Open Access
ARTICLE
A Nature-Inspired AI Framework for Accurate Glaucoma Diagnosis
1 School of Software, Northwestern Polytechnical University, Xi’an, 710072, China
2 Shifa College of Medicine, Shifa Tameer-e-millat University, Islamabad, 44000, Pakistan
3 College of Information Science and Technology, Hainan Normal University, Haikou, 571158, China
4 Department of Computer Science and Engineering, College of Applied Studies and Community Service, King Saud University, Riyadh, 11495, Saudi Arabia
* Corresponding Author: Anas Bilal. Email:
(This article belongs to the Special Issue: Advances in AI-Driven Computational Modeling for Image Processing)
Computer Modeling in Engineering & Sciences 2025, 143(1), 539-567. https://doi.org/10.32604/cmes.2025.062301
Received 15 December 2024; Accepted 19 February 2025; Issue published 11 April 2025
Abstract
Glaucoma, a leading cause of blindness, demands early detection for effective management. While AI-based diagnostic systems are gaining traction, their performance is often limited by challenges such as varying image backgrounds, pixel intensity inconsistencies, and object size variations. To address these limitations, we introduce an innovative, nature-inspired machine learning framework combining feature excitation-based dense segmentation networks (FEDS-Net) and an enhanced gray wolf optimization-supported support vector machine (IGWO-SVM). This dual-stage approach begins with FEDS-Net, which utilizes a fuzzy integral (FI) technique to accurately segment the optic cup (OC) and optic disk (OD) from retinal images, even in the presence of uncertainty and imprecision. In the second stage, the IGWO-SVM model optimizes the SVM classification process, leveraging a gray wolf-inspired optimization strategy to fine-tune the kernel function for superior accuracy. Extensive testing on three benchmark glaucoma image databases DRIONS-DB, Drishti-GS, and Rim-One-r3 demonstrates the efficacy of our method, achieving classification accuracies of 97.65%, 94.88%, and 93.2%, respectively. These results surpass existing state-of-the-art techniques, offering a promising solution for reliable and early glaucoma detection.Keywords
Cite This Article
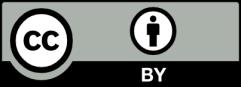
This work is licensed under a Creative Commons Attribution 4.0 International License , which permits unrestricted use, distribution, and reproduction in any medium, provided the original work is properly cited.