Open Access
ARTICLE
The Relationship between Students’ Anxiety and Internet Use Disorders: A Meta-Analysis
College of Education Science, Bohai University, Jinzhou, 121013, China
* Corresponding Authors: Shan Hu. Email: ; Huiyong Fan. Email:
International Journal of Mental Health Promotion 2023, 25(12), 1323-1341. https://doi.org/10.32604/ijmhp.2023.029096
Received 01 February 2023; Accepted 22 September 2023; Issue published 29 December 2023
Abstract
Theoretical models have predicted a positive association between anxiety and Internet use disorders. However, the findings of previous studies are conflicting, with some reporting a positive association and others proposing no relationship between the two. To explore the true relationship between the two and analyze the reasons for the differences, 100 primary studies involving 108,539 subjects were entered into a meta-analysis. The results showed that (1) there was a significant positive correlation between students’ anxiety and Internet use disorder (r = 0.330); (2) the moderating effect of anxiety type was significant. (3) The moderating effects of the measurement instrument for Internet use disorder, the measurement instrument for anxiety, the subject’s grade level, the subject’s region, and the type of publication were not significant. The question of how the different anxiety types of students and IUD have different mechanisms of action needs to be further explored.Keywords
Supplementary Material
Supplementary Material FileAs of December 2022, the total number of Internet users in China exceeds 1 billion, of which 18.7% are under 19 years old, 14.2% are between 20 and 29 years old, and about 32.9% are teenagers [1]. Due to immature psychological development, college and university students are prone to Internet Use Disorder (IUD) in the process of using the Internet [2], a concept developed by the American Psychiatric Association in an appendix to the new Diagnostic and Statistical Manual of Mental Disorders (DSM-5) [3], and it encompasses a wide range of conditions that previously included Internet Addiction (IA) [4–6], Excessive Internet Use (EIU) [7,8], and Problematic Internet Use (PIU) [9–11]. Among other related terms. According to surveys, IUD is prevalent in the adolescent population [12] and about 11.3% among college students [13,14] and about 13.4% among senior students [15]. It can be seen that the study of IUD has a very important practical significance.
In the context of the new Internet, exploring the causes and consequences of IUD is an issue that has become a focal point in the field. Among the many factors that may be associated with IUD, anxiety has received particular attention. It is important to note that anxiety is distinctly different from Anxiety Disorders (also known as Generalized Anxiety Disorder), and in order not to cause confusion, anxiety in this paper refers to the unpleasant and complex emotional state of tension, uneasiness, worry, and annoyance that an individual experiences in response to an impending danger or threat that may pose a danger [16], rather than the “Diagnostic and Statistical Manual of Mental Disorders (DSM)” [16], which is the term used in this article to refer to the complex emotional state of tension, unease, apprehension, and annoyance. Anxiety Disorders as defined by the Diagnostic and Statistical Manual of Mental Disorders (DSM-5) [3]. The relationship between anxiety and IUD has also been addressed in numerous previous theoretical models. The self-defense mechanism model of Anxiety-IUD [17] argueed that when faced with anxiety, individuals were prone to adopt negative coping styles, such as avoidance, in order to maintain inner balance in order to face it, and individuals who were more likely to adopt negative coping styles tend to be more likely to to develop IUD, and the model suggested that negative coping styles mediate the relationship between anxiety and IUD.
Gao et al. [18], Pang et al. [19], and Weinstein et al. [20] argued that the Internet is used as a tool to cope with anxiety, and that negative emotions, such as anxiety, stimulate an individual to enter a virtual environment. Spending too much time on the virtual environment can lead to IUD. Lavoie et al. [21] suggested that this compensatory internet use is a way of managing emotions by avoiding them or avoiding situations. The social-emotional model [22] and the compensatory internet use model [23] also integrate these ideas.
The Pathological Use Model (PUM) considers anxiety as an outcome of IUD. Specifically, IUD is a form of impulse control disorder [24,25], and although the anonymity of online socialization can increase an individual’s sense of control [26], online relationships are a weaker type of relationship than real relationships [27], so if the Internet is used as a “safe haven” and is overly relied upon and used, the social support received by individuals in the real world will be reduced, and individuals will be less satisfied with their real lives, and their levels of social anxiety and depression will be increased [28–30].
The Social-Psychological-Physiological Model (SPPM) suggests that anxiety can be both a cause and a consequence of IUD [31,32]. In the view of the model, IUD is a complex psychological phenomenon that is governed by social, psychological and physiological factors and can lead to a variety of social, psychological and physiological consequences. Among the psychological factors are depression and anxiety. On the one hand, anxious and depressed people are more likely to develop IUD; on the other hand, IUD leads to anxiety, i.e., prolonged use of the Internet leads to the isolation of individuals from the real world which in turn leads to anxiety and depression [31–33].
Whether as a cause or as a consequence, established theories tend to suggest that anxiety and IUD should be significantly and positively correlated. However, primary studies exploring the relationship have reported inconsistent results, with some reporting a significant positive correlation [34] and others showing no correlation [35,36]. If anxiety is not significantly correlated with IUD, then the reasoning of the previous two theories will not be supported by evidence.
As of now, no study has meta-analyzed the relationship between anxiety and IUD. Ran et al. [37] explored the relationship between social anxiety and cell phone addiction using a three-level meta-analysis and found that social anxiety significantly predicted cell phone addiction. However, cell phone addiction and IUD are not a concept at all [38]. Prizant-Passal et al. [39] conducted a meta-analysis of the relationship between social anxiety (social anxiety) and PIU, but only eight primary studies were included, and the number of primary studies was was too small. In this meta-analysis, there were 81 after 2016 and 48 before 2016. Furthermore, Prizant-Passal et al. [39] only studied social anxiety. And whether different types of anxiety may be differently related to IUD is a question that remains to be clarified. This shows that a meta-analysis of the relationship between anxiety and IUD correlation is of great theoretical importance.
Relationship between anxiety and IUD
What exactly is the relationship between anxiety and IUD? There are two views in previous primary research on the relationship between student anxiety and IUD: one view is that there is a significant positive correlation between the two, and the other view is that there is no correlation between the two.
The first view suggested that student IUD is significantly and positively correlated with anxiety [34] [40–42]. Yin et al. [43] used 478 vocational school students as subjects and collected data using the Internet Addiction Diagnostic Questionnaire as well as the Internet Consequences Scale (ICONS) and found that there was an extremely significant positive correlation between students’ Internet addiction and anxiety (r = 0.232). Chen et al. [44] surveyed 610 college students using the Self-assessment Scale for Social Anxiety (SSAS) and the Chinese Internet Addiction Scale (CIAS) and found that there was a significant positive correlation between the tendency to Internet addiction and social anxiety (r = 0.240). Tong [45] used 1947 school students over 14 years old and some school youths as subjects and collected data using the Diagnostic Criteria for Internet Addiction and the Symptom Self-Control Scale (SCL-90) and found that there was a moderate positive correlation between Internet addiction and anxiety (r = 0.316). Zhang et al. [46] collected data from 94 middle school students as subjects using the Chinese version of the Youth Diagnostic Questionnaire for Internet Addiction (YDQ) and the Revised Chinese version of the Children’s Maternal Conspicuous Anxiety Scale (RCMAS), and found that there was a significant positive correlation between Internet addiction and anxiety (r = 0.550).
The second view was that the correlation between student anxiety and IUD was not significant [35–37] [47]. Zhang et al. [47] used 355 undergraduate students as subjects and collected data using the Internet Addiction Identification Questionnaire (IAQ) developed by Young as well as the Interaction Anxiety Scale (IAS), and found that the difference in anxiety scores between non-internet-addicted and moderately internet-addicted undergraduates was not significant. Lee et al. [35] used 1168 students as subjects and collected data using the Korean version of the Adolescent PIU scale (IAI) and the Center for Epidemiological Studies Depression Scale (CESD) as measurement tools and found that the correlation between anxiety and IUD was not significant.
In summary, the first view was not only supported by the Self-Defense Mechanism Model of Anxiety-IUD, the Social-Emotional Model, the Compensatory Internet Use Model, the Pathological Use Model, and the Socio-Psycho-physiological Model, but also most empirical studies tend to support the first view, and thus we hypothesize that:
H1: There is a degree of positive correlation between students’ anxiety and IUD.
So, why do existing studies differ on the definition of the relationship between the two?
First, it may stem from differences in IUD measurement tools. In previous literature, researchers have examined the use of the Internet addiction questionnaire (Young’s Internet Addiction Text, YIAT) developed by Young, the Internet Addiction Diagnostic Criteria (IADC), Chinese Internet Addiction Scale (Revised Chen Internet Addiction Scale, CIAS-R), and Internet Addiction Scale (IAS) as IUD measurement tools, but different relationships have been drawn. Feng et al. [48] collected data using the Chinese Internet Addiction Scale (CIAS-R) and measured a correlation coefficient of r = 0.086 between Internet addiction and anxiety; Ren et al. [34] used the Internet Addiction Disorder Diagnostic Scale and came up with a correlation coefficient of r = 0.385. Cao et al. [49] used the Internet Addiction Impairment Scale (IADS), which yielded a correlation coefficient of r = 0.146 for Internet addiction and anxiety; Liu et al. [50] used the Chinese Internet Addiction Scale (CIAS-R), and found a correlation coefficient of r = 0.450. It can be seen that the correlation coefficients obtained from different measurement tools for IUD may also be different. Therefore, we hypothesized:
H2: The relationship between students’ anxiety and IUD is moderated by the IUD measurement instrument, and the relationship between anxiety and IUD obtained will be different under different conditions of the IUD measurement instrument.
Second, it may stem from differences in the type of anxiety. There exist three views on the classification of types of anxiety as follows. One, Spielberg divided anxiety into two types: state anxiety and trait anxiety [51], state anxiety is a transient emotional response that includes undesirable emotional reactions and thoughts; while trait anxiety refers to personality traits that are relatively stable anxiety tendencies with individual differences, also called generalized anxiety [52,53]. This view is also supported by Deng et al. [54]. Secondly, Anna Freud classified anxiety into instinctive anxiety, reality anxiety and superego anxiety in The ego and the mechanisms of defence [55]. Instinctive anxiety, or neurotic anxiety, refers to anxiety that arises from the fear that the ego’s impulses will overcome the ego; reality anxiety, or objective anxiety, refers to anxiety arising from real, objective dangers in reality; and superego anxiety, or moral anxiety, refers to anxiety that arises from the fear that an individual will do something that goes against the superego, and will experience guilt [56]. Other researchers believe that anxiety can be categorized into body anxiety and cognitive anxiety [57], with cognitive anxiety usually referring to worry, urgency, and those uncomfortable feelings; and body anxiety referring to physiological or physiological processes or sensations. Considering that Spielberg’s state-trait conceptual theory has played a great role in promoting anxiety research, this paper adopts Spielberg’s categorization to classify anxiety. In previous literature, the relationship between different types of anxiety and IUD has been examined by researchers, but the relationships derived by all are not the same, and the relationship between anxiety and IUD may be influenced by the type of anxiety.
Mohammadkhani et al. [58] surveyed 400 college students and reported a correlation coefficient of 0.250 between trait anxiety and IUD; Tao et al. [59] surveyed 1048 college students and reported a correlation coefficient of 0.400 between trait anxiety and IUD; Lavoie et al. [21] surveyed 2883 adolescents and reported a correlation coefficient of 0.218 between state anxiety and IUD; Zhang et al. [46] surveyed 94 middle school students and reported a correlation coefficient between state anxiety and IUD of 0.550. It can be seen that the correlation coefficients obtained may be different depending on the type of anxiety. Therefore, we hypothesized:
H3: The relationship between students’ anxiety and IUD is moderated by the type of anxiety, and different types of anxiety lead to divergent relationships between anxiety and IUD.
Third, the influence of measurement tools that may stem from anxiety. In previous literature, researchers have examined the use of the Self-Rating Anxiety Scale (SAS), the Social Anxiety Scale for Adolescents (SAS-A), the Depression, Anxiety, Stress Scale (DASS-21), the Beck Anxiety Inventory (BAI), and the Symptom Checklist-90 (SCL-90) as anxiety measurement tools. Ostovar et al. [60] collected data from 632 students using the Depression, Anxiety, and Stress Scale (DASS-21), and measured the correlation coefficient between Internet addiction and anxiety to be r = 0.549; Liu [61] collected data from the Self-assessment Scale of Anxiety (SAS), and measured the correlation coefficient between Internet addiction and anxiety was measured as r = 0.244; Seifi et al. [62] collected data using the Depression, Anxiety, Stress Scale (DASS-21), and the correlation coefficient between Internet addiction and anxiety was measured as r = 0.450; Wang et al. [63] collected data using the Social Anxiety Scale to collect data and measured the correlation coefficient between Internet addiction and anxiety as r = 0.164. It can be seen that the correlation coefficients obtained are different for different measurement tools of anxiety. Therefore, we hypothesized:
H4: The relationship between students’ anxiety and IUD is moderated by the instrument used to measure anxiety, and the relationship between anxiety and IUD obtained will be different for different conditions of the anxiety measurement instrument.
Fourth, it may stem from differences in subjects’ grade levels. In previous literature, researchers have examined the relationship between anxiety and IUD when using elementary and middle school students as well as college students as subjects, but the relationships derived by all are different. Panicker et al. [64] obtained a correlation coefficient of r = 0.200 between smartphone addiction and anxiety using college students as subjects; Javaeed et al. [65] obtained a correlation coefficient of r = 0.060 between Internet addiction and anxiety using college students as subjects.
Zhang [66] concluded that the correlation coefficient between Internet addiction and anxiety was r = 0.648 using middle school students as subjects; Zhang et al. [46] concluded that the correlation coefficient between Internet addiction and anxiety was r = 0.550 using middle school students as subjects. it can be seen that the correlation coefficients obtained may be different depending on the grade level of the subjects. Therefore, we hypothesized:
H5: The relationship between students’ anxiety and IUD is moderated by the grade level of the subjects, and the relationship between anxiety and IUD obtained will be different when students of different grade levels are chosen as subjects.
Fifth, it may stem from differences in the region where the subjects are located. In previous literature, researchers have examined the relationship between anxiety and IUD when subjects from Asia, Europe, North America, and Africa were used as study subjects, but the relationships derived were not the same. Cheng et al. [67] concluded that Internet addiction rates were negatively correlated with the Global National Life Satisfaction Index (GNLSI) and the country-specific Environmental Quality Index (EQI), and thus the region in which the subjects were located could also be a potential moderating The region of the subject may also be a potential moderating variable.
Kheyri et al. [68] surveyed 353 college student subjects in the Asian region and reported a correlation coefficient of r = 0.173; Shan et al. [69] surveyed 3380 college student subjects in Asia and reported a correlation coefficient of r = 0.158.
Koc [70] surveyed 174 college student subjects in the European region and reported a correlation coefficient of r = 0.872, while Woon [71] surveyed 125 secondary school student subjects in the European region and reported a correlation coefficient of r = 0.605. It can be seen that the correlation coefficients obtained may differ depending on the region in which the subjects are located. Therefore, we hypothesized:
H6: The relationship between students’ anxiety and IUD is moderated by the district in which the subjects are located, and the relationship between anxiety and IUD obtained will be different when students from different districts are chosen as subjects.
Sixth, it may stem from differences in publication type. Based on previous experience, publication type may also be a potential moderating variable. In previous literature, researchers have examined the relationship between anxiety and IUD in the form of journal articles and dissertations, but all have come up with different relationships. Liu [61] investigated 503 subjects in a dissertation and reported a correlation coefficient of r = 0.244; Song [72] investigated 355 subjects in a dissertation and reported a correlation coefficient of r = 0.190.
Ostovar et al. [60] surveyed 632 subjects in journal articles and reported a correlation coefficient of r = 0.549. Jiang et al. [73] surveyed 1804 subjects in journal articles and reported a correlation coefficient of r = 0.402. It can be seen that correlation coefficients obtained may be different depending on the publication type. Therefore, we hypothesized:
H7: The relationship between students’ anxiety and IUD is moderated by publication type, and the relationship between reported anxiety and IUD will vary by publication type.
Literature search and selection
This study comprehensively searched the literature up to June 2022 in both Chinese and English. The English literature search databases Google, ELSEVIER, Springer Link, SAGE, Willy, JSTOR, and Web of Science (Core Collection) were searched for students, middle school students, university student, undergraduate student, teenagers, callan, internet addiction, network addiction, excessive Internet use, pathological network use, pathological Internet use, problematic internet use, problematic network use, anxiety, anxiety, etc. Keywords such as “topic” or “full-text” or “analysis” are used in the context of the following. “full text” or “title” search fields. The Chinese literature was mainly searched through CNKI Knowledge Network Database, Wanfang Database, and Wipu Journal Network by keywords such as adolescents, middle school students, college students, students, junior high school students, high school students, Internet addiction, pathological Internet use, problematic Internet use, cell phone addiction, gaming addiction, social network addiction, anxiety, social anxiety, and attachment anxiety, etc., in the search field of “subject” or “full text” or “article title”. “ or “full text” or “title”.
Our search included documents up to February 28, 2023, and resulted in the identification of 100 primary studies for inclusion in our study. The literature search process is shown in Fig. 1.
Figure 1: Flowchart for inclusion of literature.
Literature inclusion and exclusion criteria
Combining the meta-analytic methodology and the requirements of the research topic, the criteria for inclusion in the meta-analytic study were (1) The concepts of anxiety and IUD were clearly defined, and recognized questionnaires or scales for the measurement of anxiety and IUD were used. (2) The study population was a normal student population, including elementary, middle, and high school students. (3) Specific correlation coefficient r-values, or information that can be converted to correlation coefficients such as t-values, F-values, and χ2-values were reported. (4) Specific sample sizes were reported. (5) The language was Chinese or English.
The exclusion criteria were (1) The type of article was a review, abstract, case report, etc. (2) Incomplete reporting of important data. (3) Unclear definitions of anxiety and IUD-related behaviors. (4) Repeated publications. For example, Cao et al. [74] and Zhou et al. [75] belonged to the same data repetitively published. (5) Students with special status. Such as students with anxiety disorders, as well as other mental disorders and serious physical illnesses [76].
Initially, 2778 primary studies were searched and obtained, and the final number of papers included in the meta-analysis was 100, of which 38 were in Chinese and 62 were in English, containing 100 effect sizes, with a total sample size of 108,539, and a span of publication years from 2004 to 2022.
The entire literature was included and excluded based on the above criteria, and the literature included in the meta-analysis was coded as follows (see Table 1): literature information (author’s name and year of publication of the literature), region of the literature surveyed, population of the study, sample size, measurement tool, language of the literature, type of publication, effect size, male ratio, type of anxiety, and quality score.
The coding process consists of several steps. The first step was to develop a coding plan. Based on the purpose and research questions of this study, the coding plan included the variables listed in Table 2. The second step was to extract information from the study. This study was independently coded by the first author. The third step was to perform cross-checking and validation. The first author completed the coding and then had the second author proofread it one by one. The results showed that the agreement between the two independent coders was 96%, except for a small amount of bias in some of the data. When inconsistencies in coding were encountered, revisions were made after reviewing the original study discussion.
Information extraction is primarily concerned with understanding sample characteristics in the original study and searching for potential moderating variables that may be present [150]. A number of study characteristics were systematically extracted from the primary studies that could serve as potential moderators to explain inconsistencies between the effect sizes of individual studies. These study characteristics are listed in Table 2.
The correlation coefficient (Zero order r) was used as the effect size in this study. In the calculation process, each r value was first converted to the corresponding Fisher’s Z-score with the formula Z = 0.5 × In (
Several studies have converted existing information to r. Yin et al. [43], Mamun et al. [109], and Radeef et al. [119] by comparing the Internet Addiction Disorder (IAD) group with the non-IAD group by comparing the difference in mental status scores between the IAD and non-IAD groups and reporting t-values, concluding that anxiety was significantly and positively associated with Internet addiction; Wang [132] reported χ2-values in the primary literature, concluding that anxiety was significantly and positively associated with IUD; Liu [61] and Zhang et al. [47] reported F-values in the original literature and concluded that anxiety was significantly and positively associated with Internet addiction. Instead of directly reporting correlation coefficients based on the correlation between anxiety and IUD, these literatures reported t-values, χ2-values, or F-values, which we converted to r-values using the correlation formula [151], namely
In this study, the rating criteria of Gignac et al. [152] were used and r = 0.100, r = 0.200 and r = 0.300 were considered as low, moderate and strong correlation, respectively.
The main difference between a fixed effects model and a random effects model is the source of the error. In a fixed-effects model, the variation between effect sizes is caused only by random error (from the subjects). In a random effects model, the variation between effect sizes is caused by random error and some systematic error (from a study characteristic, such as publication type). Due to the structure of the errors, the results of fixed effects models cannot be generalized to other situations, but the results of random effects models can be generalized [150]. The research aim of this study was to generalize the findings to other samples, therefore, a random effects model was selected for the meta-analysis [150,153].
The main tests for heterogeneity are the Q test and the I2 test. The Q test is a test based on total variation, assuming that the effect sizes follow a chi-square distribution, which indicates significant heterogeneity if p < 0.05; according to previous views, 25%, 50%, and 75% of the I2 values can be considered as the boundaries of low, medium, and high heterogeneity [150,154].
Publication Bias (PB) is an error caused by some unseen selection process, in which those studies with significant results receive a higher likelihood of publication [150]. Fail safe N (Nfs), Funnel plot, trim and fill method, Egger’s regression are commonly used to detect publication bias.
The failure safety factor (Fail safe N, Nfs) is defined as the number of primary studies needed to make the mean effect zero [150]. If Nfs is small, less than 5K + 10, then there is a possibility of publication bias. If Nfs is large, greater than 5K + 10, then there is a low likelihood of publication bias.
A funnel plot is a scatter plot in which the observed effect size is loaded on the x-axis and its standard error is loaded on the y-axis [150]. The degree of symmetry of the funnel plot will indicate the magnitude of publication bias, with left-right symmetry indicating less publication bias and left-right asymmetry indicating more publication bias.
Clipping is often used to adjust for asymmetries in funnel plots [155]. After adding some “missing” data as required by symmetry, the cut-and-patch method re-estimates the total effect size. A small difference in the size of the total effect size before and after clipping indicates a small effect of publication bias; a large difference indicates a large effect of publication bias.
Egger’s regression (Egger’s regression test) is a meta-analytic publication bias test by linear regression [156]. Egger’s linear regression when the intercept is not significant indicates less bias.
This study also obtained unpublished literature as much as possible during the literature search stage to minimize the effect of publication bias.
Quality assessment of original research
In this study, the Basic Quality Assessment of Primary Study, BQAPS [157] was used as a quality assessment tool to assess the quality of studies included in the meta-analysis, based on a scoring criteria, 0–6 was categorized as low quality, 6–12 was categorized as low to medium level, 12–18 was categorized as high to medium level, and 18 or more was categorized as high quality.
Impact of quality of original research
Based on the scoring criteria, quality assessment showed that 86 of the primary studies were of moderate to high quality (86.00%) and 14 were of high quality (14.00%). The moderating effect of quality scores on the relationship between anxiety and IUD was not significant (QB = 0.002, p = 0.963).
The relationship between anxiety and IUD was significantly positive in the medium- and high-quality subgroups, with a correlation coefficient of 0.329% and 95% CI [0.265, 0.389], and in the high-quality subgroup, with a correlation coefficient of 0.330% and 95% CI [0.283, 0.376].
The funnel plot shows that effect sizes are distributed on both sides of the total effect size, with the complementary black dots to the right of the mean, suggesting that there may be larger effect sizes that were not published (see Fig. 2). Egger’s linear regression resulted in a non-significant intercept of −0.978 with a 95% CI [−3.945, 1.989] and a p = 0.515, suggesting that the funnel plot was symmetric in a statistically significant way. Nfs = 16187, greater than 5K + 10 = 5 × 100 + 10 = 510, indicating that no negative results were missed. After adding 25 effect sizes, the effect size was 0.394 with a 95% CI [0.389, 0.398], a non-significant difference. Overall, the effect of publication bias on the findings in this study was negligible.
Figure 2: Funnel plot for this study.
The purpose of the heterogeneity test is to test whether the effect sizes measured between studies are heterogeneous. In this study, the relationship between student anxiety and IUD was tested for heterogeneity and the results are shown in Table 3.
The results in Table 3 show that the Q-tests for the effect values between studies were significant (p < 0.001), indicating that the effect values in the meta-analysis were heterogeneous; the total sample I2 value was 98.180%, which may be due to the effect of moderating variables.
The random effects model was used to estimate the strength of correlation between students’ anxiety and IUD, and the results showed that the correlation coefficient between the two was 0.330 with 95% CI [0.289, 0.369]. Based on the reference standard, the correlation coefficient between anxiety and IUD in this study was greater than 0.300, and it can be considered that there is a high strength positive correlation between the two [152,158].
Analysis of moderating effects
Moderating effects of IUD’s measurement tools
The moderating effect of the measurement tool for IUD was not significant (QB = 6.953, p = 0.138) (see Table 4). The relationship between anxiety and IUD was significantly and positively correlated across the five subgroups of studies analyzed in the CIAS-R, IADC, YIAT, IAS, and other, with correlation coefficients of 0.294 (95% CI [0.081, 0.481]), 0.222 (95% CI [0.028, 0.401]), 0.302 (95% CI [0.259, 0.343]), 0.257 (95% CI [0.092, 0.408]), and 0.372 (95% CI [0.324, 0.419]).
Moderating effects of anxiety types
Anxiety type had a significant moderating effect (QB = 48.883, p < 0.000) (see Table 4). The relationship between anxiety and IUD was significantly and positively correlated in all three subgroups analyzed in the study: state anxiety, trait anxiety, and state-trait anxiety, with correlation coefficients of 0.310 (95% CI [0.267, 0.351]), 0.349 (95% CI [0.287, 0.408]), and 0.156 (95% CI [0.125, 0.187]), respectively. In descending order: trait anxiety, state anxiety, and state-trait anxiety.
Moderating effects of measurement instruments for anxiety
The moderating effect of the instrument measuring anxiety was not significant (QB = 5.418, p = 0.367) (see Table 4).The correlation coefficients for the six subgroups of DASS-21, SAS, SAS-A, BAI, SCL-90, and other were 0.355 (95% CI [0.252, 0.449]), 0.330 (95% CI [0.170, 0.474]), 0.301 (95% CI [0.120, 0.463]), 0.197 (95% CI [0.078, 0.310]), 0.316 (95% CI [0.281, 0.350]), and 0.330 (95% CI [0.291, 0.369]), and the correlation coefficients of the subgroups of the measurement tools of anxiety were not significant differences.
Moderating effects of grade level
The moderating effect of grade level was not significant (QB = 2.722, p = 0.099) (see Table 4). The correlation coefficients were 0.377 (95% CI [0.321, 0.429]), and 0.307 (95% CI [0.243, 0.368]) for the two subgroups of middle school and college, respectively. The correlation coefficients for the two subgroups of grade level were not significantly different.
Moderating effects of subjects’ location
The moderating effect of the location of the subjects was not significant (QB = 1.055, p = 0.788) (see Table 4). Significant positive correlations were found across the four subgroups of studies analyzed in Asia, Europe, Africa, and North America, with correlation coefficients of 0.327 (95% CI [0.281, 0.372]), 0.347 (95% CI [0.232, 0.425]), 0.350 (95% CI [0.288, 0.409]), and 0.281 (95% CI [ 0.147, 0.405]).
Moderating effect of type of publication
The moderating effect of publication type was not significant (QB = 2.176, p = 0.140) (see Table 4). The correlation coefficients for the two subgroups of journal articles and dissertations were 0.320 (95% CI [0.273, 0.366]) and 0.384 (95% CI [0.313, 0.451]), respectively. The difference between the two groups did not reach significance.
In order to obtain the true relationship between anxiety and IUD, the present study conducted a meta-analysis of 100 primary studies and found significant moderating effects of the instrument used to measure IUD, the type of anxiety, the instrument used to measure anxiety, the grade level of the subjects, and the region in which the subjects were located.
Main effects of the relationship between student anxiety and IUD
The present study found a high-intensity significant positive correlation between student anxiety and IUD (r = 0.330), validating Hypothesis 1. This result is consistent with the ego-defense mechanism model of anxiety-IUD [17], the socioemotional model [22], the pathological use model [30], and the social-psycho-physiological model [31] were consistent in their predictions.
From a process perspective, anxiety may be both a cause and a consequence of IUD. First, anxiety may lead to IUD. the internal logic is that individuals with high levels of anxiety may have distorted self-perceptions [159,160], experience more negative affect [22], and thus search for security, satisfaction in online use [161,162].
Second, IUD may also lead to anxiety. The underlying logic is that overuse of the Internet leads to a decrease in normal social interactions and impaired social functioning [163–165], which can lead to an individual’s anxiety about the future [30,166].
The main reason why some primary studies reported non-significant results of correlation is that their sample sizes were small and not representative enough [35,36,47]. The correlation significant results from this meta-analysis are more credible after integrating the information from multiple samples.
Moderating effects of student anxiety and IUD
Moderating effects of IUD’s measurement tools
The moderating effect of the IUD measurement tool on the relationship between anxiety and IUD was not significant (QB = 6.953, p = 0.138) and did not support Hypothesis 2. The relationship between anxiety and IUD was significantly and positively correlated across the four subgroups of studies analyzed in the CIAS-R, the IADC, the YIAT, the IAS, and other, with correlation coefficients of 0.294, 0.222, 0.302, 0.257, and 0.372. The effects of the IUD measurement instruments were all on the order of 0.2 and 0.3, indicating that the relationship was consistent across IUD instruments.
Moderating effects of anxiety type
Anxiety type had a significant moderating effect on the relationship between anxiety and IUD (QB = 48.883, p < 0.000), validating Hypothesis 3. The three subgroups analyzed in the study of state anxiety, trait anxiety, and state-trait anxiety were significantly positively correlated, with correlation coefficients of 0.310, 0.349, and 0.156, respectively. These correlation coefficients can be classified into two groups, state anxiety and trait anxiety as a group with correlation coefficients greater than or equal to 0.300, and state-trait anxiety as a group with correlation coefficients less than 0.20. And in descending order, the correlation coefficients are: trait anxiety, state anxiety, and state-trait anxiety.
This result suggests that the magnitude of the association between different types of anxiety and IUD is not consistent. The reason for this result may be that different types of anxiety do not play the same role in the creation, formation, and development of IUD. Anxiety as a cause may be more distant from IUD and its association with IUD is relatively small, whereas anxiety as a consequence may be closer to IUD and its association with IUD is relatively high.
Moderating effects of measurement instruments for anxiety
The moderating effect of the anxiety measurement tool was not significant (QB = 5.418, p = 0.367) and did not support Hypothesis 4. The correlation coefficients for the relationship between anxiety and IUD across the six subgroups of the DASS-21, SAS, SAS-A, BAI, SCL-90, and other were 0.355, 0.330, 0.301, 0.107, 0.316 and 0.330, respectively. The effects of the anxiety measurement instruments were all on the order of 0.300, indicating that the relationship was consistent across anxiety instruments. One of the correlations in the SCL-90 condition was as high as 0.90, the reason for which requires further analysis.
Moderating effects of grade level
The moderating effect of grade level was not significant (QB = 2.722, p = 0.099) and did not support Hypothesis 5. The correlation coefficients for the secondary and tertiary subgroups were 0.377 and 0.307, respectively, suggesting that there may be stability in the relationship across age groups.
This result is supported by previous research. First, the correlation between the two may be high at both the middle school level and the college level. Li et al. [105] surveyed 2848 middle school students who reported low scores on both anxiety and IUD in the original study, and the correlation between the two was high, with a correlation coefficient of r = 0.430. Peng [118] surveyed 812 high school students who reported Anxiety and IUD scores were both high, and the correlation between the two was high, with a correlation coefficient of r = 0.460; Tu et al. [129] surveyed 869 college students, and both Anxiety and IUD scores reported in the original study were low, whereas the correlation between the two was high, with a correlation coefficient of r = 0.338; Zhang [146] surveyed 230 college students, and the correlation between the both anxiety and IUD scores were high, and the correlation between the two was high, with a correlation coefficient of r = 0.583. Second, there were grade-level differences in IUD use only on individual dimensions, such as the negative consequences dimension, but overall there were no significant grade-level differences in student IUD [167].
Moderating effects of subjects’ location
The moderating effect of the region where the subjects were located was not significant (QB = 1.055, p = 0.788) and did not support Hypothesis 6. Significant positive correlations were found across the four subgroups of the study analyzed in Asia, Europe, Africa, and North America, with correlation coefficients of 0.327, 0.347, 0.350, and 0.281, respectively.This result suggests that there is a consistency in the relationship of anxiety and IUD across regions. As global interactions and competition increase, global well-being is declining [168], and anxiety levels among adolescents are rising globally [169,170]. This is also supported by previous research, which confirmed through meta-analysis that both anxiety levels and cell phone dependence levels are increasing with each passing year [37].
Moderating effect of type of publication
The results of this study showed that the moderating effect of publication type on the relationship between anxiety and IUD was not significant (QB = 2.176, p = 0.140), which did not support Hypothesis 7. This is corroborated with the results of the detection of publication bias. Card [151] stated that one test for publication bias is to compare the differences between the outcomes of the different types of publication, and that if the differences are not significant, then it means that publication bias is not significant. In other words, publication type differences and publication bias in this study are negligible.
Research shortcomings and future prospects
Relative to previous literature [39], the present meta-analysis was extended in two ways. First, 100 primary studies were included in this study, making the findings more reliable. Second, the meta-analysis categorized anxiety and found a significant moderating effect. This implies that not only social anxiety but also other types of anxiety may be related to IUD. However, several previous theoretical models (cognitive-behavioral model, social-emotional model, pathological use model, and socio-psycho-physiological model) did not provide a reasonable explanation for this.
Nonetheless, there are some shortcomings in this study. First, the number of primary studies on some subgroups of moderating variables, such as the area where the subjects were located, was too small. Some had only 1 article. This could lead to potentially unstable results. Second, because the primary studies were not differentiated, the present meta-analysis also failed to separately explore the relationship between anxiety as a cause and IUD, and the relationship between anxiety as a consequence and IUD.
In addition to increasing the number of original studies, future research needs to focus on the following issues. First, further explore the different relationship models between state anxiety, trait anxiety and IUD, and deeply analyze the role of anxiety in the generation, formation and development of IUD. Second, a controlled experiment, or cross-lag study, should be conducted to further determine the causal relationship between anxiety and IUD. Third, further integrate the existing theoretical models (i.e., self-defense mechanism model of anxiety-IUD, social-emotional model, pathological use model, social-psychological-physiological model) to clarify the psychological process of the relationship between anxiety and IUD.
Under the conditions of this study, the following conclusions were obtained in this paper:
(1) There is a high intensity significant positive correlation between student anxiety and IUD.
(2) Anxiety type significantly moderated the relationship between student anxiety and IUD.
(3) The moderating effects of the measurement tool used by the problematic network, the measurement tool for anxiety, the subject’s grade level, the subject’s region, and the type of publication on the relationship between student anxiety and IUD were not significant.
Acknowledgement: The authors thank research participants for their participation in this study.
Funding Statement: This work was supported by “An Empirical Study on the Relationship between Mobile Phone Dependence and Social Anxiety among Adolescents in the Post-COVID-19 Era” (LJKMR20221512) and “LiaoNing Revitalization Talents Program” (grant no. XLYC2007134).
Author Contributions: The authors confirm contribution to the paper as follows: study conception and design: Yichi Zhang, Yuxiang Feng, Shan Hu; data collection: Yichi Zhang; analysis and interpretation of results: Yichi Zhang, Yuxiang Feng, Huiyong Fan; draft manuscript preparation: Yichi Zhang, Yuxiang Feng, Shan Hu, Huiyong Fan. All authors reviewed the results and approved the final version of the manuscript.
Availability of Data and Materials: The data will be provided upon request to the corresponding author.
Ethics Approval: Not applicable.
Conflicts of Interest: The authors declare that they have no conflicts of interest to report regarding the present study.
Supplementary Materials: The supplementary material is available online at https://doi.org/10.32604/ijmhp.2023.029096.
References
Literature with * included in meta-analysis
1. China Internet Network Information Center. The 51st statistical report on Internet development in China. Available from: https://www.cnnic.net.cn/n4/2023/0303/c88-10757.html [Accessed 2023]. [Google Scholar]
2. Lopez-Fernandez O, Honrubia-Serrano ML, Gibson W, Griffiths MD. Problematic Internet use in British adolescents: an exploration of the addictive symptomatology. Comput Hum Behav. 2014;35:224–33. [Google Scholar]
3. American Psychiatric Association. Diagnostic and statistical manual of mental disorders (DSM-5). Arlington, VA: American Psychiatric Association; 2013. [Google Scholar]
4. Chen X, Huang X, Bai G. Psychological research on Internet addiction. Adv Psychol Sci. 2003;3:355–9. [Google Scholar]
5. Griffiths M. Internet addiction: does it really exist? In: Gackenbach J, editor. Psychology and the Internet: intrapersonal, interpersonal, and transpersonal implications. San Diego, CA: Academic Press; 1998. p. 61–75. [Google Scholar]
6. Young KS. Psychology of computer use: XL. addictive use of the Internet: a case that breaks the stereotype. Psychol Rep. 1996;79(3):899–902. [Google Scholar] [PubMed]
7. Hansen S. Excessive Internet usage or Internet addiction? The implications of diagnostic categories for student users. J Comput Assist Lear. 2002;18:232–6. [Google Scholar]
8. Weinstein A, Lejoyeux M. Internet addiction or excessive Internet use. Am J Drug Alcohol Abuse. 2010;36:277–83. [Google Scholar] [PubMed]
9. Lang Y, Jia F, Li H, Su L, Zhao S. Investigation of Internet addiction in junior middle school students and analysis of related factors. Chin J Clin Psychol. 2008;16(4):417–9. [Google Scholar]
10. Caplan SE. Problematic Internet use and psychosocial well-being: development of a theory-based cognitive-behavioral measurement instrument. Comput Hum Behav. 2002;18:553–75. [Google Scholar]
11. Shapira N, Goldsmith T, Keck P, Khosla U, McElroy S. Psychiatric features of individuals with problematic Internet use. J Affect Disord. 2000;57:267–72. [Google Scholar] [PubMed]
12. Zhang H, Chen L. A study of Internet addiction and its attribution among urban and rural minors. Psychol Sci. 2009;3:748–50. [Google Scholar]
13. Deng L, Fang X, Wan J, Zhang J, Xia C. The relationship between psychological needs and their fulfillment and Internet addiction among college students. Psychol Sci. 2012;1:123–8. [Google Scholar]
14. Li L, Xu DD, Chai JX, Wang D, Li L, Zhang L, et al. Prevalence of Internet addiction disorder in Chinese university students: a comprehensive meta-analysis of observational studies. J Behav Addict. 2018;7(3):610–23. [Google Scholar] [PubMed]
15. Gao M, Teng Z, Wei Z, Jin K, Xiao J, Tang H, et al. Internet addiction among teenagers in a Chinese population: prevalence, risk factors, and its relationship with obsessive-compulsive symptoms. J Psychiat Res. 2022;153:134–40. [Google Scholar] [PubMed]
16. Huang X. Personality psychology. Hangzhou, China: Zhejiang Education Press; 2003. [Google Scholar]
17. * Ye B, Zheng Q. Mechanisms of stress on college students’ Internet addiction. Psychol Sci. 2016;39(3):621–7. [Google Scholar]
18. Gao W, Chen Z. Research on the pathopsychological mechanism of Internet addiction and comprehensive psychological intervention. Adv Psychol Sci. 2006;4:596–603. [Google Scholar]
19. Pang H, Wu Y, Zeng Y, Peng S, Chen S. A study of personality traits of adolescents with Internet addiction. Psychol Sci. 2010;(1):210–2. [Google Scholar]
20. * Weinstein A, Dorani D, Elhadif R, Bukovza Y, Yarmulnik A, Dannon P. Internet addiction is associated with social anxiety in young adults. Ann Clin Psychiatry. 2015;27(1):4–9. [Google Scholar] [PubMed]
21. * Lavoie C, Dufour M, Berbiche D, Therriault D, Lane J. The relationship between problematic Internet use and anxiety disorder symptoms in youth: specificity of the type of application and gender. Comput Hum Behav. 2023;140:107604. [Google Scholar]
22. Savci M, Aysan F. Social-emotional model of Internet addiction. Psychiatr Clin Psychopharmacol. 2017;27(4):349–58. [Google Scholar]
23. Kardefelt-Winther D. A conceptual and methodological critique of Internet addiction research: towards a model of compensatory Internet use. Comput Hum Behav. 2014;31:351–4. [Google Scholar]
24. Young KS. What makes the Internet addictive: potential explanations for pathological Internet use. In: 105th Annual Conference of the American Psychological Association, 1997;Chicago, USA. [Google Scholar]
25. Young KS, Rodgers R. The relationship between depression using the BDI and pathological Internet use. In: 105th Annual Meeting of the American Psychological Association, 1997;Chicago, USA. [Google Scholar]
26. He J, Chen C, He S, Zhou Z. Is there a lower level of social anxiety in online socialization? Adv Psychol Sci. 2014;22(2):288–94. [Google Scholar]
27. Kraut R, Patterson M, Lundmark V, Kiesler S, Mukophadhyay T, Scherlis W. Internet paradox: a social technology that reduces social involvement and psychological well-being? Am Psychol. 1998;53(9):1017–31. [Google Scholar] [PubMed]
28. Weidman AC, Fernandez KC, Levinson CA, Augustine AA, Larsen RJ, Rodebaugh TL. Compensatory Internet use among individuals higher in social anxiety and its implications for well-being. Pers Indiv Differ. 2012;53(3):191–5. [Google Scholar]
29. Wang F, Ni M, Li H, Wo J. Diagnosis and treatment of Internet addiction. Psychol Sci. 2008;3:714–6. [Google Scholar]
30. Gentile DA, Choo H, Liau A, Sim T, Li D, Fung D, et al. Pathological video game use among youth: a two-year longitudinal study. Pediatrics. 2011;127:e319–29. [Google Scholar] [PubMed]
31. Liu S, Zhang Z. A socio-psychophysiological model of Internet addiction and research perspectives. Appl Psychol. 2004;2:48–54. [Google Scholar]
32. Young KS, Rogers RC. The relationship between depression and Internet addiction. Cyberpsychol Behav. 1998;1(1):25–8. [Google Scholar]
33. Jin S, Yu Q, Guo Y, Zhang L, Zhu Y, Wu G. The effect of adolescents’ frequency of online social use on Internet addiction: the moderating role of family economic status. Psychol Sci. 2017;4:885–91. [Google Scholar]
34. * Ren YJ, Yang J, Liu LQ. Social anxiety and Internet addiction among rural left-behind children: the mediating effect of loneliness. Iran J Public Health. 2017;46(12):1659–68. [Google Scholar] [PubMed]
35. Lee YK, Chang CT, Cheng ZH, Lin Y. How social anxiety and reduced self-efficacy induce smartphone addiction in materialistic people. Soc Sci Comput Rev. 2018;36(1):36–56. [Google Scholar]
36. Leménager T, Hoffmann S, Dieter J, Reinhard I, Mann K, Kiefer F. The links between healthy, problematic, and addicted Internet use regarding comorbidities and self-concept-related characteristics. J Behav Addict. 2018;7(1):31–43. [Google Scholar]
37. Ran G, Li J, Zhang Q, Niu X. The association between social anxiety and mobile phone addiction: a three-level meta-analysis. Comput Hum Behav. 2022;130:107198. [Google Scholar]
38. Li J, Hu D, Ji J, Fu H. Development of mobile Internet addiction and a discussion on the concept. Chin J Behav Med Sci. 2015;24(12):1138–40. [Google Scholar]
39. Prizant-Passal S, Shechner T, Aderka IM. Social anxiety and Internet use—A meta-analysis: what do we know? What are we missing? Comput Hum Behav. 2016;62:221–9. [Google Scholar]
40. Dai Me, Sun J. The relationship between Internet addiction and mental health of adolescent students. J Weifang Coll. 2008;8(3):134–6. [Google Scholar]
41. Jiang Y, Qu L, Liu G, Zheng X. Internet addiction among college students: a survey and reflection. J Guangxi Normal University: Philos Soc Sci Ed. 2005;26(1):37–42. [Google Scholar]
42. Ma J, Li R, Zhu C, Song G, Liu C, Huo F, et al. The relationship between Internet addiction and mental health in adolescent students. China Chronic Dis Prev Control. 2008;16(2):161–4. [Google Scholar]
43. * Yin F, Zhou S. Characteristics and physiological-psychological-social consequences of adolescent Internet addiction. Chinese J Health Psychol. 2012;20(2):233–5. [Google Scholar]
44. * Chen D, Zhang J, Shen L, Liao Z. Internet addiction and its relationship with social anxiety among college students. Chinese J Health Psychol. 2009;2:151–2. [Google Scholar]
45. * Tong H. Analysis of the correlation between Internet addiction and mental health factors among school-age adolescents in Pingliang City. Chinese J Health Psychol. 2012;20(5):753–5. [Google Scholar]
46. * Zhang M, Bian Y. An analysis of the brain structures underlying the link between pathological Internet use and anxiety. Addict Behav. 2021;112(4):106632. [Google Scholar] [PubMed]
47. * Zhang X, Wang E, Wei L. Exploring the psychological mechanism of Internet addiction among college students. J Xingtai Vocat Tech Coll. 2008;25(6):62–6. [Google Scholar]
48. * Feng Y, Ma Y, Zhong Q. The relationship between adolescents’ stress and Internet addiction: a mediated-moderation model. Front Psychol. 2019;10:2248. [Google Scholar] [PubMed]
49. * Cao J, Cai Y. Analysis of Internet addiction and its influencing factors among junior high school students in Daqing. China School Health. 2010;12:1461–2. [Google Scholar]
50. * Liu S, Zou S, Zhang D, Wang X, Wu X. Problematic Internet use and academic engagement during the COVID-19 lockdown: the indirect effects of depression, anxiety, and insomnia in early, middle, and late adolescence. J Affect Disorders. 2022;309:9–18. [Google Scholar] [PubMed]
51. Endler NS, Parker JD, Bagby RM, Cox BJ. Multidimensionality of state and trait anxiety: factor structure of the endler multidimensional anxiety scales. J Pers Soc J Pers social Psychol. 1991;60(6):919–26. [Google Scholar]
52. Chen M, Huang S, Xu N, Li J, Wu Q. Effects of trait anxiety on college students’ WeChat overuse: chain-mediated effects of cognitive reappraisal and sense of meaning in life. Chinese J Health Psychol. 2023;2:249–54. [Google Scholar]
53. Bander RS, Betz NE. The relationship of sex and sex role to trait and situationally specific anxiety types. J Res Pers. 1981;15(3):312–22. [Google Scholar]
54. Deng W, Lei L. An experimental study of self-schemas for different anxiety types. Psychol Inq. 2004;1:70–3. [Google Scholar]
55. Freud A. The ego and the mechanisms of defence. London: Routledge; 2018. [Google Scholar]
56. Zheng R, Jiang G, Wu X. Contemporary psychological counseling and therapy system. Beijing: Higher Education Press; 2006. [Google Scholar]
57. Fox JE, Houston BK. Distinguishing between cognitive and somatic trait and state anxiety in children. J Pers Soc Psychol. 1983;45(4):862–70. [Google Scholar] [PubMed]
58. * Mohammadkhani P, Alkasir E, Pourshahbaz A, Jafarian Dehkordi F, Soleimani Sefat E. Internet addiction in high school students and its relationship with the symptoms of mental disorders. Iran Rehabil J. 2017;15(2):141–8. [Google Scholar]
59. * Tao Z, Wu G, Wang Z. The relationship between high residential density in student dormitories and anxiety, binge eating and Internet addiction: a study of Chinese college students. SpringerPlus. 2016;5:1579. [Google Scholar] [PubMed]
60. * Ostovar S, Allahyar N, Aminpoor H, Moafian F, Nor MBM, Griffiths MD. Internet addiction and its psychosocial risks (depression, anxiety, stress and loneliness) among Iranian adolescents and young adults: a structural equation model in a cross-sectional study. Int J Ment Health Ad. 2016;14:257–67. [Google Scholar]
61. * Liu W. Analysis of the consequences of Internet overuse and related factors among college students (Master’s Thesis). Central South University: Hunan, China; 2011. [Google Scholar]
62. * Seifi A, Ayati M, Fadaei M. The study of the relationship between Internet addiction and depression, anxiety and stress among students of Islamic Azad University of Birjand. Int J Econ Manage Soc Sci. 2014;3:28–32. [Google Scholar]
63. * Wang P, Yuan Q, Wang H. The mediating role of college students’ self-esteem on social anxiety and Internet addiction. Chinese J Behav Med Brain Sci. 2011;6:558–9. [Google Scholar]
64. * Panicker J, Sachdev R. Relations among loneliness, depression, anxiety, stress and problematic Internet use. Int J Res Appl Nat Soc Sci. 2014;2(9):1–10. [Google Scholar]
65. * Javaeed A, Bint Zafar M, Iqbal M, Ghauri SK. Correlation between Internet addiction, depression, anxiety and stress among undergraduate medical students in Azad Kashmir. Pak J Med Sci. 2019;35(2):506–9. [Google Scholar] [PubMed]
66. * Zhang J. The effects of basic psychological needs on adolescents’ problematic mobile social network use: a moderated mediation model. Liaocheng University: Liaocheng, China; 2020. [Google Scholar]
67. Cheng C, Li AYL. Internet addiction prevalence and quality of (real) life: a meta-analysis of 31 nations across seven world regions. Cyberpsychol Behav Soc Netw. 2014;17(12):755–60. [Google Scholar] [PubMed]
68. * Kheyri F, Azizifar A, Valizadeh R, Veisani Y, Aibod S, Cheraghi F, et al. Investigation the relationship between Internet dependence with anxiety and educational performance of high school students. J Educ Health Promot. 2019;29(8):213. [Google Scholar]
69. * Shan X, Ou Y, Ding Y, Yan H, Chen J, Zhao J, et al. Associations between Internet addiction and gender, anxiety, coping styles and acceptance in university freshmen in South China. Front Psychiatry. 2021;12:558080. [Google Scholar] [PubMed]
70. * Koc M. Internet addiction and psychopathology. Turk Online J Educ Technol-TOJET. 2011;10(1):143–8. [Google Scholar]
71. * Woon LSC. Internet addiction and mental well-being among secondary school students in a Malaysian district: a cross-sectional. J Clin Psychiat. 2020;23(4):526–8. [Google Scholar]
72. * Song Z. Pathological Internet use and academic performance of middle school students: a study of the mediating role of academic anxiety and group counseling. School of Psychology, Central China Normal University: Hubei, China; 2020. [Google Scholar]
73. * Jiang Y, Jin T. The relationship between narcissistic personality and adolescents’ problematic mobile social network use: the role of omission anxiety and positive self-presentation. Chinese Special Educ. 2018;11:64–70. [Google Scholar]
74. * Cao J, Zhou Y, Wang X, Zhang H, Yang J. An analysis of the advantages of predicting factors of Internet addiction among college students in Daqing City. Chinese J General Med. 2010;22:2482–4. [Google Scholar]
75. Zhou Y, Cao J, Wang X, Zhang H, Yang J. Analysis of college students’ Internet addiction status and influencing factors. Chinese General Med. 2010;13(31):3528–30. [Google Scholar]
76. Qiu J, Shen J, Wu Q, Yuan Q, Kuang X. Intervention study of EEG biofeedback therapy on Internet addiction in adolescent depression patients. China Med Innov. 2022;21:146–9. [Google Scholar]
77. * Adalıer A, Balkan E. The relationship between Internet addiction and psychological symptoms. Int J Glob Educ. 2012;1(2):42–9. [Google Scholar]
78. * Akin A, Iskender M. Internet addiction and depression, anxiety and stress. Int Online J Educ Sci. 2011;3(1):138–48. [Google Scholar]
79. * Al Shawi AF, Hameed AK, Shalal AI, Abd Kareem SS, Majeed MA, Humidy ST. Internet addiction and its relationship to gender, depression and anxiety among medical students in Anbar Governorate-West of Iraq. Commun Health Equity Res Policy. 2022;42(3):253–6. [Google Scholar]
80. * Ali A, Horo A, Swain MR, Gujar NM, Gujar NM, Deuri SP. The prevalence of Internet addiction and its relationship with depression, anxiety and stress among higher secondary school students: a North-East perspective. J Indian Assoc for Child Adolesc Ment Health. 2019;15(1):13–26. [Google Scholar]
81. * Ayar D, Gerçeker G Ö, Özdemir EZ, Bektas M. The effect of problematic Internet use, social appearance anxiety, and social media use on nursing students’ nomophobia levels. CIN: Comput Inform Nurs. 2018;36(12):589–95. [Google Scholar]
82. * Azher M, Khan RB, Salim M, Bilal M, Hussain A, Haseeb M. The relationship between Internet addiction and anxiety among students of University of Sargodha. Int J Humanit Soc Sci. 2014;4(1):288–93. [Google Scholar]
83. * Bernal-Ruiz C, Rosa-Alcázar AI, González-Calatayud V, Rosa-Alcázar E. Is there a relationship between problematic Internet use and responses of social anxiety, obsessive-compulsive and psychological well-being among adolescents? Anales de Psicología. 2017;33(2):269–76. [Google Scholar]
84. * Chen Y, Zhong S, Dai L, Deng Y, Liu X. Effects of attachment anxiety on social network addiction among college students: a mediated model with moderation. Chinese J Clin Psychol. 2019;3:497−500+505. [Google Scholar]
85. * Choi SW, Kim DJ, Choi JS, Ahn H, Choi EJ, Song WY, et al. Comparison of risk and protective factors associated with smartphone addiction and Internet addiction. J Behav Addict. 2015;4(4):308–14. [Google Scholar] [PubMed]
86. * Çınar Özbay S, Kanbay Y, Firat M, Özbay Ö. The mediating effect of social anxiety on the relationship between Internet addiction and aggression in teenagers. Psychol Rep. 2022. doi:10.1177/00332941221133006. [Google Scholar] [PubMed] [CrossRef]
87. * Dalbudak E, Evren C, Aldemir S, Coskun KS, Ugurlu H, Yildirim FG. Relationship of Internet addiction severity with depression, anxiety, and alexithymia, temperament and character in university students. Cyberpsychol Behav Soc Netw. 2013;16(4):272–8. [Google Scholar] [PubMed]
88. * Dalbudak E, Evren C, Aldemir S, Evren B. The severity of Internet addiction risk and its relationship with the severity of borderline personality features, childhood traumas, dissociative experiences, depression and anxiety symptoms among Turkish university students. Psychiat Res. 2014;219(3):577–82. [Google Scholar]
89. * Ding Q, Wei H, Zhang Y, Zhou Z. Effects of self-concealment on Internet addiction among college students: multiple mediating roles of social anxiety and loneliness. Chinese J Clin Psychol. 2016;24(2):293–7. [Google Scholar]
90. * Dong H, Yang F, Lu X, Hao W. Internet addiction and related psychological factors among children and adolescents in China during the coronavirus disease 2019 (COVID-19) epidemic. Front Psychiatry. 2020;11:751. [Google Scholar]
91. * Du S, Wang C, Wang W, He H, Liu J, Gu H, et al. The relationship between social anxiety and Internet addiction among rural junior high school freshmen—The moderating role of whether or not to stay behind. China Spec Educ. 2020;8:61−66+87. [Google Scholar]
92. * Durak M, Durak EŞ. Associations of social anxiety and depression with cognitions related to problematic Internet use in youths. Eğitim ve Bilim. 2013;38(169):19–29. [Google Scholar]
93. * Erceg T, Buljan Flander G, Brezinšćak T. The relationship between compulsive Internet use and symptoms of depression and anxiety in adolescence. Alcoholism Psychiatry Res: J Psychiatr Res Addict. 2018;54(2):101–12. [Google Scholar]
94. * Gao H. The relationship between Internet addiction and anxiety in high school students: a moderated mediation effect analysis (Master’s Thesis). Mudanjiang Normal College: Mudanjiang, China; 2021. [Google Scholar]
95. * Gao T, Li J, Zhang H, Gao J, Kong Y, Hu Y, et al. Association between parental marital conflflict and Internet addiction: a moderated mediation analysis. J Affect Disord. 2018;24:27–32. [Google Scholar]
96. * Gholamian B, Shahnazi H, Hassanzadeh A. The prevalence of Internet addiction and its association with depression, anxiety, and stress, among high-school students. Int J Pediatr. 2017;5(4):4763–70. [Google Scholar]
97. * Goel D, Subramanyam A, Kamath R. A study on the prevalence of Internet addiction and its association with psychopathology in Indian adolescents. Indian J Psychiat. 2013;55(2):140–3. [Google Scholar]
98. * Guo X. The effects of social exclusion on problematic Internet use among college students: the roles of rejection sensitivity and social anxiety. (Master’s Thesis). Harbin Normal University: Harbin, China; 2021. [Google Scholar]
99. * Ismail WSW, Sim ST, Tan KA, Bahar N, Ibrahim N, Mahadevan R, et al. The relations of Internet and smartphone addictions to depression, anxiety, stress, and suicidality among public university students in Klang Valley, Malaysia. Perspect Psychiatr care. 2020;56(4):949–55. [Google Scholar]
100. * Kim N, Hughes TL, Park CG, Quinn L, Kong ID. Resting-state peripheral catecholamine and anxiety levels in Korean male adolescents with Internet game addiction. Cyberpsychol Behav Soc Netw. 2016;19(3):202–8. [Google Scholar] [PubMed]
101. * Lai CM, Mak KK, Watanabe H, Jeong J, Kim D, Bahar N, et al. The mediating role of Internet addiction in depression, social anxiety, and psychosocial well-being among adolescents in six Asian countries: a structural equation modelling approach. Public Health. 2015;129(9):1224–36. [Google Scholar] [PubMed]
102. * Lebni JY, Toghroli R, Abbas J, NeJhaddadgar N, Salahshoor MR, Mansourian M, et al. A study of Internet addiction and its effects on mental health: a study based on Iranian University students. J Educ Health Promot. 2020;9(1):205. [Google Scholar] [PubMed]
103. * Li X, Zheng X. The effect of parental alienation on left-behind children’s pathological Internet use: the mediating role of social anxiety. J Jiaozuo University. 2015;12:4. [Google Scholar]
104. * Li G, Hou G, Yang D, Jian H, Wang W. Relationship between anxiety, depression, sex, obesity, and Internet addiction in Chinese adolescents: a short-term longitudinal study. Addict Behav. 2019;90:421–7. [Google Scholar] [PubMed]
105. * Li X, Shek DT, Shek EY. Offline victimization, psychological morbidity, and problematic online behavior among Chinese secondary school students. Int J Env Res Public Health. 2021;18(18):9462. [Google Scholar]
106. * Li H. Social anxiety and adolescents’ online interpersonal relationship addiction and coping measures (Master’s Thesis). Central China Normal University: Hubei, China; 2022. [Google Scholar]
107. * Liu Y. The relationship between social efficacy and Internet dependence among middle school students (Master’s Thesis). Kashi University: Xinjiang, China; 2021. [Google Scholar]
108. * Malak MZ, Khalifeh AH, Shuhaiber AH. Prevalence of Internet addiction and associated risk factors in Jordanian school students. Comput Hum Behav. 2017;70:556–63. [Google Scholar]
109. * Mamun MA, Hossain MS, Siddique AB, Sikder MT, Kuss DJ, Griffiths MD. Problematic Internet use in Bangladeshi students: the role of socio-demographic factors, depression, anxiety, and stress. Asian J Psychiatry. 2019;44:48–54. [Google Scholar] [PubMed]
110. * Molavi P, Mikaeili N, Ghaseminejad MA, Kazemi Z, Pourdonya M. Social anxiety and benign and toxic online self-disclosures: an investigation into the role of rejection sensitivity, self-regulation, and Internet addiction in college students. J Nerv Ment Dis. 2018;206(8):598–605. [Google Scholar] [PubMed]
111. * Musa MAH, Vahedi M. Study of the relationship between Internet addiction and anxiety: determination of the extent of Internet addiction and anxiety among Iranian students. J Appl Environ Biol Sci. 2014;4(2):201–9. [Google Scholar]
112. * Naeim M, Rezaeisharif A, Zandian H. The relationship between Internet addiction and social adjustment, and test anxiety of the students of Ardabil University of Medical sciences. Shiraz E-Med J. 2020;21(11):e99209. [Google Scholar]
113. * Ni X, Yan H, Chen S, Liu Z. Factors influencing Internet addiction in a sample of freshmen university students in China. Cyberpsychol Behav. 2009;12(3):327–30. [Google Scholar] [PubMed]
114. * Odacı H, Kalkan M. Problematic Internet use, loneliness and dating anxiety among young adult university students. Comput Educ. 2010;55(3):1091–97. [Google Scholar]
115. * Odacı H, Çikrıkci Ö. Differences in problematic Internet use based on depression, anxiety, and stress levels. Addict: Turk J Addict. 2017;4(1):41–61. [Google Scholar]
116. * Othman Z, Lee CW. Internet addiction and depression among college students in Malaysia. Int Med J. 2017;24(6):447–50. [Google Scholar]
117. * Ozturk FO, Ekinci M, Ozturk O, Canan F. The relationship of affective temperament and emotional-behavioral difficulties to Internet addiction in Turkish teenagers. Int Scholarly Res Notices. 2013;28:1–6. [Google Scholar]
118. * Peng J. The relationship between social anxiety and problematic Internet use among high school students: a study of the mediating role of misplaced fear and its intervention. School of Psychology, Central China Normal University: Hubei, China; 2021. [Google Scholar]
119. * Radeef AS, Faisal GG. Prevalence of Internet addiction and its association with depression, anxiety and stress among medical students in Malaysia. Mediterr J Clin Psychol. 2018;6(3):1–17. [Google Scholar]
120. * Ranjan LK, Gupta PR, Srivastava M, Gujar NM. Problematic Internet use and its association with anxiety among undergraduate students. Asian J Soc Health Behav. 2021;4(4):137–41. [Google Scholar]
121. * Sayed M, Naiim CM, Aboelsaad M, Ibrahim MK. Internet addiction and relationships with depression, anxiety, stress and academic performance among Egyptian pharmacy students: a cross-sectional designed study. Bmc Public Health. 2022;22(1):1826. [Google Scholar] [PubMed]
122. * Shaikhamadi S, Yousefi F, Taymori P, Roshani D. The relationship between Internet addiction with depression and anxiety among Iranian adolescents. Chronic Dis J. 2017;5(2):41–9. [Google Scholar]
123. * Shen Y, Meng F, Xu H, Li X, Zhang Y, Huang C, et al. Internet addiction among college students in a Chinese population: prevalence, correlates, and its relationship with suicide attempts. Depress Anxiety. 2020;37(8):812–21. [Google Scholar] [PubMed]
124. * Stavropoulos V, Gomez R, Steen E, Beard C, Liew L, Griffiths MD. The longitudinal association between anxiety and Internet addiction in adolescence: the moderating effect of classroom extraversion. J Behav Addict. 2017;6(2):237–47. [Google Scholar] [PubMed]
125. * Sun C, Fei X, Xia W, Wu L. Analysis of the current situation of Internet addiction and its influencing factors among middle school students in Harbin. China School Health. 2007;4:331–2. [Google Scholar]
126. * Teng X, Lei H, Li J, Wen Z. Impact of social anxiety on social network addiction among college students: moderating effects of intentional self-regulation. Chinese J Clin Psychol. 2021;3:514–7. [Google Scholar]
127. * Tong W. Boredom and adolescents’ problematic mobile social network use: a multiple mediation model. Chinese J Clin Psychol. 2019;5(27):932–6. [Google Scholar]
128. * Tras Z, Öztemel K, BALTACI U. Role of problematic Internet use, sense of belonging and socialappearance anxiety in facebook use intensity of university students. Int Educ Studies. 2019;12(8):1–10. [Google Scholar]
129. * Tu W, Wang M, Li Y, Nie Y. The relationship between college students’ Internet attitudes and Internet addiction: chain-mediated effects. Chinese J Health Psychol. 2022;6:905–9. [Google Scholar]
130. * Wang L. Research on pathological Internet use and its influencing factors among college and high school students (Master’s Thesis). Jiangxi Normal University: Jiangxi, China;2004. [Google Scholar]
131. * Wang D, Zhang L, Zhang Z. A longitudinal study of the relationship between problematic Internet use and well-being, social anxiety, and depression. Psychol Behav Res. 2017;15(4):569. [Google Scholar]
132. * Wang Y. Research on the current situation and influencing factors of Internet addiction among college students in five universities in Anhui Province (Master’s Thesis). Wannan Medical College: Anhui, China; 2020. [Google Scholar]
133. * Wang T, Wei H, Lu X, Zhang K, Zhang X, Zhang J, et al. Relationship between social anxiety social support and depression of Internet addiction among senior college students. Chinese School Health. 2020;11:1610–3. [Google Scholar]
134. * Wang X. A study of the effects of peer relationships and social anxiety on the use of problematic social networks among middle school students and intervention (Master’s Thesis). Huaibei Normal University: Anhui, China; 2022. [Google Scholar]
135. * Xie X, Cheng H, Chen Z. Anxiety predicts Internet addiction, which predicts depression among male college students: a cross-lagged comparison by sex. Front Psychol. 2022;13:1–10. [Google Scholar]
136. * Yadav P, Banwari G, Parmar C, Maniar R. Internet addiction and its correlates among high school students: a preliminary study from Ahmedabad, India. Asian J Psychiatry. 2013;6(6):500–5. [Google Scholar] [PubMed]
137. * Yang Y. Perceived parental conflict and problematic network use among middle school students: a moderated mediation model (Master’s Thesis). Shandong Normal University: Shandong, China; 2017. [Google Scholar]
138. * Yang K. Research on the relationship between anxiety, depression, personality characteristics and Internet overuse among junior middle school students in Jinan (Master’s degree Thesis). Shandong University: Shandong, China; 2021. [Google Scholar]
139. * Yen JY, Ko CH, Yen CF, Chen SH, Chung WL, Chen CC. Psychiatric symptoms in adolescents with Internet addiction: comparison with substance use. Psychiat Clin Neuros. 2008;62(1):9–16. [Google Scholar]
140. * Yıldız Durak H. Modeling of variables related to problematic Internet usage and problematic social media usage in adolescents. Curr Psychol. 2020;39:1375–87. [Google Scholar]
141. * Younes F, Halawi G, Jabbour H, El Osta N, Karam L, Hajj A, et al. Internet addiction and relationships with insomnia, anxiety, depression, stress and self-esteem in university students: a cross-sectional designed study. PLoS One. 2016;11(9):e0161126. [Google Scholar] [PubMed]
142. * Yücens B, Üzer A. The relationship between Internet addiction, social anxiety, impulsivity, self-esteem, and depression in a sample of Turkish undergraduate medical students. Psychiat Res. 2018;267:313–8. [Google Scholar]
143. * Zhang H, Su L, Wang M. A study of the relationship between state-trait anxiety, self-esteem, and Internet addiction in higher vocational students. Chinese J Health Psychol. 2009;5:619–21. [Google Scholar]
144. * Zhang M, Zhao X, Sun Y, Hu X, Yang W, Zhao Q, et al. Internet addiction among college students and its impact on interaction anxiety. Chinese J Soc Med. 2013;1:39–41. [Google Scholar]
145. * Zhang Y, Liu Q, Long Z, Ai T. The relationship between trait anxiety and Internet addiction in college students: a moderated mediation model. Psychol Dev Educ. 2016;6:745–52. [Google Scholar]
146. * Zhang Z. College students’ loneliness and problematic social networking site use: the chain mediating role of social anxiety, social self-efficacy (Master’s Thesis). Shanghai Normal University: Shanghai, China; 2019. [Google Scholar]
147. * Zhang J, Chang F, Huang D, Wen X. Relationship between neurotic personality and adolescents’ problematic mobile social network use: the chain-mediated role of anxiety and positive self-presentation. Chinese J Clin Psychol. 2021;3(29):598–602. [Google Scholar]
148. * Zhang Y, Ding Q, Wang Z. Why parental phubbing is at risk for adolescent mobile phone addiction: a serial mediating model. Child Youth Serv Rev. 2021;121:105873. [Google Scholar]
149. * Zhang Y, Hou Z, Wu S, Li X, Hao M, Wu X. The relationship between Internet addiction and aggressive behavior among adolescents during the COVID-19 pandemic: anxiety as a mediator. Acta Psychol. 2022;227:103612. [Google Scholar]
150. Borenstein M, Cooper H, Hedges L, Valentine J. Effect sizes for continuous data. Handbook Res Synth Meta-Analysis. 2009;2:221–35. [Google Scholar]
151. Card NA. Applied meta-analysis for social science research. New York: Guilford Press; 2012. [Google Scholar]
152. Gignac GE, Szodorai ET. Effect size guidelines for individual differences researchers. Pers Indiv Differ. 2016;102:74–8. [Google Scholar]
153. Fan H, Li J, Zhao M, Li H. Mental health of kindergarten teachers: a meta-analysis of SCL-90 scale-based studies. Adv Psychol Sci. 2016;24(1):9–20. [Google Scholar]
154. Higgins JP, Thompson SG, Deeks JJ, Altman DG. Measuring inconsistency in meta-analyses. BMJ. 2003;327(7414):557–60. [Google Scholar] [PubMed]
155. Duval S, Tweedie R. A nonparametric “trim and fill” method of accounting for publication bias in meta-analysis. J Am Stat Assoc. 2000;95(449):89–98. [Google Scholar]
156. Sterne JA, Egger M. Regression methods to detect publication and other bias in meta-analysis. publication bias in meta-analysis: prevention, assessment and adjustments. New Jersey: John Wiley & Sons, Ltd.; 2005. p. 99–110. [Google Scholar]
157. Xu J, Guo S, Feng Y, Ma Y, Zhang Y, Núñez JC, et al. Parental homework involvement and students’ achievement: a three-level meta-analysis. Spain: Psicothema; 2024;1. [Google Scholar]
158. Cohen J. Set correlation and contingency tables. Appl Psych Meas. 1988;12(4):425–34. [Google Scholar]
159. Davis RA. A cognitive-behavioral model of pathological Internet use. Comput Hum Behav. 2001;17(2):187–95. [Google Scholar]
160. Kim HK, Davis KE. Toward a comprehensive theory of problematic Internet use: evaluating the role of self-esteem, anxiety, flow, and the self rated importance of Internet activities. Comput Hum Behav. 2009;25(2):490–500. [Google Scholar]
161. Campbell AJ, Cumming SR, Hughes I. Internet use by the socially fearful: addiction or therapy? Cyberpsychol Behav. 2006;9(1):69–81. [Google Scholar] [PubMed]
162. Tian Y, Bian Y, Han P, Gao F, Wang P. Associations between psychosocial factors and generalized pathological Internet use in Chinese university students: a longitudinal cross-lagged analysis. Comput Hum Behav. 2017;72:178–88. [Google Scholar]
163. Morahan-Martin J, Schumacher P. Loneliness and social uses of the Internet. Comput Hum Behav. 2003;19(6):659–71. [Google Scholar]
164. Caplan S, Williams D, Yee N. Problematic Internet use and psychosocial well-being among MMO players. Computers in Human Behav. 2009;25(6):1312–9. [Google Scholar]
165. Shen C, Williams D. Unpacking time online: connecting intemet and massively multiplayer online game use with psy chological well-being. communication Res. 2010;20(10):1–27. [Google Scholar]
166. Chen X. Culture, peer interaction, and socioemotional development. Child Dev Perspect. 2012;6(1):27–34. [Google Scholar]
167. Xu F, Zhang W. The relationship between adolescent alienation and pathological Internet use: a test of the moderating effects of family functioning and peer acceptance. Psychol J. 2011;43(4):410–9. [Google Scholar]
168. Helliwell JF, Huang H, Wang S. Changing world happiness. World Happiness Report. 2019. [Google Scholar]
169. Baxter AJ, Scott KM, Vos T, Whiteford HA. Global prevalence of anxiety disorders: a systematic review and meta-regression. Psychol Med. 2013;43(5):897–910. [Google Scholar] [PubMed]
170. Daniali H, Martinussen M, Flaten MA. A global meta-analysis of depression, anxiety, and stress before and during COVID-19. Health Psychol. 2023;42(2):124–38. [Google Scholar] [PubMed]
Cite This Article
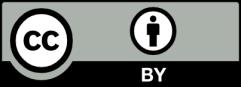