Open Access
ARTICLE
The Early Emotional Responses and Central Issues of People in the Epicenter of the COVID-19 Pandemic: An Analysis from Twitter Text Mining
1 Department of Nursing, Kyungin Women’s University, Incheon, 21041, South Korea
2 Red Cross College of Nursing, Chung-Ang University, Seoul, 06974, South Korea
* Corresponding Author: Yun-Jung Choi. Email:
International Journal of Mental Health Promotion 2023, 25(1), 21-29. https://doi.org/10.32604/ijmhp.2022.022641
Received 18 March 2022; Accepted 28 September 2022; Issue published 29 November 2022
Abstract
This study aimed to explore citizens’ emotional responses and issues of interest in the context of the coronavirus disease 2019 (COVID-19) pandemic. The dataset comprised 65,313 tweets with the location marked as New York State. The data collection period was four days of tweets when New York City imposed a lockdown order due to an increase in confirmed cases. Data analysis was performed using R Studio. The emotional responses in tweets were analyzed using the Bing and NRC (National Research Council Canada) dictionaries. The tweets’ central issue was identified by Text Network Analysis. When tweets were classified as either positive or negative, the negative sentiment was higher. Using the NRC dictionary, eight emotional classifications were devised: “trust,” “fear,” “anticipation,” “sadness,” “anger,” “joy,” “surprise,” and “disgust.” These results indicated that citizens showed negative and trusting emotional reactions in the early days of the pandemic. Moreover, citizens showed a strong interest in overcoming and coping with other people such as social solidarity. Citizens were concerned about the confirmation of COVID-19 infection status and death. Efforts should be made to ensure citizens’ psychological stability by promptly informing them of the status of infectious disease management and the route of infection.Graphic Abstract
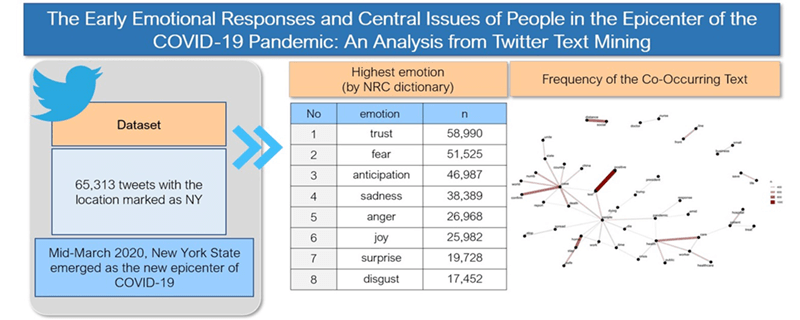
Keywords
On March 13, 2022, the World Health Organization (WHO) announced that over 455 million confirmed cases and over 6 million deaths have been reported globally from coronavirus disease 2019 (COVID-19) [1]. This novel coronavirus infection had spread worldwide since it was first reported in December 2019 in Hubei Province, China [2]. By mid-March 2020, New York State emerged as the new epicenter of COVID-19 as the number of cases increased. The governor of New York ordered citizens to stay indoors to contain the spread and closed businesses. In addition, hospitals reported daily shortages of ventilators and masks [3].
The pandemic has caused anxiety, stress, and tension among citizens [4–6]. Most concerns surrounding COVID-19 are related to quarantine and infection statistics, whereas the emotions and major interests of citizens have not been considered [7,8]. After the Severe acute respiratory syndrome (SARS) and the Ebola virus outbreaks of 2003 and 2014, respectively, citizens reported experiencing long-term fear and overreaction [9,10]. Therefore, it is important to study the sensibilities and main interests of citizens during the COVID-19 pandemic to protect their mental health and psychological stability [11].
The WHO recommends that individuals share their experiences during COVID-19. Additionally, it encourages individuals to maintain their daily life and to exchange up-to-date information while maintaining engagement on Social Network Services (SNS) [12]. Son et al. have reported that, in the event of a disaster, citizens quickly propagate social issues related to shocking events through social networks and express their experiences, attitudes, and sensibility to the events honestly [13,14]. However, few studies have identified the public emotions and central themes through SNS data during the COVID-19 pandemic. Data collected from SNS are important resources that can be used for various policies and decisions [15,16].
This study aimed to analyze the sentiments of citizens during the COVID-19 pandemic to investigate their emotional responses and identify their central issues of interest.
2.1 SNS Data Collection and Processing
Data were collected for 4 days: March 26–28, and April 02, 2020. We collected tweets showing location information from New York State. This is because the number of confirmed COVID-19 cases was rapidly increasing in New York when compared with other regions, and the lack of medical services has been widely reported [17,18]. The search query for data collection was: “#covid19 or #covid-19 or #coronavirus or #covid2019 or #covid_19 or #CoronavirusOutbreak or #COVID-19.” Tweets duplicated in languages other than English were deleted. As a first step in the preprocessing stage, special symbols, abbreviations, emoticons, numbers, URLs, and hashtags were removed. The second step was to change punctuation, spaces, and uppercase letters to lowercase. Using the SMART dictionary, unnecessary words such as articles and pronouns were treated as stopwords. The words “covid,” “corona,” “virus,” and “coronavirus” were removed because of their high frequency but low importance. The third step completed lemmatization, a process of changing the form of derived words to the basic, root form.
SNS data analysis was performed using the R Studio Version 1.3.959. After deleting duplicate tweets from 65,313 tweets, 26,466 were used for data analysis. After pre-processing, the number of words decreased from 837,868 to 608,229.
First, during the COVID-19 pandemic, citizens’ emotions were analyzed using sentiment analysis. In this study, we analyzed the data based on the sentiment dictionary provided through the tidy text package. The Bing dictionary divides 6,778 words into positive and negative categories. The National Research Council Canada (NRC) dictionary divides 13,891 words into eight categories: anger, anticipation, disgust, fear, joy, sadness, surprise, and trust. One word can belong to several emotions at the same time; for example, the word “abandon” is included simultaneously in the negative, sadness, and fear categories. In this study, the text was classified into positive and negative through the Bing dictionary; eight emotions were classified using the NRC dictionary to analyze public emotion.
Second, citizens’ interest was identified using the Text Network Analysis method, especially co-occurrence network analysis. Text network analysis refers to a method of displaying a relationship between the text as a link and analyzing the connection and relationship structurally [19]. The basic unit of analysis is a node; in this study “text” was the node. The higher the number of nodes connected to one node, the higher the centrality of the connection. This indicated higher importance within the network [20]. If the number of co-occurrences is adjusted to low for this analysis, the structure of the network is complicated; therefore, it is difficult to analyze the contents. In this study, co-occurrence was identified in the structure >200 times; thicker connection lines indicated greater frequency.
This study aimed to analyze user sentiment in the COVID-19 pandemic region of New York State to assess emotional responses and identify the central issues of interest to individuals during that time. The top 10 words, which appeared >1500 times, were “people,” “case,” “pandemic,” “time,” “test,” “work,” “crisis,” “health,” “make,” and “Trump” (Fig. 1).
Figure 1: Top 20 words tweeted more than 1500 times
The analysis results of the tweets related to COVID-19 using the Bing dictionary showed that negative sentiment was higher than positive sentiment (52,088 and 38,387 negative and positive sentiment words, respectively. The proper noun, “Trump” (relating to the US president at the time) word and “positive,” which indicated confirmation of a COVID-19 infection, were classified as positive; therefore, they were excluded from the sentiment analysis. The top 10 words that appeared as negative words were “crisis,” “death,” “outbreak,” “die,” “risk,” “numb,” “emergency,” “symptom,” “lose,” and “break.” Positive words were “work,” “patient,” “support,” “safe,” “good,” “relief,” “great, “protect,” and “love” (Fig. 2).
Figure 2: Top 20 emotional texts using the Bing dictionary
The analysis of the tweets using the NRC dictionary showed that the highest emotion expressed by citizens in the COVID-19 pandemic at this time in New York State was “trust” = 58,990. This was followed by, “fear” = 51,525, “anticipation” = 46,987, “sadness” = 38,389, “anger” = 26,968, “joy” = 25,982, “surprise” = 19,728, and “disgust” = 17,452. The trust emotions were: “hospital,” “medical,” “safe,” “share,” “show,” “continue,” “good,” “president,” “doctor,” and “provide.” The fear emotions were: “case,” “pandemic,” “death,” “hospital,” “fight,” “medical,” “watch,” “die,” “risk,” “government,” and “emergency” (Fig. 3).
Figure 3: Top 10 emotional texts using the NRC dictionary
Next, a co-occurrence network analysis was conducted on the text network analysis results to identify the central issue for citizens during the COVID-19 pandemic. This analysis included “Trump” and “positive.” The analysis indicated that the most common co-occurring texts were: “test”–“positive” = 958, “social”–“distance” = 771, “stay”–“home” = 726, “confirm”–“case” = 677, and “health”–“care” = 526 (Table 1).
In the entire network, words associated with words that were highly central to connectivity were grouped into one subnetwork group. Sub-groups were classified into “people,” “case,” and “health,” which represented the central issues of interest to citizens. The words associated with the “people” group were: “dying,” “pandemic,” “die,” “time,” “work,” “home,” “spread,” “case,” and “test.” Words associated with the “case” group were: “country,” “China,” “people,” “death,” “report,” “confirm,” “world,” “numb,” and “state.” Words associated with the “health” group were: “pandemic,” “care,” “worker,” “public,” “crisis,” and “die” (Fig. 4).
Figure 4: Co-occurrence network
This study collected tweets in mid-March when New York State emerged as a COVID-19 hotspot and a lockdown policy was announced. Data were analyzed to assess sentiment and word co-occurrence frequency. This study aimed to identify the sentiments and interests of citizens in the early stages of a pandemic.
The analysis showed that words were more likely to be classified as having negative emotions than positive; however, when words were classified into eight categories, the “trust” emotion was high. Interestingly, in the early stages of the pandemic, citizens showed strong trust emotions to overcome this situation and interests in their community, treatment, and sharing. A study of the emotions experienced by earthquake survivors has revealed that disaster survivors value relationships more and experience positive emotions of worrying about others even in painful situations [21]. These results are similar to this study. An additional study of disaster survivors in Europe has reported that survivors calmly take the first action to help others, followed by an assessment of the severity of the situation [22]. This result shows that people who have suffered a crisis have a sense of solidarity toward others from the beginning of the disaster.
The second highest emotion was “fear.” Factors that trigger negative emotional responses from citizens may be related to information on COVID-19, such as confirmed cases, risks, and government announcements. Uncertain symptoms and transmission of COVID-19 can cause additional fears and negatively affect the mental health of citizens [11]. In a study that analyzed tweets in Japan, citizens reported fear and anxiety in the early days of an earthquake [23]. Another study analyzed tweets from May 10–24, 2020, when New York City eased its COVID-19 lockdown; they have reported that the dominant theme perceived by the public is ‘fear,’ which supports this study’s results [24]. The feeling of fear may increase the level of social confusion in crisis situations. In West Africa, the Ebola virus killed 11,000 people in approximately four years since 2013. In this case, citizens were afraid of contagious diseases and they took actions that prevented treatment, such as escaping, avoiding treatment, and physical contact. This further spread the infectious disease [9,25]. In a pandemic situation, it is important to understand the citizens’ fears and identify the causes of their fears. At the time of the tweet collection, New York had emerged as a new hotspot of infection worldwide. In the news every day, the number of confirmed cases, social lockdown measures, and shortages of medical supplies dominated the media [3]. Tweets during the 2014 Ebola virus outbreak showed that Twitter users sought health information about the epidemic. The message of the show media can either amplify or reduce citizens’ fear [9]. Several studies have found that the fear, depression, and anxiety experienced in a COVID-19 situation can cause psychological distress, such as post-traumatic stress [26,27]. Therefore, it is important to identify the cause of citizens’ fear and provide sufficient information by recognizing that negative emotions can cause citizens’ posttraumatic stress.
The central issues during the COVID-19 pandemic were identified in three subgroups. First, through the sub-group “people,” citizens identified the issue of “staying in the house” as a result of “fear of death” and “diagnostic tests.” It is presumed that citizens are sharing the news of what people are experiencing through tweets [28]. At the time, New York State had just implemented a lockdown order. Therefore, citizens and the government’s guidelines are recommending staying at home. Citizens seemed to urgently share the situation of virus spread, COVID-19 policies, and social change through social networks. These results were similar to research showing that people share information and support through the use of social media during the COVID-19 pandemic [29]. A study analyzing the Twitter community also found cohesiveness and social connection among people within Twitter during the pandemic [30]. In a pandemic situation, citizens are trying to manage the crisis by promptly communicating the social problem. However, one study that compared news media tweets and citizen-generated tweets also found that citizens share emotions and personal experiences more than logical analyzes related to COVID-19 [31]. Citizens are more responsive to breaking news [31]; therefore, they will feel more secure when governments provide objective information correctly. Reliable information provided by the government was a factor that increased citizens’ protective behavior during COVID-19 [32,33]. This suggests that providing a lot of information about the situation, or news about others and how individuals can deal with the crisis, can help citizens’ psychological stability.
In the “case” group, interest in issues related to the pandemic such as the number of confirmed patients, the current situation in each country, for example, China and Italy, and the increase in mortality rate. This is similar to the findings of Karami et al. [34]; they analyzed tweets following a flood disaster and identified central themes for direct damage, such as “victim” and “damage”. Fake news can cause fear [35]; these findings suggest that a system to effectively deliver accurate information about the pandemic is necessary.
Finally, in the “health” group, positive issues about treatment appeared in relation to health, safety, and healthcare. In the early days of COVID-19, people cannot only communicate negative topics through tweets but also demonstrate a willingness to share and overcome the crisis. According to a study analyzing tweets after the Ebola outbreak, a positive role for tweets that alleviated people’s anxiety was reported [36]. Therefore, in the event of a national crisis, the use of SNS can help identify citizens’ interests and opinions and help guide the initial strategy during the crisis.
This study’s objective was to analyze citizen sentiments during the early stages of the COVID-19 pandemic in New York State to assess their emotional responses and identify their central issues. COVID-19 has spread rapidly worldwide and has had a tremendous social and economic impact. This study analyzed tweets during the four days of New York State’s lockdown order due to the spread of the virus.
Words from tweets were classified into eight emotions using the NRC dictionary; ‘trust’ occupied the highest emotion. This suggested that in the early stages of the crisis, citizens were interested in solidarity with others. The next highest emotion was ‘fear.’ This suggested that the government should quickly disseminate objective information to reduce citizens’ fears and provide psychological stability.
This study has several limitations. First, only tweets containing location information from New York State were analyzed. Therefore, generalizing the results of this study to the entire population is not possible because tweets that allow location information account for only 2% of the total tweets [37]. Second, the analysis method of this study only categorized emotions into eight categories, which prevents the analysis of complex emotions. Thus, further studies are recommended that apply a more rigorous methodology using an ongoing collection of tweets associated with COVID-19 which is openly available for researchers. Third, the co-occurrence word frequency described the number of simultaneous connections and may not accurately represent real interests of citizens’ lives. Fourth, this study did not present statistical rigor because it focused more on finding the meaning of the study results.
Nevertheless, Twitter is a useful tool for citizens to express their opinions quickly and can provide helpful insights into their feelings and interests at a specific point in time. It is significant that citizens have confirmed their interest in overcoming and coping with crisis situations through social network. They used terms such as health, treatment, social distance, and safety and negative issues such as confirmation, mortality, and death. In the future, the collection period should be expanded to broaden the research and analyze any change in emotions over time.
Funding Statement: This work was supported by the National Research Foundation of Korea (NRF) Grant Funded by the Korea Government (MSIT) (NRF-2020R1A2B5B0100208).
Conflicts of Interest: The authors declare that they have no conflicts of interest to report regarding the present study.
References
1. World Health Organization (2020). Coronavirus disease 2019 (COVID-19) situation report-83. https://www.who.int/docs/default-source/coronaviruse/situation-reports/20200412-sitrep-83-covid-19.pdf. [Google Scholar]
2. Li, Q., Guan, X., Wu, P., Wang, X., Zhou, L. et al. (2020). Early transmission dynamics in Wuhan, China, of novel coronavirus–infected pneumonia. New England Journal of Medicine, 382(13), 1199–1207. DOI 10.1056/NEJMoa2001316. [Google Scholar] [CrossRef]
3. McKinley, J. (2020). New York City region is now an epicenter of the coronavirus pandemic. The New York Times, 22. https://www.nytimes.com/2020/03/22/nyregion/Coronavirus-new-York-epicenter.html. [Google Scholar]
4. Censolo, R., Morelli, M. (2020). COVID-19 and the potential consequences for social stability. Peace Economics, Peace Science and Public Policy, 26(3). DOI 10.1515/peps-2020-0045. [Google Scholar] [CrossRef]
5. Lakhan, R., Agrawal, A., Sharma, M. (2020). Prevalence of depression, anxiety, and stress during COVID-19 pandemic. Journal of Neurosciences in Rural Practice, 11(4), 519–525. DOI 10.1055/s-0040-1716442. [Google Scholar] [CrossRef]
6. Deng, J., Zhou, F., Hou, W., Silver, Z., Wong, C. et al. (2021). The prevalence of depression, anxiety, and sleep disturbances in COVID-19 patients: A meta-analysis. Annals of the New York Academy of Sciences, 1486(1), 90–111. DOI 10.1111/nyas.14506. [Google Scholar] [CrossRef]
7. van Dorn, A., Cooney, R. E., Sabin, M. L. (2020). COVID-19 exacerbating inequalities in the US. Lancet, 395, 1243–1244. DOI 10.1016/S0140-6736(20)30893-X. [Google Scholar] [CrossRef]
8. Torales, J., O’Higgins, M., Castaldelli-Maia, J. M., Ventriglio, A. (2020). The outbreak of COVID-19 coronavirus and its impact on global mental health. International Journal of Social Psychiatry, 66, 317–320. DOI 10.1177/0020764020915212. [Google Scholar] [CrossRef]
9. Shultz, J. M., Cooper, J. L., Baingana, F., Oquendo, M. A., Espinel, Z. et al. (2016). The role of fear-related behaviors in the 2013–2016 West Africa Ebola virus disease outbreak. Current Psychiatry Reports, 18, 104. DOI 10.1007/s11920-016-0741-y. [Google Scholar] [CrossRef]
10. Mak, I. W. C., Chu, C. M., Pan, P. C., Yiu, M. G. C., Chan, V. L. (2009). Long-term psychiatric morbidities among SARS survivors. General Hospital Psychiatry, 31, 318–326. DOI 10.1016/j.genhosppsych.2009.03.001. [Google Scholar] [CrossRef]
11. Dong, L., Bouey, J. (2020). Public mental health crisis during COVID-19 pandemic, China. Emerging Infectious Diseases, 26, 1616–1618. DOI 10.3201/eid2607.200407. [Google Scholar] [CrossRef]
12. World Health Organization (2020). Mental health and psychosocial considerations during the COVID-19 outbreak. https://www.who.int/docs/default-source/coronaviruse/mental-health-considerations.pdf. [Google Scholar]
13. Son, S., Kim, D., Gil, M. S., Moon, Y. S. (2017). A storm-based tag cloud platform for multiple SNS users. IEEE 3rd International Conference on Big Data Security on the Cloud. IEEE International Conference on High Performance and Smart Computing; IEEE International Conference on Intelligent Data and Security, pp. 185–189. Beijing, China. DOI 10.1109/BigDataSecurity.2017.21. [Google Scholar] [CrossRef]
14. Woo, H. J., Kim, Y. H. (2017). Spatial distribution patterns of Twitter data with topic modeling. Journal of the Korean Association of Regional Geographers, 23, 376–387. DOI 10.26863/JKARG.2017.05.23.2.376. [Google Scholar] [CrossRef]
15. Kongthon, A., Haruechaiyasak, C., Pailai, J., Kongyoung, S. (2014). The role of social media during a natural disaster: A case study of the 2011 Thai flood. International Journal of Innovation and Technology Management, 11, 2227–2232. DOI 10.1142/S0219877014400124. [Google Scholar] [CrossRef]
16. Liu, S. B., Palen, L., Sutton, J., Hughes, A. L., Vieweg, S. (2008). In search of the bigger picture: The emergent role of on-line photo sharing in times of disaster. On-Line Photo Sharing in Times of Disaster. https://www.xueshufan.com/publication/193135713. [Google Scholar]
17. Olson, D. R., Huynh, M., Fine, A., Baumgartner, J., Castro, A. et al. (2020). Preliminary estimate of excess mortality during the COVID-19 outbreak—New York City, March 11–May 2, 2020. Morbidity and Mortality Weekly Report (MMWR), 69, 603–605. DOI 10.15585/mmwr.mm6919e5. [Google Scholar] [CrossRef]
18. Ross, J., Diaz, C. M., Starrels, J. L. (2020). The disproportionate burden of COVID-19 for immigrants in the Bronx, New York. JAMA Internal Medicine, 180(8), 1043–1044. DOI 10.1001/jamainternmed.2020.2131. [Google Scholar] [CrossRef]
19. Leydesdorff, L. (2011). ‘Meaning’ as a sociological concept: A review of the modeling, mapping and simulation of the communication of knowledge and meaning. Social Science Information, 50(3–4), 391–413. DOI 10.1177/0539018411411021. [Google Scholar] [CrossRef]
20. He, Q. (1999). Knowledge discovery through co-word analysis. Library Trends, 48, 133–159. [Google Scholar]
21. Vázquez, C., Cervellón, P., Pérez-Sales, P., Vidales, D., Gaborit, M. (2005). Positive emotions in earthquake survivors in El Salvador. Journal of Anxiety Disorders, 19, 313–328. DOI 10.1016/j.janxdis.2004.03.002. [Google Scholar] [CrossRef]
22. Grimm, A., Hulse, L., Preiss, M., Schmidt, S. (2014). Behavioural, emotional, and cognitive responses in European disasters: Results of survivor interviews. Disasters, 38(1), 62–83. DOI 10.1111/disa.12034. [Google Scholar] [CrossRef]
23. Vo, B. K. H., Collier, N. (2013). Twitter emotion analysis in earthquake situations. International Journal of Computational Linguistics and Applications, 4, 159–173. [Google Scholar]
24. Osakwe, Z. T., Ikhapoh, I., Arora, B. K., Bubu, O. M. (2021). Identifying public concerns and reactions during the COVID-19 pandemic on Twitter: A text-mining analysis. Public Health Nursing, 38(2), 145–151. DOI 10.1111/phn.12843. [Google Scholar] [CrossRef]
25. Espinola, M., Shultz, J. M., Espinel, Z., Althouse, B. M., Cooper, J. et al. (2016). Fear-related behaviors in situations of mass threat. Disaster Health, 3(4), 102–111. DOI 10.1080/21665044.2016.1263141. [Google Scholar] [CrossRef]
26. Villarreal-Zegarra, D., Copez-Lonzoy, A., Vilela-Estrada, A. L., Huarcaya-Victoria, J. (2021). Depression, post-traumatic stress, anxiety, and fear of COVID-19 in the general population and health-care workers: Prevalence, relationship, and explicative model in Peru. BMC Psychiatry, 21(1), 1–14. DOI 10.1186/s12888-021-03456-z. [Google Scholar] [CrossRef]
27. Abad, A., da Silva, J. A., de Paiva Teixeira, L. E. P., Antonelli-Ponti, M., Bastos, S. et al. (2020). Evaluation of fear and peritraumatic distress during COVID-19 pandemic in Brazil. Advances in Infectious Diseases, 10(3), 184–194. DOI 10.4236/aid.2020.103019. [Google Scholar] [CrossRef]
28. Simon, T., Goldberg, A., Aharonson-Daniel, L., Leykin, D., Adini, B. (2014). Twitter in the cross fire—The use of social media in the Westgate Mall terror attack in Kenya. PLoS One, 9(8), e104136. DOI 10.1371/journal.pone.0104136. [Google Scholar] [CrossRef]
29. Abbas, J., Wang, D., Su, Z., Ziapour, A. (2021). The role of social media in the advent of COVID-19 pandemic: Crisis management, mental health challenges and implications. Risk Management and Healthcare Policy, 14, 1917–1932. DOI 10.2147/RMHP.S284313. [Google Scholar] [CrossRef]
30. Odlum, M., Hwayoung, C. H. O., Broadwell, P., Davis, N., Patrao, M. et al. (2020). Application of topic modeling to Tweets to learn insights on the African American lived experience of COVID-19. Studies in Health Technology and Informatics, 272, 24–27. DOI 10.3233/SHTI200484. [Google Scholar] [CrossRef]
31. Han, C., Yang, M., Piterou, A. (2021). Do news media and citizens have the same agenda on COVID-19? An empirical comparison of Twitter posts. Technological Forecasting and Social Change, 169, 120849. DOI 10.1016/j.techfore.2021.120849. [Google Scholar] [CrossRef]
32. Ning, L., Niu, J., Bi, X., Yang, C., Liu, Z. et al. (2020). The impacts of knowledge, risk perception, emotion and information on citizens’ protective behaviors during the outbreak of COVID-19: A cross-sectional study in China. BMC Public Health, 20(1), 1–12. DOI 10.1186/s12889-020-09892-y. [Google Scholar] [CrossRef]
33. Mansoor, M. (2021). Citizens’ trust in government as a function of good governance and government agency’s provision of quality information on social media during COVID-19. Government Information Quarterly, 38(4), 101597. DOI 10.1016/j.giq.2021.101597. [Google Scholar] [CrossRef]
34. Karami, A., Shah, V., Vaezi, R., Bansal, A. (2020). Twitter speaks: A case of national disaster situational awareness. Journal of Information Science, 46, 313–324. DOI 10.1177/0165551519828620. [Google Scholar] [CrossRef]
35. Lazard, A. J., Scheinfeld, E., Bernhardt, J. M., Wilcox, G. B., Suran, M. (2015). Detecting themes of public concern: A text mining analysis of the Centers for Disease Control and Prevention’s Ebola live Twitter chat. American Journal of Infection Control, 43, 1109–1111. DOI 10.1016/j.ajic.2015.05.025. [Google Scholar] [CrossRef]
36. Ratzan, S. C., Moritsugu, K. P. (2014). Ebola crisis-communication chaos we can avoid. Journal of Health Communication, 19, 2013–2015. DOI 10.1080/10810730.2014.977680. [Google Scholar] [CrossRef]
37. Burton, S. H., Tanner, K. W., Giraud-Carrier, C. G., West, J. H., Barnes, M. D. (2012). “Right time, right place” health communication on Twitter: Value and accuracy of location information. Journal of Medical Internet Research, 14(6), e2121. DOI 10.2196/jmir.2121. [Google Scholar] [CrossRef]
Cite This Article
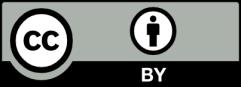
This work is licensed under a Creative Commons Attribution 4.0 International License , which permits unrestricted use, distribution, and reproduction in any medium, provided the original work is properly cited.