Open Access
ARTICLE
An Effective Lung Cancer Diagnosis Model Using Pre-Trained CNNs
1 Department of Business Information Technology, Princess Sumaya University for Technology, Amman, 11941, Jordan
2 Department of Computer Engineering, Al Yamamah University, Riyadh, 11512, Saudi Arabia
3 Department of Computer Science, John Jay College and the Graduate Center, The City University of New York, New York, NY 10019, USA
4 Department of Computer Science, John Jay College at the City University of New York, New York, NY 10019, USA
5 LIMOSE Laboratory, Department of Computer Science, M’hamed Bougara University, Boumerdes, 35000, Algeria
6 Department of Professional Security Studies, New Jersey City University, Jersey City, NJ 07305, USA
* Corresponding Author: Majdi Rawashdeh. Email:
(This article belongs to the Special Issue: Exploring the Impact of Artificial Intelligence on Healthcare: Insights into Data Management, Integration, and Ethical Considerations)
Computer Modeling in Engineering & Sciences 2025, 143(1), 1129-1155. https://doi.org/10.32604/cmes.2025.063765
Received 23 January 2025; Accepted 13 March 2025; Issue published 11 April 2025
Abstract
Cancer is a formidable and multifaceted disease driven by genetic aberrations and metabolic disruptions. Around 19% of cancer-related deaths worldwide are attributable to lung and colon cancer, which is also the top cause of death worldwide. The malignancy has a terrible 5-year survival rate of 19%. Early diagnosis is critical for improving treatment outcomes and survival rates. The study aims to create a computer-aided diagnosis (CAD) that accurately diagnoses lung disease by classifying histopathological images. It uses a publicly accessible dataset that includes 15,000 images of benign, malignant, and squamous cell carcinomas in the lung. In addition, this research employs multiscale processing to extract relevant image features and conducts a comprehensive comparative analysis using four Convolutional Neural Network (CNN) based on pre-trained models such as AlexNet, VGG (Visual Geometry Group)16, ResNet-50, and VGG19, after hyper-tuning these models by optimizing factors such as batch size, learning rate, and epochs. The proposed (CNN + VGG19) model achieves the highest accuracy of 99.04%. This outstanding performance demonstrates the potential of the CAD system in accurately classifying lung cancer histopathological images. This study contributes significantly to the creation of a more precise CNN-based model for lung cancer identification, giving researchers and medical professionals in this vital sector a useful tool using advanced deep learning techniques and publicly available datasets.Keywords
Cite This Article
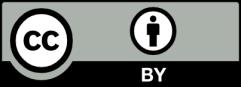
This work is licensed under a Creative Commons Attribution 4.0 International License , which permits unrestricted use, distribution, and reproduction in any medium, provided the original work is properly cited.