Open Access
ARTICLE
Performance vs. Complexity Comparative Analysis of Multimodal Bilinear Pooling Fusion Approaches for Deep Learning-Based Visual Arabic-Question Answering Systems
1 Electrical Engineering Department, Faculty of Engineering at Shoubra, Benha University, Cairo, 11629, Egypt
2 Computer Science Department, Faculty of Computing and Information Technology, King Abdulaziz University, Jeddah, 21589, Saudi Arabia
3 Department of Computer and Systems Engineering, Faculty of Engineering and Technology, Badr University in Cairo (BUC), Cairo, 11829, Egypt
4 Communication Systems Engineering Department, Faculty of Engineering, Benha National University, Obour, 11846, Qalyubia, Egypt
* Corresponding Author: Sarah M. Kamel. Email:
Computer Modeling in Engineering & Sciences 2025, 143(1), 373-411. https://doi.org/10.32604/cmes.2025.062837
Received 29 December 2024; Accepted 04 March 2025; Issue published 11 April 2025
Abstract
Visual question answering (VQA) is a multimodal task, involving a deep understanding of the image scene and the question’s meaning and capturing the relevant correlations between both modalities to infer the appropriate answer. In this paper, we propose a VQA system intended to answer yes/no questions about real-world images, in Arabic. To support a robust VQA system, we work in two directions: (1) Using deep neural networks to semantically represent the given image and question in a fine-grained manner, namely ResNet-152 and Gated Recurrent Units (GRU). (2) Studying the role of the utilized multimodal bilinear pooling fusion technique in the trade-off between the model complexity and the overall model performance. Some fusion techniques could significantly increase the model complexity, which seriously limits their applicability for VQA models. So far, there is no evidence of how efficient these multimodal bilinear pooling fusion techniques are for VQA systems dedicated to yes/no questions. Hence, a comparative analysis is conducted between eight bilinear pooling fusion techniques, in terms of their ability to reduce the model complexity and improve the model performance in this case of VQA systems. Experiments indicate that these multimodal bilinear pooling fusion techniques have improved the VQA model’s performance, until reaching the best performance of 89.25%. Further, experiments have proven that the number of answers in the developed VQA system is a critical factor that affects the effectiveness of these multimodal bilinear pooling techniques in achieving their main objective of reducing the model complexity. The Multimodal Local Perception Bilinear Pooling (MLPB) technique has shown the best balance between the model complexity and its performance, for VQA systems designed to answer yes/no questions.Keywords
Cite This Article
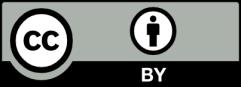
This work is licensed under a Creative Commons Attribution 4.0 International License , which permits unrestricted use, distribution, and reproduction in any medium, provided the original work is properly cited.