Open Access
ARTICLE
A Privacy-Preserving Graph Neural Network Framework with Attention Mechanism for Computational Offloading in the Internet of Vehicles
Department of Computer Science and Engineering, College of Engineering Guindy, Anna University, Chennai, 600025, India
* Corresponding Author: Aishwarya Rajasekar. Email:
Computer Modeling in Engineering & Sciences 2025, 143(1), 225-254. https://doi.org/10.32604/cmes.2025.062642
Received 23 December 2024; Accepted 27 February 2025; Issue published 11 April 2025
Abstract
The integration of technologies like artificial intelligence, 6G, and vehicular ad-hoc networks holds great potential to meet the communication demands of the Internet of Vehicles and drive the advancement of vehicle applications. However, these advancements also generate a surge in data processing requirements, necessitating the offloading of vehicular tasks to edge servers due to the limited computational capacity of vehicles. Despite recent advancements, the robustness and scalability of the existing approaches with respect to the number of vehicles and edge servers and their resources, as well as privacy, remain a concern. In this paper, a lightweight offloading strategy that leverages ubiquitous connectivity through the Space Air Ground Integrated Vehicular Network architecture while ensuring privacy preservation is proposed. The Internet of Vehicles (IoV) environment is first modeled as a graph, with vehicles and base stations as nodes, and their communication links as edges. Secondly, vehicular applications are offloaded to suitable servers based on latency using an attention-based heterogeneous graph neural network (HetGNN) algorithm. Subsequently, a differential privacy stochastic gradient descent training mechanism is employed for privacy-preserving of vehicles and offloading inference. Finally, the simulation results demonstrated that the proposed HetGNN method shows good performance with 0.321 s of inference time, which is 42.68%, 63.93%, 30.22%, and 76.04% less than baseline methods such as Deep Deterministic Policy Gradient, Deep Q Learning, Deep Neural Network, and Genetic Algorithm, respectively.Keywords
Cite This Article
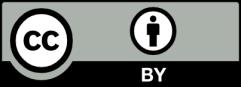
This work is licensed under a Creative Commons Attribution 4.0 International License , which permits unrestricted use, distribution, and reproduction in any medium, provided the original work is properly cited.