Open Access
ARTICLE
MLRT-UNet: An Efficient Multi-Level Relation Transformer Based U-Net for Thyroid Nodule Segmentation
1 Department of Computer Science and Engineering, RMK College of Engineering and Technology, Tiruvallur, 601206, India
2 School of Computer Science and Engineering, Vellore Institute of Technology, Chennai, 600127, India
* Corresponding Author: Kaku Haribabu. Email:
Computer Modeling in Engineering & Sciences 2025, 143(1), 413-448. https://doi.org/10.32604/cmes.2025.059406
Received 07 October 2024; Accepted 07 February 2025; Issue published 11 April 2025
Abstract
Thyroid nodules, a common disorder in the endocrine system, require accurate segmentation in ultrasound images for effective diagnosis and treatment. However, achieving precise segmentation remains a challenge due to various factors, including scattering noise, low contrast, and limited resolution in ultrasound images. Although existing segmentation models have made progress, they still suffer from several limitations, such as high error rates, low generalizability, overfitting, limited feature learning capability, etc. To address these challenges, this paper proposes a Multi-level Relation Transformer-based U-Net (MLRT-UNet) to improve thyroid nodule segmentation. The MLRT-UNet leverages a novel Relation Transformer, which processes images at multiple scales, overcoming the limitations of traditional encoding methods. This transformer integrates both local and global features effectively through self-attention and cross-attention units, capturing intricate relationships within the data. The approach also introduces a Co-operative Transformer Fusion (CTF) module to combine multi-scale features from different encoding layers, enhancing the model’s ability to capture complex patterns in the data. Furthermore, the Relation Transformer block enhances long-distance dependencies during the decoding process, improving segmentation accuracy. Experimental results show that the MLRT-UNet achieves high segmentation accuracy, reaching 98.2% on the Digital Database Thyroid Image (DDT) dataset, 97.8% on the Thyroid Nodule 3493 (TG3K) dataset, and 98.2% on the Thyroid Nodule3K (TN3K) dataset. These findings demonstrate that the proposed method significantly enhances the accuracy of thyroid nodule segmentation, addressing the limitations of existing models.Keywords
Cite This Article
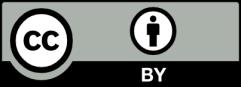
This work is licensed under a Creative Commons Attribution 4.0 International License , which permits unrestricted use, distribution, and reproduction in any medium, provided the original work is properly cited.