Open Access
ARTICLE
Design an Artificial Neural Network by MLP Method; Analysis of the Relationship between Demographic Variables, Resilience, COVID-19 and Burnout
1
Office of Research and Development, Tunghai University, Taichung, 407224, Taiwan
2
Nanyang Institute of Management, The Central 059817, Singapore
3
Krirk University, Thanon Ram Intra, Khwaeng Anusawari, Khet Bang Khen, Krung Thep Maha Nakhon, 10220, Thailand
4
National Taiwan Normal University, Graduate Institute of Information and Computer Education, Taipei, 10610, Taiwan
5
Institute of Optics and Quantum Electronics, Friedrich Schiller University Jena, Jena, 07743, Germany
6
Managamenet and Entrepreneurship Department, Razi University, Kermanshah, 67146, Iran
* Corresponding Author: Ehsan Eftekhari-Zadeh. Email:
International Journal of Mental Health Promotion 2022, 24(6), 825-841. https://doi.org/10.32604/ijmhp.2022.021899
Received 11 January 2022; Accepted 01 July 2022; Issue published 28 September 2022
Abstract
In addition to the effect that the COVID-19 pandemic has had on the physical and mental health of individuals, it has also led to a change in the mental and emotional state of many employees. Especially among businesses and private companies, which faced many restrictions due to the special conditions of the pandemic. Therefore, the present study aimed to design an artificial neural network with MLP technique to analyze the relationship between demographic variables, resilience, COVID-19 and burnout in start-ups in Iran. The research method was quantitative. Managers and employees of start-ups formed the statistical population of the study, based on the statistical sample size of the unlimited community, 384 of them were tested. For data gathering, standard questionnaires include of MBI-GS and BRCS and researcher-made questionnaire of stress caused by COVID-19 were used. The validity of the questionnaires was confirmed by a panel of experts and their reliability was confirmed by Cronbach’s alpha coefficient. The number of neurons in the input layer was equal to 10, the number of neurons in the 1st hidden layer was equal to 7, the number of neurons in the output layer was equal to 1, and the number of epochs was equal to 500. 70% of the data were used for training and 30% for testing. In the designed artificial neural network, all experiment data except one were correctly predicted and the obtained MAE error was less than 0.012%. Finally, he precision and correction of the presented model was confirmed by the obtained results.Keywords
The World Health Organization identified COVID-19 as a widespread pandemic on March 11, 2020, when infections and deaths increased exponentially. The first case was reported in Wuhan, China [1]. According to statistics, from the beginning of this pandemic until September 30, 2021, 233,136,147 cases have been infected worldwide, of which 4,771,408 have died [2]. However, COVID-19 is not just a major health crisis, but the virus is changing the structure of the global order in business and the economy [3]. Such pandemics, in addition to affecting the physical health of individuals, will also have long-term effects on mental health [4]. In other words, COVID-19 has affected the quality of life of many citizens, and the nature of the individual pandemic experience has varied from person to person depending on demographic and social factors [5]. During the pandemic, quarantine laws required citizens to reduce social interactions to reduce the risk of new infections [6]. Job closures, entertainment closures, increased working hours in hospitals and medical centers, travel bans, and the like were among the restrictions that were enforced in many countries. These protocols and restrictions, along with the fears and anxieties of individuals and families getting COVID-19, reduced communication and interaction, and consequently reduced business income, led to anxiety and mental distress for many people [7].
In this regard, one of the important consequences of chronic stress caused by COVID-19 is burnout; burnout is a psychological syndrome that occurs in response to chronic job-related stress and is characterized by occupational stressful experiences, increased workload, decreased work quality and social isolation [8]. In this case, the job loses its importance and meaning for the person. A person who has suffered from burnout, feels chronically exhausted and tired, has an aggressive mood, is somewhat pessimistic and suspicious in interpersonal relationships, and will be mostly negative [9]. Therefore, because the nature of some crises and experiences is such that people inevitably suffer from mental health problems, attention to individual and mental capacities with the help of which people can resist in crisis situations, has been considered by positive psychologists [10]. Psychologists believe that there are moderating factors between stressful events and psychological disorders that cause stressful events to have different effects on individuals [11]. As a result, researchers seek to reinforce variables within individuals that increase their level of adaptation and health [12]. One of these modifying personality traits is resilience. Resilience is one of the most important human abilities that causes effective adaptation to critical and stressful factors in stressful situations. Hence, resilience is recognized as a factor in successful adaptation to change and the ability to withstand adversity [13]. Resilience refers to the ability of a person to adapt in the face of experiences and crises, to overcome and even to be strengthened by those experiences [14].
Meanwhile, in Iran, according to the statistics of the World Health Organization, the number of patients with COVID-19 as of September 30, 2021, was 5,587,040, of which 120,428 died [15]. Given the foregoing and the restrictions and closures of many businesses, including start-ups, for controlling COVID-19, burnout for managers and their employees is inevitable, because many of these companies were forced to reduce their workforce, reduce their activity and consequently reduce sales and revenue, and sometimes shut down. These companies, unlike large companies, due to limited resources and start-ups, are not able to survive in conditions of instability for a long time and have many challenges, while COVID-19 is nearly two years old, it has led to restrictions for them. Therefore, considering that there is no immediate solution to reduce the stress and burnout of managers and employees of these start-ups, and the constant pressure has created a long-term harmful situation for start-ups that needs serious attention. Therefore, the main purpose of this study was to investigate the dimensions of burnout in the COVID-19 pandemic and to evaluate the relationship between them with demographic variables and resilience among managers and employees of start-ups.
The human living environment includes physical, psychological and social factors, each of which play a role in human health. A significant part of each person’s daily life is spent in the workplace [16]. So, one of the concepts that have been considered by, industrial psychologists in recent years is the signs, causes and effects of burnout. Burnout refers to the physical and mental fatigue caused by pressures in the workplace and self-employment as well as the symptoms and states of exhaustion, frustration, isolation and despair [17] and is one of the occupational hazards that has received serious attention in recent years due to its negative impact on employees, organizations and businesses [18]. Burnout is not only an issue arising from the sheer weakness or incapacity of employees, but also related to the workplace and the mismatch between individuals’ personal characteristics and the nature of their job [19]. In other words, burnout indicates a mismatch between the employee and his/her workplace, and its gradual growth leads to the use of inappropriate and ineffective coping strategies for protecting themselves from work-related stress [20].
Concepts of resilience in various scientific fields such as crisis management [21], medicine [22], supply chains [23,24] or collaboration networks [25] have been studied, but there is currently no consensus on a specific definition. Francis et al. defined resilience as the system’s ability to reduce the likelihood of shock, shock absorption if it occurs, and rapid recovery after a shock [26]. Resilience has been cited by Newman (2005) as an ability to cope with difficulties [27]. What prevents people from coping with stress are the methods they use to manage stress. These efficient methods are based on resilience characteristics [28]. Resilience, as a popular field in recent years, studies and discovers individual abilities and individual insights that lead to progress and resistance in difficult situations [29].
1. Investigating the relationship between resilience and burnout of managers and employees of start-ups.
2. Investigating the relationship between stress of COVID-19 and resilience of managers and employees of start-ups.
3. Investigating the relationship between stress of COVID-19 and burnout of managers and employees of start-ups.
4. Studying of the relationship between demographic variables with resilience and burnout and stress of COVID-19 among managers and employees of start-ups.
5. Design of artificial neural network including 10 input variables (five demographic variables including age, gender, marital status, job experience and children, stress of COVID-19, exhaustion, cynicism, professional efficiency, resilience) and burnout as output variables.
The present study is applied in terms of purpose and has a quantitative-cross-sectional approach. The study population was the managers and employees of start-ups in the country. According to the latest statistics available in the August 2021, 2223 start-ups are operating in the country. According to the protocol for estimating the sample size for an unlimited statistical population, 384 people were selected as the statistical sample. Samples were collected between early August 2021 and late September 2021.
In this study, a questionnaire was used for collecting data.
Maslach Burnout Inventory-general survey (MBI-GS) [30]: This questionnaire includes 3 dimensions of exhaustion, professional efficiency and cynicism. Exhaustion includes 6 items (1, 2, 3, 4, 6), professional efficiency includes 6 items (5, 7, 10, 11, 12, 16), and cynicism consists of 5 items (8, 9, 13, 14, 15). High exhaustion, low professional efficiency and high cynicism indicate burnout. To answer the six-point Likert scale as follows: 0 for never, 1 for several times a year or less, 2 for once a month or less, 3 for several times a month, 4 for once a week, 5 for several times a week and 6 for each day. Since the questionnaire was standard, its validity and reliability are confirmed. According to Maslach and Jackson (1981), the 22-item tool with Cronbach’s alpha was 0.9 for the exhaustion dimension, 0.79 for the cynicism dimension, 0.71 for the professional efficiency dimension, and finally, Cronbach’s alpha for all items was 0.76 [30]. Also, in the present study, to confirm the validity was used the opinions of panel of experts, and to confirm the reliability was used the pilot test, that Cronbach’s alpha calculated 0.82.
Brief Resilience Coping Scale (BRCS) [27]: This questionnaire includes 4 items on a Likert scale from 1 to 5. A score of 1 means “this item does not describe me at all” and a score of 5 means “this item describes me completely”. In this questionnaire, a score of 17 or higher indicates high resilience. This questionnaire also has validity and reliability due to its standardization [31] and Cronbach’s alpha for it is 0.86. Also in the present study, a panel of experts was used to confirm the validity and a pilot test was used to confirm the reliability. Cronbach’s alpha was calculated to be 0.83.
Researcher-made questionnaire for assessing stress of COVID-19: This questionnaire includes questions related to demographic variables such as age, gender, marital status, job experience, and children’s status, as well as 6 questions related to the assessment of stress caused by COVID-19. The validity of this questionnaire was confirmed by a panel of experts and Cronbach’s alpha for its reliability was calculated to be 0.79, which indicates the confirmation of the validity and reliability of the questionnaire.
Questionnaires were completed online. After approving the research protocol, managers and employees of start-ups were asked to participate in this study. It should be noted that the research information was reviewed confidentially.
The statistical samples tested in terms of demographic characteristics were described in Table 1. Accordingly, 167 of the statistical samples (43.5%) were women and 217 (56.5%) were men. The mean age of women was 40.43 years and the mean age of men was 42.84 years. Also, the average job experience of women was equal to 11.86 years and the job experience of men was equal to 14.63 years.
133 women were married and 34 were single, and for men 131 were married and 86 were single. Finally, 100 women and 118 men had children (Table 1).
The collected data were analyzed using SPSS software version 23 for descriptive statistics and inferential statistics and MATLAB software for artificial neural network design. Accordingly, burnout (presence vs. absence) with a nominal nature (presence of burnout = 0 and absence of 247 burnout = 1) was selected as the output or dependent variable. Also, 10 inputs were selected as follows:
• 3 nominal variables including gender (women = 1 & men = 0), marital status (single/widow/divorced = 0 & married = 1) and children (presence of child = 1 & no child = 0).
• Seven quantitative variables including age, job experience, stress of COVID-19, exhaustion, cynicism, professional efficiency and resilience.
The characteristics recognition of a complex nonlinear system has some analytical and mathematical solutions; using artificial neural networks (ANNs) is one of these solutions. As the idea of ANNs was expressed since now, there are some types of them, which help engineers in wide ranges of applications such as modeling, prediction, classification, clustering, pattern recognition, optimization and etc. [32]. The ANN type choosing depends on the issue that must be solved. Multi-layer perceptron (MLP) ANNs have been used widely in many applications such as classification and especially prediction [33–35]. MLP is a feed-forward network with at least three layers: input layer, hidden layer and output layer. Generally, the layers that are placed between the input and output layers can be more than one layers as hidden layers [35]. Each layer contains many neurons with an activation function, and each neuron is interconnected with weighted connections to neurons in the following layer, and also biased with a constant value [35]. The train procedure is the way of calculating the networks weights and biases. There are several methods for MLP training [32]. The number of hidden layers and neurons per hidden layers are adjusted during the training process to balance model accuracy during training [35]. In this work, Levenberg-Marquardt (LM) is applied to train the designed ANN. Because of many advantages [35,36], the LM algorithm has become a popular candidate in ANN learning, especially for MLP networks. For example, there are a small number of iterations with this algorithm. MATLAB software with version of R2016a is performed to train the proposed network.
By considering the type of the problem in this work (ten inputs and one output), the prototype MLP schematic with inputs of Ij (j is the number of inputs) and one output of Y can be illustrated as shown in Fig. 1. Therefore, the output can be achieved from:
Figure 1: The prototype a MLP with one output
where, n, b, Wi and fn are the number of hidden layers, the bias term for each neuron, the weighting factors and the activation function of the hidden layers, respectively. The equation related to the tansig activation function is given below:
The designed MLP topology in this study has been illustrated in Fig. 2. This topology includes ten inputs. The used inputs are: age, gender, job experience, marital status, children, stress of COVID-19, exhaustion, cynicism, professional efficacy and resilience and the output is the burnout. The designed MLP architecture contains one hidden layer. The only used hidden layer contains seven neurons.
Figure 2: Architecture of the designed MLP network
The used data-set was obtained from questionnaires and were divided into two parts: 70% (269 samples) as training data and 30% (115 samples) as testing data. The configuration of the designed network is illustrated in Table 2. It should be noted that the validation set was hidden in training set. Training set is presented to the network during training, and the network is adjusted according to its error. Validation set is used to measure network generalization, and to halt training when generalization stops improving. Testing set have no effect on training and so provide an independent measure of network performance during and after training. Therefore, in this study 269 samples (70%) of data has been used for training and 115 samples (30%) has been used for testing.
Many different architectures with different configurations were tested based on the below algorithm in order to find the optimized structure:
• The data set is defined.
• The counter parameters are defined.
• The desired error is defined.
• The initial values of other parameters for breaking loops are set.
• Several nested loops are created in order to test all of the arcituctures.
• ANNs with different number of hidden layers, neurons, epochs and activation functions are tested in created loops by usage of the defined counter parameters.
• The efficiency of network in each step is checked using the defined error.
• The best network with lowest error is saved.
The following results have been obtained in order to meet the objectives of the research:
The results of Pearson correlation analysis showed that burnout has a direct and significant relationship with exhaustion (rp = 0.594, p < 0.001) and cynicism (rp = 0.467, p < 0.001), but has a negative and significant relationship with professional efficiency (rp = −0.322, p < 0.01). Also, there is a significant negative relationship between resilience and burnout (rp = −0.222, p < 0.01), which means that with increasing resilience, the level of burnout has decreased.
The results of inferential statistics showed that the rate of stress of COVID-19 was 6.24 among men and 8 among women, which indicates a higher level of stress in women than men. Also, this stress was 8.94 among married women with children and 7.97 among married men.
According to Pearson analysis, there is a significant inverse relationship between stress of COVID-19 and resilience, meaning that with increasing stress caused by COVID-19, the level of resilience decreases (rp = −0.306, p < 0.01).
The relationship between stress of COVID-19 and burnout, based on statistical analysis, was direct and significant, meaning that with increasing stress of COVID-19, the level of burnout also increased (rp = 0.499, p < 0.001 ). Also, stress of COVID-19 has a direct and significant relationship with exhaustion (rp = 0.532, p < 0.001), with professional efficiency has an inverse and significant relationship (rp = −0.200, p < 0.01) and with cynicism has a direct and significant relationship. (rp = 0.427, p < 0.001).
The results obtained regarding the resilience of managers and employees of start-up showed that the average resilience of women was equal to 11.86 and the average resilience of men was equal to 13.73. Also, the resilience rate among married women with children was 9.44 and among married men with children was 10.06. Finally, the resilience rate for single women was 15.76 and for single men was 17.77. These results show that according to the standards of the resilience questionnaire where the number 13 and less indicates low resilience, it can be said that the level of resilience of single people is higher than married people. According to the results, 194 people (about 50% of the statistical population) had low resilience, 56 people (about 15% of the statistical population) had moderate resilience and 134 people (about 35% of the statistical population) had high resilience.
On the other hand, the results of inferential statistics show that 232 out of 384 people in the statistical population (61%) have burnout syndrome (high exhaustion, low professional efficiency and high cynicism). Of these, 115 are women and 117 are men. The results also show that burnout is higher among married men and women (221) than single men and women. Finally, the mean scores of the exhaustion variable were 3.99, the mean scores of the professional efficiency variable were 2.97 and the mean scores of the cynicism variable were 3.72, which were higher for the two variables of exhaustion and cynicism than the average for the Likert scale and lower for the professional efficiency variable. It has been one of the average scores of the Likert scale.
In this section, the results obtained for the first four objectives of the study were described and the relationship between demographic variables, dimensions of burnout, resilience and stress of COVID-19 was described using descriptive and inferential statistics. In the following, the artificial neural network designed with MLP technique is described, and the relationships between input and output variables are predicted.
A comparison between the MLP simulated results and the real results can be expressed as regression plots for training and testing operations. The regression plots were shown in Figs. 3 and 4, respectively.
Figure 3: The regression plot of training
Figure 4: The regression plot of testing
The real and predicted data for testing set were tabulated in Table 3 which shows the accuracy and precision of the presented model for predicting the burnout for every people based on 10 input features.
As be seen in Table 3, only the sample number 13 has been recognized wrongly and the rest data has been recognized correctly. In fact, 1 mistake in 384 samples show the very high precision of presented model which could be used in various applications.
The defined prevalent errors are Mean Absolute Error percentage (MAE %) and Root Mean Square error (RMSE) that are depicted in Table 4. The errors can be achieved with below equations [35]:
where N is the number of data and ‘X (Real)’ and ‘X (Predicted)’ are real data and predicted data using MLP network, respectively. There are very low obtained errors for the test data, which validate the proposed MLP network to model the burnout based on age, gender, job experience, marital status, children, stress of COVID-19, exhaustion, cynicism, professional efficacy and resilience.
The obtained results for burnout prediction using MLPNN and the detailed comparison with the real data are shown for training and testing sets in Figs. 5 and 6. These graphs show the very high precision of the presented model for burnout prediction.
Figure 5: Regression diagram and comparison of real data and predicted one for train data
Figure 6: Regression diagram and comparison of real data and predicted one for test data
The aim of this study was to investigate the relationship between demographic variables, resilience, COVID-19 and burnout with an artificial neural network design approach among start-ups in Iran. It was necessary to address this issue in the impact of the stress of COVID-19 on the state and performance of businesses and the mental state of managers and their employees. This issue has not been studied in other researches in the country so far and therefore the theoretical gap in this field has been very serious. Therefore, this research aimed at theorizing and reducing this theoretical gap with a practical purpose. For this purpose, in this study, five main objectives were examined;
According to the results, more than 65% of the statistical sample had medium and low resilience levels (16 and below). Also, 61% of the statistical sample have burnout. The rate of resilience is lower among women than men. In addition, the results show that among married men and women with children, there is the highest percentage of burnout. On the other hand, the rate of stress caused by COVID-19 is higher among women than men and the highest rate (8.94) is allocated to married women with children.
According to Pearson correlation analysis, the relationship between stress of COVID-19 and resilience is inverse and significant and with direct and significant burnout. Also, the relationship between resilience and burnout is negative and significant. Neural network analysis also shows that people with older age and more job experience who are married and have children have a higher rate of burnout, and this situation is more pronounced among women. Therefore, with the help of this network, with very high accuracy, by entering information about ten variables of age, gender, marital status, job experience and children, stress of COVID-19, exhaustion, professional efficiency, cynicism and resilience, burnout status of managers and explained and predicted the employees of start-up and to attract or not to attract them in the company, designed an incentive system for employees, designed a targeted incentive system, redesigned the employee evaluation system and the like.
Due to the fact that in research related to social sciences and humanities, less use is made of engineering methods such as neural network design, the present study is innovative in terms of subject and methodology and its results can be used by researchers and experts in the field. Business and entrepreneurship and organizational behavior, engineering sciences and sustainability issues, students and managers and employees of start-ups.
In this paper, a novel mathematical model based on MLP neural network was presented in order to predict the burnout in start-ups. The required data was gathered using questionnaires and were divided into two parts: 70% as training data and 30% as testing data. The best ANN architecture with lowest error was found using trial and error. The presented model has 10 inputs, 1 output and 1 hidden layer with 7 neurons. Age, gender, job experience, marital status, children, stress of COVID-19, exhaustion, cynicism, professional efficacy and resilience were considered as inputs and the burnout was considered as output. All of the training data was recognized correctly and only 1 mistake was occurred in the testing set. The obtained MAE error was less than 0.012% which is very low value. The precision and correction of the presented model was confirmed by the obtained results.
Authorship: The authors confirm contribution to the paper as follows: study conception and design: Chao-Hsi Huang, Tsung-Shun Hsieh, Saba Amiri; data collection: Saba Amiri; analysis and interpretation of results: Chao-Hsi Huang, Tsung-Shun Hsieh, Ehsan Eftekhari-Zadeh, Saba Amiri, Hsiao-Ting Chien; draft manuscript preparation: Tsung-Shun Hsieh. Hsiao-Ting Chien. All authors reviewed the results and approved the final version of the manuscript.
Funding Statement: We acknowledge support of the Open Access Publication Fund of the Thueringer Universitaets-und Landesbibliothek Jena.
Conflicts of Interest: The authors declare that they have no conflicts of interest to report regarding the present study.
References
1. WHO (2020). COVID-19–China. https://www.who.int/emergencies/disease-outbreak-news/item/2020-DON233. [Google Scholar]
2. WHO (2021a). Coronavirus disease (COVID-19) situation dashboard. https://COVID19.who.int/. [Google Scholar]
3. Mckinsey Company (2020). COVID-19 and the great reset: Briefing note. https://www.mckinsey.com/~/media/McKinsey/Business%20Functions/Risk/Our%20Insights/COVID%2019%20Implications%20for%20business/COVID%2019%20July%2030/COVID-19-Briefing-note-Number-16-July-30-2020.pdf. [Google Scholar]
4. Kisely, S., Warren, N., McMahon, L., Dalais, C., Henry, I. et al. (2020). Occurrence, prevention and management of the psychological effects of emerging virus outbreaks on healthcare workers: Rapid review and meta-analysis. British Medical Journal, 369, m1642. DOI 10.1136/bmj.m1642. [Google Scholar] [CrossRef]
5. Epifanio, M. S., Andrei, F., Mancini, G., Agostini, F., Piombo, M. et al. (2021). The impact of COVID-19 pandemic and lockdown measures on quality of life among Italian general population. Journal of Clinical Medicine, 10(2), 289. DOI 10.3390/jcm10020289. [Google Scholar] [CrossRef]
6. Marchetti, D., Fontanesi, L., di Giandomenico, S., Mazza, C., Roma, P. et al. (2020). The effect of parent psychological distress on child hyperactivity/inattention during the COVID-19 lockdown: Testing the mediation of parent verbal hostility and child emotional symptoms. Frontiers in Psychology, 11, 567052. DOI 10.3389/fpsyg.2020.567052. [Google Scholar] [CrossRef]
7. Rodríguez-Rey, R., Garrido-Hernansaiz, H., Collado, S. (2020). Psychological impact of COVID-19 in Spain: Early data report. Psychological Trauma: Theory, Research, Practice, and Policy, 12(5), 550–552. DOI 10.1037/tra0000943. [Google Scholar] [CrossRef]
8. Murali, A., Bhargava, A., Wright, E. S. (2018). IDTAXA: A novel approach for accurate taxonomic classification of microbiome sequences. Microbiome, 6(140), 1–14. DOI 10.1186/s40168-018-0521-5. [Google Scholar] [CrossRef]
9. Elghazally, S., Alkarn, A., Elkhayat, H., Ibrahim, A., Elkhayat, M. (2021). Burnout impact of COVID-19 pandemic on health-care professionals at Assiut university hospitals. International Journal of Environmental Research and Public Health, 18(10), 5368. DOI 10.3390/ijerph18105368. [Google Scholar] [CrossRef]
10. Linley, P., Joseph, S. (2004). Positive change following trauma and adversity: A review. Journal of Traumatic Stress, 17(1), 11–21. DOI 10.1002/(ISSN)1573-6598. [Google Scholar] [CrossRef]
11. Beltman, S. (2020). Understanding and examining teacher resilience from multiple perspectives. In Mansfield, C. F. (EdsCultivating teacher resilience. Springer, Singapore. DOI 10.1007/978-981-15-5963-1_2. [Google Scholar]
12. Mahdiani, H., Ungar, M. (2021). The dark side of resilience. Adversity and Resilience Science, 2(3), 147–155. DOI 10.1007/s42844-021-00031-z. [Google Scholar] [CrossRef]
13. Ahern, N., Norris, A. (2011). Examining factors that increase and decrease stress in adolescent community college students. Journal of Pediatric Nursing, 26(6), 530–540. DOI 10.1016/j.pedn.2010.07.011. [Google Scholar] [CrossRef]
14. Diener, E., Wirtz, D., Tov, W. (2010). New measures of well-being: Flourishing and positive and negative feelings. Social Indicators Research, 97(2), 143–156. DOI 10.1007/s11205-009-9493-y. [Google Scholar] [CrossRef]
15. WHO (2021b). Coronavirus disease (COVID-19) situation dashboard. https://COVID19.who.int/region/emro/country/ir. [Google Scholar]
16. Shoman, Y., El May, E., Marca, S. C., Wild, P., Bianchi, R. et al. (2021). Predictors of occupational burnout: A systematic review. International Journal of Environmental Research and Public Health, 18(17), 9188. DOI 10.3390/ijerph18179188. [Google Scholar] [CrossRef]
17. Kraft, U. (2006). The burned out. Scientific American Mind, 17(3), 28–33. [Google Scholar]
18. Nishimura, Y., Miyoshi, T., Hagiya, H., Kosaki, Y., Otsuka, F. (2021). Burnout of healthcare workers amid the COVID-19 pandemic: A Japanese cross-sectional survey. International Journal of Environmental Research and Public Health, 18(5), 2434. DOI 10.3390/ijerph18052434. [Google Scholar] [CrossRef]
19. Pressley, T. (2021). Factors contributing to teacher burnout during COVID-19. Educational Researcher, 50(5), 325–327. DOI 10.3102/0013189X211004138. [Google Scholar] [CrossRef]
20. Schaufeli, W., Rhenen, W. (2009). How changes in job demands and resources predict burnout, work engagement, and sickness absenteeism. Journal of Organizational Behavior, 30(7), 893–917. DOI 10.1002/job.595. [Google Scholar] [CrossRef]
21. Park, J. S., Okumura, Y., Tachikawa, H., Neiman, A. M. (2013). SPO71 encodes a developmental stage-specific partner for Vps13 in saccharomyces cerevisiae. Eukaryotic Cells, 12(11), 1530–1537. DOI 10.1128/EC.00239-13. [Google Scholar] [CrossRef]
22. Kim, T. Y., Kim, Y., Kim, J. Y. (2019). Role of resilience in (De) motivation and second language proficiency: Cases of Korean elementary school students. Journal of Psycholinguistic Research, 48(1), 1–20. DOI 10.1007/s10936-018-9609-0. [Google Scholar] [CrossRef]
23. Dolgui, A., Ivanov, D., Sokolov, B. (2018). Ripple effect in the supply chain: An analysis and recent literature. International Journal of Production Research, 56(1–2), 414–430. DOI 10.1080/00207543.2017.1387680. [Google Scholar] [CrossRef]
24. Zhao, K., Zuo, Z., Blackhurst, J. V. (2019). Modelling supply chain adaptation for disruptions: An empirically grounded complex adaptive systems approach. Journal of Operations Management, 65(6), 606–608. DOI 10.1002/joom.1046. [Google Scholar] [CrossRef]
25. Dixit, V., Verma, P., Tiwari, M. K. (2020). Assessment of pre and post-disaster supply chain resilience based on network structural parameters with CVaR as a risk measure. International Journal of Production Economics, 227(15), 107655. DOI 10.1016/j.ijpe.2020.107655. [Google Scholar] [CrossRef]
26. Francis, R., Bekera, B. (2014). A metric and frameworks for resilience analysis of engineered and infrastructure systems. Reliability Engineering & System Safety, 121(4), 90–103. DOI 10.1016/j.ress.2013.07.004. [Google Scholar] [CrossRef]
27. Newman, R. (2005). APA’s resilience initiative. Professional Psychology: Research and Practice, 36(3), 227–229. DOI 10.1037/0735-7028.36.3.227. [Google Scholar] [CrossRef]
28. Mcallister, M., McKinnon, J. (2009). The importance of teaching and learning resilience in the health disciplines: A critical review of the literature. Nurse Education Today, 29(4), 371–379. DOI 10.1016/j.nedt.2008.10.011. [Google Scholar] [CrossRef]
29. Alameddine, M., Bou-Karroum, K., Ghalayini, W., Abiad, F. (2021). Resilience of nurses at the epicenter of the COVID-19 pandemic in Lebanon. International Journal of Nursing Sciences, 8(4), 432–438. DOI 10.1016/j.ijnss.2021.08.002. [Google Scholar] [CrossRef]
30. Maslach, C., Jackson, S. (1981). Maslach Burnout Inventory, 20 ed, vol. 665, Palo Alto, CA, USA: Consulting Psychologists Press. [Google Scholar]
31. Moret-Tatay, C., Fernández-Muñoz, J. J., Civera-Mollá, C., Navarro-Pardo, E., Alcover-de-la-Hera, C. (2015). Propiedades psicométricas y estructura factorial del BRCS en una muestra de personas mayores españolas. Anales de Psicología/Annals of Psychology, 31(3), 1030–1034. DOI 10.6018/analesps.31.3.188401. [Google Scholar] [CrossRef]
32. Roshani, G. H., Farzin, S., Seyed, A. H. F. (2013). Some applications of artificial neural network in nuclear engineering. Republic of Moldova: LAP LAMBERT Academic Publishing. [Google Scholar]
33. Roshani, G. H., Seyed, A. H. F., Farzin, S., Abolfazl, S. (2014). Prediction of materials density according to number of scattered gamma photons using optimum artificial neural network. Journal of Computational Methods in Physics, 2014(1), 305345. DOI 10.1155/2014/305345. [Google Scholar] [CrossRef]
34. Roshani, G. H., Eftekhari-Zadeh, E., Shama, F., Salehizadeh, A. (2017). Combined application of neutron activation analysis using IECF device and neural network for prediction of cement elements. Radiation Detection Technology and Methods, 1(2), 23. DOI 10.1007/s41605-017-0025-z. [Google Scholar] [CrossRef]
35. Roshani, G. H., Nazemi, E., Shama, F. (2019). Utilizing features extracted from registered 60 co gamma-ray spectrum in one detector as inputs of artificial neural network for independent flow regime void fraction prediction. MAPAN, 34(2), 189–196. DOI 10.1007/s12647-018-0298-9. [Google Scholar] [CrossRef]
36. Gao, J., Zhang, Y., Du, Y., Li, Q. (2019). Optimization of the tire ice traction using combined Levenberg-Marquardt (LM) algorithm and neural network. Journal of the Brazilian Society of Mechanical Sciences and Engineering, 41(1), 40–41. DOI 10.1007/s40430-018-1545-2. [Google Scholar] [CrossRef]
Cite This Article
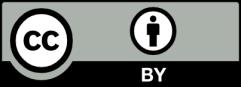