their personal goals. Based on the literature review, this study applied the SRL theory as the theoretical foundation for model construction, proposing seven research hypotheses. A hypothetical model was established to explore the relationships between short video addiction, three types of SRL strategies, and learning well-being, as shown in Fig. 1.
Figure 1: Hypothetical model.
This study was approved by the Academic Ethics Committee of Hainan Vocational University of Science and Technology. This study used snowball sampling based on the internet characteristics of short videos, and conducted an online survey through the Wenjuanxing platform (https://www.wjx.cn/, accessed on 30 July 2024). All student users of short videos were eligible to participate in this study. The data collection period for the questionnaire was from 15 to 29 January 2024. A total of 824 questionnaires were collected from Chinese undergraduate students.
This study was approved by the Academic Ethics Committee of Hainan Vocational University of Science and Technology (Approval No. HKD-2024-3). On the first page of the electronic questionnaire, an informed consent statement was provided, which included information on the study title, research background, survey purpose, data usage, anonymity principles, privacy protection, research leaders, and contact details. Participants were informed that participating in the survey would be considered as providing informed consent.
The participants in this study (number of questionnaires collected) totaled 824. Subsequently, invalid data were removed based on principles such as excessively short response times, overly consistent response patterns, and incomplete answers, resulting in the deletion of 118 questionnaires. The number of valid participants was 706, with an effective recovery rate of 85.7%. The average age was 20.5 years (standard deviation of 1.12 years). Other basic information is shown in Table 1.
This research was a cross-sectional, confirmatory research study. The questionnaire consisted of two parts: participant background and variable content. All variable items were either cited or adapted from studies with good reliability and validity. An expert validity review was conducted by three educational psychology experts. The assessment was carried out using a 5-point Likert scale, where 1 represents “strongly disagree” and 5 represents “strongly agree”.
This study referenced and adapted the Short Video Addiction Scale from Ye et al. [36], consisting of 10 items to measure participants’ perceived levels of short video usage. An example item is: “I will put aside things that need to be done or executed to spend time watching short videos.” Higher scores indicate more severe overuse or problematic use of short videos by students. The original questionnaire had a Cronbach’s α value of 0.93, a factor loading (FL) value of 0.83, a composite reliability (CR) value of 0.93, and an average variance extracted (AVE) value of 0.68.
This study used the SROL tool developed by Hong et al. to reclassify the original six subscales into three types of learning strategies [37], totaling 24 items, to measure participants’ application of SRL strategies. An example item for preparatory strategy is: “Before studying, I adjust my environment to avoid distractions.” An example item for performance strategy is: “I plan my study time every day to ensure learning.” An example item for appraisal strategy is: “After studying, I use available resources to enhance learning efficiency.” Higher scores indicate better performance in SRL strategies among students. The original questionnaire had Cronbach’s α values ranging from 0.73 to 0.89, FL values ranging from 0.66 to 0.92, CR values ranging from 0.87 to 0.93, and AVE values ranging from 0.66 to 0.82.
For this study, we adapted and revised the Learning Well-Being Scale developed by Ye et al. [36], consisting of 10 items designed to measure participants’ subjective well-being in their academic lives. An example item is: “Learning makes me feel more accomplished about my performance.” Higher scores indicate higher levels of perceived well-being in learning contexts. The original questionnaire reported a Cronbach’s α value of 0.86, a FL value of 0.86, a CR value of 0.95, and an AVE value of 0.75.
This study followed confirmatory research by employing structural equation modeling to validate the theoretical causal relationships of the hypothesized pathways. SPSS 27.0 and AMOS 26.0 were used to conduct measurement model analysis, reliability and validity analysis, overall fit analysis, hypothesis model verification, and mediation effect analysis. The results are presented in the following section. A threshold p-value of 0.05 was used to determine statistical significance.
Confirmatory factor analysis was conducted in AMOS 25.0 to estimate the five-factor measurement model. The results indicated an acceptable fit in this model; additionally, the t-values for each dimension ranged from 27.12 to 61.27 (***p < 0.001), demonstrating that all items in this study could reliably capture the varying responses from different samples [38]. Thus, the item reduction results were as follows: short video addiction was reduced from ten to six items; preparation strategy from eight to six items; performance strategy and method strategy from eight to five items; and learning well-being from 10 to six items (Table 2).
Reliability and validity analysis
The Cronbach’s α values and CR values for each dimension in this study ranged from 0.93 to 0.97, the FL values ranged from 0.83 to 0.91, and the AVE values ranged from 0.67 to 0.84, as shown in Table 3. When Cronbach’s α and CR exceed 0.7, FL exceeds 0.5, and AVE exceeds 0.5 [39,40], it indicates sufficient reliability and validity.
The square root of the AVE values was mostly higher than the correlations between the variables, indicating that discriminant validity was supported [41]. As shown in Table 4, each dimension in this study exhibited acceptable discriminant validity.
This study used AMOS to evaluate the model fit, which showed an acceptable fit in the hypothesized structural model: χ2 = 1485, df = 344, χ2/df = 4.32, RMSEA = 0.07, GFI = 0.87, AGFI = 0.51, NFI = 0.94, NNFI = 0.94, CFI = 0.95, IFI = 0.95, RFI = 0.93, PNFI = 0.81, and PGFI = 0.84.
The model verification results indicated that all six hypotheses were supported. Specifically, short video addiction was negatively correlated with preparatory strategy (β = −0.59***), performance strategy (β = −0.57***), and appraisal strategy (β = −0.61***). Additionally, preparatory strategy (β = 0.23***), performance strategy (β = 0.32***), and appraisal strategy (β = 0.28***) were positively correlated with well-being.
Short video addiction explained 35% of the variance in preparatory strategy with an f2 of 0.54, 32% of the variance in performance strategy with an f2 of 0.47, and 37% of the variance in appraisal strategy with an f2 of 0.59. The three types of SRL strategies explained 39% of the variance in learning well-being with an f2 of 0.64, as shown in Fig. 2.
Figure 2: Research model validation. Note: ***p < 0.001.
This study investigated the mediating effect of short video addiction on learning well-being through three types of SRL strategies. The total indirect effect of short video addiction on learning well-being was assessed by using a bootstrapping procedure with 5000 bootstrap samples to examine the 95% confidence intervals [42]. The results indicated that the total indirect effect of short video addiction on learning well-being through the three types of SRL strategies was significant (total standardized indirect effect = −0.49, p < 0.001), thus supporting H7 (Table 5).
Short video addiction can negatively predict self-regulated learning
The analysis results indicated a negative correlation between short video addiction and three types of SRL strategies: the preparatory, performance, and appraisal strategies. This implies that as students’ tendencies of short video addiction worsen, their use of SRL strategies becomes poorer. Furthermore, short video addiction itself is a consequence of inadequate self-regulated strategies. Since SRL is typically conceptualized to involve academic domain regulation, it similarly has adverse effects on self-regulated strategies in learning contexts. The reason for this might be attributed to the highly dynamic nature of learning, necessitating learners to self-regulate. Ideally, they should set goals, plan their learning processes, employ strategies, observe, and evaluate whether they can achieve their goals. If not, they must react and regulate their behaviors [25]. Additionally, the findings of this study align with Liu et al., who discovered that smartphone addiction, by increasing negative learning strategies, tends to lead to poorly managed time among college students prone to smartphone addiction, potentially increasing procrastination [10]. Moreover, Xie et al. suggested that students addicted to short videos may perceive themselves as unable to obtain quick academic rewards, leading to a loss of confidence in learning and an inability to complete academic tasks on time [3].
In addition, there are different levels of negative associations between short video addiction and self-regulation strategies in terms of different path coefficients. First, short video addiction may lead to a greater negative impact on an individual’s approach strategy, as addictive behaviors may weaken an individual’s ability to reflect, learn and select appropriate learning methods (appraisal strategy). This can make it difficult for individuals to learn from experience and improve their learning strategies, which can have a negative impact on their learning outcomes. Although short video addiction may also reduce the amount of time and effort an individual spends preparing for a learning activity, leading to inadequate or poorly executed learning plans (preparation strategy), this effect may not be as direct and far-reaching as the approach strategy. Finally, although short video addiction may distract learners and affect performance during the learning process (performance strategy), this effect is likely to be relatively small because the performance strategy relies more on immediate self-regulation skills than on long-term learning strategies or reflective processes.
Self-regulated learning can positively predict learning well-being
The analysis results showed a positive correlation between three types of SRL strategies and learning well-being. This indicates that the better students master and apply SRL strategies, the more likely they are able to experience learning well-being. These findings are consistent with the general belief that learning produces positive emotional and psychological outcomes [12]. In other words, knowing how to learn and being able to explore your learning methods directly impacts your well-being [27]. Additionally, the study by Fomina et al. pointed out that SRL strategies positively predict students’ psychological well-being, emphasizing the importance of conscious self-regulation in learning activities as a crucial resource for students’ mental health [28]. Similarly, the research by Wang et al. found that goal-oriented self-regulated behavior is positively correlated with young students’ psychological health [29].
Furthermore, in terms of the difference in path coefficients, this implies that performance strategy has the most significant impact on learning well-being of the three stages. Performance strategy involves the active participation, monitoring and adjustment of the learner during the actual learning process. This may include emotion management, concentration, and maintenance of motivation, which has a direct impact on learners’ immediate experience and learning outcomes. Appraisal strategy involves reflection on learning methods, assessment of learning outcomes and adjustment of future learning plans. Although the impact of the appraisal strategy on learning well-being is not as direct as that of the performance strategy, it enhances learning well-being by helping learners to learn from experience and to improve their learning strategies. The preparatory strategy occurs at the initial stage of the learning process and involves the learner’s analyses of the learning task.
Short video addiction can indirectly negatively predict learning well-being
The validation results showed that short video addiction is indirectly negatively correlated with well-being (through SRL strategies). The results indicate that students’ short video addiction may be related to their learning health and well-being, as their short video addiction tendency reduces their commitment to their learning goals. Short video addiction, also known as pathological short video use or excessive short video use, refers to users’ inability to properly control their behavior, which is generally considered harmful to users [1]. The reason for this may be that users increase their internet addiction to escape negative emotions in daily life and promote more positive emotions, thereby reducing their well-being and life satisfaction [31]. Additionally, the study by Çiftci and Yıldız found a significant relationship between social media addiction and well-being [32]. As the degree of social media addiction increases, well-being decreases. Longobardi et al. found that reducing social media addiction and online victimization has a positive indirect effect on subjective well-being [35]. Furthermore, Ye et al. found an indirect negative correlation between short video addiction and learning well-being [36]. As continuous internet use allows users to “deepen” their addictive behavior, internet addiction only increases negative rather than positive emotions [31]. Overall, short video addiction may weaken students’ use of SRL strategies. This is because short video addiction can take up a great deal of time and energy for learning, which in turn affects, for example, learning planning. This weakened SRL further reduces learning well-being. As a result, learners may not be able to effectively achieve their learning goals and are more likely to become frustrated in their learning.
Short-video addiction is an emerging area of research with rather limited international empirical findings at present [1]. Nong et al. stated that exploration of different forms of internet addiction will help us to better comprehend how these behavioral addictions impact students’ learning, life and mental health [33]. Therefore, this study theoretically explored the effects of short video addiction on SRL, as past research has generally focused on the influence of SRL strategies on media addiction or media problematic use, with few addressing the impact of addiction on SRL. The results of this study highlight the negative issues caused by short video addiction and underscore the importance of cultivating self-regulation abilities and media literacy. Trait self-regulation refers to cognitive, behavioral, and motivational strategies that maintain consistency between thoughts and actions with goals and intentions throughout daily life, representing a broad psychological process. State self-regulation, on the other hand, involves self-monitoring in a specific situation, but does not imply that learners can complete this regulatory process in all circumstances or at any time. Short video addiction results from a failure in trait self-regulation, which naturally impairs the ability to effectively engage in state self-regulation during learning. This also confirms that students’ addiction to short videos poses a number of potential risks to learning outcomes [36]. Therefore, teachers need to provide learning strategy prompts and metacognitive feedback to improve the frequency and quality of students’ application of self-regulation abilities. Overall, the SRL model forms a comprehensive and coherent theoretical framework for teaching students how to conduct high-quality learning more effectively.
Moreover, although in this study respondents reported low levels of addictive behavior, the descriptive analysis in fact showed that most of them watched short videos frequently and for long periods of time. This discrepancy might be because they chose responses that align with social norms when filling out the questionnaire, or it could be due to a cognitive gap whereby they do not perceive their usage behavior as addictive. However, the path analysis results indicated the harmful effects of short video addiction on their learning processes. Therefore, from a practical perspective, we suggest that parents and teachers need to pay more attention to students’ use of online media and provide guidance in their use [43]. Additionally, developers of online media, including short videos, need to fulfill their corporate social responsibility by creating better usage mechanisms to prevent overuse, improper use, and harmful consumption by users. Simultaneously, government regulatory bodies need to increase oversight to find the optimal balance between market mechanisms and regulatory mechanisms.
Limitations and future research
Technology addiction, including short video addiction, is a global public health issue. However, the number of studies on short video addiction is not proportionate to the level of attention it receives from governments, schools, society, and parents. Due to the unique algorithmic mechanisms of short video media compared to other social media, the contributing factors may also differ. Despite the increasing influence of short videos, there is a lack of research on Short Video Addiction (SVA). Therefore, more researchers need to focus on the topic of short video addiction. For example, short videos provide users with high emotional value, and the stimulating content can trigger dopamine release in the brain, leading to what is known as “TikTok Brain.” Thus, it is crucial to continuously understand the causes and consequences of short video addiction.
There are several limitations to this study. First, as this study is based on self-reported data, there was no objective assessment of students’ learning abilities conducted by experts. Therefore, future research should consider incorporating objective data for assessment and analysis to more comprehensively explore the impact of short video addiction on learning. Second, the sample for this study was drawn from Chinese university students, which may limit the generalizability of the findings. Thus, future research should expand the sample to include participants from different cultures and age groups to verify the universality of the conclusions. Additionally, this study did not fully consider the motivations for using short videos and the types of content viewed, which may influence the degree of short video addiction and its impact on learning.
Future research should take note of these limitations and address them to enhance the robustness and generalizability of the findings. Beyond the issue of short video addiction, many platform-based online media problems also deserve extensive attention from researchers. Of course, usage is a double-edged concept; the outcomes of effective use vs. problematic use differ significantly, depending on the motivations for use. Therefore, it is important to study the impact of different media use motivations on usage outcomes and effects. At the same time, there is a need to further explore whether the type of content viewed by users has an impact on the amount of time spent watching short videos.
Furthermore, among the various categories of short videos, a new emerging content type is the “short drama series (micro-dramas).” Due to the characteristics of short videos, the short drama industry is developing rapidly. However, aside from high-quality productions, there are many creators who aim to attract viewers and increase video traffic within a short time, often resorting to sensationalism, unethical practices, and vulgar content. In China, many short drama series are considered harmful and influence people’s perceptions and cognition through the unique nature of short videos. Future research could focus on comparing high-quality and low-quality short drama series and their effects on viewers.
This study examined the role of short video addiction in learning based on SRL theory. The results indicate that short video addiction is negatively correlated with three types of SRL strategies (preparatory, performance, and appraisal strategies), while these strategies are positively correlated with learning well-being. Additionally, short video addiction is indirectly negatively correlated with well-being. From the students’ perspective, all these strategies can be adaptive as long as they successfully restore well-being. The use of short videos is not inherently right or wrong, but excessive or problematic use needs attention and improvement. These findings underscore the importance for parents, educators, student users, and short video developers to understand the appropriate use of short videos. Early identification of problematic usage patterns in short videos may be a crucial factor in preventing declines in psychological health and learning behaviors among student users.
Acknowledgement: The authors thank research participants for their participation in this study.
Funding Statement: This work was supported by Fundamental Research Funds for the Central Universities in China (Grant Number: 2022NTSS52), First-Class Education Discipline Development of Beijing Normal University: Excellence Action Project (Grant Number: YLXKPY-XSDW202408), and Beijing Institute of Education 2024 Youth Research Projects “Research on the Transformation of Training Aimed at Improving the Work of School Principals in the Capital” (Grant Number: QZ2024-02).
Author Contributions: Jian-Hong Ye: Conceptualization, Data curation, Formal analysis, Funding acquisition, Investigation, Methodology, Writing—original draft, Writing—review & editing. Yuting Cui: Formal analysis, Funding acquisition, Investigation, Methodology, Writing—original draft, Writing—review & editing. Li Wang: Project administration, Validation, Writing—original draft. Jhen-Ni Ye: Project administration, Validation, Writing—review & editing. All authors reviewed the results and approved the final version of the manuscript.
Availability of Data and Materials: The datasets generated during and/or analyzed during the current study are available from the corresponding author on reasonable request.
Ethics Approval: This study was approved by the Academic Ethics Committee of Hainan Vocational University of Science and Technology (Approval No. HKD-2024-3), and informed consent was obtained from all participants.
Conflicts of Interest: The authors declare that they have no conflicts of interest to report regarding the present study.
References
1. Ye JH, He Z, Yang X, Lee YS, Nong W, Ye JN, et al. Predicting the learning avoidance motivation, learning commitment, and silent classroom behavior of Chinese vocational college students caused by short video addiction. Healthcare. 2023;11(7):985. doi:10.3390/healthcare11070985. [Google Scholar] [PubMed] [CrossRef]
2. Liu Y, Ni X, Niu G. Perceived stress and short-form video application addiction: a moderated mediation model. Front Psychol. 2021;12:747656. doi:10.3389/fpsyg.2021.747656. [Google Scholar] [PubMed] [CrossRef]
3. Xie J, Xu X, Tan Y, Wu D, Huang H. The effect of short-form video addiction on undergraduates’ academic procrastination: a moderated mediation model. Front Psychol. 2023;14:1298361. doi:10.3389/fpsyg.2023.1298361. [Google Scholar] [PubMed] [CrossRef]
4. Hernández C, Ottenberger DR, Moessner M, Crosby RD, Ditzen B. Depressed and swiping my problems for later: the moderation effect between procrastination and depressive symptomatology on internet addiction. Comput Hum Behav. 2019;97(7):1–9. doi:10.1016/j.chb.2019.02.027. [Google Scholar] [CrossRef]
5. Zhang MX, Wu AM. Effects of smartphone addiction on sleep quality among Chinese university students: the mediating role of self-regulation and bedtime procrastination. Addict Behav. 2020;111(1):106552. doi:10.1016/j.addbeh.2020.106552. [Google Scholar] [PubMed] [CrossRef]
6. Saad MAE, Khalifa AG. Modeling self-regulated learning: the mediating role in the relationship between academic procrastination and problematic smartphone use among third year-middle school learning disabled students. Electron J Res Educ Psychol. 2020;18(52):507–22. doi:10.25115/ejrep.v18i52.2987. [Google Scholar] [CrossRef]
7. Iyiola ET, Azikiwe JC, Omolayo BO. Undergraduates self-regulated learning: the place of social media addiction and learning strategies. Indones J Curric Educ Technol Stud. 2021;9(1):25–33. doi:10.15294/ijcets.v9i1.43726. [Google Scholar] [CrossRef]
8. Booth A, Hennessy E, Doyle O. Self-regulation: learning across disciplines. J Child Fam Stud. 2018;27(12):3767–81. doi:10.1007/s10826-018-1202-5. [Google Scholar] [CrossRef]
9. Schumacher C, Ifenthaler D. Investigating prompts for supporting students’ self-regulation—a remaining challenge for learning analytics approaches? Internet High Educ. 2021;49(1):100791. doi:10.1016/j.iheduc.2020.100791. [Google Scholar] [CrossRef]
10. Liu F, Xu Y, Yang T, Li Z, Dong Y, Chen L, et al. The mediating roles of time management and learning strategic approach in the relationship between smartphone addiction and academic procrastination. Psychol Res Behav Manag. 2022;15:2639–48. doi:10.2147/PRBM.S373095. [Google Scholar] [PubMed] [CrossRef]
11. Du J, Hew KF, Liu L. What can online traces tell us about students’ self-regulated learning? A systematic review of online trace data analysis. Comput Educ. 2023;201(5):104828. doi:10.1016/j.compedu.2023.104828. [Google Scholar] [CrossRef]
12. Watson D, Tregaskis O, Gedikli C, Vaughn O, Semkina A. Well-being through learning: a systematic review of learning interventions in the workplace and their impact on well-being. Eur J Work Organ Psychol. 2018;27(2):247–68. doi:10.1080/1359432X.2018.1435529. [Google Scholar] [CrossRef]
13. Akhtar P, Ma L, Waqas A, Naveed S, Li Y, Rahman A, et al. Prevalence of depression among university students in low and middle income countries (LMICs): a systematic review and meta-analysis. J Affect Disord. 2020;274(3):911–9. doi:10.1016/j.jad.2020.03.183. [Google Scholar] [CrossRef]
14. Korhonen J, Linnanmäki K, Aunio P. Learning difficulties, academic well-being and educational dropout: a person-centred approach. Learn Individ Differ. 2014;31(1):1–10. doi:10.1016/j.lindif.2013.12.011. [Google Scholar] [CrossRef]
15. Kleinkorres R, Stang-Rabrig J, McElvany N. Comparing parental and school pressure in terms of their relations with students’ well-being. Learn Individ Differ. 2023;104(2):102288. doi:10.1016/j.lindif.2023.102288. [Google Scholar] [CrossRef]
16. Kross E, Verduyn P, Sheppes G, Costello CK, Jonides J, Ybarra O. Social media and well-being: pitfalls, progress, and next steps. Trends Cogn Sci. 2021;25(1):55–66. doi:10.1016/j.tics.2020.10.005. [Google Scholar] [CrossRef]
17. Ye JH, Chen MY, Wu YF. The causes, counseling, and prevention strategies for maladaptive and deviant behaviors in schools. Behav Sci. 2024;14(2):118. doi:10.3390/bs14020118. [Google Scholar] [CrossRef]
18. Rodríguez S, González-Suárez R, Vieites T, Piñeiro I, Díaz-Freire FM. Self-regulation and students well-being: a systematic review 2010–2020. Sustainability. 2022;14(4):2346. doi:10.3390/su14042346. [Google Scholar] [CrossRef]
19. Zeidner M, Stoeger H. Self-regulated learning (SRL): a guide for the perplexed. High Abil Stud. 2019;30(1–2):9–51. doi:10.1080/13598139.2019.1589369. [Google Scholar] [CrossRef]
20. Panadero E. A review of self-regulated learning: six models and four directions for research. Front Psychol. 2017;8:422. doi:10.3389/fpsyg.2017.00422. [Google Scholar] [CrossRef]
21. Pintrich PR. The role of goal orientation in self-regulated learning. In: Boekaerts M, Pintrich PR, Zeidner M, editors. Handbook of self-regulation. San Diego, CA: Academic Press; 2000. p. 452–502. [Google Scholar]
22. Zimmerman BJ. Chapter 2-attaining self-regulation: a social cognitive perspective. In: Boekaerts M, Zeidner M, Zeidner PR, editors. Handbook of self-regulation. San Diego, CA: Academic Press; 2000. p. 13–39. doi:10.1016/B978-012109890-2/50031-7. [Google Scholar] [CrossRef]
23. Adam NL, Alzahri FB, Soh SC, Bakar NA, Kamal NA. Self-regulated learning and online learning: a systematic review. In: Zaman HB, Robinson P, Smeaton AF, Shih TK, Velastin S, Terutoshi T, Jaafar A, Ali NM, editors. Advances in visual informatics. Cham: Springer; 2017. p. 143–54. [Google Scholar]
24. Liu X, He W, Zhao L, Hong JC. Gender differences in self-regulated online learning during the COVID-19 lockdown. Front Psychol. 2021;12:752131. doi:10.3389/fpsyg.2021.752131. [Google Scholar] [CrossRef]
25. Seufert T. The interplay between self-regulation in learning and cognitive load. Educ Res Rev. 2018;24(6):116–29. doi:10.1016/j.edurev.2018.03.004. [Google Scholar] [CrossRef]
26. Boekaerts M, Corno L. Self-regulation in the classroom: a perspective on assessment and intervention. Appl Psychol. 2005;54(2):199–231. [Google Scholar]
27. O’Toole L. Understanding individual patterns of learning: implications for the well-being of students. Eur J Educ. 2008;43(1):71–86. doi:10.1111/j.1465-3435.2007.00335.x. [Google Scholar] [CrossRef]
28. Fomina T, Burmistrova-Savenkova A, Morosanova V. Self-regulation and psychological well-being in early adolescence: a two-wave longitudinal study. Behav Sci. 2020;10(3):67. doi:10.3390/bs10030067. [Google Scholar] [CrossRef]
29. Wang H, Yang J, Li P. How and when goal-oriented self-regulation improves college students’ well-being: a weekly diary study. Curr Psychol. 2022;41(11):7532–43. doi:10.1007/s12144-020-01288-w. [Google Scholar] [CrossRef]
30. Evli M, Şimşek N. The effect of COVID-19 uncertainty on internet addiction, happiness and life satisfaction in adolescents. Arch Psychiatr Nurs. 2022;41(3):20–6. doi:10.1016/j.apnu.2022.07.008. [Google Scholar] [CrossRef]
31. Longstreet P, Brooks S, Gonzalez ES. Internet addiction: when the positive emotions are not so positive. Technol Soc. 2019;57:76–85. doi:10.1016/j.techsoc.2018.12.004. [Google Scholar] [CrossRef]
32. Çiftci N, Yıldız M. The relationship between social media addiction, happiness, and life satisfaction in adults: analysis with machine learning approach. Int J Ment Health Addict. 2023;21(5):3500–16. doi:10.1007/s11469-023-01118-7. [Google Scholar] [CrossRef]
33. Nong W, He Z, Ye JH, Wu YF, Wu YT, Ye JN, et al. The relationship between short video flow, addiction, serendipity, and achievement motivation among Chinese vocational school students: the post-epidemic era context. Healthcare. 2023;11(4):462. doi:10.3390/healthcare11040462. [Google Scholar] [CrossRef]
34. Wang X, Wuji S, Liu Y, Luo R, Qiu C. Study on the impact of recommendation algorithms on user perceived stress and health management behaviour in short video platforms. Inf Process Manag. 2024;61(3):103674. doi:10.1016/j.ipm.2024.103674. [Google Scholar] [CrossRef]
35. Longobardi C, Settanni M, Fabris MA, Marengo D. Follow or be followed: exploring the links between Instagram popularity, social media addiction, cyber victimization, and subjective happiness in Italian adolescents. Child Youth Serv Rev. 2020;113(2):104955. doi:10.1016/j.childyouth.2020.104955. [Google Scholar] [CrossRef]
36. Ye JH, Wu YT, Wu YF, Chen MY, Ye JN. Effects of short video addiction on the motivation and well-being of Chinese vocational college students. Front Public Health. 2022;10:847672. doi:10.3389/fpubh.2022.847672. [Google Scholar] [CrossRef]
37. Hong JC, Lee YF, Ye JH. Procrastination predicts online self-regulated learning and online learning ineffectiveness on the COVID-19 lockdown. Pers Individ Differ. 2021;174(7):110673. doi:10.1016/j.paid.2021.110673. [Google Scholar] [CrossRef]
38. Green SB, Salkind N. Using SPSS for windows and macintosh: analyzing and understanding data. 4th ed. Englewood Cliffs, NJ: Prentice-Hall; 2004. [Google Scholar]
39. Hair JF, Ringle CM, Sarstedt M. PLS-SEM: indeed a silver bullet. J Mark Theory Pract. 2011;19(2):139–52. doi:10.2753/MTP1069-6679190202. [Google Scholar] [CrossRef]
40. Hair JF, Black WC, Babin BJ, Anderson RE, Tatham RL. Multivariate data analysis. 8th ed. London, UK: Cengage; 2019. [Google Scholar]
41. Awang Z. SEM made simple, a gentle approach to learning structural equation modeling. Bangi, Selangor, Malaysia: MPWS Rich Publication; 2015. [Google Scholar]
42. Preacher KJ, Hayes AF. Asymptotic and resampling strategies for assessing and comparing indirect effects in multiple mediator models. Behav Res Methods. 2008;40(3):879–91. doi:10.3758/BRM.40.3.879. [Google Scholar] [CrossRef]
43. Sun R, Sun G, Ye JH. The effect of online game addiction on reduced academic achievement motivation among Chinese college students: the mediating role of learning engagement. Front Psychol. 2023;14:1185353. doi:10.3389/fpsyg.2023.1185353. [Google Scholar] [CrossRef]
Cite This Article
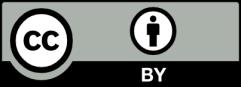
This work is licensed under a Creative Commons Attribution 4.0 International License , which permits unrestricted use, distribution, and reproduction in any medium, provided the original work is properly cited.