Open Access
ARTICLE
Improving Shallow Foundation Settlement Prediction through Intelligent Optimization Techniques
1 Faculty of Earth Sciences Engineering, Arak University of Technology, Arak, 38135-1177, Iran
2 School of Civil and Environmental Engineering, University of Technology Sydney, Sydney, NSW 2007, Australia
* Corresponding Author: Danial Jahed Armaghani. Email:
(This article belongs to the Special Issue: Computational Intelligent Systems for Solving Complex Engineering Problems: Principles and Applications-II)
Computer Modeling in Engineering & Sciences 2025, 143(1), 747-766. https://doi.org/10.32604/cmes.2025.062390
Received 17 December 2024; Accepted 18 March 2025; Issue published 11 April 2025
Abstract
In contemporary geotechnical projects, various approaches are employed for forecasting the settlement of shallow foundations (Sm). However, achieving precise modeling of foundation behavior using certain techniques (such as analytical, numerical, and regression) is challenging and sometimes unattainable. This is primarily due to the inherent nonlinearity of the model, the intricate nature of geotechnical materials, the complex interaction between soil and foundation, and the inherent uncertainty in soil parameters. Therefore, these methods often introduce assumptions and simplifications, resulting in relationships that deviate from the actual problem’s reality. In addition, many of these methods demand significant investments of time and resources but neglect to account for the uncertainty inherent in soil/rock parameters. This study explores the application of innovative intelligent techniques to predict Sm to address these shortcomings. Specifically, two optimization algorithms, namely teaching-learning-based optimization (TLBO) and harmony search (HS), are harnessed for this purpose. The modeling process involves utilizing input parameters, such as the width of the footing (B), the pressure exerted on the footing (q), the count of SPT (Standard Penetration Test) blows (N), the ratio of footing embedment (Df/B), and the footing’s geometry (L/B), during the training phase with a dataset comprising 151 data points. Then, the models’ accuracy is assessed during the testing phase using statistical metrics, including the coefficient of determination (R2), mean square error (MSE), and root mean square error (RMSE), based on a dataset of 38 data points. The findings of this investigation underscore the substantial efficacy of intelligent optimization algorithms as valuable tools for geotechnical engineers when estimating Sm. In addition, a sensitivity analysis of the input parameters in Sm estimation is conducted using @RISK software, revealing that among the various input parameters, the N exerts the most pronounced influence on Sm.Keywords
Cite This Article
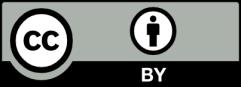
This work is licensed under a Creative Commons Attribution 4.0 International License , which permits unrestricted use, distribution, and reproduction in any medium, provided the original work is properly cited.