Open Access
ARTICLE
Multi-Neighborhood Enhanced Harris Hawks Optimization for Efficient Allocation of Hybrid Renewable Energy System with Cost and Emission Reduction
Department of Information Management, National Dong Hwa University, Hualien, 97401, Taiwan
* Corresponding Author: Elaine Yi-Ling Wu. Email:
(This article belongs to the Special Issue: Meta-heuristic Algorithms in Materials Science and Engineering)
Computer Modeling in Engineering & Sciences 2025, 143(1), 1185-1214. https://doi.org/10.32604/cmes.2025.064636
Received 20 February 2025; Accepted 19 March 2025; Issue published 11 April 2025
Abstract
Hybrid renewable energy systems (HRES) offer cost-effectiveness, low-emission power solutions, and reduced dependence on fossil fuels. However, the renewable energy allocation problem remains challenging due to complex system interactions and multiple operational constraints. This study develops a novel Multi-Neighborhood Enhanced Harris Hawks Optimization (MNEHHO) algorithm to address the allocation of HRES components. The proposed approach integrates key technical parameters, including charge-discharge efficiency, storage device configurations, and renewable energy fraction. We formulate a comprehensive mathematical model that simultaneously minimizes levelized energy costs and pollutant emissions while maintaining system reliability. The MNEHHO algorithm employs multiple neighborhood structures to enhance solution diversity and exploration capabilities. The model’s effectiveness is validated through case studies across four distinct institutional energy demand profiles. Results demonstrate that our approach successfully generates practically feasible HRES configurations while achieving significant reductions in costs and emissions compared to conventional methods. The enhanced search mechanisms of MNEHHO show superior performance in avoiding local optima and achieving consistent solutions. Experimental results demonstrate concrete improvements in solution quality (up to 46% improvement in objective value) and computational efficiency (average coefficient of variance of 24%–27%) across diverse institutional settings. This confirms the robustness and scalability of our method under various operational scenarios, providing a reliable framework for solving renewable energy allocation problems.Keywords
Cite This Article
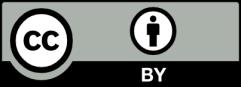
This work is licensed under a Creative Commons Attribution 4.0 International License , which permits unrestricted use, distribution, and reproduction in any medium, provided the original work is properly cited.