Open Access
ARTICLE
Coupling the Power of YOLOv9 with Transformer for Small Object Detection in Remote-Sensing Images
Department of Electrical Engineering, College of Engineering, Northern Border University, Arar, 91431, Saudi Arabia
* Corresponding Author: Mohammad Barr. Email:
(This article belongs to the Special Issue: Advances in AI-Driven Computational Modeling for Image Processing)
Computer Modeling in Engineering & Sciences 2025, 143(1), 593-616. https://doi.org/10.32604/cmes.2025.062264
Received 14 December 2024; Accepted 11 March 2025; Issue published 11 April 2025
Abstract
Recent years have seen a surge in interest in object detection on remote sensing images for applications such as surveillance and management. However, challenges like small object detection, scale variation, and the presence of closely packed objects in these images hinder accurate detection. Additionally, the motion blur effect further complicates the identification of such objects. To address these issues, we propose enhanced YOLOv9 with a transformer head (YOLOv9-TH). The model introduces an additional prediction head for detecting objects of varying sizes and swaps the original prediction heads for transformer heads to leverage self-attention mechanisms. We further improve YOLOv9-TH using several strategies, including data augmentation, multi-scale testing, multi-model integration, and the introduction of an additional classifier. The cross-stage partial (CSP) method and the ghost convolution hierarchical graph (GCHG) are combined to improve detection accuracy by better utilizing feature maps, widening the receptive field, and precisely extracting multi-scale objects. Additionally, we incorporate the E-SimAM attention mechanism to address low-resolution feature loss. Extensive experiments on the VisDrone2021 and DIOR datasets demonstrate the effectiveness of YOLOv9-TH, showing good improvement in mAP compared to the best existing methods. The YOLOv9-TH-e achieved 54.2% of mAP50 on the VisDrone2021 dataset and 92.3% of mAP on the DIOR dataset. The results confirm the model’s robustness and suitability for real-world applications, particularly for small object detection in remote sensing images.Keywords
Object detection methodologies and satellite techniques are constantly improving, making remote sensing target detection more important in many areas. This is especially true for applications related to marine resource management [1], military monitoring [2], and traffic control [3]. Automatically recognizing and localizing certain objects inside images captured by satellites, planes, or spacecraft is its principal goal when used for remote sensing images. Anything from houses to cars to bodies of water and other obstacles can be targeted.
Nevertheless, detecting standard objects in general datasets like ImageNet [4], Pascal Visual Object Classes (VOC) [5], etc., is fundamentally different from detecting objects inside remote sensing images due to the variable shooting angles and great distances involved. Among the many small objects seen in remote sensing images are clusters of objects with a high density of information and objects that are extremely small (less than 10 pixels). In addition, there is a wide range of situations in which the targets’ sizes and the complexity of the backdrops coexist; some images may even contain several objects or background categories of different sizes all at once [6]. The size of automobiles in remote sensing images, for instance, might vary greatly from that of buildings and airplanes. As a result, improving detection accuracy becomes much more difficult when dealing with low-resolution and dense small objects [7].
Object detection in remote sensing images is crucial for various applications, including urban planning, environmental monitoring, disaster management, and surveillance. In these applications, detecting small objects—such as vehicles, pedestrians, or small infrastructure—plays a critical role in improving the accuracy and reliability of automated systems. However, the detection of small objects in remote-sensing images presents several challenges. The scale of objects can vary dramatically due to the difference in elevation during image capture, which affects the appearance and detectability of objects. Furthermore, small objects are often densely packed, making them difficult to distinguish from each other or from complex backgrounds. A prominent example is the detection of small vehicles or pedestrians in high-resolution satellite imagery, where the challenge is to distinguish these objects from the surrounding environment or from other closely spaced objects. Another significant challenge is the motion blur effect, which can obscure small objects in aerial imagery, particularly in videos taken from drones or aircraft.
Overcoming these challenges is essential for applications such as traffic monitoring, disaster relief (e.g., detecting victims or damage in disaster areas), and precision agriculture (e.g., identifying crop health issues or small wildlife). Despite advances in deep learning techniques for object detection, small object detection in remote sensing remains an open problem due to the limitations of traditional models in handling variations in scale and the presence of noise. This paper presents an approach to improve small object detection in remote sensing images by enhancing the YOLOv9 framework with a transformer head (YOLOv9-TH), specifically designed to address these challenges.
Deep Learning, especially convolutional neural networks, has made great strides in object detection tasks in recent years [8,9]. Important benchmark datasets that support object detection application development including MS COCO [10] and PASCAL VOC [11] have been widely employed. On the other hand, the vast majority of convolutional neural networks have been developed for use with images of natural scenes. However, in remote sensing images, there is a dramatic shift in the object scale due to the variable flying height. The second issue is that occlusion between objects is more common in remote sensing images because of the high density of objects in them. Thirdly, due to the vast areas covered, remote sensing images often include geographical features that are difficult to decipher. Object recognition in remote sensing images is extremely tough due to the three issues discussed above.
An essential part of one-stage detectors in object detection tasks is the You Only Look Once (YOLO) series [12–15]. The YOLO models have been widely used for automotive applications. To address these three issues, we provide YOLOv9-TH, an enhanced model built on YOLOv9 [15]. As in the previous version, YOLOv9-TH is based on the backbone with additional modules. Adding an extra head for small object detection is the first step in the head section. In all, YOLOv9-TH has four distinct detection heads for detecting small, medium, big, and very small objects. Furthermore, in order to investigate the prediction potential, Transformer Heads (TH) [16] are used in place of the original prediction heads. Our enhanced YOLOv9-TH is superior to YOLOv9 when it comes to processing remote-sensing images.
Additionally, we present the ghost convolution hierarchical graph (GCHG) block, which integrates ghost convolutions [17] with the cross-stage partial (CSP) approach [18]. By utilizing redundant feature maps efficiently, expanding the receptive field, and reliably extracting multi-scale objects and sophisticated semantic information from complicated backdrops, this upgrade may be used. We improve YOLOv9-TH’s capacity to extract multi-scale object features and sophisticated semantic information by building its feature extraction backbone using the GCHG block. Further, to overcome the low-resolution feature loss constraint, we improve the accuracy of recognizing low-resolution features against complex backgrounds by creating the Adaptive-SimAM, which makes use of residual approaches by upgrading the SimAM module [19]. Lastly, we rebuild the neck structure and add a tiny detection head to increase the capacity to recognize tightly packed small objects. Designed to better focus on tightly packed small objects, this extra tiny detection head fully leverages the fine-grained information in shallow feature maps. Incorporating these improvements naturally allows our proposed YOLO-GE to improve the extraction and integration method for feature information across scales even further.
In order to make YOLOv9-TH even more effective, we use a set of methods. In particular, we support adaptation for large changes in object size in images by using data augmentation during training. The use of multi-model ensemble techniques and multi-scale testing during inference helps to get a more convincing detection performance. Furthermore, by examining the failure scenarios, we discover that our suggested design possesses superior localization capabilities but subpar categorization abilities, particularly when it comes to some related categories such as “tricycle” and “awning-tricycle”. Using the self-trained classifier, ResNet18 [20], which uses the cropped image patches as a training set for classification, fixed this issue. The proposed approach achieves an Average Processing (AP) value improvement of 0.8% to 1.0% when using a self-trained classifier.
Below is a summary of the article’s main contributions:
• The integration of a transformer head (TH) in YOLOv9 to improve multi-scale object detection performance, particularly for small and densely packed targets.
• The development of novel strategies, including the ghost convolution hierarchical graph (GCHG) and the E-SimAM attention mechanism, to enhance feature extraction and improve robustness against low-resolution feature loss.
• The introduction of a cross-stage partial (CSP) mechanism to leverage redundant feature maps and widen the receptive field for improved detection in complex backgrounds.
Comprehensive evaluation of the VisDrone2021 dataset, demonstrating state-of-the-art performance with significant gains in mAP and robust interpretability for real-world applications. Here is how the remaining portion of this research is structured. Section 2 discusses related works. The proposed YOLOv9-TH algorithm is described in Section 3. We conduct extensive tests to evaluate the efficacy of our suggested upgrades and the performance of YOLOv9-TH in Section 4. The study’s conclusion is presented in Section 5.
The field of computer vision relies heavily on convolutional neural networks (CNN) for tasks such as object recognition and object positioning in images and video. This method has become very important in many domains, such as autonomous cars, robots, and surveillance systems. A two-stage and a one-stage CNN-based object detection approach are the two main types. The two-stage approach breaks down object detection into two separate steps: First, a region proposal network proposes a set of boxes that might contain the target objects; second, these boxes are classified and localized to identify and pinpoint the detected targets. A few examples of two-stage approaches are R-CNN [21], Fast R-CNN [22], Faster R-CNN [23], and Cascade R-CNN [24]. These approaches have great detection accuracy but aren’t great for real-time situations because they require candidate regions to be generated before object detection can be done, which is time-consuming.
The one-stage method, on the other hand, may sacrifice accuracy for faster detection rates by immediately regressing category probabilities and bounding box coordinates instead of creating candidate regions. Many algorithms, such as the YOLO series [25], SSD [26], RetinaNet [27], etc., are part of the one-stage techniques. The YOLO, first proposed by Redmon et al. [28] in 2016, uses a single network to directly forecast location frames and category probabilities over the whole image. Coarse object localization and inadequate detection performance for small objects are the results of using the same fully connected layer for classification and regression. The advent of algorithms such as YOLOv2 [29] to YOLOv9 [15] is a result of the substantial research efforts of many scholars who set out to solve these problems. After a huge effort, the algorithms in the YOLO series now strike an impressive balance between speed and accuracy.
When it comes to the backbone and neck, YOLOv8 uses the C2f structure with improved gradient flow instead of the C3 structures, which is an improvement over YOLOv5. Furthermore, it differentiates between the detection and classification heads by replacing the coupled head with the popular decoupled head architecture. Moreover, YOLOv8 shifts from an anchor-based to an anchor-free methodology, using an assigned method to match ground truth and forecast boxes. Five different versions of YOLOv8 are defined by the number of parameters and network channels. Situations calling for more precise detection are better suited to YOLOv8 because of its superior accuracy and intricate model structure. When applied to remote sensing imagery, the YOLO object detection methods still face challenges, such as detecting objects at multiple scales, dealing with complicated backgrounds in various scenes, and meeting the needs of real-time surveillance applications, even though they perform very well on generic datasets. More recent efforts have concentrated on improving the accuracy of small object identification using the YOLO series of algorithms to overcome these restrictions. YOLOv9 was created to solve such problems.
To enhance detection performance, Zakria et al. [30] presented the YOLOv4-based classification setting of the non-maximum suppression threshold and K-means anchor frame scheme. The problem is that these hyperparameters are static and won’t work with datasets that vary. These hyperparameters need to be empirically defined when dealing with datasets that contain tightly packed small objects and large-scale fluctuations. In their presentation of the YOLO-extract method, which was based on YOLOv5, Liu et al. [31] used residual ideas to enhance the feature extraction capability. The model also included the coordinate attention mechanism and mixed dilated convolution. To replace CIoU loss and speed up the model’s convergence, Focal-α EIoU was implemented. Nevertheless, the method has a dedicated micro item detection head to enhance the identification of small and densely packed objects. However, it diminishes detection performance for medium and large-scale objects by eliminating two detection heads for medium and big objects, which hinders the ability to capture high-level semantic information.
Drawing inspiration from YOLOv5, Lin et al. [32] created the YOLO-DA method, which uses an attention module and a simplified decoupled detecting head with a convolutional block attention module (CBAM) module [17] to achieve a speed-accuracy trade-off. A lightweight detection technique called CSPPartial-YOLO for Remote Sensing Images was proposed by Xie et al. [33] using the Partial Hybrid Dilated Convolution blocks and the CSP strategy. In order to detect objects against complicated backdrops, this method makes efficient use of redundant feature maps, decreases the number of parameters in the model, and expands the receptive field. The detection efficiency for remote sensing objects has been enhanced with YOLO-DA and CSPPartialYOLO, however, the detection accuracy has not been much improved. The YOLO-SSP model, developed by Liu et al. [34], is an upgraded version of the YOLOv8 model. By using hierarchical pooling procedures to extract weights from various locations and enhancing the downsampling layers to capture finer information, this model boosts its detection accuracy. Although numerous efforts have been made to improve the accuracy of multi-scale object detection, a significant barrier still exists in accurately detecting small targets that are densely packed inside remote sensing images.
Furthermore, many work explores the combination of depth and image data for multimodal object detection using transformer models [35]. The integration of these two data sources provides richer, more detailed information that enhances object detection in environments where depth data is critical. This approach can be deployed for remote sensing, particularly in scenarios where combining visual data with other types of sensors (such as LiDAR, Light Detection and Ranging) can significantly improve object detection performance, especially in cluttered environments. Besides, a study focusing on the use of masked autoencoders for pre-training LiDAR perception [36] was proposed, which enables efficient 3D sensing with less data. The concept of pre-training and leveraging self-supervised learning has become increasingly important in remote sensing, especially in reducing the dependency on large annotated datasets. This allows for improving object detection in remote sensing, where 3D data, such as LiDAR, plays a crucial role in detecting small or occluded objects in complex scenes.
To build a robust detector in remote sensing images, we proposed YOLOv9-TH. The model was designed based on the YOLOv9 with additional modules to enhance its capability to detect very small objects. In this section, we will provide a detailed description of the proposed model. Section 3.1 will provide an overview of the background of the YOLOv9 model. Section 3.2 will be reserved for presenting YOLOv9-TH with its additional modules and the used detection head based on transformer.
YOLOv9 introduces innovative methods, including the Generalized Efficient Layer Aggregation Network (GELAN) and Programmable Gradient Information (PGI), which improve real-time object detection significantly. Using the MS COCO dataset as a baseline, this model shows new levels of efficiency, accuracy, and adaptability. A distinct open-source team developed YOLOv9, but it shows how the AI research community works together by extending the strong codebase of Ultralytics YOLOv5.
While YOLOv9 has made great strides toward a solution, data loss remains a big issue for deep neural networks. Built on top of the Information Bottleneck Principle and uniquely utilizing Reversible Functions, YOLOv9 ensures that it will always be accurate and efficient. According to the information bottleneck concept, data X could cause information loss during transformation, as Eq. (1).
I(X,X)≥I(X,fθ(X))≥I(X,gφ(fθ(X)))I(X,X)≥I(X,fθ(X))≥I(X,gφ(fθ(X)))(1)
where θ and ϕ are parameters of f and g transformation functions, respectively, and I represent mutual information.
The functions of fθ(.)fθ(.) and gφgφ(.) are used to represent the two subsequent layers of a deep neural network, correspondingly. The risk of erasing the initial data grows in proportion to the number of network levels, as shown in Eq. (1). After fresh gradients are generated by calculating the loss function, the network is updated; however, the parameters of the deep neural network depend on both the network’s output and the given target. The output of a deeper neural network will naturally struggle to retain all of the prediction target’s nuances. Using partial information during network training will result in unreliable gradients and poor convergence.
Increasing the size of the model is one way to address the previous problem. Building a model with a large number of parameters may lead to a more thorough data transformation. No matter what happens to data during the data feedforward process, if you follow the previous procedure, you have a better chance of keeping enough data to complete the mapping to the destination. Because of the issue described earlier, most models now emphasize breadth rather than depth. Very deep neural networks still have a basic problem with employing untrustworthy gradients, and this is something that the prior conclusion failed to tackle. In YOLOv9, you’ll learn the fundamentals of reversible function problem-solving and relative analysis.
The existence of an inverse transformation function v for a function r is what makes it a reversible function, as Eq. (2).
X=vρ(rτ(X))X=vρ(rτ(X))(2)
where the parameters of rr and vv are denoted by ττ and ρρ, respectively. As seen in Eq. (3), data X is transformed using a reversible function while preserving all of its information.
I(X,X)≥I(X,rτ(X))≥I(X,vρ(rτ(X)))I(X,X)≥I(X,rτ(X))≥I(X,vρ(rτ(X)))(3)
Using reversible functions in the network’s transformation function produces more precise gradients for updating the model. Almost every prominent deep learning algorithm follows the reversible feature, demonstrated in Eq. (4).
Xl+1=Xl+fl+1θ(Xl)Xl+1=Xl+fl+1θ(Xl)(4)
The l-th layer of the PreAct ResNet is denoted by l and its transformation function is denoted by f. Repeatedly, PreAct ResNet [37] sends the starting data X to each layer that follows. This design may be able to train a thousand-layer ANN to converge, but it defeats the purpose of such networks. When faced with difficult problems, it is not easy for us to discover simple mapping functions that link facts to objectives. This explains why ResNet outperforms PreAct ResNet at low layer counts [38].
Similar to how the transformer model effectively utilized masked modeling, YOLOv9 also considered this technique. We try to find the inverse transformation v of r using approximation approaches as Eq. (5), such that the modified features can retain enough information with sparse features.
X=vρ(rτ(X)⋅M)X=vρ(rτ(X)⋅M)(5)
M stands for the dynamic binary mask. Another common method for determining the inverse is its inability to process a large amount of raw data, the lightweight model will exhibit shortcomings when subjected to the earlier method. The reason is that the critical piece of information I(Y, X) that links data X to the desired Y is also impacted by the challenging problem. This problem will be investigated using function is the use of a diffusion model; another popular method is variational autoencoders. Due to the concept of an information bottleneck [39]. The mathematical expression for a data bottleneck is Eq. (6).
I(X,X)≥I(X,Y)≥I(X,fθ(X))≥…≥I(Y,ˆY)I(X,X)≥I(X,Y)≥I(X,fθ(X))≥…≥I(Y,ˆY)(6)
In order to resolve the previously mentioned concerns, YOLOv9 presents a new auxiliary supervision structure called Programmable Gradient Information (PGI). This method is depicted in Fig. 1d. Primary PGI components include the main branch, the auxiliary reversible branch, and the multi-level auxiliary information. Since PGI’s inference approach solely employs the main branch, as demonstrated in Fig. 1d, further inference costs are unnecessary.
Figure 1: Programmable gradient information (PGI)
The other two components are employed in deep learning methods to either expedite or resolve several critical issues. The additional reversible branch deals with problems that deep neural networks cause. The loss function will no longer be able to produce reliable gradients as the network depth increases due to an information bottleneck. The error accumulation is a problem with deep supervision; the architecture and lightweight model of the multiple prediction branch are designed to tackle this problem using multi-level auxiliary information.
An enhanced variant of the previously suggested Efficient Layer Aggregation Network (ELAN) [40], GELAN is the second primary component of YOLOv9. Developing ELAN was mainly driven by a desire to tackle the problem of deep model convergence deteriorating with time due to model scale.
Investigating the shortest and longest gradient channels through each network layer allows for the construction of layer aggregation topologies that have efficient gradient propagation paths. ELAN, which is primarily composed of CSPNet [18] and VoVNet [41], maximizes the network’s gradient length by utilizing the stack structure in computational blocks. A more in-depth description of the computational block’s stack follows. In comparison to other comparable methods, the GELAN procedure is shown in Fig. 2.
Figure 2: Comparison of the E-ELAN against other techniques
Due to our research into the VisDrone2021 dataset, an additional prediction head for the detection of very small objects was added, as the dataset contains numerous such cases. When our four-headed structure is utilized in conjunction with the other three prediction heads, it can mitigate the harmful impact of scale variance in violent objects. The first prediction head, shown in Fig. 3, is trained using a low-level, high-resolution feature map, making it more attuned to fine details. The performance of detecting small objects improves significantly after adding an extra detection head, despite the fact that the compute and memory cost increases. An overview of the main pipeline of the YOLOv9-TH is presented in Fig. 3.
Figure 3: Overview of the proposed YOLOv9-TH
Drawing inspiration from the vision transformer [16], we substituted some of the convolutional and CSP bottleneck sections seen in the original YOLOv9 version with encoder blocks from the transformer. The structure can be seen in Fig. 4. The transformer encoder block can capture more widespread and rich contextual information than the original bottleneck block in the backbone. There are two sub-layers in every transformer encoder. Two sub-layers comprise the model: a fully connected layer (MLP) and a multi-head attention layer (MIL). Every sub-layer takes advantage of the residual connections. The capacity to capture various types of local information is enhanced by transformer encoder blocks. Using a self-attention mechanism, it can also investigate the possibility of feature representation [42]. Transformer encoder blocks outperform other methods on the VisDrone2021 dataset when dealing with densely obstructed objects.
Figure 4: Design of the proposed YOLOv9-TH
The Transformer Prediction Head (TH) and the end of the backbone are formed by applying transformer encoder blocks to the head section in accordance with YOLOv9. The reason is that the feature maps generated by the network’s final stages are not very detailed. By utilizing TH on feature maps with low resolution, the computational and memory expenses can be reduced. The availability of the training process is enhanced when we optionally remove some TH blocks at early layers in order to accommodate higher-resolution input images.
Fig. 4 shows the entire YOLOv9-TH algorithm’s design. Among the main components of the backbone are the recently suggested G-HG block and GELAN. An innovative GhostConv-based module, the GCHG block, was designed to replace the convolution layer for feature extraction. To fuse features, the neck makes use of upsampling and the GELAN.
To overcome the limitations of the SimAM method, an improved attention mechanism, Adaptive-SimAM, was developed based on the SimAM. Also, a detecting head and a features fusion structure with a 4× down-sampling rate were added to help rebuild the head and neck structures.
We have termed our YOLOv9-TH algorithm YOLOv9-TH-s, YOLOv9-TH-m, YOLOv9-TH-c, and YOLOv9-TH-e according to the depth and width scales, just like the YOLOv9 algorithm. Because of this, it is possible to choose a suitable model based on the application case. The YOLOv9-TH compound scales are presented in Table 1.
Improving feature fusion in later phases is the primary goal of the backbone network, which mostly comprises extracting features across scales and advanced semantic information for different objectives. Nevertheless, the backbone’s parameters and computational cost both rise with the continual stacking of 3 × 3 convolutions. In an effort to overcome this obstacle and set up a strong foundation for feature extraction, we try to substitute lightweight convolution with 3 × 3 convolution. One small module that can capture more information at little computational expense is ghost convolution [17]. As shown in Fig. 5, the ghost convolution is carried out in a two-stage procedure. The first step is to create an intrinsic feature map using a conventional convolution module and a reduced channel count. Afterward, the acquired intrinsic feature maps are used to produce new ghost feature maps using inexpensive techniques. The last step in creating the best feature maps is to combine the two sets of maps. Ghost convolution takes advantage of feature maps’ correlations and redundancies more effectively than other lightweight convolutions. So, instead of using regular convolution to extract features, we try using ghost convolution.
Figure 5: The proposed GCHG block in comparison with the HG block
On the other hand, swapping out the backbone’s convolutional modules for ghost convolutions results in worse feature extraction and somewhat worse detection accuracy. The feature maps produced by the inexpensive processes of ghost convolutions are insufficiently comprehensive. If more redundant feature maps and a larger receptive field for effective feature extraction are needed, more ghost convolutions must be stacked. Fig. 5 shows how we use the HG block [43] from its backbone to replace convolution operations with ghost convolutions, drawing inspiration from the recent success of RT-DETR [44]. Furthermore, as shown in Fig. 5, we suggest an improved version of the GCHG block to improve the extraction of advanced semantic characteristics.
In order to combine features from several convolution layers, a hierarchical stacking method was adopted for the HG block. Following the previous tactic of stacking ghost convolutions to increase the receiving field for enhanced semantic feature extraction, this improves the model’s ability to interpret both coarse-grained and fine-grained data. Two 1 × 1 convolutional modules are used to perform hierarchical feature fusion once all the feature maps produced by ghost convolutions have been combined. This considerably enhances non-linearity while maintaining the dimensions of the feature maps. It becomes difficult to obtain advanced semantic information as the network depth increases because of the risk of losing gradient information, even though the HG block is good at extracting feature map information by stacking convolutional layers to enhance the receptive field. We reorganize the HG block and add the GCHG block using the CSP [17] technique and the channel shuffle [45] method to make it even more effective. Starting with the initial 1 × 1 convolution, channel shuffling is applied to the feature map. After channel shuffling, the feature map that comes out of it is joined with the original one. Lastly, the final feature map is created by refining the obtained map using the second 1 × 1 convolution. Channel shuffling is a technique that further integrates the features within the channels. In order to help the network learn and extract features, the CSP technique injects gradient flow information.
Due to the intricacy of ever-changing backgrounds and the dense distribution of small targets, reliably identifying multi-scale targets becomes a substantial difficulty in practical remote sensing applications. The attention mechanism has attracted a lot of interest from academics looking for a better way to extract the characteristics of these targets. Several attention processes that have been suggested in earlier studies have the potential to overcome the drawbacks of convolutional models [46]. As a result, many different attention processes have been developed in an effort to improve detection accuracy. The convolutional block attention module (CBAM), first proposed by Woo et al. [20], learns to extract features from both the channel and spatial dimensions by progressively inferring a 1D channel and 2D spatial attention map. Using rotation operations and residual transformations, Misra et al. [47] built the triplet attention module, which extracts inter-dependencies. The ACmix attention module was introduced by Pan et al. [46]. It efficiently extracts semantic features by combining self-attention and convolution. The effectiveness of these attention mechanisms has not been without the constant development in network complexity and parameter counts. Fig. 6a shows the SimAM attention module that was suggested by Yang et al. [19]. This module determines the three-dimensional attention weights for the input feature map by using the optimized energy function to determine the importance of individual neurons. Particularly noteworthy is the fact that the mechanism has a straightforward design devoid of superfluous factors. We incorporate the SimAM module and suggest an improved version called the Adaptive-SimAM attention module to optimize the model for efficiency and improve detection precision while collecting small objects, as shown in Fig. 6b.
Figure 6: The proposed (a) adaptive-SimAM vs. (b) the SimAM
In order to create the attention weights in three dimensions, the optimal energy function was used to determine the importance of each neuron in the input feature maps. If the input feature maps are for X∈RH×W×C, then the variables t, i, and xi represent the target neuron, the index in the spatial dimension, and other neurons in the feature maps’ channel. X.M=H×W denotes the total quantity of neurons within that channel. The energy function can be optimized as Eq. (7).
et=4(σ2+λ)(t−μ)2+2σ2+2λμ=1M∑Mi=1xiσ2=1M∑Mi=1(xi−μ)2(7)
where σ2 and μ denote the mean and variance of all neurons, and et is the lower energy of neuron t. Hence, 1/et May be used to assess the relevance of individual neurons, and the SimAM can be computed as Eq. (8).
X′=sigmoid(1E)⨀X(8)
The output feature maps of SimAM are denoted as X′, and E is used to aggregate all et across channel and spatial dimensions. Nevertheless, as a non-linear function, the Sigmoid function guarantees that its output will consistently fall inside the range of 0 and 1. In small targets, neurons with lesser features or poorer resolution may be lost, but neurons with strong ones can be preserved. To overcome this shortcoming, we improved the attention mechanism by introducing the residual connection [42]. The first step is to process the acquired feature maps X′, further using a 1 × 1 convolutional module to guarantee the use of effective features in feature extraction. Afterward, the freshly acquired feature maps are integrated with the initial feature maps X. This method helps with the detection and localization of small targets by preserving features with lower resolution or weaker features while reinforcing dominant features. The proposed Adaptive-SimAM module can be computed as Eq. (9).
Y=X+conv1×1(X′)(9)
For getting the most out of Adaptive-SimAM’s attention mechanism, it was integrated after the backbone network’s feature map concatenation operation and the neck’s up-sampling feature map. This allows to quickly prepare for multi-scale fusion by faster extracting crucial features from the backbone and neck feature map fusion.
This section is reserved for the evaluation of the proposed model and discussing the achieved results with a presentation of an ablation study to investigate the impact of the proposed contributions. Section 4.1 will present the implementation details. In Section 4.2, we will detail the used dataset. Section 4.3 provides an overview of the evaluation metrics. The achieved results will be presented in Section 4.4 alongside with a comparison study. In Section 4.5, a deep discussion on the results will be performed. We will finish with an ablation study in Section 4.6.
We use Pytorch 1.8.1 to implement YOLOv9-TH. Train and test of the model were performed on an NVIDIA GTX960 GPU. YOLOv9-TH and YOLOv9 share most of the backbone and some of the head, many weights from YOLOv9 were transferred to YOLOv9-TH during the training phase and save a lot of training time by using these weights. Due to the relatively modest size of the VisDrone2021 training set, the model is trained for 65 epochs using the VisDrone2021 trainset, with the first 2 epochs serving as warm-up. We train with the Adam optimizer using the cosine LR schedule and an initial learning rate of 3 × 10−4. For the last epoch, the learning rate has dropped to 0.12 of its original value. Our model uses an extremely big input image—its length is 1536 pixels—which results in a batch size of only 2.
Based on our prior technical knowledge, it is crucial to review the training dataset. There are a lot of tiny, unrecognizably-there items in some of the images since the camera was set too high. Bounding boxes within the VisDrone2021 dataset have been examined. With a 1536 × 1536 input image size, 622 out of 342,391 labels are smaller than 3 pixels. Fig. 7 shows the distribution of the instances per class. It is common practice to employ data augmentation as a means to enhance performance and decrease generalization mistakes while training neural network models to address computer vision problems. To enable a model to generate predictions on several image versions, it is possible to apply image data augmentation to the test dataset. To improve prediction performance, it is possible to average the enhanced images’ predictions. In multi-scale testing, we first flip the test images horizontally, and then scale them to three different sizes, yielding six unique images in total. The final test result is obtained by testing six distinct images and then merging the data.
Figure 7: Instance distribution per class for the VisDrone2021 dataset
The AISKYEYE group at China’s Tianjin University’s Lab of Machine Learning and Data Mining collected the VisDrone2021 dataset. The benchmark dataset is comprised of 263 video clips with 179,264 frames, and 10,209 static images. These were shot using a variety of drone-mounted cameras and covered a broad range of topics, such as location (including 14 cities in China that are thousands of kilometers apart), environment (including both urban and rural settings), objects (including pedestrians, vehicles, bicycles, and more), and density (including both sparse and crowded scenes). The dataset was gathered in a variety of situations, with varying types of weather and illumination, and using a wide range of drone platforms. Over 2.5 million bounding boxes or points of targets of common interest, including people, vehicles, bicycles, and tricycles, have been painstakingly tagged onto these frames. Scene visibility, object class, and occlusion are some of the crucial features that are offered for improved data use.
For the purpose of further evaluating our YOLOv9-TH model, we performed experiments using the widely used Dataset for Object Detection in Aerial Images (DIOR). Created by Northwestern Polytechnical University, the DIOR dataset is a massive benchmark that has 23,463 images and 190,288 instances and was designed for object detection in remote sensing videos. Images in the dataset range in size from 800 × 800 pixels and represent spatial resolutions between half a meter and thirty meters. Included in its twenty object classes are a wide variety of things, such as buildings, vehicles, airplanes, and more. Benefits of the DIOR dataset include large-scale data, diverse instance object sizes, rich image diversity, high inter-class similarity, and significant intra-class differences, in comparison to other datasets.
The mean average precision (mAP) is used as the assessment metric to measure the performance of our technique. The recall rate (R) and the precision (P), where P is the proportion of properly predicted samples to total samples and R is the proportion of correctly predicted samples to all real positive samples. R and P can be computed as Eqs. (10) and (11), respectively.
P=TPTP+FP(10)
R=TPTP+FN(11)
FP stands for false positive, whereas TP stands for true positive. To the contrary, FN stands for false negatives. The definitions of average precision (AP) and mean average precision (mAP) are presented as Eqs. (12) and (13), respectively.
AP=∫10P(R)dR(12)
mAP=1NN∑i=1APi(13)
N refers to the number of categories in the dataset.
4.4 Results and Comparison Study
The achieved results demonstrate that YOLOv9-TH achieves a significant improvement in the detection of small objects, such as pedestrians and bicycles, compared to existing methods. The metrics also show consistent performance in larger-scale objects, underscoring the versatility of the proposed approach. This validates the efficacy of our model in handling multi-scale object detection challenges in remote sensing images. Table 2 presents the per-class precision achieved by the proposed model. The YOLOv9-TH series, particularly the YOLOv9-TH-e model, demonstrates significant advantages in detecting small objects compared to earlier YOLO versions such as YOLOv5, YOLOv6, YOLOv8, and even standard YOLOv9-c. Analyzing the detection performance across different models, YOLOv9-TH-e consistently achieves the highest accuracy across all object categories, with particularly outstanding improvements in recognizing small objects like bicycles, awning-tricycles, pedestrians, and people. For instance, YOLOv9-TH-e detects bicycles with an accuracy of 47.33, which is significantly higher than 20.28 in YOLOv5-n, 25.57 in YOLOv6-n, 30.71 in YOLOv8-l, and 40.27 in YOLOv9-c. Similarly, for pedestrians, YOLOv9-TH-e scores 54.37, outperforming 28.06 in YOLOv5-n, 35.28 in YOLOv6-n, 40.81 in YOLOv8-l, and 51.37 in YOLOv9-c. These results indicate that while earlier models struggled with small objects, YOLOv9-TH-e achieves nearly 2.5× better accuracy than YOLOv5 and over 50% better performance than YOLOv8.
These gains are due to several architectural improvements. Firstly, YOLOv9-TH incorporates a Transformer-based Head (TH), which provides better global context awareness and enhances the detection of small, distant, or overlapping objects—something traditional convolutional-based models like YOLOv5 and YOLOv6 struggle with. Secondly, improved multi-scale feature fusion enables YOLOv9-TH models to better extract fine details, making them more effective at capturing small objects compared to YOLOv8’s FPN + PAN architecture. Additionally, YOLOv9-TH-e and YOLOv9-TH-c integrate deeper feature extraction layers, unlike YOLOv6 and YOLOv5, which rely on shallower backbones, resulting in weaker small object detection. Compared to standard YOLOv9-c, the YOLOv9-TH variants, especially TH-e, benefit from superior data augmentation (e.g., mosaic augmentation and adaptive scaling), making them more robust to varying object scales.
Overall, the YOLOv9-TH series consistently surpasses YOLOv5, YOLOv6, YOLOv8, and standard YOLOv9-c, with YOLOv9-TH-e leading in accuracy and robustness. This makes it the optimal choice for object detection in remote-sensing images.
In order to confirm that our YOLOv9-TH is effective and efficient, it was compared to various state-of-the-art approaches. To start, we compared the evaluation metric curves as we went through training. Next, we compared a number of well-known algorithms, including both one-stage approaches, such as SSD [24], and two-stage techniques, such as Faster R-CNN [13], with more recent ones. A final set of comparison experiments was run using the VisDrone2021 dataset to validate YOLOv9-TH robustness. Training on the VisDrone2021 dataset is shown in Fig. 7 by the evaluation curves. Remarkably, mAP0.5 and mAP0.5:0.95 continuously outperform YOLOv9, showing a consistent and rising trend with increasing iterations. In comparison to YOLOv10, our method also shows better continuing learning ability and a faster convergence speed. Thus, when comparing YOLOv8 to our YOLOv9-TH, we find that it has better convergence and detection performance. Using the VisDrone2021 dataset, we compared performance and presented our findings in Table 3. As far as detection accuracy is concerned, YOLOv9-TH outperforms competing methods with comparable parameter sizes. With a mAP50 of 43.5%, YOLOv9-TH-s outperforms lightweight variants like YOLOv5-n and YOLOv6-n by 9.7% and 3.9% per correspondingly. By reducing parameter size by 21.1% and improving detection accuracy by 2.2% in mAP0.5 and 2.3% in mAP50:95, respectively, YOLOv9-TH-e outperforms the YOLOv9-e model. While YOLOv10-s obtains high mAP50 and mAP50:95, YOLOv9-TH-s reduces parameter size by 43.0% and GFLOPs by 26.3%, respectively, in comparison to YOLOv10-s [48].
We performed additional evaluation on more datasets to prove the generalization of the proposed model. In response, we have conducted additional experiments using the DIOR dataset. The results of these experiments are presented in Table 4, demonstrating that YOLOv9-TH consistently outperforms existing methods across datasets, particularly in detecting small and densely packed objects.
The model’s computation complexity has been slightly increased due to the backbone and Adaptive-SimAM attention method. On the other hand, combining the GCHG block with the networks results in using redundant feature maps to increase the receptive field and improve the performance of multi-scale objects and advanced semantic features in complicated backgrounds. To further improve the accuracy of identifying low-resolution features against intricate backdrops, the Adaptive-SimAM attention mechanism makes use of residual approaches to overcome the low-resolution feature loss constraint. All of the improvements enhanced the model’s detection accuracy, as seen in Table. Notably, compared to algorithms that apply just one improvement, our proposed YOLOv9-TH obtains the maximum detection accuracy when all techniques are combined. The results show that the model’s overall performance is greatly improved by our suggested YOLOv9-TH algorithm, which successfully incorporates the advantages of all three enhancement methods. Results comparing YOLOv9-TH to other algorithms tested on the same dataset are shown in Table 2. Our YOLOv9-TH consistently achieves better results than any other detection algorithm, including the most cutting-edge ones in the field of remote sensing target detection, such as YOLOv8 and YOLOv10. Not only that, our method works wonderfully in a variety of contexts; it particularly shines in the more difficult dataset, outperforming YOLOv9 in terms of detection accuracy. This might be because our improved method is so good at enhancing detection accuracy by extracting information from very densely packed small objects. On the VisDrone2021 dataset, some detection results are presented in Fig. 8. The results show that our YOLOv9-TH is able to detect objects of different sizes and types with high accuracy, regardless of the lighting or detecting scenario. To summarize, our proposed enhancements have been proven useful and robust through numerous tests conducted on varied datasets.
Figure 8: Output detection of the YOLOv9-TH under different conditions
Despite the overall improvement in detection accuracy, our model showed slightly reduced performance in very cluttered or highly occluded scenes. We attribute this to the complexity of detecting small objects in environments where multiple objects overlap or where background noise is prevalent. This result highlights the need for further refinement in handling occlusions and noise, which could be addressed through additional data augmentation techniques or the introduction of more advanced attention mechanisms.
In some cases, especially when evaluating on lower-resolution images, we observed a drop in detection accuracy for smaller objects. While our model leverages the E-SimAM attention mechanism to address low-resolution feature loss, the results indicate that further improvements are needed for optimal performance in low-resolution scenarios. This finding suggests that fine-tuning the model’s architecture or using multi-resolution approaches could further enhance performance in such conditions.
Another unexpected result was the occasional increase in inference time, particularly when processing images with a very high number of objects. This is likely due to the added complexity introduced by the transformer heads and multi-scale feature extraction. We believe that optimizing the model’s computational efficiency, possibly through model pruning or hardware acceleration techniques, could mitigate this issue.
Our findings have significant implications for a wide range of remote sensing applications, particularly those requiring accurate detection of small objects in complex environments. The ability to accurately detect small objects such as vehicles, pedestrians, and infrastructure in remote sensing imagery is crucial for surveillance applications. For example, in urban monitoring or traffic management, the proposed YOLOv9-TH model can be used to detect vehicles in crowded city scenes or track pedestrians in large-scale outdoor environments. Our results show that the model significantly improves detection accuracy for small objects, which is essential for enhancing the reliability of real-time surveillance systems.
In disaster management scenarios, such as after an earthquake, flood, or wildfire, detecting small or partially occluded objects (e.g., survivors, vehicles, or debris) is vital for efficient rescue and recovery operations. Our model’s ability to detect small objects in challenging environments, even with cluttered backgrounds, makes it well-suited for use in emergency response systems that rely on drone or satellite imagery. This could facilitate quicker and more accurate assessments of disaster-affected areas, improving the overall response time and effectiveness of relief efforts.
For applications like precision agriculture, detecting small features such as crops or individual animals is crucial for monitoring crop health, pest infestation, or wildlife populations. The ability of our model to detect small objects in high-resolution satellite or drone imagery can help farmers or environmentalists obtain more accurate insights. This, in turn, could lead to better-informed decisions for crop management, resource allocation, and environmental conservation efforts.
While our experiments focused on traditional visual data, our approach can be extended to work with multimodal data, such as LiDAR or infrared sensing. By combining our model with LiDAR data, for instance, it could enable more robust detection of objects in low-visibility conditions or complex environments. This opens the door to broader applications in remote sensing, where multimodal sensing is becoming increasingly important for applications like autonomous vehicles, forestry, and infrastructure inspection.
We conducted three sets of ablation experiments, with YOLOv9-m serving as the baseline, to evaluate the efficacy of our proposed YOLOv9-TH. Three tests were performed in the first set of studies to assess the efficacy of the enhancements achieved by incorporating the GCHG block. When the first experiment was conducted, YOLOv9-m was used as a standard for comparison analysis. Using the GCHG block, which was created by replacing the regular convolutions in the HG block with ghost convolutions, we confirmed its efficacy. Then, model performance with various backbones is compared in Table 5. The baseline model demonstrates respectable performance, with 48.8% mAP0.5 and 29.4% mAP50:95. However, the model’s detection accuracy has been marginally enhanced since GCHG was introduced. While the baseline achieved a precision of 48.8%, GCHG block improved it to 49.3% and achieved much greater improvements over the HG block. This proves that the GCHG block can improve the performance. Specifically, it proves that the backbone can increase its feature extraction capabilities and detect objects at several scales.
Three additional experiments were conducted during the second set of studies to confirm that the Adaptive-SimAM upgrades were effective. Once again, YOLOv9-m was used as the reference baseline for comparison analysis in the initial trial. In the second trial, we tested the model’s responsiveness after adding the SimAM attention mechanism to its neck structure. In order to confirm the model’s effectiveness, we used the improved attention mechanism in the third experiment. The results of comparing the performance of models that incorporate various attention strategies are shown in Table 6. The accuracy went up to 88.9% after using the SimAM attention mechanism, but the recall and mAP went down a notch. This finding lends credence to the results of the earlier investigation, which showed that this attention mechanism has a tendency to reduce detection accuracy by ignoring low-resolution or featureless objects. Although the Adaptive-SimAM method’s recall was significantly improved to 80.7%, its precision was slightly lower at 88.7% when compared to SimAM. Significant improvements of 86.3% and 62.3% in mAP0.5 and mAP0.5:0.95, respectively, were also attained by the model. This proves that E-SimAM is effective at maintaining detection accuracy even when dealing with low-resolution or subtle features. The enhanced detection performance demonstrates the promise of the Adaptive-SimAM in improving models for the task of detecting tiny objects, even though the number of computational parameters was slightly increased.
The original YOLOv9’s number of layers and GFLOPs increases due to the addition of a detection head for small objects. While this increases the amount of calculation, the mAP improvement is very high. YOLOv9-TH performs well when detecting small objects, so the increase in calculation is worthwhile. After using the transformer encoder block, the total number of layers and GFLOPs decreases. Using transformer encoder blocks can increase mAP and reduce the size of the network. It also plays a role in the detection of dense objects and large objects.
Our goal in this last round of ablation studies was to quantify the effect on the performance of three suggested upgrades: the GE-HGNet for the backbone, the E-SimAM attention mechanism, and improvements to the fusion modules in the detecting head and neck structure. The ablation results of the various modifications examined on the VisDrone2021 dataset are detailed in Table 7. Using the GCHG block in the second experiment keeps precision at 88.8%, boosts recall to 81.1%, and improves mAP0.5 and mAP0.5:0.95 to 86.2% and 62.4%, respectively. In Experiment 3, the Adaptive-SimAM attention mechanism is implemented within the neck structure. This results in a small drop in precision but a significant boost in recall to 80.7%. Additionally, mAP0.5 and mAP0.5:0.95 are improved to 86.3% and 62.3%, respectively. With no change to the recall rate, the detection precision increased to 89.4% in experiment 4 because of improved fusion modules of the neck structure and detecting head. Also improved were the mAP0.5 and mAP0.5:0.95, which reached 86.5% and 62.1%, respectively. Each of the three trials we stated above significantly improved the mAP0.5 and mAP0.5:0.95, indicating that our suggested enhancements are effective. Table 7 shows the results of our trials 5–8, which we ran to confirm the impact of incorporating various enhancements. The E-SimAM attention mechanism and the GE-HGNet backbone are combined in Experiment 5. The sixth experiment integrates the GE-HGNet backbone with the head and neck fusion modules. Experiment 7 involves combining the E-SimAM attention mechanism with the head and neck fusion modules. The results of the experiments demonstrate that mAP0.5 and mAP0.5:0.95 are further improved by combining these upgrades in pairs. In particular, when considering improvements to both the mAP0.5 and mAP0.5:0.95 metrics, the results obtained by combining these enhancements in pairs outperform those of any individual improvement. Finally, we evaluate our ultimate YOLO-GE approach’s performance by integrating all changes in the final experiment. The model scored the maximum detection accuracy, showing recall, mAP0.5, and mAP0.5:0.95, at 81.9%, 87.4%, and 63.8%, respectively, despite a minor drop in precision. Table 7 shows the findings, which prove that our suggested enhancements work and show how these enhancements, when combined organically, boost the model’s detection capabilities, which proves that the YOLO-GE algorithm is both reasonable and effective.
The extensive use of remote sensing technology across various sectors highlights the vital strategic importance of remote target detection for aerial remote sensing technology. In order to tackle the challenges associated with multi-scale object detection in remote sensing and to improve detection accuracy, we introduce YOLOv9-TH, which is built upon YOLOv9. We present three proposed enhancements: the GCHG block for the backbone, the Adaptive-SimAM attention mechanism, and improvements to the fusion modules in the neck structure and use transformer head for detection. Through comprehensive experimental validation on the VisDrone2021 datasets, we have thoroughly demonstrated the effectiveness and robustness of our proposed enhancements. The integrated application of these improvements has been shown to enhance significantly the ability to detect small objects in remote sensing images, highlighting its effectiveness and rationale. The YOLOv9-TH demonstrates superior performance over existing mainstream algorithms by attaining the highest detection precision, all while maintaining a lower number of model parameters in comparison to several larger models. Considering that YOLOv9-TH includes multiple models with enhanced detection accuracy, it facilitates the choice of an appropriate model tailored to the specific application scenario, showcasing extensive application potential. The incorporation of the GCHG block in the backbone and Adaptive-SimAM attention mechanism, in addition to an extra detection head for very small objects, has enhanced the model’s detection accuracy; however, this advancement has also led to a rise in the number of model parameters and GFLOPs, consequently elevating the computational complexity. Consequently, deploying it on embedded devices with constrained computational resources presents a considerable challenge. The YOLOv9-TH-e has achieved 54.8 of mAP50, outperforming state-of-the-art models with a wide margin. In future work, we plan to improve the YOLOv9-TH algorithm by utilizing techniques like network pruning and knowledge distillation to achieve parameter compression, all while ensuring the preservation of detection accuracy. Furthermore, we intend to enhance the model’s detection performance by refining methods associated with localization or classification loss functions.
Acknowledgement: Not applicable.
Funding Statement: The author received no specific funding for this study.
Availability of Data and Materials: The datasets generated and/or analyzed during the current study are available in the github repository, https://github.com/VisDrone/VisDrone-Dataset (accessed on 18 November 2024).
Ethics Approval: Not applicable.
Conflicts of Interest: The author declares no conflicts of interest to report regarding the present study.
References
1. Fan X, Hu Z, Zhao Y, Chen J, Wei T, Huang Z. A small-ship object detection method for satellite remote sensing data. IEEE J Sel Top Appl Earth Obs Remote Sens. 2024;17:11886–98. doi:10.1109/JSTARS.2024.3419786. [Google Scholar] [CrossRef]
2. Pushkarenko Y, Zaslavskyi V. Research on the state of areas in Ukraine affected by military actions based on remote sensing data and deep learning architectures. Radioelectron Comput Syst. 2024;2024(2):5–18. doi:10.32620/reks.2024.2.01. [Google Scholar] [CrossRef]
3. Gao H, Wu S, Wang Y, Kim JY, Xu Y. FSOD4RSI: few-shot object detection for remote sensing images via features aggregation and scale attention. IEEE J Sel Top Appl Earth Obs Remote Sens. 2024;17:4784–96. doi:10.1109/JSTARS.2024.3362748. [Google Scholar] [CrossRef]
4. Deng J, Dong W, Socher R, Li LJ, Kai L, Li FF. ImageNet: a large-scale hierarchical image database. In: 2009 IEEE Conference on Computer Vision and Pattern Recognition; 2009 Jun 20–25; Miami, FL, USA. p. 248–55. doi:10.1109/CVPR.2009.5206848. [Google Scholar] [CrossRef]
5. Hoiem D, Divvala SK, Hays J. Pascal VOC 2008 challenge. World Lit Today. 2009;24(1):1–4. [Google Scholar]
6. Zhang Y, Ye M, Zhu G, Liu Y, Guo P, Yan J. FFCA-YOLO for small object detection in remote sensing images. IEEE Trans Geosci Remote Sens. 2024;62:5611215. doi:10.1109/TGRS.2024.3363057. [Google Scholar] [CrossRef]
7. Jing R, Zhang W, Liu Y, Li W, Li Y, Liu C. An effective method for small object detection in low-resolution images. Eng Appl Artif Intell. 2024;127(9):107206. doi:10.1016/j.engappai.2023.107206. [Google Scholar] [CrossRef]
8. Ayachi R, Afif M, Said Y, Atri M, Ben Abdelali A. Integrating recurrent neural networks with convolutional neural networks for enhanced traffic light detection and tracking. Trait Du Signal. 2023;40(6):2577–86. doi:10.18280/ts.400620. [Google Scholar] [CrossRef]
9. Ayachi R, Afif M, Said Y, Ben Abdelali A. Lightweight neural networks for pedestrian detection in intelligent vehicles. In: Handbook of Research on AI Methods and Applications in Computer Engineering. Hershey, PA, USA: IGI Global; 2023. p. 478–96. [Google Scholar]
10. Lin TY, Michael M, Serge B, James H, Pietro P, Deva R, et al. Microsoft COCO: common objects in context. In: Computer Vision-ECCV 2014: 13th European Conference; 2014 Sep 6–12; Zurich, Switzerland. Berlin/Heidelberg, Germany: Springer International Publishing. p. 740–55. [Google Scholar]
11. Everingham M, Van Gool L, Williams CKI, Winn J, Zisserman A. The pascal visual object classes (VOC) challenge. Int J Comput Vis. 2010;88(2):303–38. doi:10.1007/s11263-009-0275-4. [Google Scholar] [CrossRef]
12. Farhadi A, Redmon J. YOLOv3: an incremental improvement. In: Computer Vision and Pattern Recognition; 2018 Jun 18–22; Salt Lake City, UT, USA. Berlin/Heidelberg, Germany: Springer; 2018. p. 1–6. [Google Scholar]
13. Li C, Li L, Jiang H, Weng K, Geng Y, Li L, et al. YOLOv6: a single-stage object detection framework for industrial applications. arXiv:2209.02976. 2022. [Google Scholar]
14. Wang CY, Bochkovskiy A, Liao HM. YOLOv7: trainable bag-of-freebies sets new state-of-the-art for real-time object detectors. In: 2023 IEEE/CVF Conference on Computer Vision and Pattern Recognition (CVPR); 2023 Jun 17–24; Vancouver, BC, Canada. p. 7464–75. doi:10.1109/CVPR52729.2023.00721. [Google Scholar] [CrossRef]
15. Wang CY, Yeh IH, Liao HY. YOLOv9: learning what you want to learn using programmable gradient information. arXiv:2402.13616. 2024. [Google Scholar]
16. Dosovitskiy A. An image is worth 16 × 16 words: transformers for image recognition at scale. arXiv:2010.11929. 2020. [Google Scholar]
17. Han K, Wang Y, Tian Q, Guo J, Xu C, Xu C. GhostNet: more features from cheap operations. In: 2020 IEEE/CVF Conference on Computer Vision and Pattern Recognition (CVPR); 2020 Jun 13–19; Seattle, WA, USA. p. 1577–86. doi:10.1109/cvpr42600.2020.00165. [Google Scholar] [CrossRef]
18. Wang CY, Mark Liao HY, Wu YH, Chen PY, Hsieh JW, Yeh IH. CSPNet: a new backbone that can enhance learning capability of CNN. In: 2020 IEEE/CVF Conference on Computer Vision and Pattern Recognition Workshops (CVPRW); 2020 Jun 14–19; Seattle, WA, USA. p. 1571–80. doi:10.1109/cvprw50498.2020.00203. [Google Scholar] [CrossRef]
19. Yang L, Zhang RY, Li L, Xie X. SimAM: a simple, parameter-free attention module for convolutional neural networks. In: International Conference on Machine Learning; 2021 Jul 18–24; online. p. 11863–74. [Google Scholar]
20. Woo S, Park J, Lee JY, Kweon IS. CBAM: convolutional block attention module. In: Proceedings of the European Conference on Computer Vision (ECCV); 2018 Sep 8–14; Munich, Germany. p. 3–19. [Google Scholar]
21. Girshick R, Donahue J, Darrell T, Malik J. Rich feature hierarchies for accurate object detection and semantic segmentation. In: 2014 IEEE Conference on Computer Vision and Pattern Recognition; 2014 Jun 23–28; Columbus, OH, USA. p. 580–7. doi:10.1109/CVPR.2014.81. [Google Scholar] [CrossRef]
22. Girshick R. Fast R-CNN. arXiv:1504.08083. 2015. [Google Scholar]
23. Ren S, He K, Girshick R, Sun J. Faster R-CNN: towards real-time object detection with region proposal networks. IEEE Trans Pattern Anal Mach Intell. 2017;39(6):1137–49. doi:10.1109/TPAMI.2016.2577031. [Google Scholar] [PubMed] [CrossRef]
24. Cai Z, Vasconcelos N. Cascade R-CNN: delving into high quality object detection. In: 2018 IEEE/CVF Conference on Computer Vision and Pattern Recognition; 2018 Jun 18–23; Salt Lake City, UT, USA. p. 6154–62. doi:10.1109/CVPR.2018.00644. [Google Scholar] [CrossRef]
25. Vijayakumar A, Vairavasundaram S. YOLO-based object detection models: a review and its applications. Multimed Tools Appl. 2024;83(35):83535–74. doi:10.1007/s11042-024-18872-y. [Google Scholar] [CrossRef]
26. Liu W, Anguelov D, Erhan D, Szegedy C, Reed S, Fu CY, et al. SSD: single shot multibox detector. In: Computer Vision–ECCV 2016: 14th European Conference; 2016 Oct 11–14; Amsterdam, The Netherlands. Berlin/Heidelberg, Germany: Springer International Publishing; 2016. p. 21–37. [Google Scholar]
27. Lin TY, Goyal P, Girshick R, He K, Dollár P. Focal loss for dense object detection. In: 2017 IEEE International Conference on Computer Vision (ICCV); 2017 Oct 22–29; Venice, Italy. p. 2999–3007. doi:10.1109/ICCV.2017.324. [Google Scholar] [CrossRef]
28. Redmon J, Divvala S, Girshick R, Farhadi A. You only look once: unified, real-time object detection. In: 2016 IEEE Conference on Computer Vision and Pattern Recognition (CVPR); 2016 Jun 27–30; Las Vegas, NV, USA. p. 779–88. doi:10.1109/CVPR.2016.91. [Google Scholar] [CrossRef]
29. Redmon J, Farhadi A. YOLO9000: better, faster, stronger. In: 2017 IEEE Conference on Computer Vision and Pattern Recognition (CVPR); 2017 Jul 21–26; Honolulu, HI, USA. p. 6517–25. doi:10.1109/CVPR.2017.690. [Google Scholar] [CrossRef]
30. Zakria Z, Deng J, Kumar R, Khokhar MS, Cai J, Kumar J. Multiscale and direction target detecting in remote sensing images via modified YOLO-v4. IEEE J Sel Top Appl Earth Obs Remote Sens. 2022;15(8):1039–48. doi:10.1109/JSTARS.2022.3140776. [Google Scholar] [CrossRef]
31. Liu Z, Gao Y, Du Q, Chen M, Lv W. YOLO-extract: improved YOLOv5 for aircraft object detection in remote sensing images. IEEE Access. 2023;11:1742–51. doi:10.1109/ACCESS.2023.3233964. [Google Scholar] [CrossRef]
32. Lin J, Zhao Y, Wang S, Tang Y. YOLO-DA: an efficient YOLO-based detector for remote sensing object detection. IEEE Geosci Remote Sens Lett. 2023;20:6008705. doi:10.1109/LGRS.2023.3303896. [Google Scholar] [CrossRef]
33. Xie S, Zhou M, Wang C, Huang S. CSPPartial-YOLO: a lightweight YOLO-based method for typical objects detection in remote sensing images. IEEE J Sel Top Appl Earth Obs Remote Sens. 2023;17(1):388–99. doi:10.1109/JSTARS.2023.3329235. [Google Scholar] [CrossRef]
34. Liu Y, Yang D, Song T, Ye Y, Zhang X. YOLO-SSP: an object detection model based on pyramid spatial attention and improved downsampling strategy for remote sensing images. Vis Comput. 2025;41(3):1467–84. doi:10.1007/s00371-024-03434-y. [Google Scholar] [CrossRef]
35. Mahjourian N, Nguyen V. Multimodal object detection using depth and image data for manufacturing parts. arXiv:2411.09062. 2024. [Google Scholar]
36. Tayebati S, Tulabandhula T, Trivedi AR. Sense less, generate more: pre-training LiDAR perception with masked autoencoders for ultra-efficient 3D sensing. arXiv:2406.07833. 2024. [Google Scholar]
37. He K, Zhang X, Ren S, Sun J. Identity mappings in deep residual networks. In: Computer Vision–ECCV 2016: 14th European Conference; 2016 Oct 11–14; Amsterdam, The Netherlands. Berlin/Heidelberg, Germany: Springer International Publishing. p. 630–45. [Google Scholar]
38. He K, Zhang X, Ren S, Sun J. Deep residual learning for image recognition. In: 2016 IEEE Conference on Computer Vision and Pattern Recognition (CVPR); 2016 Jun 27–30; Las Vegas, NV, USA. p. 770–8. doi:10.1109/CVPR.2016.90. [Google Scholar] [CrossRef]
39. Tishby N, Zaslavsky N. Deep learning and the information bottleneck principle. In: 2015 IEEE Information Theory Workshop (ITW); 2015 Apr 26–May 1; Jerusalem, Israel. p. 1–5. doi:10.1109/ITW.2015.7133169. [Google Scholar] [CrossRef]
40. Wang CY, Liao HY, Yeh IH. Designing network design strategies through gradient path analysis. arXiv:2211.04800. 2022. [Google Scholar]
41. Lee Y, Hwang JW, Lee S, Bae Y, Park J. An energy and GPU-computation efficient backbone network for real-time object detection. In: 2019 IEEE/CVF Conference on Computer Vision and Pattern Recognition Workshops (CVPRW); 2019 Jun 16–17; Long Beach, CA, USA. p. 752–60. doi:10.1109/cvprw.2019.00103. [Google Scholar] [CrossRef]
42. Vaswani A, Shazeer N, Parmar N, Uszkoreit J, Jones L, Gomez AN, et al. Attention is all you need. In: Advances in Neural Information Processing Systems 30 (NIPS 2017); 2017 Dec 4–9; Long Beach, CA, USA. p. 1–11. [Google Scholar]
43. Wang J, Sun K, Cheng T, Jiang B, Deng C, Zhao Y, et al. Deep high-resolution representation learning for visual recognition. IEEE Trans Pattern Anal Mach Intell. 2021;43(10):3349–64. doi:10.1109/TPAMI.2020.2983686. [Google Scholar] [PubMed] [CrossRef]
44. Zhao Y, Lv W, Xu S, Wei J, Wang G, Dang Q, et al. DETRs beat YOLOs on real-time object detection. In: 2024 IEEE/CVF Conference on Computer Vision and Pattern Recognition (CVPR); 2024 Jun 16–22; Seattle, WA, USA. p. 16965–74. doi:10.1109/CVPR52733.2024.01605. [Google Scholar] [CrossRef]
45. Zhang X, Zhou X, Lin M, Sun J. ShuffleNet: an extremely efficient convolutional neural network for mobile devices. In: Proceedings of the IEEE Conference on Computer Vision and Pattern Recognition; 2018 Jun 18–22; Salt Lake City, UT, USA. p. 6848–56. [Google Scholar]
46. Pan X, Ge C, Lu R, Song S, Chen G, Huang Z, et al. On the integration of self-attention and convolution. In: Proceedings of the IEEE/CVF Conference on Computer Vision and Pattern Recognition; 2022 Jun 18–24; New Orleans, LA, USA. p. 815–25. [Google Scholar]
47. Misra D, Nalamada T, Arasanipalai AU, Hou Q. Rotate to attend: convolutional triplet attention module. In: 2021 IEEE Winter Conference on Applications of Computer Vision (WACV); 2021 Jan 3–8; Waikoloa, HI, USA. p. 3138–47. doi:10.1109/WACV48630.2021.00318. [Google Scholar] [CrossRef]
48. Chen H, Lin LL, Ding JH. YOLOv10: real-time end-to-end object detection. arXiv:2405.14458. 2024. [Google Scholar]
Cite This Article
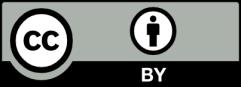
This work is licensed under a Creative Commons Attribution 4.0 International License , which permits unrestricted use, distribution, and reproduction in any medium, provided the original work is properly cited.