Open Access
ARTICLE
Association between Occupational Change Trajectories and Mental Health: Results from the Korean Longitudinal Study of Aging
1 Institute for Digital Life Convergence, Dankook University, Cheonan, 31116, South Korea
2 Department of Public Health, General Graduate School of Dankook University, Cheonan, 31116, South Korea
3 Department of Health Administration, College of Health Science, Dankook University, Cheonan, 31116, South Korea
* Corresponding Author: Jae Hyun Kim. Email:
International Journal of Mental Health Promotion 2023, 25(4), 579-594. https://doi.org/10.32604/ijmhp.2022.027498
Received 01 November 2022; Accepted 20 December 2022; Issue published 01 March 2023
Abstract
Objectives: This study was to longitudinally investigate the association between occupational change trajectories and mental health in the Korean population aged 45 years and older from the Korean Longitudinal Study of Aging (KLoSA). Methods: After excluding missing values, the data of 6,224 participants from the first to eighth waves of the KLoSA were analyzed using t-test, Analysis of variance (ANOVA), Group-based Trajectory Model (GBTM) from 1–5th KLoSA and Time-Lagged Generalized estimating equation (GEE) model from 5–8th KLoSA to analyze the association between occupational change trajectories and mental health in the Korean population aged 45 years and older. Results: An analysis of the association between occupational change trajectories and mental health demonstrated that, among all age group, the “sustained in unemployment” group had a worse MMSE and CESD score compared to “sustained WC” group. unlike the under-54 age group, those aged ≥55 years demonstrated reductions in the MMSE and CESD scores in the sustained unemployment and the changed working condition from Standard BC to Unemployment groups, whereas those who underwent a change from the Standard WC to unemployment group only demonstrated a reduction in MMSE scores. Conclusions: There was a close relationship between occupational change trajectories and mental health. Therefore, this study can serve as the foundation for policies and institutional measures to manage the deteriorate mental health in the late middle-aged and older population.Keywords
Nomenclature
ANOVA | Analysis of Variance |
WC | White Collar |
BC | Blue Collar |
GBTM | Group-Based Trajectory Model |
GEE | Generalized Estimating Equation |
KLoSA | Korean Longitudinal Study of Aging |
OECD | Organization for Economic Cooperation and Development |
WHO | World Health Organization |
According to the “Future Population Estimation” published by Statistics Korea, the proportion of older adults aged ≥65 will be 20.4% by 2025, resulting in a super-aged society [1]. Individuals in an ageing society may experience various problems such as deteriorating health and death of a spouse [2]. Furthermore, older adulthood is known as the stage of life in which individuals undergo occupational changes and retirement, which make up the largest proportion of the life cycle [3]. A long-term, regular working condition is defined as the most basic activity for acquiring economic satisfaction and a sense of belonging and self-realization by maintaining social networks and positively maintaining one’s physical and mental health [4].
However, according to reports from the Organization for Economic Cooperation and Development (OECD) [5], the number of years employed, a measure of employment stability, in Korea demonstrated serious employment instability at 5.6 years, compared to the OECD average of 9.5 years. Moreover, the average retirement age in Korea was reported to be 55 years, which is earlier than that in other countries [6], and most retired individuals faced involuntary retirement, causing them to find secondary careers in occupations of lower status or in other industries [7,8].
As such, consequences of changes in working condition, such as reductions in income, social welfare, and legal qualifications, can impact mental health [8,9]. The development of mental illness into severe mental disorders is an important social problem as the consequential series of diseases can lead to an increase in the socioeconomic burden [10]. According to reports from the World Health Organization (WHO), the number of individuals with mental health issues such as dementia or depression exceeded 970 million in 2019 [11]. In Korea, 10.2% of the over-65 years population was diagnosed with dementia [12], of which most individuals suffered from dementia and depression simultaneously, leading to severe mental disorders. Thus, active research to identify factors that deteriorate mental health at the national level to minimize social and economic deficits is being conducted [12,13].
According to a previous study that analyzed the correlation between changes in occupational activities and depression among Korean adults, depression was more common in the group that experienced changes in working condition, compared to that in those with sustained working condition [14]. In particular, depression levels were highest in the group that experienced unemployment [14]. Furthermore, in a previous European study that analyzed the correlation between changes in employment and mental disorders among 2,732,426 Italian employees, individuals in groups that underwent a positive change in employment had a reduced incidence of mental disorders, whereas those who underwent a negative change in employment, such as transitioning from a permanent to a contract position, demonstrated a higher incidence of dementia, in addition to a greater use of antipsychotic drugs [9].
Although some studies have presented findings on the effect of changes in working condition on mental health, most studies were conducted in countries with higher employment stability than Korea [5,9]. Furthermore, most studies in Korea were based on a small sample or fail to describe the correlation between changes in working condition and mental health [14]. In terms of change in working condition, most previous studies examined only small changes between permanent employment, contract employment, and unemployment. Finally, despite the short duration of occupational activity observed in countries that have high employment instability such as South Korea, most studies observe annual working condition changes, and there is a paucity of longitudinal studies examining working condition changes and mental health among middle-aged and older adults.
With regards to the current state of literature, this study aims to analyze the association between occupational change trajectories and mental health among middle-aged or older adults using MMSE and CESD measures, based on the 1st–8th KLoSA. Furthermore, based on the previous finding that the rate of occupational change increases from approximately 55 years of age [6], we analyzed the correlation between occupational change trajectories and mental disorders in detail and present the results as preliminary data for policy and institutional measures to prevent the exacerbation of dementia and depression within cohorts with occupational change trajectories that are vulnerable to mental health.
2.1 Data Source & Study Population
The data used for the analyses were derived from the Korean Longitudinal Study of Aging (KLoSA) from 2006 to 2020. As a study that possesses both the strengths of cross-sectional and time series data, the KLoSA was conducted by repeatedly surveying identical content for the same respondents every year. Thus, all variables surveyed by the KLoSA were repeatedly measured from the first to fourth waves to collect observation cases at multiple points in time. This biennial survey involves multistage stratified sampling based on geographical areas and housing types across Korea. Participants were selected randomly using multistage stratified probability sampling to create a nationally representative sample of community-dwelling Koreans aged 45 and older. Participant selection was performed by the Korea Labor Institute, including individuals from both urban and rural areas. In case of refusal to participate, another participant was selected from an additional, similar sample from the same district.
In the first survey in 2006, 10,254 individuals from 6,171 households (1.7 per household) were interviewed. The second survey, in 2008, followed up with 8,875 participants, who represented 86.6% of the original panel. The third survey, in 2010, followed up with 8,229 participants, who represented 81.7% of the original panel. The fourth survey, in 2012, followed up with 7,813 participants, who represented 80.1% of the original panel. The fifth survey, in 2014, followed up with 8,387 participants (including 920 new participants), who represented 80.4% of the original panel. The sixth survey, in 2016, followed up with 7,893 participants (including 878 new participants), who represented 79.6% of the original panel. The seventh survey, in 2018, followed up with 7,491 participants (including 817 new participants) who represented 78.8% of the original panel. Finally, the eighth survey, in 2020, followed up with 7,000 participants (including 786 new participants) who represented 78.1% of the original panel.
To investigate the association between occupational change trajectories and mental health, among 10,254 individuals who were registered in the 1st KLoSA, we excluded 3,750 individuals who lacked information on type of occupation during 1st to 5th wave (Because they did not respond to the questionnaire on employment status), 271 individuals who lacked information on dependent variables during 5th to 8th wave (Because they did not responded to the questionnaire on MMSE or CESD score) and 9 missing values for control variables during 5th to 8th wave.
Finally, we included 6,224 participants during the observation period from the 1st to 8th KLoSA. Additionally, we excluded new participants in 5∼8th KLoSA to verify consistent occupational change trajectories. Fig. 1 shows the inclusion and exclusion process of participants.
Figure 1: Flow chart for sample selection
2.2.1 Occupational Change Trajectory
Prior to identifying the occupational change trajectory, we established seven types of occupations. type of occupations was assessed by the response to the following question at first wave (1st KLoSA): “Are you currently working which includes wage work, self-employment work and etc.” if respondents responded ‘No’, then they were classified as ‘unemployment’ group. or if respondents responded ‘Yes’, then they were additionally received following questions: “What form of employment are you employed at work?” and “what do you usually do at work?”. Finally, type of occupation variables was categorized into seven groups: Standard white collar (WC), Self-employment WC, Non-standard WC, Standard blue collar (BC), Self-employment BC, Non-standard BC, and unemployment.
Occupational change trajectories were assessed by using Group-Based Trajectory Model (GBTM) based on the type of occupations and categorized into 5 groups: Sustained in self-employment WC (Group 1), Sustained in unemployment (Group 2), Sustained in self-employment BC (Group 3), Changed standard WC to unemployment (Group 4) and changed standard BC to unemployment (Group 5).
2.3.1 Mini Mental State Examination (MMSE)
The Korean Mini-Mental State Examination (K-MMSE) included 11 items in 7 categories of cognitive functions, including orientation for time and place, registration, attention & calculation, recall, language, and visual construction [15,16]. The total score of the measure ranges from 0 to 30; higher the score, better the cognitive function. The validity of the K-MMSE was reported elsewhere [15].
2.3.2 Center for Epidemiologic Studies Depression Scale (CESD)
The CESD was created in 1977 by Radloff [17]. The scale is well known and remains one of the most widely used instruments in the field of psychiatric epidemiology [18,19]. We used the CESD-10 Korean edition for measuring depressive symptoms defined by the American Psychiatric Association’ Diagnostic and Statistical Manual (DSM-IV). The total score of the measure ranges from 0 to 30; lower the score, better the depression symptoms.
2.4.1 Socioeconomic and Demographic Factors
‘Age’ group was divided into four categories: 45–54, 55–64, 65–74 and 75 or older. ‘Sex’ group was divided into two categories: male, and female. ‘Marital statuses’ were divided into two categories: married and single (including separated, divorced). ‘Educational level’ was categorized into four groups: elementary school or lower, middle school, high school, and college or higher. ‘Residential region’ was categorized as urban (Seoul, Daejeon, Daegu, Busan, Incheon, Kwangju, or Ulsan), and rural (not classified as a city). ‘Income Level’ was divided into four categories: low, middle-low, middle-high, and high.
2.4.2 Health Status and Behavioral Factors
‘The number of chronic diseases (Hypertension, diabetes, cancer, chronic obstructive pulmonary disease, liver disease, cardiovascular disease, cerebrovascular disease and arthritis)’ was divided into four groups: 0, 1, 2 and 3 or more. ‘ADL & IADL’ was divided into three groups: Normal, Mild, and Impaired. ‘Smoke statuses was categorized into two groups: Never and Ever. ‘Alcohol statuses also was divided into two groups: Never and ever. Also, we added the lagged MMSE and CESD score as a covariate to control for cognitive function scores in the previous survey.
2.5 Analytical Approach and Statistics
The t-test, Analysis of variance (ANOVA), GBTM and Time-Lagged Generalized Estimating Equation (GEE) model were used to investigate the association between occupational change trajectories and mental health in the late middle-aged and older Korean population.
Trajectory modeling provides a method by which we can develop a probable representation of unobserved group classification and group differences, based on observed information and user-specified constraints. Once the occupational change trajectories were derived from the trajectory modeling for identifying homogeneous subpopulations within the larger heterogeneous population based on the Bayesian information criterion (BIC) value [Appendix 1] [20], average posterior probability of each trajectory group (>0.85) [Appendix 2] [21] and inclusion of at least 2% of the participants within each trajectory group [22] to select distinguishable trajectories and for describing longitudinal change within each unobserved sub-population, the trajectory classes were then coded into a series of CNORM model to examine the relationship between the occupational change trajectories and mental health using the time-lagged GEE regression models.
Time-Lagged GEE analyses with unstructured correlation structure were conducted and controlled for confounders to provide estimates for MMSE and CESD scores. GEE analysis was performed for correlated data within a subject, such as repeated panel data. This approach was used for extending the generalized linear model using quasi-likelihood estimation, which identified the effect occupational change trajectories and other covariates on mental health. In GEE, proc genmod was used, with distribution normal. GEE model, the clustered approaches to analysis, controls for the characteristics of individuals that change over time, such as confounding variables. The clustering can be expressed in terms of correlation among the measurements on units within the same cluster. Covariates of interest from all subjects were added to the model to determine their effects on the probability of reporting mental health. To determine whether the probability of mental health changed over time, we included time (year) in the model as a categorical covariate.
Considering the measurement times of in trajectory analyses, sufficient sample size, and follow-up time as long as possible, we set 5th KLoSA as the baseline. The data of waves 1–5th KLoSA (2006–2014) were used to explore occupational change trajectories using GBTM method, and the data of waves 5–8th KLoSA (2014–2020) were adopted to investigate the association between occupational change trajectories and mental health using GEE model. Fig. 2 displays the timeline and procedure of this study.
Figure 2: Study flow chart
For all analyses, the criterion for statistical significance was p < 0.05, two-tailed. They were conducted using the SAS statistical software package, version 9.4 (SAS Institute Inc., Cary, NC, USA).
3.1 Trajectory Class of Occupational Change over Time
Fig. 3 shows the occupational change trajectory during eight years (1st to 5th Wave). The sustained in WC group was a trajectory that continuously in the WC (515 participants [8.3%], and the sustained in unemployment group was a trajectory that continuously unemployed (3,844 participants [61.8%]). The sustained in self-employment BC group was one that continuously worked in self-employment BC (1,200 participants [19.3%]), and both changed WC to unemployment and changed BC to unemployment were groups whose trajectories of work condition changed to job loss (Changed WC to unemployment: 239 participants [3.8%], Changed BC to unemployment: 426 participants [6.8%]).
Figure 3: Occupational change trajectories of all age group during 1–5th KLoSA
The baseline characteristics of the occupational change trajectory are shown in Table 1. The MMSE and CESD score of the 6,224 participants were 25.06 point (SD: 5.66) and 7.08 point (SD: 4.92), respectively.
Regarding the occupational change trajectory, 8.3% (n = 515) were classified as the “sustained in WC” and the resulting MMSE and CESD score were 28.00 point (SD: 2.72) and 5.82(SD: 4.28). Approximately 61.8% (n = 3,844) of the participants were classified as “Sustained in unemployment” and their MMSE and CESD score were 23.78 point (SD: 6.28) and 7.55 point (SD: 5.20) respectively. The number of participants who classified as the “Sustained in BC” group was 19.3% (n = 1,200) and their MMSE and CESD score were 27.16 point (SD: 3.37) and 6.30 point (SD: 4.05). In terms of changes in employment status groups, 3.8% (n = 239) were classified as the “changed WC to unemployment” and the resulting MMSE and CESD score were 27.64 point (SD: 3.11) and 6.45 point (SD: 4.63), respectively. The number of participants classified as the “changed BC to unemployment” group was 6.8% (n = 426) and their MMSE and CESD score were 25.72 point (SD: 4.94) and 6.81 point (SD: 4.80).
3.3 Adjusted Association Between Occupational Change Trajectories and Mental Health
Table 2 shows the results of the panel data analysis using Time-lagged GEE model, which investigated the association between occupational change trajectories and mental health. The “sustained in unemployment” group had a lower MMSE score (B: −0.19, p-value: 0.0038) and had a higher CESD score (B: 0.28, p-value: 0.0147) compared to “sustained WC” group.
3.4 Relationship between Occupational Change Trajectories and Mental Health by Age
Table 3 shows the results of the subgroup analysis stratified by age. we found that in model 1 which included under 54 years participants, there were not find out the association between occupational trajectories and mental health. However, In model 2 which included over 55 years participants, “Sustained in unemployment” group was worse than “Sustained in WC” group in MMSE score (B: −0.41, p-value: 0.001) and CESD score (B: 0.56, p-value: 0.003), and “Changed BC to unemployment” group was also worse than reference group in MMSE score (B: −0.54, p-value: 0.001) and CESD score (B: 0.69, p-value: 0.002). but “Changed WC to unemployment” group was only worse MMSE score than reference group (B: −0.70, p-value: 0.000).
The labor market in Korea experienced a drastic change in the once-stable employment system due to the International Monetary Fund (IMF) economic crisis in 1997, resulting in reliance on outsourcing to reduce labor costs in large enterprises [23]. Moreover, the implementation of IT resulted in the reduction of administrative or managerial staff, increasing the rate of contract-based employment [24]. Thus, job insecurity is increasing among workers, whereas lay-offs and the implementation of the “voluntary early retirement system” have worsened job insecurity, naturally leading to the increase in the incidence and severity of mental disorders [25].
Therefore, in this study, we investigated the association between occupational change trajectories and mental health using MMSE and CESD measures. This study showed a significant relationship between occupational change and mental health after controlling for other factors based on a large population-based sample with 14-year follow-up database. These findings suggest an important role of occupation change in mental health aspects.
The major findings of our study are as follows: First, sustained in unemployment group had a lower MMSE score and had a higher CESD score compared to “sustained WC” group. Second, under 54 years participants, there were not find out the association between occupational trajectories and mental health. Third, unlike the under-54 age group, those aged ≥55 years demonstrated reductions in the MMSE and CESD scores in the Sustained Unemployment and the changed working condition from Standard BC to Unemployment groups, whereas those who underwent a change from the Standard WC to unemployment group only demonstrated a reduction in MMSE scores.
The sustained in unemployment group demonstrated lower MMSE and CESD scores compared to the sustained in WC group, supporting the findings of previous studies [26,27]. According to an American study, the Unemployment group experienced greater levels of stress from employment anxiety and limited accessibility to financial and social resources compared to the Standard Occupation group, demonstrating an incidence of depression of 1.66 OR higher [26]. Meanwhile, in a study on 6,938 middle-aged and older adults, those in the unemployment group experienced reductions in social support, loss of a sense of belonging, and the lack of familial protection as compared to the Standard Occupation group, which led to an overall deterioration in mental health, including dementia and depression [27].
The findings of this study posit that occupational change trajectories and mental health were correlated among workers aged ≥55, unlike the under-54 group, and that Unemployed or Changed working condition groups suffered from deteriorating mental health as compared to Sustained WC groups, supporting previous research findings [28–32]. Indeed, a previous study that analyzed the correlation between occupational change trajectories and mental health by age in 6,096 Canadian adults proposed that in younger age groups, who had less dependents and expenses and higher distress resistance, changes in employment or the loss of a job did not translate into a deterioration of mental health such as depression or dementia [28]. However, older groups with higher amounts of expenses or dependents often experienced financial stress and depression and had less “distress resistance,” leading to a deterioration in mental health. Thus, a stronger correlation existed between occupational change and retirement and the deterioration of mental health with increasing age [28]. An American study on 9,538 adults aged ≥55 years demonstrated that employment instability occurring prior to unemployment during the occupational change phase caused an increase in blood pressure, fluctuations in weight, and a negative subjective perception of health, which ultimately led to deteriorations in not only physical health, such as stroke or cardiovascular disease, but also in mental health. The degree of employment instability increased with time, exacerbating the etiology of psychological stress, sleep disturbances, hypertension, obesity, and cardiovascular disease and increasing the risk of their development into severe mental disorders such as dementia [29,30]. Furthermore, according to other studies, cognitive function in groups that underwent a change from WC to unemployment reduced as much as 38% faster than that in the Sustained WC groups [31]. In particular, occupational activity among middle-aged to older adults had the effect of “mental exercise” in itself; therefore, in countries like Korea, where the age of retirement is lower, cognitive function demonstrated a more rapid deterioration [32].
Furthermore, the study result that the group that changed from Standard BC to Unemployment was more vulnerable to depression than the group that changed from Standard WC to Unemployment also coincided with the prior studies [33–37]. Depression is not only caused by individual characteristics but is also affected by the work environment of individuals. BC groups, in comparison to WC groups, tend to have poorer working conditions [33], with higher rates of cigarette smoking and alcohol consumption and low engagement in health-promoting activities, and these individuals are often in an unhealthy state accompanied by hypertension, hyperlipidemia, and depression even after retirement [34–36]. Furthermore, in a study that analyzed the difference in the incidence of depression between WC and BC retirees among European individuals aged ≥65 years, BC retirees demonstrated a significantly higher incidence of depression compared to their WC counterparts due to chronic diseases caused by their work environment, lack of awareness regarding health management, and low levels of physical activity [37].
Occupational change in middle-aged to older adults leads to the deterioration of mental health. Thus, despite the need for the management of mental health, the usage rate of mental health services in Korea was 17.5%, which was significantly lower than the 32.9% observed in the United States [38]. Moreover, a mental health promotion program needs to be developed for groups that underwent unemployment and occupational change, whose mental health was found to be the most vulnerable [38]. In particular, the need for a mental health promotion program for groups that underwent unemployment and occupational change has not been reflected in “Health Plan 2030,” which is planned and carried out every 10 years by the Ministry of Health and Welfare of South Korea for the promotion of mental health among Korean citizens [39]. Thus, based on our findings, a mental health promotion program may might be developed to manage the deterioration of mental health and alleviate the incidence of mental disorders caused by occupational change among workers aged ≥55 years.
There is a number of strengths and limitations of this study. A major strength of the current study is that the participants were assessed and followed for 14 years. In addition, the study obtained a large sample size, so the results can be generalized to adults aged 45 years and older within the Korea population. Furthermore, unlike previous studies focusing on the narrow range of types of occupation and the occupational change over a short period of time, in this study, analysis was conducted using various type of occupation and sufficient time for occupational change trajectories.
Nevertheless, several limitations of our study warrant mention. First, this study has a subjective bias due to the KLoSA used in the analysis mixed with the opinions of the respondents. Second, we analyzed longitudinal data, but the results may reflect the inverse causal relationship between occupational change trajectories and mental health. In particular, despite limitation of KLoSA, we cannot find out whether the change in occupational trajectories occur before the deterioration of mental health. Third, although our trajectory model was fitted based on assigned trajectories, we did not fully consider the uncertainty of class membership of each participant, which signifies that the variance estimates from this model are likely to be underestimated. Finally, we were unable to adjust for unknown confounding factors highly associated with the investigated relationship.
Despite these limitations, our study is predictive of future mental health in middle aged and older participants. These findings demonstrate the need for more studies that examine the mechanisms of this association and explore the potential of occupational change and mental health as a modifiable risk factor for healthy aging in Asian populations.
Based on the basic survey of the l∼8th KLoSA carried out on adults aged 45 years and older, we investigated the association between occupational change trajectories and mental health. Among the age group 55 years and older, the group sustaining occupation was not associated with deterioration of mental health, but sustained in unemployment group and occupational change group were associated with deterioration of mental health, In particular, in the trajectory group changing from BC to unemployment, both Cognitive and mental health were deteriorated.
Therefore, this study can serve as the foundation for policies and institutional measures to manage the deteriorate mental health in the late middle-aged and older Korean population. Also, public health professionals and policymakers should consider our findings when developing optimal health policies for the prevention of mental health deterioration.
Funding Statement: The authors received no specific funding for this study.
Author Contributions: JM Yang designed this study, performed statistical analysis, drafted and completed the manuscript. HJ Lee contributed to the concept and design of the study, and provided statistical expertise and interpretation. JH Kim conceived, designed and directed this study.
Conflicts of Interest: The authors declare that they have no conflicts of interest to report regarding the present study.
References
1. Korean Statistics Information Service (2022). Estimated future population. Daejeon: Statistics Korea. [Google Scholar]
2. Choi, N. G. (1996). Older persons who move: Reasons and health consequences. Journal of Applied Gerontology, 15(3), 325–344. DOI 10.1177/073346489601500304. [Google Scholar] [CrossRef]
3. Hayward, M. D., Friedman, S., Chen, H. (1998). Career trajectories and older men’s retirement. The Journals of Gerontology Series B: Psychological Sciences and Social Sciences, 53(2), S91–S103. DOI 10.1093/geronb/53B.2.S91. [Google Scholar] [CrossRef]
4. Au, D. W. H., Crossley, T. F., Schellhorn, M. (2005). The effect of health changes and long-term health on the work activity of older Canadians. Health Economics, 14(10), 999–1018. DOI 10.1002/(ISSN)1099-1050. [Google Scholar] [CrossRef]
5. Organization for Economic Cooperation and Development (OECD)(2020). Employment by job tenure intervals: Average tenure. [Google Scholar]
6. Lee, T., Cho, J. (2022). Unintended consequences of the retirement-age extension in South Korea. Asian-Pacific Economic Literature, 36(1), 105–125. DOI 10.1111/apel.12351. [Google Scholar] [CrossRef]
7. Lee, A. Y., Go, K. P. (2018). The effect of retirement on mental health and cognitive function. Sejong: Korea Institute for Health and Social Affairs. [Google Scholar]
8. Herzog, A., House, J. S., Morgan, J. N. (1991). Relation of work and retirement to health and well-being in older age. Psychology and Aging, 6(2), 202. DOI 10.1037/0882-7974.6.2.202. [Google Scholar] [CrossRef]
9. Moscone, F., Tosetti, E., Vittadini, G. (2016). The impact of precarious employment on mental health: The case of Italy. Social Science & Medicine, 158, 86–95. DOI 10.1016/j.socscimed.2016.03.008. [Google Scholar] [CrossRef]
10. Whiteford, H. A., Ferrari, A. J., Degenhardt, L., Feigin, V., Vos, T. (2015). The global burden of mental, neurological and substance use disorders: An analysis from the global burden of disease study 2010. PLoS one, 10(2), e0116820. DOI 10.1371/journal.pone.0116820. [Google Scholar] [CrossRef]
11. Word Health Organization (WHO) (2022). Mental disorders. [Google Scholar]
12. Hong, J., Park, S., Lee, J. (2019). Changes in depression and stress of the middle-aged and elderly through participation in a forest therapy program for dementia prevention. Journal of People, Plants, and Environment, 22(6), 699–709. DOI 10.11628/ksppe.2019.22.6.699. [Google Scholar] [CrossRef]
13. Korczyn, A. D., Halperin, J. (2009). Depression and dementia. Journal of the Neurological Sciences, 283(1), 139–142. DOI 10.1016/j.jns.2009.02.346. [Google Scholar] [CrossRef]
14. Byun, G. S., Lee, H. W. (2018). The impact of employment instability on mental health: The case of South Korea. Health and Social Welfare Review, 38(3), 129–160. DOI 10.15709/hswr. [Google Scholar] [CrossRef]
15. Kang, Y., NA, D. L., Hahn, S. (1997). A validity study on the Korean mini-mental state examination (K-MMSE) in dementia patients. Journal of the Korean Neurological Association, 15(2), 300–308. [Google Scholar]
16. Escobar, J., Burnam, A., Karno, M., Forsythe, A., Landsverk, J. et al. (1986). Use of the mini-mental state examination (MMSE) in a community population of mixed ethnicity: Cultural and linguistic artifacts. Journal of Nervous and Mental Disease, 174(10), 607–614. DOI 10.1097/00005053-198610000-00005. [Google Scholar] [CrossRef]
17. Radloff, L. S. (1977). The CES-D scale: A self-report depression scale for research in the general population. Applied Psychological Measurement, 1(3), 385–401. DOI 10.1177/014662167700100306. [Google Scholar] [CrossRef]
18. Naughton, M. J., Wiklund, I. (1993). A critical review of dimension-specific measures of health-related quality of life in cross-cultural research. Quality of Life Research, 2(6), 397–432. DOI 10.1007/BF00422216. [Google Scholar] [CrossRef]
19. Shin, J. Y., Park, E. C., Lee, S. G., Choi, Y., Kim, J. H. et al. (2016). The cross-interaction between global and age-comparative self-rated health on depressive symptoms–considering both the individual and combined effects. BMC Psychiatry, 16(1), 1–11. DOI 10.1186/s12888-016-1098-9. [Google Scholar] [CrossRef]
20. Jones, B. L., Nagin, D. S., Roeder, K. (2001). A SAS procedure based on mixture models for estimating developmental trajectories. Sociological Methods & Research, 29(3), 374–393. DOI 10.1177/0049124101029003005. [Google Scholar] [CrossRef]
21. Chen, Y., Campbell, P., Strauss, V. Y., Foster, N. E., Jordan, K. P. et al. (2018). Trajectories and predictors of the long-term course of low back pain: Cohort study with 5-year follow-up. Pain, 159(2), 252–260. DOI 10.1097/j.pain.0000000000001097. [Google Scholar] [CrossRef]
22. Mirza, S. S., Wolters, F. J., Swanson, S. A., Koudstaal, P. J., Hofman, A. et al. (2016). 10-year trajectories of depressive symptoms and risk of dementia: A population-based study. The Lancet Psychiatry, 3(7), 628–635. DOI 10.1016/S2215-0366(16)00097-3. [Google Scholar] [CrossRef]
23. Shin, W. (2001). The formation and evolution of firm internal labor markets: A case study of the shipbuilding industry in South Korea. Seoul National University, Department of Sociology (in Korean). [Google Scholar]
24. Kye, B. (2008). Internal labor markets and the effects of structural change: Job mobility in Korean labor markets between 1998 and 2000. Research in Social Stratification and Mobility, 26(1), 15–27. DOI 10.1016/j.rssm.2007.10.002. [Google Scholar] [CrossRef]
25. Kim, M. S., Hong, Y. C., Yook, J. H., Kang, M. Y. (2017). Effects of perceived job insecurity on depression, suicide ideation, and decline in self-rated health in korea: A population-based panel study. International Archives of Occupational and Environmental Health, 90(7), 663–671. DOI 10.1007/s00420-017-1229-8. [Google Scholar] [CrossRef]
26. Lee, J. O., Kapteyn, A., Clomax, A., Jin, H. (2021). Estimating influences of unemployment and underemployment on mental health during the COVID-19 pandemic: Who suffers the most? Public Health, 201, 48–54. DOI 10.1016/j.puhe.2021.09.038. [Google Scholar] [CrossRef]
27. Abrams, L. R., Finlay, J. M., Kobayashi, L. C. (2021). Job transitions and mental health outcomes among U.S. adults aged 55 and older during the COVID-19 pandemic. The Journals of Gerontology: Series B, 77(7), 106–116. [Google Scholar]
28. Breslin, F. C., Mustard, C. (2003). Factors influencing the impact of unemployment on mental health among young and older adults in a longitudinal, population-based survey. Scandinavian Journal of Work, Environment & Health, 29(1), 5–14. DOI 10.5271/sjweh.698. [Google Scholar] [CrossRef]
29. Spira, A. P., Chen-Edinboro, L. P., Wu, M. N., Yaffe, K. (2014). Impact of sleep on the risk of cognitive decline and dementia. Current Opinion Psychiatry, 27(6), 478–483. DOI 10.1097/YCO.0000000000000106. [Google Scholar] [CrossRef]
30. Yu, X. X., Langa, K. M., Cho, T. C., Kobayashi, L. C. (2022). Association of perceived job insecurity with subsequent memory function and decline among adults 55 years or older in england and the US, 2006 to 2016. JAMA Network Open, 5(4), e227060–e227060. DOI 10.1001/jamanetworkopen.2022.7060. [Google Scholar] [CrossRef]
31. Livingston, G., Huntley, J., Sommerlad, A., Ames, D., Ballard, C. et al. (2020). Dementia prevention, intervention, and care: 2020 report of the lancet commission. Lancet, 396, 413–446. DOI 10.1016/S0140-6736(20)30367-6. [Google Scholar] [CrossRef]
32. Rohwedder, S., Willis, R. J. (2010). Mental retirement. The Journal of Economic Perspectives: A Journal of the American Economic Association, 24(1), 119–138. DOI 10.1257/jep.24.1.119. [Google Scholar] [CrossRef]
33. Newbury-Birch, D., Kamali, F. (2001). Psychological stress, anxiety, depression, job satisfaction, and personality characteristics in preregistration house officers. Postgraduate Medical Journal, 77(904), 109–111. DOI 10.1136/pmj.77.904.109. [Google Scholar] [CrossRef]
34. Morris, W. R., Conrad, K. M., Marcantonio, R. J., Marks, M. A., Ribisl, K. M. et al. (1999). Do blue-collar workers perceive the worksite health climate differently than white-collar workers? American Journal of Health Promotion, 13(6), 319–324. DOI 10.4278/0890-1171-13.6.319. [Google Scholar] [CrossRef]
35. Erfurt, J. C. (1995). The wellness outreach at work program: A step-by-step guide. 1995: National Institutes of Health, National Heart, Lung, and Blood Institute. [Google Scholar]
36. Nourjah, P., Wagener, D. K., Eberhardt, M., Horowitz, A. M. (1994). Knowledge of risk factors and risk behaviors related to coronary heart disease among blue and white collar males. Journal of Public Health Policy, 15(4), 443–459. DOI 10.2307/3343026. [Google Scholar] [CrossRef]
37. Pieczynska, A., Zasadzka, E., Trzmiel, T., Pawlaczyk, M. (2019). Physical activity and fitness in white- and blue-collar retired men. American Journal of Men’s Health, 13(6). DOI 10.1177/1557988319891360. [Google Scholar] [CrossRef]
38. Lee, S. Y. (2012). Future directions and issues of mental health policies in korea. Health and Welfare Policy Forum, 187(5), 68–77. [Google Scholar]
39. Oh, Y. (2021). The national health plan 2030: Its purpose and directions of development. Journal of Preventive Medicine and Public Health, 54(3), 173–181. DOI 10.3961/jpmph.21.198. [Google Scholar] [CrossRef]
Cite This Article
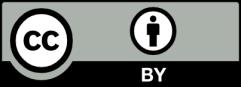
This work is licensed under a Creative Commons Attribution 4.0 International License , which permits unrestricted use, distribution, and reproduction in any medium, provided the original work is properly cited.